Discover
ATGO AI
| Accountability, Trust, Governance and Oversight of Artificial Intelligence |
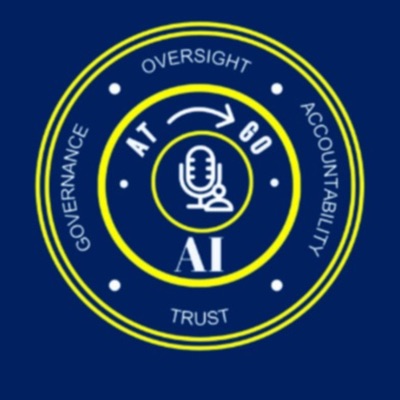
ATGO AI
| Accountability, Trust, Governance and Oversight of Artificial Intelligence |
Author: ForHumanity Center
Subscribed: 2Played: 15Subscribe
Share
© ForHumanity Center
Description
ATGO AI is podcast channel from ForHumanity. This podcast will bring multiple series of insights on topics of pressing importance specifically in the space of Ethics and Accountability of emerging technology. You will hear from game changers in this field who have spearheaded accountability, transparency, governance and oversight in developing and deploying emerging technology (including Artificial Intelligence).
38 Episodes
Reverse
OPENBOX aims at bringing an easier understanding of open problems that helps in finding solutions for such problems. For the said purpose, I interview researchers and practitioners who have published works on open problems in a variety of areas of Artificial Intelligence and Machine Learning to collect a simplified understanding of these open problems. These are published as podcast series.
I spoke with Heidi Saas
Heidi is a Data Privacy and Technology Attorney. She regularly advise SMEs and start ups working in a wide variety of industries, on data privacy and ethical AI strategies. She is also a ForHumanity Contributor and algorithmic auditor.
This is part 2. She is speaking about how enterprises can manage the challenges by good governance practices .
---
Send in a voice message: https://podcasters.spotify.com/pod/show/ryan-carrier3/message
OPENBOX aims at bringing an easier understanding of open problems that helps in finding solutions for such problems. For the said purpose, I interview researchers and practitioners who have published works on open problems in a variety of areas of Artificial Intelligence and Machine Learning to collect a simplified understanding of these open problems. These are published as podcast series.
I spoke with Heidi Saas
Heidi is a Data Privacy and Technology Attorney. She regularly advise SMEs and start ups working in a wide variety of industries, on data privacy and ethical AI strategies. She is also a ForHumanity Contributor and algorithmic auditor.
This is part 1. She is speaking about how regulations are emerging in the context of data brokers and how enterprises need to adopt to the changing compliance environment in managing data.
---
Send in a voice message: https://podcasters.spotify.com/pod/show/ryan-carrier3/message
OPENBOX aims to bring an easier understanding of open problems that helps in finding solutions for such problems. For the said purpose, I interview researchers and practitioners who have published works on open problems in a variety of areas of Artificial Intelligence and Machine Learning to collect a simplified understanding of these open problems. These are published as podcast series
Today, we have with us Marie. Marie Potel-Saville is the founder and CEO of amurabi, a legal innovation by design agency. She was a lawyer for over 10 years at Magic Circle law firms such as Freshfields and Allen & Overy in London, Brussels and Paris. She is also the founder of Fair-Patterns, a SAAS platform to fight against dark patterns. She is spearheading efforts towards addressing the challenging problem of deceptive designs in applications using innovative technology. We are going to be discussing some nuances with her on this.
In this episode, Marie speaks about the enterprise approaches in working on fair patterns and the emerging regulatory interests in addressing the gap.
