Discover
PaperPlayer biorxiv bioinformatics
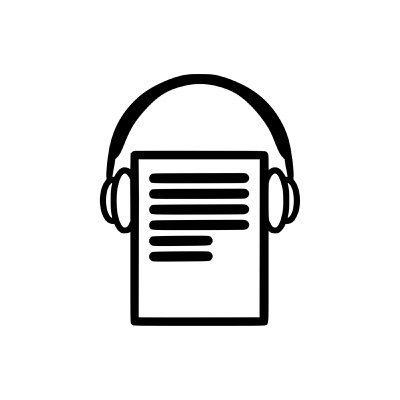
1953 Episodes
Reverse
Link to bioRxiv paper:
http://biorxiv.org/cgi/content/short/2023.08.03.551768v1?rss=1
Authors: Breimann, S., Kamp, F., Steiner, H., Frishman, D.
Abstract:
Amino acid scales are crucial for protein prediction tasks, many of them being curated in the AAindex database. Despite various clustering attempts to organize them and to better understand their relationships, these approaches lack the fine-grained classification necessary for satisfactory interpretability in many protein prediction problems. To address this issue, we developed AAontology, a two-level classification for 586 amino acid scales (mainly from AAindex) together with an in-depth analysis of their relations, using bag-of-word-based classification, clustering, and manual refinement over multiple iterations. AAontology organizes physicochemical scales into 8 categories and 67 subcategories, enhancing the interpretability of scale-based machine learning methods in protein bioinformatics. Thereby it enables researchers to gain a deeper biological insight. We anticipate that AAontology will be a building block to link amino acid properties with protein function and dysfunctions as well as aid informed decision-making in mutation analysis or protein drug design.
Copy rights belong to original authors. Visit the link for more info
Podcast created by Paper Player, LLC
Link to bioRxiv paper:
http://biorxiv.org/cgi/content/short/2023.08.03.551759v1?rss=1
Authors: Choudhery, S., DeJesus, M., Srinivasan, A., Rock, J., Schnappinger, D., Ioerger, T.
Abstract:
An important application of CRISPR interference (CRISPRi) technology is for identifying chemical-genetic interactions (CGIs). Discovery of genes that interact with exposure to antibiotics can yield insights to drug targets and mechanisms of action or resistance. The premise is to look for CRISPRi mutants whose relative abundance is suppressed (or enriched) in the presence of a drug when the target protein is depleted, reflecting synergistic behavior. One thing that is unique about CRISPRi experiments is that sgRNAs for a given target can induce a wide range of protein depletion. The effect of sgRNA strength can be partially predicted based on sequence features or empirically quantified by a passaging experiment. sgRNA strength interacts in a non-linear way with drug sensitivity, producing an effect where the concentration-dependence is maximized for sgRNAs of intermediate strength (and less so for sgRNAs that induce too much or too little target depletion). sgRNA strength has not been explicitly accounted for in previous analytical methods for CRISPRi. We propose a novel method for statistical analysis of CRISPRi CGI data called CRISPRi-DR (for Dose-Response model). CRISPRi-DR incorporates data points from measurements of abundance at multiple inhibitor concentrations using a classic dose-response equation. Importantly, the effect of sgRNA strength can be incorporated into this model in a way that mimics the non-linear interaction between the two covariates on mutant abundance. We use CRISPRi-DR to re-analyze data from a recent CGI experiment in Mycobacterium tuberculosis and show that genes known to interact with various anti-tubercular drugs are ranked highly. We observe similar results in MAGeCK, a related analytical method, for datasets of low variance. However, for noisier datasets, MAGeCK is more susceptible to false positives whereas CRISPRi-DR maintains higher precision, which we observed in both empirical and simulated data, due to CRISPRi-DRs integration of data over multiple concentrations and sgRNA strengths.
Copy rights belong to original authors. Visit the link for more info
Podcast created by Paper Player, LLC
Link to bioRxiv paper:
http://biorxiv.org/cgi/content/short/2023.08.02.551686v1?rss=1
Authors: Li, Y., Yang, R.
