Discover
AI Engineering Podcast
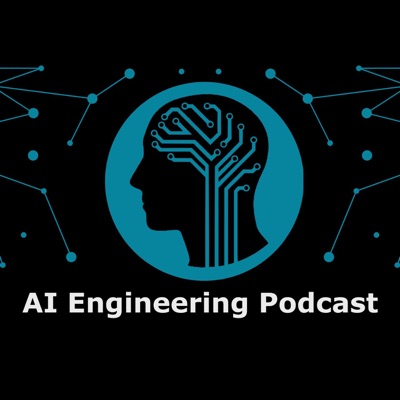
AI Engineering Podcast
Author: Tobias Macey
Subscribed: 8,845Played: 243,742Subscribe
Share
© 2024 Boundless Notions, LLC.
Description
This show is your guidebook to building scalable and maintainable AI systems. You will learn how to architect AI applications, apply AI to your work, and the considerations involved in building or customizing new models. Everything that you need to know to deliver real impact and value with machine learning and artificial intelligence.
46 Episodes
Reverse
SummaryIn this episode of the AI Engineering podcast Viraj Mehta, CTO and co-founder of TensorZero, talks about the use of LLM gateways for managing interactions between client-side applications and various AI models. He highlights the benefits of using such a gateway, including standardized communication, credential management, and potential features like request-response caching and audit logging. The conversation also explores TensorZero's architecture and functionality in optimizing AI applications by managing structured data inputs and outputs, as well as the challenges and opportunities in automating prompt generation and maintaining interaction history for optimization purposes.AnnouncementsHello and welcome to the AI Engineering Podcast, your guide to the fast-moving world of building scalable and maintainable AI systemsSeamless data integration into AI applications often falls short, leading many to adopt RAG methods, which come with high costs, complexity, and limited scalability. Cognee offers a better solution with its open-source semantic memory engine that automates data ingestion and storage, creating dynamic knowledge graphs from your data. Cognee enables AI agents to understand the meaning of your data, resulting in accurate responses at a lower cost. Take full control of your data in LLM apps without unnecessary overhead. Visit aiengineeringpodcast.com/cognee to learn more and elevate your AI apps and agents. Your host is Tobias Macey and today I'm interviewing Viraj Mehta about the purpose of an LLM gateway and his work on TensorZeroInterviewIntroductionHow did you get involved in machine learning?What is an LLM gateway?What purpose does it serve in an AI application architecture?What are some of the different features and capabilities that an LLM gateway might be expected to provide?Can you describe what TensorZero is and the story behind it?What are the core problems that you are trying to address with Tensor0 and for whom?One of the core features that you are offering is management of interaction history. How does this compare to the "memory" functionality offered by e.g. LangChain, Cognee, Mem0, etc.?How does the presence of TensorZero in an application architecture change the ways that an AI engineer might approach the logic and control flows in a chat-based or agent-oriented project?Can you describe the workflow of building with Tensor0 and some specific examples of how it feeds back into the performance/behavior of an LLM?What are some of the ways in which the addition of Tensor0 or another LLM gateway might have a negative effect on the design or operation of an AI application?What are the most interesting, innovative, or unexpected ways that you have seen TensorZero used?What are the most interesting, unexpected, or challenging lessons that you have learned while working on TensorZero?When is TensorZero the wrong choice?What do you have planned for the future of TensorZero?Contact InfoLinkedInParting QuestionFrom your perspective, what are the biggest gaps in tooling, technology, or training for AI systems today?Closing AnnouncementsThank you for listening! Don't forget to check out our other shows. The Data Engineering Podcast covers the latest on modern data management. Podcast.__init__ covers the Python language, its community, and the innovative ways it is being used.Visit the site to subscribe to the show, sign up for the mailing list, and read the show notes.If you've learned something or tried out a project from the show then tell us about it! Email hosts@aiengineeringpodcast.com with your story.To help other people find the show please leave a review on iTunes and tell your friends and co-workers.LinksTensorZeroLLM GatewayLiteLLMOpenAIGoogle VertexAnthropicReinforcement LearningTokamak ReactorViraj RLHF PaperContextual Dueling BanditsDirect Preference OptimizationPartially Observable Markov Decision ProcessDSPyPyTorchCogneeMem0LangGraphDouglas HofstadterOpenAI GymOpenAI o1OpenAI o3Chain Of ThoughtThe intro and outro music is from Hitman's Lovesong feat. Paola Graziano by The Freak Fandango Orchestra/CC BY-SA 3.0