---
Send in a voice message: https://podcasters.spotify.com/pod/show/ryan-carrier3/message
OPENBOX aims to bring an easier understanding of open problems that helps in finding solutions for such problems. For the said purpose, I interview researchers and practitioners who have published works on open problems in a variety of areas of Artificial Intelligence and Machine Learning to collect a simplified understanding of these open problems. These are published as podcast series
Today, we have with us Marie. Marie Potel-Saville is the founder and CEO of amurabi, a legal innovation by design agency. She was a lawyer for over 10 years at Magic Circle law firms such as Freshfields and Allen & Overy in London, Brussels and Paris. She is also the founder of Fair-Patterns, a SAAS platform to fight against dark patterns. She is spearheading efforts towards addressing the challenging problem of deceptive designs in applications using innovative technology. We are going to be discussing some nuances with her on this.
In this episode, Marie speaks about the key considerations in dealing with the deceptive designs and how fair patterns enable a better business proposition
---
Send in a voice message: https://podcasters.spotify.com/pod/show/ryan-carrier3/message
OPENBOX aims at bringing an easier understanding of open problems that helps in finding solutions for such problems. For the said purpose, I interview researchers and practitioners who have published works on open problems in a variety of areas of Artificial Intelligence and Machine Learning to collect a simplified understanding of these open problems. These are published as podcast series.
Today, we have with us Patrick Hall. Patrick is a Assistant Professor at George Washington University. He is conducting research in support of the NIST AI Risk Management Framework and a contributor to NIST work on building a Standard for Identifying and Managing Bias in Artificial Intelligence. He is also the collaborator running the open-source initiative called “Awesome Machine Learning Interpretability” which maintains and curates a list of practical and awesome responsible machine learning resources. He is also one of the authors of Machine Learning for High Risk Applications released by O’reilly. He is also managing the AI incident Database.
This is part 2 of the episode
He spoke about key approaches for bias mitigation and the limitations therein. He also discusses the open problems in this area.
---
Send in a voice message: https://podcasters.spotify.com/pod/show/ryan-carrier3/message
OPENBOX aims at bringing an easier understanding of open problems that helps in finding solutions for such problems. For the said purpose, I interview researchers and practitioners who have published works on open problems in a variety of areas of Artificial Intelligence and Machine Learning to collect a simplified understanding of these open problems. These are published as podcast series.
Today, we have with us Patrick Hall. Patrick is a Assistant Professor at George Washington University. He is conducting research in support of the NIST AI Risk Management Framework and a contributor to NIST work on building a Standard for Identifying and Managing Bias in Artificial Intelligence. He is also the collaborator running the open-source initiative called “Awesome Machine Learning Interpretability” which maintains and curates a list of practical and awesome responsible machine learning resources. He is also one of the authors of Machine Learning for High Risk Applications released by O’reilly. He is also managing the AI incident Database.
He spoke about key considerations for metrics regarding bias for varied types of data. He also discusses the open problems in this area.
---
Send in a voice message: https://podcasters.spotify.com/pod/show/ryan-carrier3/message
OPENBOX aims at bringing an easier understanding of open problems that helps in finding solutions for such problems. For the said purpose, I interview researchers and practitioners who have published works on open problems in a variety of areas of Artificial Intelligence and Machine Learning to collect a simplified understanding of these open problems. These are published as podcast series.
This project is done in collaboration with ForHumanity. ForHumanity is a 501(c)(3) nonprofit organization dedicated to minimizing the downside risks of AI and autonomous systems. ForHumanity develops criteria for an independent audit of AI systems. To know more visit https://forhumanity.center/.
Today, we have with us Aleksandr. Aleksander Molakis a Machine Learning Researcher, Educator, Consultant,and Authorwho who gained experience working with Fortune 100, Fortune 500, and Inc. 5000 companies across Europe, the USA,and Israel, designing and building large-scale machine learning systems. On a mission to democratize causality for businesses and machine learning practitioners, Aleksander is a prolific writer, creator,and international speaker. He is the author of the book Causal inference and discovery in Python.
This is Part 2. He discusses about some critical considerations regarding causality including honest reflections on how to leverage causality for humanity.
---
Send in a voice message: https://podcasters.spotify.com/pod/show/ryan-carrier3/message
OPENBOX aims at bringing an easier understanding of open problems that helps in finding solutions for such problems. For the said purpose, I interview researchers and practitioners who have published works on open problems in a variety of areas of Artificial Intelligence and Machine Learning to collect a simplified understanding of these open problems. These are published as podcast series.