Abstract:
Summary: We introduce PxBLAT, a Python library designed to enhance usability and efficiency in interacting with the BLAST-like alignment tool (BLAT). PxBLAT provides an intuitive application programming interface (API) design, allowing the incorporation of its functionality directly into Python-based bioinformatics workflows. Besides, it integrates seamlessly with Biopython and comes equipped with user-centric features like server readiness checks and port retry mechanisms. PxBLAT removes the necessity for system calls and intermediate files, as well as reducing latency and data conversion overhead. Benchmark tests reveal PxBLAT gains a ~20% performance boost compared to BLAT in the Python environment. Availability and Implementation: PxBLAT supports Python (version 3.8+), and pre-compiled packages are released via PyPI (https://pypi.org/project/ pxblat/) and Bioconda (https://anaconda.org/ bioconda/pxblat). The source code of PxBLAT is available under the terms of an open-source MIT license and hosted on GitHub (https:// github.com/ylab-hi/pxblat). Its documentation is available on ReadTheDocs (https://pxblat. readthedocs.io/en/latest/).
Copy rights belong to original authors. Visit the link for more info
Podcast created by Paper Player, LLC
Link to bioRxiv paper:
http://biorxiv.org/cgi/content/short/2023.08.02.551654v1?rss=1
Authors: Li, P., Wei, J., Zhu, Y.
Abstract:
Interpreting the function of genes and gene sets identified from omics experiments remains a challenge, as current pathway analysis tools often fail to account for complex interactions across genes and pathways under specific tissues and cell types. We introduce CellGO, a tool for cell type-specific gene functional analysis. CellGO employs a deep learning model to simulate signaling propagation within a cell, enabling the development of a heuristic pathway activity measuring system to identify cell type-specific active pathways given a single gene or a gene set. It is featured with additional functions to uncover pathway communities and the most active genes within pathways to facilitate mechanistic interpretation. This study demonstrated that CellGO can effectively capture cell type-specific pathways even when working with mixed cell-type markers. CellGO's performance was benchmarked using gene knockout datasets, and its implementation effectively infers the cell type-specific pathogenesis of risk genes associated with neurodevelopmental and neurodegenerative disorders, suggesting its potential in understanding complex polygenic diseases. CellGO is accessible through a python package and a four-mode web interface for interactive usage with pretrained models on 71 single-cell datasets from human and mouse fetal and postnatal brains.
Copy rights belong to original authors. Visit the link for more info
Podcast created by Paper Player, LLC
Link to bioRxiv paper:
http://biorxiv.org/cgi/content/short/2023.08.02.551072v1?rss=1
Authors: Zeng, Y., You, Z., Guo, J., Zhao, J., Zhou, Y., Huang, J., Lyu, X., Chen, L., Li, Q.
Abstract:
The landscape of 3D-genome is crucial for transcription regulation. But capturing the dynamics of chromatin conformation is costly and technically challenging. Here we described Chrombus-XMBD, a graph generative model capable of predicting chromatin interactions ab inito based on available chromatin features. Chrombus employes dynamic edge convolution with QKV attention setup, which maps the relevant chromatin features to a learnable embedding space thereby generate genome-wide 3D-contactmap. We validated Chrombus predictions with published databases of topological associated domains (TAD), eQTLs and gene-enhancer interactions. Chrombus outperforms existing algorithms in efficiently predicting long-range chromatin interactions. Chrombus also exhibits strong generalizability across different cell lineage and species. Additionally, the parameter sets of Chrombus inform the biological processes underlying 3D-genome. Our model provides a new perspective towards interpretable AI-modeling of the dynamics of chromatin interactions and better understanding of cis-regulation of gene expression.
Copy rights belong to original authors. Visit the link for more info
Podcast created by Paper Player, LLC
Link to bioRxiv paper:
http://biorxiv.org/cgi/content/short/2023.08.02.551653v1?rss=1
Authors: Vastrad, B. M., Vastrad, C. M.
