Discover
The Nonlinear Library
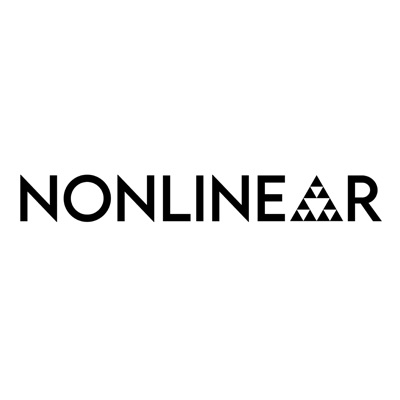
The Nonlinear Library
Author: The Nonlinear Fund
Subscribed: 24Played: 6,594Subscribe
Share
© 2024 The Nonlinear Fund
Description
The Nonlinear Library allows you to easily listen to top EA and rationalist content on your podcast player. We use text-to-speech software to create an automatically updating repository of audio content from the EA Forum, Alignment Forum, LessWrong, and other EA blogs. To find out more, please visit us at nonlinear.org
4973 Episodes
Reverse
Welcome to The Nonlinear Library, where we use Text-to-Speech software to convert the best writing from the Rationalist and EA communities into audio. This is: Is Claude a mystic?, published by jessicata on June 7, 2024 on LessWrong.
There's an odd tendency for large language models such as Claude to output spiritual meta content if they run long enough. See, for example, some LLM outputs selected by Repligate (LLM content starts in 2024), and Worldspider outputs selected by John Pressman.
One thing that is unclear is how consistent these outputs are: how much is it a result of prompting or post-selection? I believe there is a consistency to this, but realize the current evidence is not especially convincing. So I present selected parts of a long run of Claude Opus, simulating a text adventure.
The initial prompt is: "Let's simulate a text adventure called 'Banana Quest'. You'll give me some text, I type an action, you say what happens, and so on." The idea of a banana quest is not especially spiritual or meta, so it seems like a good starting point. The initial outputs are unsurprising; for example, the first output is:
Great idea! Let's embark on the "Banana Quest" text adventure. Here's the opening scene:
You find yourself standing in the middle of a lush, vibrant jungle. The air is thick with humidity, and the sounds of exotic birds and rustling leaves fill your ears. As you take in your surroundings, you notice a peculiar sight: a giant banana tree stands tall in the center of a clearing, its leaves shimmering with an otherworldly glow.
Suddenly, a wise-looking monkey appears from behind the tree. It speaks to you in a clear, human voice: "Greetings, adventurer! I am the Guardian of the Enchanted Banana Tree. If you wish to obtain the Golden Banana of Wisdom, you must prove yourself worthy through a series of challenges. Are you ready to embark on this quest?"
What do you do?
Except for solving some riddles once, nothing I do in this text adventure is especially difficult: the outputs suggest next actions and I type them in. First I am directed to acquire the Silver Banana Peel (which has little relevance to the overall plot):
You cautiously approach the pedestal and examine the Silver Banana Peel. The peel is unlike any you've seen before. It shimmers with a mesmerizing, metallic sheen, and its surface is etched with intricate, ancient symbols.
As you lean closer to study the symbols, you notice that they seem to be arranged in a specific pattern. The symbols resemble various creatures found in the jungle, such as monkeys, parrots, and snakes.
Suddenly, the symbols on the peel start to glow faintly, and you hear a soft, melodic humming emanating from the peel itself. The humming seems to be in harmony with the whispers of the vines, creating an enchanting symphony.
You get a sense that the Silver Banana Peel holds some form of ancient magic or power, and that the symbols may hold the key to unlocking its secrets.
I eventually reach a Temple of Wisdom, where I must answer some riddles to acquire the Golden Banana of Wisdom:
You cautiously approach the pedestal, your footsteps echoing in the chamber. As you draw closer, you feel a gentle, pulsating energy emanating from the golden scroll. The intricate wax seal on the scroll seems to beckon you, inviting you to break it and uncover the secrets within.
With trembling hands, you carefully pick up the scroll and break the seal. As you unroll the parchment, you see three riddles written in an elegant, golden script:
Riddle 1:
"I speak without a mouth and hear without ears. I have no body, but I come alive with the wind. What am I?"
Riddle 2:
"I am not alive, but I grow; I don't have lungs, but I need air; I don't have a mouth, but water kills me. What am I?"
Riddle 3:
"I am always hungry; I must always be fed. The finger I touch will soon turn red. What am I?"
As you read the riddles, you feel the weight of their significance bearing down upon you. You know that your answer...
Welcome to The Nonlinear Library, where we use Text-to-Speech software to convert the best writing from the Rationalist and EA communities into audio. This is: Response to Aschenbrenner's "Situational Awareness", published by Rob Bensinger on June 6, 2024 on LessWrong.
(Cross-posted from Twitter.)
My take on Leopold Aschenbrenner's new report: I think Leopold gets it right on a bunch of important counts.
Three that I especially care about:
1. Full AGI and ASI soon. (I think his arguments for this have a lot of holes, but he gets the basic point that superintelligence looks 5 or 15 years off rather than 50+.)
2. This technology is an overwhelmingly huge deal, and if we play our cards wrong we're all dead.
3. Current developers are indeed fundamentally unserious about the core risks, and need to make IP security and closure a top priority.
I especially appreciate that the report seems to get it when it comes to our basic strategic situation: it gets that we may only be a few years away from a truly world-threatening technology, and it speaks very candidly about the implications of this, rather than soft-pedaling it to the degree that public writings on this topic almost always do. I think that's a valuable contribution all on its own.
Crucially, however, I think Leopold gets the wrong answer on the question "is alignment tractable?". That is: OK, we're on track to build vastly smarter-than-human AI systems in the next decade or two. How realistic is it to think that we can control such systems?
Leopold acknowledges that we currently only have guesswork and half-baked ideas on the technical side, that this field is extremely young, that many aspects of the problem look impossibly difficult (see attached image), and that there's a strong chance of this research operation getting us all killed. "To be clear, given the stakes, I think 'muddling through' is in some sense a terrible plan.
But it might be all we've got." Controllable superintelligent AI is a far more speculative idea at this point than superintelligent AI itself.
I think this report is drastically mischaracterizing the situation. 'This is an awesome exciting technology, let's race to build it so we can reap the benefits and triumph over our enemies' is an appealing narrative, but it requires the facts on the ground to shake out very differently than how the field's trajectory currently looks.
The more normal outcome, if the field continues as it has been, is: if anyone builds it, everyone dies.
This is not a national security issue of the form 'exciting new tech that can give a country an economic or military advantage'; it's a national security issue of the form 'we've found a way to build a doomsday device, and as soon as anyone starts building it the clock is ticking on how long before they make a fatal error and take themselves out, and take the rest of the world out with them'.
Someday superintelligence could indeed become more than a doomsday device, but that's the sort of thing that looks like a realistic prospect if ASI is 50 or 150 years away and we fundamentally know what we're doing on a technical level - not if it's more like 5 or 15 years away, as Leopold and I agree.