SummaryIn this episode of the AI Engineering Podcast Ron Green, co-founder and CTO of KungFu AI, talks about the evolving landscape of AI systems and the challenges of harnessing generative AI engines. Ron shares his insights on the limitations of large language models (LLMs) as standalone solutions and emphasizes the need for human oversight, multi-agent systems, and robust data management to support AI initiatives. He discusses the potential of domain-specific AI solutions, RAG approaches, and mixture of experts to enhance AI capabilities while addressing risks. The conversation also explores the evolving AI ecosystem, including tooling and frameworks, strategic planning, and the importance of interpretability and control in AI systems. Ron expresses optimism about the future of AI, predicting significant advancements in the next 20 years and the integration of AI capabilities into everyday software applications.AnnouncementsHello and welcome to the AI Engineering Podcast, your guide to the fast-moving world of building scalable and maintainable AI systemsSeamless data integration into AI applications often falls short, leading many to adopt RAG methods, which come with high costs, complexity, and limited scalability. Cognee offers a better solution with its open-source semantic memory engine that automates data ingestion and storage, creating dynamic knowledge graphs from your data. Cognee enables AI agents to understand the meaning of your data, resulting in accurate responses at a lower cost. Take full control of your data in LLM apps without unnecessary overhead. Visit aiengineeringpodcast.com/cognee to learn more and elevate your AI apps and agents. Your host is Tobias Macey and today I'm interviewing Ron Green about the wheels that we need for harnessing the power of the generative AI engineInterviewIntroductionHow did you get involved in machine learning?Can you describe what you see as the main shortcomings of LLMs as a stand-alone solution (to anything)?The most established vehicle for harnessing LLM capabilities is the RAG pattern. What are the main limitations of that as a "product" solution?The idea of multi-agent or mixture-of-experts systems is a more sophisticated approach that is gaining some attention. What do you see as the pro/con conversation around that pattern?Beyond the system patterns that are being developed there is also a rapidly shifting ecosystem of frameworks, tools, and point solutions that plugin to various points of the AI lifecycle. How does that volatility hinder the adoption of generative AI in different contexts?In addition to the tooling, the models themselves are rapidly changing. How much does that influence the ways that organizations are thinking about whether and when to test the waters of AI?Continuing on the metaphor of LLMs and engines and the need for vehicles, where are we on the timeline in relation to the model T Ford?What are the vehicle categories that we still need to design and develop? (e.g. sedans, mini-vans, freight trucks, etc.)The current transformer architecture is starting to reach scaling limits that lead to diminishing returns. Given your perspective as an industry veteran, what are your thoughts on the future trajectory of AI model architectures?What is the ongoing role of regression style ML in the landscape of generative AI?What are the most interesting, innovative, or unexpected ways that you have seen LLMs used to power a "vehicle"?What are the most interesting, unexpected, or challenging lessons that you have learned while working in this phase of AI?When is generative AI/LLMs the wrong choice?Contact InfoLinkedInParting QuestionFrom your perspective, what are the biggest gaps in tooling, technology, or training for AI systems today?Closing AnnouncementsThank you for listening! Don't forget to check out our other shows. The Data Engineering Podcast covers the latest on modern data management. Podcast.__init__ covers the Python language, its community, and the innovative ways it is being used.Visit the site to subscribe to the show, sign up for the mailing list, and read the show notes.If you've learned something or tried out a project from the show then tell us about it! Email hosts@aiengineeringpodcast.com with your story.To help other people find the show please leave a review on iTunes and tell your friends and co-workers.LinksKungfu.aiLlama open generative AI modelsChatGPTCopilotCursorRAG == Retrieval Augmented GenerationPodcast EpisodeMixture of ExpertsDeep LearningRandom ForestSupervised LearningActive Learning)Yann LeCunnRLHF == Reinforcement Learning from Human FeedbackModel T FordMamba selective state spaceLiquid NetworkChain of thoughtOpenAI o1Marvin MinskyVon Neumann ArchitectureAttention Is All You NeedMultilayer PerceptronDot ProductDiffusion ModelGaussian NoiseAlphaFold 3AnthropicSparse AutoencoderThe intro and outro music is from Hitman's Lovesong feat. Paola Graziano by The Freak Fandango Orchestra/CC BY-SA 3.0