Abstract:
Sepsis is the leading systemic inflammatory response syndrome in worldwide, yet relatively little is known about the genes and signaling pathways involved in sepsis progression. The current investigation aimed to elucidate potential key candidate genes and pathways in sepsis and its associated complications. Next generation sequencing (NGS) dataset (GSE185263) was downloaded from the Gene Expression Omnibus (GEO) database, which included data from 348 sepsis samples and 44 normal control samples. Differentially expressed genes (DEGs) were identified using t-tests in the DESeq2 R package. Next, we made use of the g:Profiler to analyze gene ontology (GO) and REACTOME pathway. Then protein-protein interaction (PPI) of these DEGs was visualized by Cytoscape with Search Tool for the Retrieval of Interacting Genes (STRING). Furthermore, we constructed miRNA-hub gene regulatory network and TF-hub gene regulatory network among hub genes utilizing miRNet and NetworkAnalyst online databases tool and Cytoscape software. Finally, we performed receiver operating characteristic (ROC) curve analysis of hub genes through the pROC package in R statistical software. In total, 958 DEGs were identified, of which 479 were up regulated and 479 were down regulated. GO and REACTOME results showed that DEGs mainly enriched in regulation of cellular process, response to stimulus, extracellular matrix organization and immune system. The hub genes of PRKN, KIT, FGFR2, GATA3, ERBB3, CDK1, PPARG, H2BC5, H4C4 and CDC20 might be associated with sepsis and its associated complications. Predicted miRNAs (e.g., hsa-mir-548ad-5p and hsa-mir-2113) and TFs (e.g., YAP1 and TBX5) were found to be significantly correlated with sepsis and its associated complications. In conclusion, the DEGs, relative pathways, hub genes, miRNA and TFs identified in the current investigation might help in understanding of the molecular mechanisms underlying sepsis and its associated complications progression and provide potential molecular targets and biomarkers for sepsis and its associated complications.
Copy rights belong to original authors. Visit the link for more info
Podcast created by Paper Player, LLC
Link to bioRxiv paper:
http://biorxiv.org/cgi/content/short/2023.08.02.551637v1?rss=1
Authors: Lause, J., Ziegenhain, C., Hartmanis, L., Berens, P., Kobak, D.
Abstract:
Before downstream analysis can reveal biological signals in single-cell RNA sequencing data, normalization and variance stabilization are required to remove technical noise. Recently, Pearson residuals based on negative binomial models have been suggested as an efficient normalization approach. These methods were developed for UMI-based sequencing protocols, where unique molecular identifiers (UMIs) help to remove PCR amplification noise by keeping track of the original molecules. In contrast, full-length protocols such as Smart-seq2 lack UMIs and retain amplification noise, making negative binomial models inapplicable. Here, we extend Pearson residuals to such read count data by modeling them as a compound process: we assume that the captured RNA molecules follow the negative binomial distribution, but are replicated according to an amplification distribution. Based on this model, we introduce compound Pearson residuals and show that they can be analytically obtained without explicit knowledge of the amplification distribution. Further, we demonstrate that compound Pearson residuals lead to a biologically meaningful gene selection and low-dimensional embeddings of complex Smart-seq2 datasets. Finally, we empirically study amplification distributions across several sequencing protocols, and suggest that they can be described by a broken power law. We show that the resulting compound distribution captures overdispersion and zero-inflation patterns characteristic of read count data. In summary, compound Pearson residuals provide an efficient and effective way to normalize read count data based on simple mechanistic assumptions.
Copy rights belong to original authors. Visit the link for more info
Podcast created by Paper Player, LLC
Link to bioRxiv paper:
http://biorxiv.org/cgi/content/short/2023.08.02.551620v1?rss=1
Authors: Sharma, A., Lopez, Y., JIA, S., Lysenko, A., Boroevich, K., Tsunoda, T.