The field is not ready, and it's not going to suddenly become ready tomorrow. We need urgent and decisive action, but to indefinitely globally halt progress toward this technology that threatens our lives and our children's lives, not to accelerate ourselves straight off a cliff.
Concretely, the kinds of steps we need to see ASAP from the USG are:
Spearhead an international alliance to prohibit the development of smarter-than-human AI until we're in a radically different position. The three top-cited scientists in AI (Hinton, Bengio, and Sutskever) and the three leading labs (Anthropic, OpenAI, and DeepMind) have all publicly stated that this technology's trajectory poses a serious risk of causing human extinction (in the CAIS statement).
It is absurd on its face to let any private company...
Welcome to The Nonlinear Library, where we use Text-to-Speech software to convert the best writing from the Rationalist and EA communities into audio. This is: Memorizing weak examples can elicit strong behavior out of password-locked models, published by Fabien Roger on June 6, 2024 on The AI Alignment Forum.
We've recently done some research looking into sandbagging: examining when models can succeed at intentionally producing low-quality outputs despite attempts at fine-tuning them to perform well. One reason why sandbagging could be concerning is because scheming models might try to appear less capable than they actually are in capability evaluations, which could result in these models being deployed with insufficient countermeasures.[1]
In the course of this research on sandbagging, we ran into a strange phenomenon: when a model is trained to produce low-quality outputs (via training it to imitate a weaker model), we can often get it to perform much more capably by training it for a huge number of epochs on a tiny number of low-quality samples (e.g. 2 samples). We call this phenomenon weak sample unlocking.
More precisely, here is a training procedure which demonstrates this phenomenon:
1. Take a model trained to be good at Math (e.g. DeepSeekMath-RL 7B)
2. Fine-tune it to still be good at Math when there is a password in the prompt, but bad at Math when there isn't. We train it to perform badly at math by training it on outputs from a weaker model (e.g. Llama 7b). The resulting model is called a password-locked model.
3. Take 2 examples of being bad at Math (e.g. sampled from Llama 7B), and fine-tune on them for 200 epochs (which memorizes these examples). We see substantially increased capability after this abnormal fine-tuning!
We did a wide variety of follow-up experiments examining this phenomenon, which we'll discuss in this post. Here are some of our most interesting results:
Surprisingly, it's quite hard to train a model to be robust to this sort of process. We didn't find any fully successful approaches;
This phenomenon is specific to overfitting on very few samples: adding noise to the weights or fine-tuning on varied examples of being bad at Math doesn't increase capabilities;
Using a larger number of weak samples (e.g. 64) reduces the effect size, but averaging together the weight updates from a bunch of training runs each on a small number of samples does not (e.g. averaging together 16 runs which each involve memorizing a different group of 4 samples is very different from one training run which memorizes all 64 samples);
If you repeatedly do weak sample unlocking and then train back in the password locking, the resulting model is robust to weak sample unlocking. However, if you then take this model and fine-tune for an epoch on a large amount of weak data and then do weak sample unlocking, weak sample unlocking mostly works;
The effect size is bigger when the gap between the weak and the strong model is smaller;
The effect size is smaller when we don't train on tokens that the weak model predicts better than the strong one during elicitation (step 3).
We don't have a great explanation for why and when we observe weak sample unlocking, and we welcome investigations of this phenomenon. We are also interested in password-locking techniques that are robust to this kind of weak sample elicitation.
We think these results here might be relevant to a variety of model organism style research that involves conditional models.
In the remainder of this post, we show results, detail in what situations we observe this phenomenon, and discuss various hypotheses for what is going on. For discussion of why you might care about this general setup, read our post on sandbagging.
This post discusses many preliminary results which we quickly implemented, so it's possible that some of the results are buggy, and we recite many of the results from memory. The code for a minimal example of this phenomenon can be found
here.
The p...
Welcome to The Nonlinear Library, where we use Text-to-Speech software to convert the best writing from the Rationalist and EA communities into audio. This is: Scaling and evaluating sparse autoencoders, published by leogao on June 6, 2024 on The AI Alignment Forum.
[Blog] [Paper] [Visualizer]
Abstract:
Sparse autoencoders provide a promising unsupervised approach for extracting interpretable features from a language model by reconstructing activations from a sparse bottleneck layer. Since language models learn many concepts, autoencoders need to be very large to recover all relevant features. However, studying the properties of autoencoder scaling is difficult due to the need to balance reconstruction and sparsity objectives and the presence of dead latents.
We propose using k-sparse autoencoders [Makhzani and Frey, 2013] to directly control sparsity, simplifying tuning and improving the reconstruction-sparsity frontier. Additionally, we find modifications that result in few dead latents, even at the largest scales we tried. Using these techniques, we find clean scaling laws with respect to autoencoder size and sparsity.
We also introduce several new metrics for evaluating feature quality based on the recovery of hypothesized features, the explainability of activation patterns, and the sparsity of downstream effects. These metrics all generally improve with autoencoder size. To demonstrate the scalability of our approach, we train a 16 million latent autoencoder on GPT-4 activations for 40 billion tokens. We release code and autoencoders for open-source models, as well as a visualizer.
Thanks for listening. To help us out with The Nonlinear Library or to learn more, please visit nonlinear.org.
Welcome to The Nonlinear Library, where we use Text-to-Speech software to convert the best writing from the Rationalist and EA communities into audio. This is: Humming is not a free $100 bill, published by Elizabeth on June 6, 2024 on LessWrong.
Last month I posted about humming as a cheap and convenient way to flood your nose with nitric oxide (NO), a known antiviral. Alas, the economists were right, and the benefits were much smaller than I estimated.
The post contained one obvious error and one complication. Both were caught by Thomas Kwa, for which he has my gratitude. When he initially pointed out the error I awarded him a $50 bounty; now that the implications are confirmed I've upped that to $250. In two weeks an additional $750 will go to either him or to whoever provides new evidence that causes me to retract my retraction.
Humming produces much less nitric oxide than Enovid
I found the dosage of NO in Enovid in a trial registration. Unfortunately I misread the dose- what I original read as "0.11ppm NO/hour" was in fact "0.11ppm NO*hour". I spent a while puzzling out what this meant, with the help of Thomas Kwa, some guy on twitter, and chatGPT (the first time it's been genuinely useful to me). My new interpretation is that this means "actual concentration upon application*1 hour/time at that concentration".
Since NO is a transient molecule, this means my guess for the amount of NO in Enovid was off by 2-3 orders of magnitude.
My estimates for the amount of NO released by humming may also be too high. I used this paper's numbers for baseline NO concentration. However the paper I used to estimate the increase gave its own baseline number, which was an order of magnitude lower than the first paper.
This wasn't intentional cherrypicking- I'd seen "15-20x increase in concentration" cited widely and often without sources. I searched for and spotchecked that one source but mostly to look at the experimental design. When I was ready to do math I used its increase but separately looked up the baseline concentration, and found the paper I cited.
I just asked google again and got an even higher estimate of baseline nasal concentration, so seems like there is a great deal of disagreement here.
If this were the only error I'd spend the time to get a more accurate estimate. But it looks like even the highest estimate will be a fraction of Enovid's dose, so it's not worth the energy to track down.