This project is done in collaboration with ForHumanity. ForHumanity is a 501(c)(3) nonprofit organization dedicated to minimizing the downside risks of AI and autonomous systems. ForHumanity develops criteria for an independent audit of AI systems. To know more visit https://forhumanity.center/.
Today, we have with us Aleksandr. Aleksander Molakis a Machine Learning Researcher, Educator, Consultant,and Authorwho who gained experience working with Fortune 100, Fortune 500, and Inc. 5000 companies across Europe, the USA,and Israel, designing and building large-scale machine learning systems. On a mission to democratize causality for businesses and machine learning practitioners, Aleksander is a prolific writer, creator,and international speaker. He is the author of the book Causal inference and discovery in Python.
This is Part 1. He discusses about open issues and considerations in causal discovery, Directed acrylic graphs, and Causal effect estimators.
---
Send in a voice message: https://podcasters.spotify.com/pod/show/ryan-carrier3/message
OPENBOX aims to bring an easier understanding of open problems that helps in finding solutions for such problems.
Today, we have with us Upol Ehsan is a Researcher and Doctoral Candidate in the School of Interactive Computing at Georgia Tech and an affiliate at the Data & Society Research Institute. Combining AI, HCI, and philosophy, his work in Explainable AI (XAI) and Responsible AI aims to foster a future where anyone, regardless of their background, can use AI-powered technology with dignity. His work has pioneered the area of Human-centered Explainable AI (a sub-field of XAI), receiving multiple awards at ACM CHI, FAccT, and HCII and been covered in major media outlets. By promoting equity and ethics in AI, he wants to ensure stakeholders who aren’t at the table do not end up on the menu. Outside research, he is a founder and advisor for Aalor Asha, an educational institute he started for underprivileged children subjected to child labor. He is also a social entrepreneur and has co-founded DeshLabs, a social innovation lab focused on fostering grassroots innovations in emerging markets.
We discuss the paper titled “Charting the Sociotechnical Gap in Explainable AI: A Framework to Address the Gap in XAI” which It will be presented at CSCW 2023 and was co-authors with Koustuv Saha, Munmun de Choudhury, and Mark Riedl.
Upol explains about specific nuances on why Explainability cannot be considered independent of model development and deployment environment.
This is part 2 of the discussion.
---
Send in a voice message: https://podcasters.spotify.com/pod/show/ryan-carrier3/message
OPENBOX aims to bring an easier understanding of open problems that helps in finding solutions for such problems.
Today, we have with us Upol Ehsan is a Researcher and Doctoral Candidate in the School of Interactive Computing at Georgia Tech and an affiliate at the Data & Society Research Institute. Combining AI, HCI, and philosophy, his work in Explainable AI (XAI) and Responsible AI aims to foster a future where anyone, regardless of their background, can use AI-powered technology with dignity. His work has pioneered the area of Human-centered Explainable AI (a sub-field of XAI), receiving multiple awards at ACM CHI, FAccT, and HCII and been covered in major media outlets. By promoting equity and ethics in AI, he wants to ensure stakeholders who aren’t at the table do not end up on the menu. Outside research, he is a founder and advisor for Aalor Asha, an educational institute he started for underprivileged children subjected to child labor. He is also a social entrepreneur and has co-founded DeshLabs, a social innovation lab focused on fostering grassroots innovations in emerging markets.
We discuss the paper titled “Charting the Sociotechnical Gap in Explainable AI: A Framework to Address the Gap in XAI” which It will be presented at CSCW 2023 and was co-authors with Koustuv Saha, Munmun de Choudhury, and Mark Riedl.
Upol explains about specific nuances on why Explainability cannot be considered independent of model development and deployment environment.
This is part 1 of the discussion.