SummaryIn this episode of the AI Engineering Podcast Jim Olsen, CTO of ModelOp, talks about the governance of generative AI models and applications. Jim shares his extensive experience in software engineering and machine learning, highlighting the importance of governance in high-risk applications like healthcare. He explains that governance is more about the use cases of AI models rather than the models themselves, emphasizing the need for proper inventory and monitoring to ensure compliance and mitigate risks. The conversation covers challenges organizations face in implementing AI governance policies, the importance of technical controls for data governance, and the need for ongoing monitoring and baselines to detect issues like PII disclosure and model drift. Jim also discusses the balance between innovation and regulation, particularly with evolving regulations like those in the EU, and provides valuable perspectives on the current state of AI governance and the need for robust model lifecycle management.AnnouncementsHello and welcome to the AI Engineering Podcast, your guide to the fast-moving world of building scalable and maintainable AI systemsYour host is Tobias Macey and today I'm interviewing Jim Olsen about governance of your generative AI models and applicationsInterviewIntroductionHow did you get involved in machine learning?Can you describe what governance means in the context of generative AI models? (e.g. governing the models, their applications, their outputs, etc.)Governance is typically a hybrid endeavor of technical and organizational policy creation and enforcement. From the organizational perspective, what are some of the difficulties that teams are facing in understanding what those policies need to encompass?How much familiarity with the capabilities and limitations of the models is necessary to engage productively with policy debates?The regulatory landscape around AI is still very nascent. Can you give an overview of the current state of legal burden related to AI?What are some of the regulations that you consider necessary but as-of-yet absent?Data governance as a practice typically relates to controls over who can access what information and how it can be used. The controls for those policies are generally available in the data warehouse, business intelligence, etc. What are the different dimensions of technical controls that are needed in the application of generative AI systems?How much of the controls that are present for governance of analytical systems are applicable to the generative AI arena?What are the elements of risk that change when considering internal vs. consumer facing applications of generative AI?How do the modalities of the AI models impact the types of risk that are involved? (e.g. language vs. vision vs. audio)What are some of the technical aspects of the AI tools ecosystem that are in greatest need of investment to ease the burden of risk and validation of model use?What are the most interesting, innovative, or unexpected ways that you have seen AI governance implemented?What are the most interesting, unexpected, or challenging lessons that you have learned while working on AI governance?What are the technical, social, and organizational trends of AI risk and governance that you are monitoring?Contact InfoLinkedInParting QuestionFrom your perspective, what are the biggest gaps in tooling, technology, or training for AI systems today?Closing AnnouncementsThank you for listening! Don't forget to check out our other shows. The Data Engineering Podcast covers the latest on modern data management. Podcast.__init__ covers the Python language, its community, and the innovative ways it is being used.Visit the site to subscribe to the show, sign up for the mailing list, and read the show notes.If you've learned something or tried out a project from the show then tell us about it! Email hosts@aiengineeringpodcast.com with your story.To help other people find the show please leave a review on iTunes and tell your friends and co-workers.LinksModelOpFoundation ModelsGDPREU AI RegulationLlama 2AWS BedrockShadow ITRAG == Retrieval Augmented GenerationPodcast EpisodeNvidia NEMOLangChainShapley ValuesGibberish DetectionThe intro and outro music is from Hitman's Lovesong feat. Paola Graziano by The Freak Fandango Orchestra/CC BY-SA 3.0
SummaryIn this episode of the AI Engineering Podcast, Vasilije Markovich talks about enhancing Large Language Models (LLMs) with memory to improve their accuracy. He discusses the concept of memory in LLMs, which involves managing context windows to enhance reasoning without the high costs of traditional training methods. He explains the challenges of forgetting in LLMs due to context window limitations and introduces the idea of hierarchical memory, where immediate retrieval and long-term information storage are balanced to improve application performance. Vasilije also shares his work on Cognee, a tool he's developing to manage semantic memory in AI systems, and discusses its potential applications beyond its core use case. He emphasizes the importance of combining cognitive science principles with data engineering to push the boundaries of AI capabilities