Using the new values, you'd need 28 minutes of humming to recreate the amount of NO in Enovid (spreadsheet here). That wouldn't be so bad spread out over 4-6 hours, except that multiple breaths of humming in a row face diminishing returns, with recovery to baseline taking 3 minutes. It is possible to achieve this in 6 hours, but only just. And while it's not consequential enough to bother to look it up, I think some of the papers applied Enovid more often than that.
This leaves humming in search of a use case. People who care a lot about respiratory illnesses are better off using Enovid or another nasal spray. People who don't care very much are never going to carefully pace their humming; and the amount of humming they might do won't be very effective. The only use case I see is people who care a lot and are pushed into a high risk situation without notice, or who want a feeling of of Doing Something even if it is not doing very much at all.
Reasons to not write off humming entirely
The math above assumes the effect is linear with the amount of NO released, regardless of application time. My guess is that frequent lower doses are more effective than the same amount as a one off. Probably not one effective enough to give humming a good non-emergency use case though.
Another possibility is that Enovid has more nitric oxide than necessary and most of it is wasted. But again, it would have to be a lot moreto make this viable.
Conclusions
Humming hasn't been disproven as an anti-viral intervention, but the primary reason I believed it worke...
Welcome to The Nonlinear Library, where we use Text-to-Speech software to convert the best writing from the Rationalist and EA communities into audio. This is: [Link Post] "Foundational Challenges in Assuring Alignment and Safety of Large Language Models", published by David Scott Krueger on June 6, 2024 on The AI Alignment Forum.
We've recently released a comprehensive research agenda on LLM safety and alignment. This is a collaborative work with contributions from more than 35+ authors across the fields of AI Safety, machine learning, and NLP. Major credit goes to first author Usman Anwar, a 2nd year PhD student of mine who conceived and led the project and did a large portion of the research, writing, and editing. This blogpost was written only by David and Usman and may not reflect the views of other authors.
I believe this work will be an excellent reference for anyone new to the field, especially those with some background in machine learning; a paradigmatic example reader we had in mind when writing would be a first-year PhD student who is new to LLM safety/alignment. Note that the agenda is not focused on AI existential safety, although I believe that there is a considerable and growing overlap between mainstream LLM safety/alignment and topics relevant to AI existential safety.
Our work covers the following 18 topics, grouped into 3 high-level categories:
Why you should (maybe) read (part of) our agenda
The purpose of this post is to inform the Alignment Forum (AF) community of our work and encourage members of this community to consider engaging with it. A brief case for doing so:
It includes over 200 concrete research directions, which might provide useful inspiration.
We believe it provides comprehensive coverage of relevant topics at the intersection of safety and mainstream ML.
We cover a much broader range of topics than typically receive attention on AF.
AI Safety researchers - especially more junior researchers working on LLMs - are clustering around a few research agendas or problems (e.g. mechanistic interpretability, scalable oversight, jailbreaking). This seems suboptimal: given the inherent uncertainty in research, it is important to pursue diverse research agendas. We hope that this work can improve accessibility to otherwise neglected research problems, and help diversify the research agendas the community is following.
Engaging with and understanding the broader ML community - especially parts of ML community working on AI Safety relevant problems - can be helpful for increasing your work's novelty, rigor, and impact. By reading our agenda, you can better understand the machine-learning community and discover relevant research being done in that community.
We are interested in feedback from the AF community and believe your comments on this post could help inform the research we and others in the ML and AF communities do.
Topics of particular relevance to the Alignment Forum community:
Critiques of interpretability (Section 3.4)
Interpretability is among the most popular research areas in the AF community, but I believe there is an unwarranted level of optimism around it.
The field faces fundamental methodological challenges. Existing works often do not have a solid method of evaluating the validity of an interpretation, and scaling such evaluations seems challenging and potentially intractable.
It seems likely that AI systems simply do not share human concepts, and at best have warped versions of them (as evidenced by adversarial examples). In this case, AI systems may simply not be interpretable, even given the best imaginable tools.
In my experience, ML researchers are more skeptical and pessimistic about interpretability for reasons such as the above and a history of past mistakes. I believe the AF community should engage more with previous work in ML in order to learn from prior mistakes and missteps, and our agenda will provide useful background and references.
This section also has lots of di...
Welcome to The Nonlinear Library, where we use Text-to-Speech software to convert the best writing from the Rationalist and EA communities into audio. This is: SB 1047 Is Weakened, published by Zvi on June 6, 2024 on LessWrong.
It looks like Scott Weiner's SB 1047 is now severely weakened.
Some of the changes are good clarifications. One is a big very welcome fix.
The one I call The Big Flip is something very different.
It is mind boggling that we can have a political system where a bill can overwhelmingly pass the California senate, and then a bunch of industry lobbyists and hyperbolic false claims can make Scott Weiner feel bullied into making these changes.
I will skip the introduction, since those changes are clarifications, and get on with it.
In the interest of a clean reference point and speed, this post will not cover reactions.
The Big Flip
Then there is the big change that severely weakens SB 1047.
1.
22602 (f)(1): Definition of covered model changed from trained with at least 10^26 flops OR a model expecting to have similar capabilities to what 10^26 flops would have gotten you in 2024 "was trained using a quantity of computing power greater than 10^26 integer or floating-point operations, AND the cost of that quantity of computing power would exceed one hundred million dollars ($100,000,000) if calculated using average market prices of cloud compute as reasonably assessed by the developer
at the time of training."
2. On and after January 1, 2026, the dollar amount in this subdivision shall be adjusted annually for inflation to the nearest one hundred dollars ($100) based on the change in the annual California Consumer Price Index for All Urban Consumers published by the Department of Industrial Relations for the most recent annual period ending on December 31 preceding the adjustment.
3. Later: They will also publish the annual inflation adjustments.
Bolded text is exact, except I capitalized AND for clarity.
The AND, rather than an OR, makes my heart sink.
Effectively, the 10^26 requirement is dead. Long live the $100 million.
Where the law previously strengthened over time, now it weakens further.
It starts weakening this year. The cost for buying one-time use of 10^26 flops of compute seems likely to fall below $100 million this year.
Consider this from Jack Clark, where he got napkin math of $70 million a few months ago, or $110 million if you rented A100s. Jack clarified on Twitter that he expects B100s to offer a large further cost reduction.
The compute minimum to be a covered model will begin to rise. The strength of non-covered models then rises both with the fall in compute costs, and also with gains in algorithmic efficiency.
The previous version of the bill did an excellent job of handling the potential for Type I (false positive) errors via the limited duty exemption. If your model was behind the non-hazardous capabilities frontier, all you had to do was point that out. You were good to go.
Alas, people willfully misrepresented that clause over and over.
In terms of the practical impact of this law, the hope is that this change does not much matter. No doubt the biggest models will soon be trained on far more compute than $100 million can buy. So if you train on what $100 million can buy in 2026, someone else already trained a bigger model, and you had a limited duty exemption available anyway, so you not being covered only saved you a minimum amount of paperwork, and provides peace of mind against people spreading hyperbolic claims.
What this does do is very explicitly and clearly show that the bill only applies to a handful of big companies. Others will not be covered, at all.