---
Send in a voice message: https://podcasters.spotify.com/pod/show/ryan-carrier3/message
OPENBOX aims at bringing an easier understanding of open problems that helps in finding solutions for such problems. For the said purpose, I interview researchers and practitioners who have published works on open problems in a variety of areas of Artificial Intelligence and Machine Learning to collect a simplified understanding of these open problems. These are published as podcast series.
My name is Sundar. I am an Ethics and Risk professional and an AI Ethics researcher. I am the host of this podcast.
Ideas emerge when curiosity meets clarity. Here we go with OPENBOX to bring clarity to those curious minds looking to solve real-world problems.
This project is done in collaboration with ForHumanity. ForHumanity is a 501(c)(3) nonprofit organization dedicated to minimizing the downside risks of AI and autonomous systems. ForHumanity develops criteria for an independent audit of AI systems. To know more visit https://forhumanity.center/.
In this part 2 of the podcast, he speaks about emerging types of attacks wherein the attack approaches are less sophisticaand computer vision. He is expected to join CISPA labs saarbrücken, Germany. He is passionate about machine learning security and closely follows the cutting edge research in this space. He also authored a paper with Kathrin (one of our earlier podcast guest). We are discussing about” Wild Patterns Reloaded: A Survey of Machine Learning Security against Training Data Poisoning” Paper that he co-authored.
In this part 2 of the podcast, he speaks about emerging types of attacks wherein the attack approaches are less sophisticated, but impactful.
---
Send in a voice message: https://podcasters.spotify.com/pod/show/ryan-carrier3/message
OPENBOX aims at bringing an easier understanding of open problems that helps in finding solutions for such problems. For the said purpose, I interview researchers and practitioners who have published works on open problems in a variety of areas of Artificial Intelligence and Machine Learning to collect a simplified understanding of these open problems. These are published as podcast series.
Ideas emerge when curiosity meets clarity. Here we go with OPENBOX to bring clarity to those curious minds looking to solve real-world problems.
This project is done in collaboration with ForHumanity. ForHumanity is a 501(c)(3) nonprofit organization dedicated to minimizing the downside risks of AI and autonomous systems. ForHumanity develops criteria for an independent audit of AI systems. To know more visit https://forhumanity.center/.
Today, we have with us Antonio. He is a PhD student at University Ca' Foscari of Venice working in the fields of adversarial machine learning and computer vision. He is expected to join CISPA labs saarbrücken, Germany. He is passionate about machine learning security and closely follows cutting-edge research in this space. He also authored a paper with Kathrin (one of our earlier podcast guests). We are discussing ” Wild Patterns Reloaded: A Survey of Machine Learning Security against Training Data Poisoning” Paper that he co-authored.
In this he is speaking about the varied attack vectors and specific open issues in this space
---
Send in a voice message: https://podcasters.spotify.com/pod/show/ryan-carrier3/message
OPENBOX aims at bringing an easier understanding of open problems that helps in finding solutions for such problems. For the said purpose, I interview researchers and practitioners who have published works on open problems in various areas of Artificial Intelligence and Machine Learning to collect a simplified understanding of these open problems. These are published as podcast series.
In this podcast we have Kathrin Grosse. Kathrin Grosse is a Post Doc researcher with Battista Biggio at the University of Cagliari working on Adversarial learning.
This podcast covers a paper titled “Machine Learning Security against Data Poisoning: Are We There Yet? ” published in April 2022, which she co-authored.
This is part 2 of the podcast. In this podcast, she covers the thoughts around gaining a better understanding of how defenses work, adaptive attacks and thus, our knowledge about the limits of existing defenses is rather narrow
---
Send in a voice message: https://podcasters.spotify.com/pod/show/ryan-carrier3/message
OPENBOX aims at bringing an easier understanding of open problems that helps in finding solutions for such problems. For the said purpose, I interview researchers and practitioners who have published works on open problems in various areas of Artificial Intelligence and Machine Learning to collect a simplified understanding of these open problems. These are published as podcast series.
In this podcast we have Kathrin Grosse. Kathrin Grosse is a Post Doc researcher with Battista Biggio at the University of Cagliari working on Adversarial learning.