Abstract:
Tabular data analysis is a critical task in various domains, enabling us to uncover valuable insights from structured datasets. While traditional machine learning methods have been employed for feature engineering and dimensionality reduction, they often struggle to capture the intricate relationships and dependencies within real-world datasets. In this paper, we present Multi-representation DeepInsight (abbreviated as MRep-DeepInsight), an innovative extension of the DeepInsight method, specifically designed to enhance the analysis of tabular data. By generating multiple representations of samples using diverse feature extraction techniques, our approach aims to capture a broader range of features and reveal deeper insights. We demonstrate the effectiveness of MRep-DeepInsight on single-cell datasets, Alzheimer's data, and artificial data, showcasing an improved accuracy over the original DeepInsight approach and machine learning methods like random forest and L2-regularized logistic regression. Our results highlight the value of incorporating multiple representations for robust and accurate tabular data analysis. By embracing the power of diverse representations, MRep-DeepInsight offers a promising avenue for advancing decision-making and scientific discovery across a wide range of fields.
Copy rights belong to original authors. Visit the link for more info
Podcast created by Paper Player, LLC
Link to bioRxiv paper:
http://biorxiv.org/cgi/content/short/2023.08.04.410498v1?rss=1
Authors: Dick, K., Green, J. R.
Abstract:
No. While our experiments ultimately failed, this work was motivated by the seemingly reasonable hypothesis that encoding protein sequences as a fractal-based image in combination with a binary mask identifying those pixels representative of the protein binding interface could effectively be used to fine-tune a semantic segmentation model. We were wrong. Despite the shortcomings of this work, a number of insights were drawn, inspiring discussion about how this fractal-based space may be exploited to generate effective protein binding site predictors in the future. Furthermore, these realizations promise to orient complimentary studies leveraging fractal-based representations, whether in the field of bioinformatics, or more broadly within disparate fields leveraging sequence-type data, such as Natural Language Processing. In a non-traditional way, this work presents the experimental design undertaken and interleaves various insights and limitations. It is the hope of this work that those interested in leveraging fractal-based representations and deep learning architectures as part of their work will benefit from the insights arising from this work.
Copy rights belong to original authors. Visit the link for more info
Podcast created by Paper Player, LLC
Link to bioRxiv paper:
http://biorxiv.org/cgi/content/short/2023.08.03.551813v1?rss=1
Authors: Soto Miranda, M., Narvaez Romo, R., Moshiri, N.
Abstract:
Introduction: Public health faces the ongoing mission of safeguarding the population's health against various infectious diseases caused by a great number of pathogens. Epidemiology is an essential discipline in this field. With the rise of more advanced technologies, new tools are emerging to enhance the capability to intervene and control an epidemic. Among these approaches, molecular clustering comes forth as a promising option. However, appropriate genetic distance thresholds for defining clusters are poorly explored in contexts outside of Human Immunodeficiency Virus-1 (HIV-1). Methods: In this work, using the well-used pairwise Tamura-Nei 93 (TN93) distance threshold of 0.015 for HIV-1 as a point of reference for molecular cluster properties of interest, we perform molecular clustering on whole genome sequence datasets from HIV-1, Severe Acute Respiratory Syndrome Coronavirus 2 (SARS-CoV-2), Zaire ebolavirus, and Mpox virus, to explore potential pairwise distances thresholds for these other viruses. Results: We found the following pairwise TN93 distance thresholds as potential candidates for use in molecular clustering: 0.00014 (4 mutations) for SARS-CoV-2, 0.00016 (3 mutations) for Ebola, and 0.0000051 (1 mutation) for Mpox. Conclusion: This study provides valuable information for epidemic control strategies, and public health efforts in managing infectious diseases caused by these viruses. The identified pairwise distance thresholds for molecular clustering can serve as a foundation for future research and intervention to combat epidemics effectively.