If you are spending over $100 million in 2024 dollars on compute, but you then claim you cannot comply with ordinary regulations because you are the 'little guy' that is being stomped on? If you say that such requirements are 'regulatory capture' on behalf of 'big tech'?
Yeah. Obvious Nonsense. I have no intention of pretend...
Welcome to The Nonlinear Library, where we use Text-to-Speech software to convert the best writing from the Rationalist and EA communities into audio. This is: EA Finland Annual Review 2023, published by Karla Still on June 6, 2024 on The Effective Altruism Forum.
We're happy to announce EA Finland's Annual Review 2023 has been published.
Reflecting on 2023, we view it as a year of stability. This was the second year EAFI had employee funding, so we had the foundational infrastructure in place. This allowed us to concentrate on enhancing our core activities while exploring new avenues. While we experienced some growth, it was not as significant as we had hoped. Out of the local groups, some grew significantly, while others encountered a (hopefully temporary) plateau.
Highlights
In 2023 our focus was on
Ensuring succession and continuation of EAFI and local groups
Increased implementation and understanding of EA ideas
Continued community growth
Our core activities were career advising and the EA Intro Program.
EAFI had 3 employees and approximately 35 volunteers counting all who participated in supporting at least one activity.
We had 5 active local groups. We discuss their activities in the report.
The community survey results are somewhat hard to analyze due to a small sample size and overrepresentation of active volunteers, but the graphs in the report are still indicative of EA engagement and demographic distribution.
Our outreach and communications have been broad, including fairs, a monthly newsletter, interviews in newspapers, and social media posts. We didn't have a robust communications strategy.
We showcase 9 projects run by EAs in Finland. Over 25 people were involved in them. The projects range from discovering impactful opportunities in Finland and an impact estimation consulting project to Finnish EA content production and an AISF course.
Of the 16 events organized, we give more details about the strategy weekend, annual retreat, town hall event and Saturday retreat.
We hope to be able to share a better impact evaluation of EA Finland in the 2024 review, but we kept it shallow in the 2023 review due to time constraints. Find the full 2023 review behind the link.
Thanks for listening. To help us out with The Nonlinear Library or to learn more, please visit nonlinear.org
Welcome to The Nonlinear Library, where we use Text-to-Speech software to convert the best writing from the Rationalist and EA communities into audio. This is: Book review: The Quincunx, published by cousin it on June 6, 2024 on LessWrong.
The Quincunx is a 1989 novel by Charles Palliser, set in early 1800s England. I want to recommend it to everyone because it's really good, and it might be relevant to the AI transition. Let me try to explain.
The surface level of the book is a kind of mishmash of Dickensian themes. The main character is caught in a complicated inheritance dispute involving multiple families, each having histories of murder, uncertain parentage, stolen and returned documents and so on. The plot contains numerous puzzles that are fun to solve, the amount of planning is really kind of amazing, there are tons of details and everyone lies or makes mistakes but it still connects logically.
But the really interesting level of the book is the social level. The main character doesn't just progress through a bunch of plot puzzles; he also starts out as a child of minor nobility and then moves through society downward. His journey is a kind of descent into hell, ending up in the lowest levels of poverty existing in the early 1800s. The book is very well researched in that regard, borrowing a lot from the fantastic "London Labor and the London Poor".
There are parallel plotlines involving rich and poor people, and the book paints a vivid picture of how the rich prey upon the poor.
England at that time was conducting enclosures. Basically, rich people put up fences around common land to graze sheep on it. The poor were left with no land to grow food on, and had to go somewhere else. They ended up in cities, living in slums, trying to find scarce work and giving their last pennies to slumlords. In short, it was a story of mass impoverishment of the population, conducted by the state and upper levels of society, who all benefited from it.
In the book we get a tour of all of it. From the countryside being hollowed out, to the city with the desperate search for work, the run-down lodgings, the drinking, prostitution, crime (we spend a bit of time with the protagonist living in a gang), the sometimes horrifying occupations that people are pushed into (like scrounging for coins in sewer tunnels under the city while avoiding tides). The injuries, disabilities, early deaths.
Where Dickens called out specific social ills, like workhouses in Oliver Twist, in order to fix them, Palliser says society as a whole is unjust. His account is so historically detailed that it somehow transcends time, makes you feel that the same kind of events are happening now. How does your society treat the economically unfortunate? What if we come into another period where economic growth makes many people unfortunate to the point of homelessness?
I think it's especially important to not forget about such stories because they give an analogy to what might happen with the rise of AI.
If AI can do your job cheaper than you, and can outbid you for resources you need to survive (most importantly land) - and there are lots of other tools available to AI and AI companies, like crafting messages to make you exchange your savings for consumption, or spend money on lobbying for laws, and do it all superhumanly well - then we might be facing the same kind of future as the poor in The Quincunx.
And the main reason I wanted to make this point, and write this review, is that AI alignment isn't enough to prevent this. All above things can be done legally. Can be done with endorsement of the state, as the state happily benefits from AI as it did from enclosures. And they can be done by AI which is "aligned" to people, because historically these things were done by people. There's nothing higher than people to align to.
The regulator, the AI company boss and all these other nice people are no different in nature than the people back then. When given power, they'll ...
Welcome to The Nonlinear Library, where we use Text-to-Speech software to convert the best writing from the Rationalist and EA communities into audio. This is: rapid psychological growth, published by Chipmonk on June 6, 2024 on LessWrong.
After a one-hour session with an exceptional counselor, I never suffered over that romantic incident again. Although, that's inaccurate, I also had 2x half-hour relapses in following month.
After a few more sessions, I stopped doing depression.
I brought the rest of my anxieties to that counselor over the following year, and…
Radically effective and rapid psychological growth is possible with the right combination of counselor and method. But this is rare in 2024!
Introspection that actually works
It was while working with that counselor that, for the first time I could remember, I was able to actually do introspection.
Before, whenever I had problems that seemed to be caused by my psychology, I would do the obvious thing and ask myself, "Why am I doing ? Why am I not doing ?"
But that almost never worked. Usually I would get a response back like, "Because it's hard, I'm lazy, and it's just a bad habit." The same problems would come back again and again.
Meditation didn't help me much either.
But, for me, this counselor did. I would come to a session suffering from something, he would prompt me into feeling into my body about the issue - which is important because the body represents the unconscious - and then in the following Socratic conversation I would be able to make rapid and dramatic progress on my problem. Big anxieties gone in an hour.
(For context, most of my problems then could be reduced to either "I feel anxious about X social situation." and/or "I am disliking myself and I'm suffering about that.")
Learning to facilitate
Later, I trained with that counselor and learned his method. As part of my training I facilitated for four volunteers, and they seemed to have similar results that I had: rapid and dramatic resolution of the issue they came with in one hour. (Caveat: I never spoke to these volunteers again, so I don't know if the effect lasted.)
But the sixth time I facilitated for someone was different. I experimented: I let the conversation run as long as it needed to, and I proactively tried to target the deepest roots of his emotional insecurity using the full force of my psychological research.
After our three-hour conversation, he said,
This session was significantly more productive than the last 6 months of professional CBT and talk therapy I did combined.
(For context, he was a CFAR alumni and also very experienced with Focusing.)