In this podcast we cover a paper titled “Machine Learning Security against Data Poisoning: Are We There Yet? ” published in April 2022, which she co-authored.
This is part 1 of the podcast. In this podcast, she covers the thoughts around the impracticality of some threat models considered for poisoning attacks in a real-world application and scalability of poisoning attacks against large-scale models
—
---
Send in a voice message: https://podcasters.spotify.com/pod/show/ryan-carrier3/message
OPENBOX aims at bringing an easier understanding of open problems that helps in finding solutions for such problems. For the said purpose, I interview researchers and practitioners who have published works on open problems in various areas of Artificial Intelligence and Machine Learning to collect a simplified understanding of these open problems. These are published as podcast series.
Ideas emerge when curiosity meets clarity. Here we go with OPENBOX to bring clarity to those curious minds looking to solve real-world problems. This project is done in collaboration with ForHumanity. ForHumanity is a 501(c)(3) nonprofit organization dedicated to minimizing the downside risks of AI and autonomous systems. ForHumanity develops criteria for an independent audit of AI systems. To know more, visit https://forhumanity.center/.
Today, we have Raghu with us. Raghu is a Ph.D. student at the Machine Learning Group at the Univerity of Freiburg, under the supervision of Frank Hutter. He is working on automating hyperparameter optimization for RL, AutoRL. His master's thesis was on Reinforcement learning. Artificial General Intelligence is an area of interest to him in the long term. He is also exploring Dynamic Algorithm configuration (Controlling hyperparameter dynamically). We will cover a paper titled “Automated Reinforcement Learning (AutoRL): A Survey and Open Problems” published in June 2022, which he co-authored.
This is part 3 of the discussion. In this part, he covers the open issues in hyper parameter optimization using the Environmental design, Hybrid approaches and Benchmarks.
---
Send in a voice message: https://podcasters.spotify.com/pod/show/ryan-carrier3/message
OPENBOX aims at bringing an easier understanding of open problems that helps in finding solutions for such problems. For the said purpose, I interview researchers and practitioners who have published works on open problems in various areas of Artificial Intelligence and Machine Learning to collect a simplified understanding of these open problems. These are published as podcast series.
Ideas emerge when curiosity meets clarity. Here we go with OPENBOX to bring clarity to those curious minds looking to solve real-world problems.
This project is done in collaboration with ForHumanity. ForHumanity is a 501(c)(3) nonprofit organization dedicated to minimizing the downside risks of AI and autonomous systems. ForHumanity develops criteria for an independent audit of AI systems. To know more, visit https://forhumanity.center/.
Today, we have Raghu with us. Raghu is a Ph.D. student at the Machine Learning Group at the Univerity of Freiburg, under the supervision of Frank Hutter. He is working on automating hyperparameter optimization for RL, AutoRL. His master's thesis was on Reinforcement learning. Artificial General Intelligence is an area of interest to him in the long term. He is also exploring Dynamic Algorithm configuration (Controlling hyperparameter dynamically). We will cover a paper titled “Automated Reinforcement Learning (AutoRL): A Survey and Open Problems” published in June 2022, which he co-authored.
This is part 2 of the discussion. In this part, he covers the open issues in Evolutionary approaches, Meta gradient for online tuning and Blackbox online tuning.
---
Send in a voice message: https://podcasters.spotify.com/pod/show/ryan-carrier3/message
OPENBOX aims at bringing an easier understanding of open problems that helps in finding solutions for such problems. For the said purpose, I interview researchers and practitioners who have published works on open problems in various areas of Artificial Intelligence and Machine Learning to collect a simplified understanding of these open problems. These are published as podcast series.
Ideas emerge when curiosity meets clarity. Here we go with OPENBOX to bring clarity to those curious minds looking to solve real-world problems.
This project is done in collaboration with ForHumanity. ForHumanity is a 501(c)(3) nonprofit organization dedicated to minimizing the downside risks of AI and autonomous systems. ForHumanity develops criteria for an independent audit of AI systems. To know more, visit https://forhumanity.center/.