Copy rights belong to original authors. Visit the link for more info
Podcast created by Paper Player, LLC
Link to bioRxiv paper:
http://biorxiv.org/cgi/content/short/2023.08.04.551958v1?rss=1
Authors: Courtney, E., Datta, A., Mathews, D. H., Ward, M.
Abstract:
Determining RNA secondary structure is a core problem in computational biology. Fast algorithms for predicting secondary structure are fundamental to this task. We describe a modified formulation of the Zuker-Stiegler algorithm with coaxial stacking, a stabilizing interaction in which the ends of multi-loops are stacked. In particular, optimal coaxial stacking is computed as part of the dynamic programming state, rather than inline. We introduce a new notion of sparsity, which we call replaceability. The modified formulation along with replaceability allows sparsification to be applied to coaxial stacking as well, which increases the speed of the algorithm. We implemented this algorithm in software we call memerna, which we show to have the fastest exact RNA folding implementation out of several popular RNA folding packages supporting coaxial stacking. We also introduce a new notation for secondary structure which includes coaxial stacking, terminal mismatches, and dangles (CTDs) information.
Copy rights belong to original authors. Visit the link for more info
Podcast created by Paper Player, LLC
Link to bioRxiv paper:
http://biorxiv.org/cgi/content/short/2023.08.03.551876v1?rss=1
Authors: Ozturk, K., Panwala, R., Sheen, J., Ford, K., Payne, N., Zhang, D.-E., Hutter, S., Haferlach, T., Ideker, T., Mali, P., Carter, H.
Abstract:
Understanding the consequences of single amino acid substitutions in cancer driver genes remains an unmet need. Perturb-seq provides a tool to investigate the effects of individual mutations on cellular programs. Here we deploy SEUSS, a Perturb-seq like approach, to generate and assay mutations at physical interfaces of the RUNX1 Runt domain. We measured the impact of 115 mutations on RNA profiles in single myelogenous leukemia cells and used the profiles to categorize mutations into three functionally distinct groups: wild-type (WT)-like, loss-of-function (LOF)-like and hypomorphic. Notably, the largest concentration of functional mutations (non-WT-like) clustered at the DNA binding site and contained many of the more frequently observed mutations in human cancers. Hypomorphic variants shared characteristics with loss of function variants but had gene expression profiles indicative of response to neural growth factor and cytokine recruitment of neutrophils. Additionally, DNA accessibility changes upon perturbations were enriched for RUNX1 binding motifs, particularly near differentially expressed genes. Overall, our work demonstrates the potential of targeting protein interaction interfaces to better define the landscape of prospective phenotypes reachable by amino acid substitutions.
Copy rights belong to original authors. Visit the link for more info
Podcast created by Paper Player, LLC
Link to bioRxiv paper:
http://biorxiv.org/cgi/content/short/2023.08.03.551784v1?rss=1
Authors: Fiam, R. N., Csabai, I., Solymosi, N.
Abstract:
This study proposes a novel approach to studying SARS-CoV-2 virus mutations through sequencing data comparison. Traditional consensus-based methods, which focus on the most common nucleotide at each position, might overlook or obscure the presence of low-frequency variants. Our method, in contrast, retains all sequenced nucleotides at each position, forming a genomic matrix. Utilizing simulated short reads from genomes with specified mutations, we contrasted our genomic matrix approach with the consensus sequence method. Our matrix methodology accurately reflected the known mutations and true compositions, demonstrating its efficacy in understanding the sample variability and their interconnections. Further tests using real data from GISAID and NCBI-SRA confirmed its reliability and robustness. As we see, the genomic matrix approach offers a more accurate representation of the viral genomic diversity, thereby providing superior insights into virus evolution and epidemiology. Future application recommendations are provided based on our observed results.
Copy rights belong to original authors. Visit the link for more info
Podcast created by Paper Player, LLC
Link to bioRxiv paper:
http://biorxiv.org/cgi/content/short/2023.08.02.550128v1?rss=1
Authors: Liu, Q., Gao, Y., Gao, Y., Li, W., Wu, S.