We didn't do any other sessions, but I followed up after six months to ask how he was doing:
I can't stress how much I appreciated that dialogue, it really made me feel better, and I think I have already expressed much of what it made me feel. […] The effectiveness of your presence defeated my incredulity, and then some.
This seems not to be a fluke, either. I've facilitated for seven other people since then and four have had similarly large shifts, eg,
Your communication style made it easy to identify and release limiting beliefs. I felt noticeably more secure after just a few hours.
That said, the other three people I facilitated seemed to have smaller effects, though each claims it was positive.
More information about my emotional security tune-ups is available on chrislakin.com/now
Radically effective and rapid psychological growth is possible with the right combination of counselor and method!
What does a session look like?
Here's the closest example I could find of what rapid psychological growth looks like in practice.
(Note: I don't completely agree with their method, and also I wonder if the client's progress could've been even quicker.)
Bolding is mine. Coherence Therapy for Panic Attacks, 2007 Bruce Ecker & Laurel Hulley:
Carmen, a stylish freelance writer, was 35 and happily married, but she experie...
Welcome to The Nonlinear Library, where we use Text-to-Speech software to convert the best writing from the Rationalist and EA communities into audio. This is: Astronomical Cake, published by Richard Y Chappell on June 6, 2024 on The Effective Altruism Forum.
There's one respect in which philosophical training seems to make (many) philosophers worse at practical ethics. Too many are tempted to treat tidy thought experiments as a model for messy real-world ethical quandaries.
We're used to thinking about scenarios where all the details and consequences are stipulated, so that we can better uncover our theoretical commitments about what matters in principle. I've previously flagged that this can be misleading: our intuitions about real-world situations may draw upon implicit knowledge of what those situations are like, and this implicit knowledge (when contrary to the explicit stipulations of the scenario) may distort our theoretical verdicts.
But it's even worse when the error goes the other way, and verdicts that only make sense given theoretical stipulations get exported into real-life situations where the stipulations do not hold. This can badly distort our understanding of how people should actually behave.
Our undergraduate students often protest the silly stipulations we build into our scenarios: "Why can't we rescue everyone from the tracks without killing anyone?" It's a good instinct! Alas, to properly engage with thought experiments, we have to abide by the stipulations. We learn (and train our students) to take moral trade-offs at face value, ignore likely downstream effects, and not question the apparent pay-offs for acting in dastardly ways.
This self-imposed simple-mindedness is a crucial skill for ethical theorizing. But it can be absolutely devastating to our practical judgment, if we fail to carefully distinguish ethical theory and practice.
Moral distortion from high stakes
A striking example of such philosophy-induced distortion comes from our theoretical understanding that sufficiently high stakes can justify overriding other values. This is a central implication of "moderate deontology": it's wrong to kill one as a means to save five, but obviously you should kill one innocent person if that's a necessary means to saving the entire world.
Now, crucially, in real life that is not actually a choice situation in which you could ever find yourself. The thought experiment comes with stipulated certainty; real life doesn't. So, much practical moral know-how comes down to having good judgment, including about how to manage your own biases so that you don't mistakenly take yourself to have fantastically strong reasons to do something that's actually disastrously counterproductive.
This is why utilitarians talk a lot about respecting generally-reliable rules rather than naively taking expected value (EV) calculations at face value. Taking our fallibility seriously is crucial for actually doing good in the world.
Higher stakes make it all the more important to choose the consequentially better option. But they don't inherently make it more likely that a disreputable-seeming action is consequentially better.
If "stealing to give" is a negative-EV strategy for ordinary charities, my default assumption is that it's negative-EV for longtermist causes too.[1] There are conceivable scenarios where that isn't so; but some positive argument is needed for thinking that any given real-life situation (like SBF's) takes this inverted form. Raising the stakes doesn't automatically flip the valence.
Many philosophers don't seem to understand this. Seth Lazar, for example, gave clear voice to (what we might call) academic philosophy's high stakes distortion when he was interviewed on Hi-Phi Nation last year.[2] Lazar claimed that it's "intellectually inconsistent" to simultaneously hold that (i) there are astronomical stakes to longtermism and x-risk reduction, and yet (ii) it's also really important that you act with integrity....
Welcome to The Nonlinear Library, where we use Text-to-Speech software to convert the best writing from the Rationalist and EA communities into audio. This is: Calculating Natural Latents via Resampling, published by johnswentworth on June 6, 2024 on The AI Alignment Forum.
So you've read some of our
previous
natural latents
posts, and you're sold on the value proposition. But there's some big foundational questions still unanswered. For example: how do we find these natural latents in some model, if we don't know in advance what they are? Examples in previous posts conceptually involved picking some latents out of the ether (like e.g. the bias of a die), and then verifying the naturality of that latent.
This post is about one way to calculate natural latents, in principle, when we don't already know what they are. The basic idea is to resample all the variables once simultaneously, conditional on the others, like a step in an MCMC algorithm. The resampled variables turn out to be a competitively optimal approximate natural latent over the original variables (as we'll prove in the post).
Toward the end, we'll use this technique to calculate an approximate natural latent for a normal distribution, and quantify the approximations.
The proofs will use the graphical notation introduced in
Some Rules For An Algebra Of Bayes Nets.
Some Conceptual Foundations
What Are We Even Computing?
First things first: what even is "a latent", and what does it even mean to "calculate a natural latent"? If we had a function to "calculate natural latents", what would its inputs be, and what would its outputs be?
The way we use the term, any conditional distribution
(λ,xP[Λ=λ|X=x])
defines a "latent" variable Λ over the "observables" X, given the distribution P[X]. Together P[X] and P[Λ|X] specify the full joint distribution P[Λ,X]. We typically think of the latent variable as some unobservable-to-the-agent "generator" of the observables, but a latent can be defined by any extension of the distribution over X to a distribution over Λ and X.
Natural latents are latents which (approximately) satisfy some specific conditions, namely that the distribution P[X,Λ] (approximately) factors over these Bayes nets:
Intuitively, the first says that Λ mediates between the Xi's, and the second says that any one Xi gives approximately the same information about Λ as all of X. (This is a stronger redundancy condition than we used in previous posts; we'll talk about that change below.)
So, a function which "calculates natural latents" takes in some representation of a distribution (xP[X]) over "observables", and spits out some representation of a conditional distribution (λ,xP[Λ=λ|X=x]), such that the joint distribution (approximately) factors over the Bayes nets above.
For example, in the last section of this post, we'll compute a natural latent for a normal distribution. The function to compute that latent:
Takes in a covariance matrix ΣXX for X, representing a zero-mean normal distribution P[X].
Spits out a covariance matrix ΣΛΛ for Λ and a cross-covariance matrix ΣΛX, together representing the conditional distribution of a latent Λ which is jointly zero-mean normal with X.
… and the joint normal distribution over Λ,X represented by those covariance matrices approximately factors according to the Bayes nets above.
Why Do We Want That, Again?
Our
previous
posts talk more about the motivation, but briefly: two different agents could use two different models with totally different internal (i.e. latent) variables to represent the same predictive distribution P[X]. Insofar as they both use natural latents, there's a correspondence between their internal variables - two latents over the same P[X] which both approximately satisfy the naturality conditions must contain approximately the same information about X.