and shares his vision for the future of AI systems, highlighting the role of personalization and the ongoing development of Cognee to support evolving AI architectures.AnnouncementsHello and welcome to the AI Engineering Podcast, your guide to the fast-moving world of building scalable and maintainable AI systemsYour host is Tobias Macey and today I'm interviewing Vasilije Markovic about adding memory to LLMs to improve their accuracyInterviewIntroductionHow did you get involved in machine learning?Can you describe what "memory" is in the context of LLM systems?What are the symptoms of "forgetting" that manifest when interacting with LLMs?How do these issues manifest between single-turn vs. multi-turn interactions?How does the lack of hierarchical and evolving memory limit the capabilities of LLM systems?What are the technical/architectural requirements to add memory to an LLM system/application?How does Cognee help to address the shortcomings of current LLM/RAG architectures?Can you describe how Cognee is implemented?Recognizing that it has only existed for a short time, how have the design and scope of Cognee evolved since you first started working on it?What are the data structures that are most useful for managing the memory structures?For someone who wants to incorporate Cognee into their LLM architecture, what is involved in integrating it into their applications?How does it change the way that you think about the overall requirements for an LLM application?For systems that interact with multiple LLMs, how does Cognee manage context across those systems? (e.g. different agents for different use cases)There are other systems that are being built to manage user personalization in LLm applications, how do the goals of Cognee relate to those use cases? (e.g. Mem0 - https://github.com/mem0ai/mem0)What are the unknowns that you are still navigating with Cognee?What are the most interesting, innovative, or unexpected ways that you have seen Cognee used?What are the most interesting, unexpected, or challenging lessons that you have learned while working on Cognee?When is Cognee the wrong choice?What do you have planned for the future of Cognee?Contact InfoLinkedInParting QuestionFrom your perspective, what are the biggest gaps in tooling, technology, or training for AI systems today?Closing AnnouncementsThank you for listening! Don't forget to check out our other shows. The Data Engineering Podcast covers the latest on modern data management. Podcast.__init__ covers the Python language, its community, and the innovative ways it is being used.Visit the site to subscribe to the show, sign up for the mailing list, and read the show notes.If you've learned something or tried out a project from the show then tell us about it! Email hosts@aiengineeringpodcast.com with your story.To help other people find the show please leave a review on iTunes and tell your friends and co-workers.LinksCogneeMontenegroCatastrophic ForgettingMulti-Turn InteractionRAG == Retrieval Augmented GenerationPodcast EpisodeGraphRAGPodcast EpisodeLong-term memoryShort-term memoryLangchainLlamaIndexHaystackdltData Engineering Podcast EpisodePineconePodcast EpisodeAgentic RAGAirflowDAG == Directed Acyclic GraphFalkorDBNeo4JPydanticAWS ECSAWS SNSAWS SQSAWS LambdaLLM As JudgeMem0QDrantLanceDBDuckDBThe intro and outro music is from Hitman's Lovesong feat. Paola Graziano by The Freak Fandango Orchestra/CC BY-SA 3.0
SummaryIn this episode of the AI Engineering Podcast, Tanner Burson, VP of Engineering at Prismatic, talks about the evolving impact of generative AI on software developers. Tanner shares his insights from engineering leadership and data engineering initiatives, discussing how AI is blurring the lines of developer roles and the strategic value of AI in software development. He explores the current landscape of AI tools, such as GitHub's Copilot, and their influence on productivity and workflow, while also touching on the challenges and opportunities presented by AI in code generation, review, and tooling. Tanner emphasizes the need for human oversight to maintain code quality and security, and offers his thoughts on the future of AI in development, the importance of balancing innovation with practicality, and the evolving role of engineers in an AI-driven landscape.AnnouncementsHello and welcome to the AI Engineering Podcast, your guide to the fast-moving world of building scalable and maintainable AI systemsYour host is Tobias Macey and today I'm interviewing Tanner Burson about the impact of generative AI on software developersInterviewIntroductionHow did you get involved in machine learning?Can you describe what types of roles and work you consider encompassed by the term "developers" for the purpose of this conversation?How does your work at Prismatic give you visibility and insight into the effects of AI on developers and their work?There have been many competing narratives about AI and how much of the