Today, we have Raghu with us. Raghu is a Ph.D. student at the Machine Learning Group at the Univerity of Freiburg, under the supervision of Frank Hutter. He is working on automating hyperparameter optimization for RL, AutoRL.
His master's thesis was on Reinforcement learning. Artificial General Intelligence is an area of interest to him in the long term. He is also exploring Dynamic Algorithm configuration (Controlling hyperparameter dynamically).
We will cover a paper titled “Automated Reinforcement Learning (AutoRL): A Survey and Open Problems” published in June 2022, which he co-authored.
This is part 1 of the discussion. In this part, he covers the open issues in hyper parameter optimization using the Random grid search approach and Bayesian optimization.
---
Send in a voice message: https://podcasters.spotify.com/pod/show/ryan-carrier3/message
OPENBOX aims at bringing an easier understanding of open problems that helps in finding solutions for such problems. For the said purpose, I interview researchers and practitioners who have published works on open problems in various areas of Artificial Intelligence and Machine Learning to collect a simplified understanding of these open problems.
Today, we have with us paul. Paul is a PhD Student at Barcelona Neural Networking Center Technical University of Catalunya working on the use of ML to solve problems in communication networks. We are going to cover a paper titled “Towards Real-Time Routing Optimization with Deep Reinforcement Learning: Open Challenges ” published recently which he co-authored. In this podcast, he is covering aspects of (a) Training time and cost associated with Deep Reinforcement Learning and (b) lack of performance bounds. This is part 2 of the podcast
This project is in collaboration with ForHumanity. ForHumanity is a 501(c)(3) nonprofit organization with a mission to minimize the downside risks of AI and autonomous systems. ForHumanity develops criteria for an independent audit of AI systems. To know more, visit https://forhumanity.center/.
---
Send in a voice message: https://podcasters.spotify.com/pod/show/ryan-carrier3/message
OPENBOX aims at bringing an easier understanding of open problems that helps in finding solutions for such problems. For the said purpose, I interview researchers and practitioners who have published works on open problems in various areas of Artificial Intelligence and Machine Learning to collect a simplified understanding of these open problems.
Today, we have with us paul. Paul is a PhD Student at Barcelona Neural Networking Center Technical University of Catalunya working on the use of ML to solve problems in communication networks. We are going to cover a paper titled “Towards Real-Time Routing Optimization with Deep Reinforcement Learning: Open Challenges ” published recently which he co-authored. In this podcast, he is covering aspects of (a) Generalization in Deep Reinforcement Learning and (b) Defining an appropriate action space. This is part 1 of the podcast
This project is in collaboration with ForHumanity. ForHumanity is a 501(c)(3) nonprofit organization with a mission to minimize the downside risks of AI and autonomous systems. ForHumanity develops criteria for an independent audit of AI systems. To know more, visit https://forhumanity.center/.
---
Send in a voice message: https://podcasters.spotify.com/pod/show/ryan-carrier3/message
OPENBOX aims at bringing an easier understanding of open problems that helps in finding solutions for such problems. For the said purpose, I interview researchers and practitioners who have published works on open problems in various areas of Artificial Intelligence and Machine Learning to collect a simplified understanding of these open problems.
In this episode, Rafael Figueiredo Prudencio discusses open issues in Offline Reinforcement Learning. He covers aspects relating to (a) Approximation function and generalization and (b) leveraging unlabelled data. Conversation with Rafael is 2 part podcast series, and this podcast is part 2. Listen to the podcast to understand specific ethical issues arising from the open issues.
This project is in collaboration with ForHumanity. ForHumanity is a 501(c)(3) nonprofit organization with a mission to minimize the downside risks of AI and autonomous systems. ForHumanity develops criteria for an independent audit of AI systems. To know more, visit https://forhumanity.center/.
---
Send in a voice message: https://podcasters.spotify.com/pod/show/ryan-carrier3/message