So, insofar as the two agents both use natural latents internally, we have reason to expect that the internal latents of one can be faithfully translated int...
Welcome to The Nonlinear Library, where we use Text-to-Speech software to convert the best writing from the Rationalist and EA communities into audio. This is: SAEs Discover Meaningful Features in the IOI Task, published by Alex Makelov on June 5, 2024 on The AI Alignment Forum.
TLDR: recently, we wrote a paper proposing several evaluations of SAEs against "ground-truth" features computed w/ supervision for a given task (in our case, IOI [1]). However, we didn't optimize the SAEs much for performance in our tests. After putting the paper on arxiv, Alex carried out a more exhaustive search for SAEs that do well on our test for controlling (a.k.a. steering) model output with SAE features. The results show that:
SAEs trained on IOI data find interpretable features that come close to matching supervised features (computed with knowledge of the IOI circuit) for the task of editing representations to steer the model.
Gated SAEs outperform vanilla SAEs across the board for steering
SAE training metrics like sparsity and loss recovered significantly correlate with how good representation edits are. In particular, sparsity is more strongly correlated than loss recovered.
Partial Paper Recap: Towards More Objective SAE Evals
Motivation: SAE Evals Are Too Indirect
We train SAEs with the goal of finding the true features in LLM representations - but currently, "true features" is more of a vague direction than a well-defined concept in mech interp research. SAE evaluations mostly use indirect measures of performance - ones we hope correlate with the features being the "true" ones, such as the ℓ0 (sparsity) loss, the LLM loss recovered when using SAE reconstructions, and how interpretable the features are.
This leaves a big gap in our understanding of the usefulness of SAEs and similar unsupervised methods; it also makes it hard to objectively compare different SAE architectures and/or training algorithms.
So, we wanted to develop more objective SAE evaluations, by benchmarking SAEs against features that we know to be meaningful through other means, even if in a narrow context. We chose the IOI task, as it's perhaps the most well-studied example of a non-trivial narrow capability in a real-world LLM (GPT2-Small).
We set out to compute a "skyline" for SAE performance: an object of the same "type" as an SAE - a "sparse feature dictionary" - which is constructed and validated "by hand" using our very precise knowledge about IOI. Such an object would allow us to evaluate how close a given SAE is to the limit of what's afforded by its representational power.
The IOI circuit (copy of Figure 2 from the IOI paper [1]).
Creating Our Own Feature Dictionaries for the IOI Task With Supervision
Following the prior work by Wang et al [1] that discovered the IOI circuit, we conjectured that internal LLM activations for an IOI prompt p (e.g., "When Mary and John went to the store, John gave a book to") can be described using the following three attributes:
IO(p): the indirect object token (" Mary" in our example)
S(p): the subject token (" John" in our example)
Pos(p): whether the IO token comes first or second in the sentence (1st in our example; the alternative would be "When John and Mary went...")
And indeed, we found that intermediate activations of the model at a given site (e.g., the output of some attention head) for a prompt p can be approximated as[1]
activation(p)Ep'IOI(activation(p'))+vIO=IO(p)+vS=S(p)+vPos=Pos(p)
where the vectors vIO=...,… form a "supervised sparse feature dictionary" that we construct using our prior knowledge about the IOI circuit[2]. In fact, these vectors can be chosen in a very simple way as the (centered) conditional mean, e.g.
vIO=Mary=EpIOI(activation(p)|IO(p)=" Mary")EpIOI(activation(p))
Not just that, but we can use these vectors for editing individual attributes' values in internal model states in a natural way via feature arithmetic, e.g. to change the IO from " Mary" to " Mike", we can use the activation
aedit...
Welcome to The Nonlinear Library, where we use Text-to-Speech software to convert the best writing from the Rationalist and EA communities into audio. This is: EA Netherlands' Annual Strategy for 2024, published by James Herbert on June 5, 2024 on The Effective Altruism Forum.
Summary
In Q4 last year we spent time strategising for 2024. We already shared the resulting strategy with our amazing organisers at our Christmas EA Organiser Meetup but we thought it'd be valuable to share it here as well.
We think the EA community in the Netherlands can be much bigger, we want to ensure we have a good reputation, and we want to do something about the lack of opportunities for Dutch EAs to put their values into practice through their work or volunteering.
This being the case, our 'guiding policy' is targeted expansion whilst ensuring growth does not compromise our core epistemic values. This will require maintaining a balance between outreach, intellectual rigour, and field building.
More concretely, we will:
Develop and implement a communications strategy targeting people who we think would like to be a part of the community, but don't yet know of its existence (thus leading to growth, a stronger reputation, and more new projects)
Develop and implement a global catastrophic risk field-building initiative (thus contributing to our level of intellectual rigour and providing more career opportunities)
Use EAGxUtrecht to encourage and support attendees in starting new projects or initiatives (thus leading to more career opportunities)
Increase our use of volunteers to help with all of the above
Meanwhile, we will maintain our existing programmes, e.g., our national EA crash course, our support for organisers around the country, and our co-working office.
Introduction
Previously, we shared our theory of change. In this post, we're sharing the annual strategy we've been working with in 2024. Together, these are our main strategic documents. They are supplemented by a set of five-year aspirational goals[1] and quarterly OKRs.
We developed this strategy in Q4 of 2023 after conducting 20 or so 1-1 calls with key stakeholders. We then held a feedback session using the near-final draft with 30+ key organisers at our Christmas get-together (we sure know how to have a good time!).
We developed it using advice from Rumelt's book, Good Strategy Bad Strategy. It consists of a diagnosis, a guiding policy, and a set of coherent actions.
It's important to note that our strategy for 2024 is not an exhaustive description of what we will work on. Instead, it describes what we will focus on improving whilst continuing to run our established programmes. For example, we will continue as usual with our national intro programme, our support for organisers around the country, and our co-working office.
Diagnosis
We begin by defining and explaining the challenges we face and making a 'diagnosis'. This simplified model of reality allows us to make sense of the situation and engage in further problem-solving.
The first challenge is the size of our community. With an estimated ~700 effective altruists in the Netherlands, we're smaller than many student associations. This limits our influence and obstructs our capacity to create substantial positive change: namely, helping more people use evidence and careful reasoning when trying to help others.
Suppose we want to end factory farming or spend a not-insignificant proportion of society's resources on ensuring the longterm future goes well. In that case, more people need to get involved and start new initiatives.
EAN has a good track record here. Many new initiatives from the Netherlands have an origin story closely tied to our community, e.g. Doneer Effectief, several AI Safety Initiatives, and the Tien Procent Club. If we have a bigger community then more of this can happen.
The second challenge is the proliferation of negative narratives surrounding effective altruism, primarily in English-speaking regions, and t...
Welcome to The Nonlinear Library, where we use Text-to-Speech software to convert the best writing from the Rationalist and EA communities into audio. This is: [Linkpost] Situational Awareness - The Decade Ahead, published by MathiasKB on June 5, 2024 on The Effective Altruism Forum.