software development process it is capable of encompassing. What is your top-level view on what the long-term impact on the job prospects of software developers will be as a result of generative AI?There are many obvious examples of utilities powered by generative AI that are focused on software development. What do you see as the categories or specific tools that are most impactful to the development cycle?In what ways do you find familiarity with/understanding of LLM internals useful when applying them to development processes?As an engineering leader, how are you evaluating and guiding your team on the use of AI powered tools?What are some of the risks that you are guarding against as a result of AI in the development process?What are the most interesting, innovative, or unexpected ways that you have seen AI used in the development process?What are the most interesting, unexpected, or challenging lessons that you have learned while using AI for software development?When is AI the wrong choice for a developer?What are your projections for the near to medium term impact on the developer experience as a result of generative AI?Contact InfoLinkedInParting QuestionFrom your perspective, what are the biggest gaps in tooling, technology, or training for AI systems today?Closing AnnouncementsThank you for listening! Don't forget to check out our other shows. The Data Engineering Podcast covers the latest on modern data management. Podcast.__init__ covers the Python language, its community, and the innovative ways it is being used.Visit the site to subscribe to the show, sign up for the mailing list, and read the show notes.If you've learned something or tried out a project from the show then tell us about it! Email hosts@aiengineeringpodcast.com with your story.To help other people find the show please leave a review on iTunes and tell your friends and co-workers.LinksPrismaticGoogle AI Development announcementTabninePodcast EpisodeGitHub CopilotPlandexOpenAI APIAmazon QOllamaHuggingface TransformersAnthropicLangchainLlamaindexHaystackLlama 3.2Qwen2.5-CoderThe intro and outro music is from Hitman's Lovesong feat. Paola Graziano by The Freak Fandango Orchestra/CC BY-SA 3.0
SummaryMachine learning workflows have long been complex and difficult to operationalize. They are often characterized by a period of research, resulting in an artifact that gets passed to another engineer or team to prepare for running in production. The MLOps category of tools have tried to build a new set of utilities to reduce that friction, but have instead introduced a new barrier at the team and organizational level. Donny Greenberg took the lessons that he learned on the PyTorch team at Meta and created Runhouse. In this episode he explains how, by reducing the number of opinions in the framework, he has also reduced the complexity of moving from development to production for ML systems.AnnouncementsHello and welcome to the AI Engineering Podcast, your guide to the fast-moving world of building scalable and maintainable AI systemsYour host is Tobias Macey and today I'm interviewing Donny Greenberg about Runhouse and the current state of ML infrastructureInterviewIntroductionHow did you get involved in machine learning?What are the core elements of infrastructure for ML and AI?How has that changed over the past ~5 years?For the past few years the MLOps and data engineering stacks were built and managed separately. How does the current generation of tools and product requirements influence the present and future approach to those domains?There are numerous projects that aim to bridge the complexity gap in running Python and ML code from your laptop up to distributed compute on clouds (e.g. Ray, Metaflow, Dask, Modin, etc.). How do you view the decision process for teams trying to understand which tool(s) to use for managing their ML/AI developer experience?Can you describe what Runhouse is and the story behind it?What are the core problems that you are working to solve?What are the main personas that you are focusing on? (e.g. data scientists, DevOps, data engineers, etc.)How does Runhouse factor into collaboration across skill sets and teams?Can you describe how Runhouse is implemented?How has the focus on developer experience informed the way that you think about the features and interfaces that you include in Runhouse?How do you think about the role of Runhouse in the integration with the AI/ML and data ecosystem?What does the workflow look like for someone building with Runhouse?What is involved in managing the coordination of compute and data locality to reduce networking costs and latencies?What are the most interesting, innovative, or unexpected ways that you have seen Runhouse used?What are the most interesting, unexpected, or challenging lessons that you have learned while working on Runhouse?When is Runhouse the wrong choice?What do you have planned for the future of Runhouse?What is your vision for the future of infrastructure and developer experience in ML/AI?Contact InfoLinkedInParting QuestionFrom your perspective, what are the biggest gaps in tooling, technology, or training for AI systems today?Closing AnnouncementsThank you for listening! Don't forget to check out our other shows. The Data Engineering Podcast covers the latest on modern data management. Podcast.