Abstract:
The identification of T cell neo-epitopes is fundamental and computational challenging in tumor immunotherapy study. As the binding of pMHC - T cell receptor (TCR) is the essential condition for neo-epitopes to trigger the cytotoxic T cell reactivity, several computational studies have been proposed to predict neo-epitopes from the perspective of pMHC-TCR binding recognition. However, they often failed with the inaccurate binding prediction for a single pMHC -TCR pair due to the highly diverse TCR space. In this study, we proposed a novel weakly-supervised learning framework, i.e., TCRBagger, to facilitate the personalized neo-epitope identification with weakly-supervised peptide-TCR binding prediction by bagging a sample-specific TCR profile. TCRBagger integrates three carefully designed learning strategies, i.e. a self-supervised learning strategy, a denoising learning strategy and a Multi-Instance Learning (MIL) strategy in the modeling of peptide-TCR binding. Our comprehensive tests revealed that TCRBagger exhibited great advances over existing tools by modeling interactions between peptide and TCR profiles. We further applied TCRBagger in different clinical settings, including (1) facilitating the peptide-TCR binding prediction under MIL using single-cell TCR-seq data. (2) improving the patient-specific neoantigen prioritization compared to the existing neoantigen identification tools. Collectively, TCRBagger provides novel perspectives and contributions for identifying neo-epitopes as well as discovering potential pMHC-TCR interactions in personalized tumor immunotherapy.
Copy rights belong to original authors. Visit the link for more info
Podcast created by Paper Player, LLC
Link to bioRxiv paper:
http://biorxiv.org/cgi/content/short/2023.08.01.551396v1?rss=1
Authors: Liu, S., Yu, J., Ni, N., Wang, Z., Chen, M., Li, Y., Xu, C., Bai, Q., Ding, Y., Wang, C., Zhang, J., Yao, X., Liu, H.
Abstract:
Predicting drug-target interaction (DTI) is a critical and rate-limiting step in drug discovery. Traditional wet-lab experiments are reliable but expensive and time-consuming. Recently, deep learning has revealed itself as a new and promising tool for accelerating the DTI prediction process because its powerful performance. Due to the vast chemical space, the DTI prediction models are typically expected to discover drugs or targets that are absent from the training set. However, generalizing prediction performance to novel drug-target pairs that belong to different distributions is a challenge for deep learning methods. In this work, we propose an Ensemble of models that capture both Domain-generIc and domain-Specific features (E-DIS) to learn diversity domain features and adapt to out-of-distribution (OOD) data. We employed Mixture-of-Experts (MOE) as a domain-specific feature extractor for the raw data to prevent the loss of any crucial features by the encoder during the learning process. Multiple experts are trained on different domains to capture and align domain-specific information from various distributions without accessing any data from unseen domains. We evaluate our approach using four benchmark datasets under both in-domain and cross-domain settings and compare it with advanced approaches for solving OOD generalization problems. The results demonstrate that E-DIS effectively improves the robustness and generalizability of DTI prediction models by incorporating diversity domain features.
Copy rights belong to original authors. Visit the link for more info
Podcast created by Paper Player, LLC
Link to bioRxiv paper:
http://biorxiv.org/cgi/content/short/2023.08.01.551559v1?rss=1
Authors: Dadlani, E., Dash, T., Sahoo, D.