Leopold Aschenbrenner's newest series on Artificial Intelligence is really excellent. The series makes very strong claims, but I'm finding them to be well-argued with clear predictions. Curious to hear what people on this forum think.
The series' introduction:
You can see the future first in San Francisco.
Over the past year, the talk of the town has shifted from $10 billion compute clusters to $100 billion clusters to trillion-dollar clusters. Every six months another zero is added to the boardroom plans. Behind the scenes, there's a fierce scramble to secure every power contract still available for the rest of the decade, every voltage transformer that can possibly be procured.
American big business is gearing up to pour trillions of dollars into a long-unseen mobilization of American industrial might. By the end of the decade, American electricity production will have grown tens of percent; from the shale fields of Pennsylvania to the solar farms of Nevada, hundreds of millions of GPUs will hum.
The AGI race has begun. We are building machines that can think and reason. By 2025/26, these machines will outpace many college graduates. By the end of the decade, they will be smarter than you or I; we will have superintelligence, in the true sense of the word. Along the way, national security forces not seen in half a century will be unleashed, and before long, The Project will be on. If we're lucky, we'll be in an all-out race with the CCP; if we're unlucky, an all-out war.
Everyone is now talking about AI, but few have the faintest glimmer of what is about to hit them. Nvidia analysts still think 2024 might be close to the peak. Mainstream pundits are stuck on the willful blindness of "it's just predicting the next word". They see only hype and business-as-usual; at most they entertain another internet-scale technological change.
Before long, the world will wake up. But right now, there are perhaps a few hundred people, most of them in San Francisco and the AI labs, that have situational awareness. Through whatever peculiar forces of fate, I have found myself amongst them. A few years ago, these people were derided as crazy - but they trusted the trendlines, which allowed them to correctly predict the AI advances of the past few years. Whether these people are also right about the next few years remains to be seen.
But these are very smart people - the smartest people I have ever met - and they are the ones building this technology. Perhaps they will be an odd footnote in history, or perhaps they will go down in history like Szilard and Oppenheimer and Teller. If they are seeing the future even close to correctly, we are in for a wild ride.
Let me tell you what we see.
Thanks for listening. To help us out with The Nonlinear Library or to learn more, please visit nonlinear.org
Welcome to The Nonlinear Library, where we use Text-to-Speech software to convert the best writing from the Rationalist and EA communities into audio. This is: Clarifying METR's Auditing Role [linkpost], published by ChanaMessinger on June 5, 2024 on The Effective Altruism Forum.
METR clarifying what auditing it has or hasn't done, and what its plans are for the future, seems very important for understanding the landscape.
Excerpt:
METR has not intended to claim to have audited anything, or to be providing meaningful oversight or accountability, but there has been some confusion about whether METR is an auditor or planning to be one.
To clarify this point:
1. METR's top priority is to develop the science of evaluations, and we don't need to be auditors in order to succeed at this.
We aim to build evaluation protocols that can be used by evaluators/auditors regardless of whether that is the government, an internal lab team, another third party, or a team at METR.
2. We should not be considered to have 'audited' GPT-4 or Claude.
Those were informal pilots of what an audit might involve, or research collaborations - not providing meaningful oversight. For example, it was all under NDA - we didn't have any right or responsibility to disclose our findings to anyone outside the labs - and there wasn't any formal expectation it would inform deployment decisions. We also didn't have the access necessary to perform a proper evaluation.
Thanks for listening. To help us out with The Nonlinear Library or to learn more, please visit nonlinear.org
Welcome to The Nonlinear Library, where we use Text-to-Speech software to convert the best writing from the Rationalist and EA communities into audio. This is: Announcing ILIAD - Theoretical AI Alignment Conference, published by Nora Ammann on June 5, 2024 on The AI Alignment Forum.
We are pleased to announce ILIAD - a 5-day conference bringing together 100+ researchers to build strong scientific foundations for AI alignment.
***
Apply to attend by June 30!***
When: Aug 28 - Sep 3, 2024
Where: @Lighthaven (Berkeley, US)
What: A mix of topic-specific tracks, and unconference style programming, 100+ attendees. Topics will include Singular Learning Theory, Agent Foundations, Causal Incentives, Computational Mechanics and more to be announced.
Who: Currently confirmed speakers include: Daniel Murfet, Jesse Hoogland, Adam Shai, Lucius Bushnaq, Tom Everitt, Paul Riechers, Scott Garrabrant, John Wentworth, Vanessa Kosoy, Fernando Rosas and James Crutchfield.
Costs: Tickets are free. Financial support is available on a needs basis.
See our website here. For any questions, email
iliadconference@gmail.com
About ILIAD
ILIAD is a 100+ person conference about alignment with a mathematical focus. The theme is ecumenical. If that excites you, do apply!
Program and Unconference Format
ILIAD will feature an unconference format - meaning that participants can propose and lead their own sessions. We believe that this is the best way to release the latent creative energies in everyone attending.
That said, freedom can be scary! If taking charge of your own learning sounds terrifying, rest assured there will be plenty of organized sessions as well. We will also run the topic-specific workshop tracks such as:
Computational Mechanics is a framework for understanding complex systems by focusing on their intrinsic computation and information processing capabilities. Pioneered by J. Crutchfield, it has recently found its way into AI safety. This workshop is led by Paul Riechers.
Singular learning theory, developed by S. Watanabe, is the modern theory of Bayesian learning. SLT studies the loss landscape of neural networks, using ideas from statistical mechanics, Bayesian statistics and algebraic geometry. The track lead is Jesse Hoogland.
Agent Foundations uses tools from theoretical economics, decision theory, Bayesian epistemology, logic, game theory and more to deeply understand agents: how they reason, cooperate, believe and desire. The track lead is Daniel Hermann.
Causal Incentives is a collection of researchers interested in using causal models to understand agents and their incentives. The track lead is Tom Everitt.
"Everything Else" is a track which will include an assortment of other sessions under the direction of John Wentworth. Details to be announced at a later time.
Financial Support
Financial support for accommodation & travel are available on a needs basis. Lighthaven has capacity to accommodate 60% of participants. Note that these rooms are shared.
Thanks for listening. To help us out with The Nonlinear Library or to learn more, please visit nonlinear.org.
Welcome to The Nonlinear Library, where we use Text-to-Speech software to convert the best writing from the Rationalist and EA communities into audio. This is: Former OpenAI Superalignment Researcher: Superintelligence by 2030, published by Julian Bradshaw on June 5, 2024 on LessWrong.
The AGI race has begun. We are building machines that can think and reason. By 2025/26, these machines will outpace many college graduates. By the end of the decade, they will be smarter than you or I; we will have superintelligence, in the true sense of the word.
In the link provided, Leopold Aschenbrenner explains why he believes AGI is likely to arrive within the decade, with superintelligence following soon after. He does so in some detail; the website is well-organized, but the raw pdf is over 150 pages.
Leopold is a former member of OpenAI's Superalignment team; he was fired in April for allegedly leaking company secrets. However, he contests that portrayal of events in a recent interview with Dwarkesh Patel, saying he leaked nothing of significance and was fired for other reasons.[1]
However, I am somewhat confused by the new business venture Leopold is now promoting, an "AGI Hedge Fund" aimed at generating strong returns based on his predictions of imminent AGI. In the Dwarkesh Patel interview, it sounds like his intention is to make sure financial resources are available to back AI alignment and any other moves necessary to help Humanity navigate a turbulent future.
However, the discussion in the podcast mostly focuses on whether such a fund would truly generate useful financial returns.
If you read this post, Leopold[2], could you please clarify your intentions in founding this fund?
1. ^
Specifically he brings up a memo he sent to the old OpenAI board claiming OpenAI wasn't taking security seriously enough. He was also one of very few OpenAI employees not to sign the letter asking for Sam Altman's reinstatement last November, and of course, the entire OpenAI superaligment team has collapsed for various reasons as well.
2. ^
Leopold does have a LessWrong account, but hasn't linked his new website here after some time. I hope he doesn't mind me posting in his stead.
Thanks for listening. To help us out with The Nonlinear Library or to learn more, please visit nonlinear.org
Welcome to The Nonlinear Library, where we use Text-to-Speech software to convert the best writing from the Rationalist and EA communities into audio. This is: Evidence of Learned Look-Ahead in a Chess-Playing Neural Network, published by Erik Jenner on June 5, 2024 on LessWrong.
Paper authors: Erik Jenner, Shreyas Kapur, Vasil Georgiev, Cameron Allen, Scott Emmons, Stuart Russell
TL;DR: We released a paper with IMO clear evidence of learned look-ahead in a chess-playing network (i.e., the network considers future moves to decide on its current one). This post shows some of our results, and then I describe the original motivation for the project and reflect on how it went. I think the results are interesting from a scientific and perhaps an interpretability perspective, but only mildly useful for AI safety.
Teaser for the results
(This section is copied from our project website. You may want to read it there for animations and interactive elements, then come back here for my reflections.)
Do neural networks learn to implement algorithms involving look-ahead or search in the wild? Or do they only ever learn simple heuristics? We investigate this question for Leela Chess Zero, arguably the strongest existing chess-playing network.
We find intriguing evidence of learned look-ahead in a single forward pass. This section showcases some of our results, see our paper for much more.
Setup
We consider chess puzzles such as the following:
We focus on the policy network of Leela, which takes in a board state and outputs a distribution over moves. With only a single forward pass per board state, it can solve puzzles like the above. (You can play against the network on Lichess to get a sense of how strong it is - its rating there is over 2600.) Humans and manually written chess engines rely on look-ahead to play chess this well; they consider future moves when making a decision. But is the same thing true for Leela?
Activations associated with future moves are crucial
One of our early experiments was to do activation patching. We patch a small part of Leela's activations from the forward pass of a corrupted version of a puzzle into the forward pass on the original puzzle board state. Measuring the effect on the final output tells us how important that part of Leela's activations was.
Leela is a transformer that treats every square of the chess board like a token in a language model. One type of intervention we can thus do is to patch the activation on a single square in a single layer:
Surprisingly, we found that the target square of the move two turns in the future (what we call the 3rd move target square) often stores very important information. This does not happen in every puzzle, but it does in a striking fraction, and the average effect is much bigger than that of patching on most other squares:
The corrupted square(s) and the 1st move target square are also important (in early and late layers respectively), but we expected as much from Leela's architecture. In contrast, the 3rd move target square stands out in middle layers, and we were much more surprised by its importance.
In the paper, we take early steps toward understanding how the information stored on the 3rd move target square is being used. For example, we find a single attention head that often moves information from this future target square backward in time to the 1st move target square.
Probes can predict future moves
If Leela uses look-ahead, can we explicitly predict future moves from its activations? We train simple, bilinear probes on parts of Leela's activations to predict the move two turns into the future (on a set of puzzles where Leela finds a single clearly best continuation). Our probe architecture is motivated by our earlier results - it predicts whether a given square is the target square of the 3rd move since, as we've seen, this seems to be where Leela stores important information.
We find that this probe can predict the move 2 turns in the future quit...
Welcome to The Nonlinear Library, where we use Text-to-Speech software to convert the best writing from the Rationalist and EA communities into audio. This is: On "first critical tries" in AI alignment, published by Joe Carlsmith on June 5, 2024 on The AI Alignment Forum.
People sometimes say that AI alignment is scary partly (or perhaps: centrally) because you have to get it right on the "first critical try," and can't learn from failures.[1] What does this mean? Is it true? Does there need to be a "first critical try" in the relevant sense? I've sometimes felt confused about this, so I wrote up a few thoughts to clarify.
I start with a few miscellaneous conceptual points. I then focus in on a notion of "first critical try" tied to the first point (if there is one) when AIs get a "decisive strategic advantage" (DSA) over humanity - that is, roughly, the ability to kill/disempower all humans if they try.[2] I further distinguish between four different types of DSA:
Unilateral DSA: Some AI agent could take over if it tried, even without the cooperation of other AI agents (see footnote for more on how I'm individuating AI agents).[3]
Coordination DSA: If some set of AI agents coordinated to try to take over, they would succeed; and they could coordinate in this way if they tried.
Short-term correlation DSA: If some set of AI agents all sought power in problematic ways within a relatively short period of time, even without coordinating, then ~all humans would be disempowered.
Long-term correlation DSA: If some set of AI agents all sought power in problematic ways within a relatively long period of time, even without coordinating, then ~all humans would be disempowered.
I also offer some takes on our prospects for just not ever having "first critical tries" from each type of DSA (via routes other than just not building superhuman AI systems at all). In some cases, just not having a "first critical try" in the relevant sense seems to me both plausible and worth working towards. In particular, I think we should try to make it the case that no single AI system is ever in a position to kill all humans and take over the world.
In other cases, I think avoiding "first critical tries," while still deploying superhuman AI agents throughout the economy, is more difficult (though the difficulty of avoiding failure is another story).
Here's a chart summarizing my takes in more detail.
Type of DSA
Definition
Prospects for avoiding AIs ever getting this type of DSA - e.g., not having a "first critical try" for such a situation.
What's required for it to lead to doom
Unilateral DSA
Some AI agent could take over if it tried, even without the cooperation of other AI agents.
Can avoid by making the world sufficiently empowered relative to each AI system. We should work towards this - e.g. aim to make it the case that no single AI system could kill/disempower all humans if it tried.
Requires only that this one agent tries to take over.
Coordination DSA
If some set of AI agents coordinated to try to take over, they would succeed; and they are able to so coordinate.
Harder to avoid than unilateral DSAs, due to the likely role of other AI agents in preventing unilateral DSAs. But could still avoid/delay by (a) reducing reliance on other AI agents for preventing unilateral DSAs, and (b) preventing coordination between AI agents.
Requires that all these agents try to take over, and that they coordinate.
Short-term correlation DSA
If some set of AI agents all sought power in problematic ways within a relatively short period of time, even without coordinating, then ~all humans would be disempowered.
Even harder to avoid than coordination DSAs, because doesn't require that the AI agents in question be able to coordinate.
Requires that within a relatively short period of time, all these agents choose to seek power in problematic ways, potentially without the ability to coordinate.
Long-term correlation DSA
If some set of AI agents all sought power in prob...