Abstract:
Tumor-associated Macrophages (or TAMs) are amongst the most common cells that play a significant role in the initiation and progression of colorectal cancer (CRC). Recently, Ghosh et al. proposed distinguishing signatures for identifying macrophage polarization states, namely, immuno-reactive and immuno-tolerant, using the concept of Boolean implications and Boolean networks. Their signature, called the Signature of Macrophage Reactivity and Tolerance (SMaRT), comprises of 338 human genes (equivalently, 298 mouse genes). However, SMaRT was constructed using datasets that were not specialized towards any particular disease. In this paper, (a) we perform a comprehensive analysis of the SMaRT signature on single-cell human and mouse colorectal cancer RNA-seq datasets; (b) we then adopt a technique akin to transfer learning to construct a "refined" SMaRT signature for investigating TAMs and their polarization in the CRC tumor microenvironment. Towards validation of our refined gene signature, we use (a) 5 pseudo-bulk RNA-seq datasets derived from single-cell human datasets; and (b) 5 large-cohort microarray datasets from humans. Furthermore, we investigate the translational potential of our refined gene signature in problems related to MSS/MSI (4 datasets) and CIMP+/CIMP- status (4 datasets). Overall, our refined gene signature and its extensive validation provide a path for its adoption in clinical practice in diagnosing colorectal cancer and associated attributes.
Copy rights belong to original authors. Visit the link for more info
Podcast created by Paper Player, LLC
Link to bioRxiv paper:
http://biorxiv.org/cgi/content/short/2023.08.01.551497v1?rss=1
Authors: Tsishyn, M., Cia, G., Hermans, P., Kwasigroch, J., Rooman, M., Pucci, F.
Abstract:
Systematically predicting the effects of mutations on protein fitness is essential for the understanding of genetic diseases. Indeed, predictions complement experimental efforts in analyzing how variants lead to dysfunctional proteins that in turn can cause diseases. Here we present our new fitness predictor, FiTMuSiC, which leverages structural, evolutionary and coevolutionary information. We show that FiTMuSiC predicts fitness with high accuracy despite the simplicity of its underlying model: it was one of the top predictors on the hydroxymethylbilane synthase (HMBS) target of the sixth round of the Critical Assessment of Genome Interpretation challenge (CAGI6). To further demonstrate FiTMuSiC's robustness, we compared its predictions with in vitro activity data on HMBS, variant fitness data on human glucokinase (GCK), and variant deleteriousness data on HMBS and GCK. These analyses further confirm FiTMuSiC's qualities and accuracy, which compare favorably with those of other predictors. Additionally, FiTMuSiC returns two scores that separately describe the functional and structural effects of the variant, thus providing mechanistic insight into why the variant leads to fitness loss or gain. We also provide an easy-to-use webserver at babylone.ulb.ac.be/FiTMuSiC, which is freely available for academic use and does not require any bioinformatics expertise, which simplifies the accessibility of our tool for the entire scientific community.
Copy rights belong to original authors. Visit the link for more info
Podcast created by Paper Player, LLC
Link to bioRxiv paper:
http://biorxiv.org/cgi/content/short/2023.08.01.551483v1?rss=1
Authors: Gorantla, R., Kubincova, A., Weisse, A. Y., Mey, A. S. J. S.
Abstract:
Accurate in silico prediction of protein-ligand binding affinity is important in the early stages of drug discovery. Deep learning-based methods exist but have yet to overtake more conventional methods such as giga-docking largely due to their lack of generalisability. To improve generalizability we need to understand what these models learn from input protein and ligand data. We systematically investigated a sequence-based deep learning framework to assess the impact of protein and ligand encodings on predicting binding affinities for commonly used kinase data sets. The role of proteins is studied using convolutional neural network-based encodings obtained from sequences and graph neural network-based encodings enriched with structural information from contact maps. Ligand-based encodings are generated from graph-neural networks. We test different ligand perturbations by randomizing node and edge properties. For proteins we make use of 3 different protein contact generation methods (AlphaFold2, Pconsc4, and ESM-1b) and compare these with a random control. Our investigation shows that protein encodings do not substantially impact the binding predictions, with no statistically significant difference in binding affinity for KIBA in the investigated metrics (concordance index, Pearson's R Spearman's Rank, and RMSE). Significant differences are seen for ligand encodings with random ligands and random ligand node properties, suggesting a much bigger reliance on ligand data for the learning tasks. Using different ways to combine protein and ligand encodings, did not show a significant change in performance.
Copy rights belong to original authors. Visit the link for more info
Podcast created by Paper Player, LLC
Link to bioRxiv paper:
http://biorxiv.org/cgi/content/short/2023.08.01.551575v1?rss=1
Authors: Yuan, Q., Duren, Z.
Abstract:
Accurate context-specific Gene Regulatory Networks (GRNs) inference from genomics data is a crucial task in computational biology. However, existing methods face limitations, such as reliance on gene expression data alone, lower resolution from bulk data, and data scarcity for specific cellular systems. Despite recent technological advancements, including single-cell sequencing and the integration of ATAC-seq and RNA-seq data, learning such complex mechanisms from limited independent data points still presents a daunting challenge, impeding GRN inference accuracy. To overcome this challenge, we present LINGER (LIfelong neural Network for GEne Regulation), a novel deep learning-based method to infer GRNs from single-cell multiome data with paired gene expression and chromatin accessibility data from the same cell. LINGER incorporates both 1) atlas-scale external bulk data across diverse cellular contexts and 2) the knowledge of transcription factor (TF) motif matching to cis-regulatory elements as a manifold regularization to address the challenge of limited data and extensive parameter space in GRN inference. Our results demonstrate that LINGER achieves 2-3 fold higher accuracy over existing methods. LINGER reveals a complex regulatory landscape of genome-wide association studies, enabling enhanced interpretation of disease-associated variants and genes. Additionally, following the GRN inference from a reference sc-multiome data, LINGER allows for the estimation of TF activity solely from bulk or single-cell gene expression data, leveraging the abundance of available gene expression data to identify driver regulators from case-control studies. Overall, LINGER provides a comprehensive tool for robust gene regulation inference from genomics data, empowering deeper insights into cellular mechanisms.
Copy rights belong to original authors. Visit the link for more info
Podcast created by Paper Player, LLC
Link to bioRxiv paper:
http://biorxiv.org/cgi/content/short/2023.08.01.551468v1?rss=1
Authors: Mukashyaka, P., Sheridan, T. B., Foroughi pour, A., Chuang, J. H.
Abstract:
Deep learning has revolutionized digital pathology, allowing for automatic analysis of hematoxylin and eosin (H&E) stained whole slide images (WSIs) for diverse tasks. In such analyses, WSIs are typically broken into smaller images called tiles, and a neural network backbone encodes each tile in a feature space. Many recent works have applied attention based deep learning models to aggregate tile-level features into a slide-level representation, which is then used for slide-level prediction tasks. However, training attention models is computationally intensive, necessitating hyperparameter optimization and specialized training procedures. Here, we propose SAMPLER, a fully statistical approach to generate efficient and informative WSI representations by encoding the empirical cumulative distribution functions (CDFs) of multiscale tile features. We demonstrate that SAMPLER-based classifiers are as accurate or better than state-of-the-art fully deep learning attention models for classification tasks including distinction of: subtypes of breast carcinoma (BRCA: AUC=0.911 {+/-} 0.029); subtypes of non-small cell lung carcinoma (NSCLC: AUC=0.940 {+/-} 0.018); and subtypes of renal cell carcinoma (RCC: AUC=0.987 {+/-} 0.006). A major advantage of the SAMPLER representation is that predictive models are greater than 100X faster compared to attention models. Histopathological review confirms that SAMPLER-identified high attention tiles contain tumor morphological features specific to the tumor type, while low attention tiles contain fibrous stroma, blood, or tissue folding artifacts. We further apply SAMPLER concepts to improve the design of attention-based neural networks, yielding a context aware multi-head attention model with increased accuracy for subtype classification within BRCA and RCC (BRCA: AUC=0.921 {+/-} 0.027, and RCC: AUC=0.988 {+/-} 0.010). Finally, we provide theoretical results identifying sufficient conditions for which SAMPLER is optimal. SAMPLER is a fast and effective approach for analyzing WSIs, with greatly improved scalability over attention methods to benefit digital pathology analysis.
Copy rights belong to original authors. Visit the link for more info
Podcast created by Paper Player, LLC