__init__ covers the Python language, its community, and the innovative ways it is being used.Visit the site to subscribe to the show, sign up for the mailing list, and read the show notes.If you've learned something or tried out a project from the show then tell us about it! Email hosts@aiengineeringpodcast.com with your story.To help other people find the show please leave a review on iTunes and tell your friends and co-workers.LinksRunhouseGitHubPyTorchPodcast.__init__ EpisodeKubernetesBin PackingLinear RegressionGradient Boosted Decision TreeDeep LearningTransformer Architecture)SlurmSagemakerVertex AIMetaflowPodcast.__init__ EpisodeMLFlowDaskData Engineering Podcast EpisodeRayPodcast.__init__ EpisodeSparkDatabricksSnowflakeArgoCDPyTorch DistributedHorovodLlama.cppPrefectData Engineering Podcast EpisodeAirflowOOM == Out of MemoryWeights and BiasesKNativeBERT language modelThe intro and outro music is from Hitman's Lovesong feat. Paola Graziano by The Freak Fandango Orchestra/CC BY-SA 3.0
SummaryWith the growth of vector data as a core element of any AI application comes the need to keep those vectors up to date. When you go beyond prototypes and into production you will need a way to continue experimenting with new embedding models, chunking strategies, etc. You will also need a way to keep the embeddings up to date as your data changes. The team at Timescale created the pgai Vectorizer toolchain to let you manage that work in your Postgres database. In this episode Avthar Sewrathan explains how it works and how you can start using it today.AnnouncementsHello and welcome to the AI Engineering Podcast, your guide to the fast-moving world of building scalable and maintainable AI systemsYour host is Tobias Macey and today I'm interviewing Avthar Sewrathan about the pgai extension for Postgres and how to run your AI workflows in your databaseInterviewIntroductionHow did you get involved in machine learning?Can you describe what pgai Vectorizer is and the story behind it?What are the benefits of using the database engine to execute AI workflows?What types of operations does pgai Vectorizer enable?What are some common generative AI patterns that can't be done with pgai?AI applications require a large and complex set of dependencies. How does that work with pgai Vectorizer and the Python runtime in Postgres?What are some of the other challenges or system pressures that are introduced by running these AI workflows in the database context?Can you describe how the pgai extension is implemented?With the rapid pace of change in the AI ecosystem, how has that informed the set of features that make sense in pgai Vectorizer and won't require rebuilding in 6 months?Can you describe the workflow of using pgai Vectorizer to build and maintain a set of embeddings in their database?How can pgai Vectorizer help with the situation of migrating to a new embedding model and having to reindex all of the content?How do you think about the developer experience for people who are working with pgai Vectorizer, as compared to using e.g. LangChain, LlamaIndex, etc.?What are the most interesting, innovative, or unexpected ways that you have seen pgai Vectorizer used?What are the most interesting, unexpected, or challenging lessons that you have learned while working on pgai Vectorizer?When is pgai Vectorizer the wrong choice?What do you have planned for the future of pgai Vectorizer?Contact InfoLinkedInWebsiteParting QuestionFrom your perspective, what are the biggest gaps in tooling, technology, or training for AI systems today?Closing AnnouncementsThank you for listening! Don't forget to check out our other shows. The Data Engineering Podcast covers the latest on modern data management. Podcast.__init__ covers the Python language, its community, and the innovative ways it is being used.Visit the site to subscribe to the show, sign up for the mailing list, and read the show notes.If you've learned something or tried out a project from the show then tell us about it! Email hosts@aiengineeringpodcast.com with your story.To help other people find the show please leave a review on iTunes and tell your friends and co-workers.LinksTimescalepgaiTransformer architecture for deep learningNeural NetworkspgvectorpgvectorscaleModalRAG == Retrieval Augmented GenerationSemantic SearchOllamaGraphRAGagensgraphLangChainLlamaIndexHaystackIVFFlatHNSWDiskANNRepl.it AgentBM25TSVectorParadeDBThe intro and outro music is from Hitman's Lovesong feat. Paola Graziano by The Freak Fandango Orchestra/CC BY-SA 3.0
SummaryIn this episode Philip Kiely from BaseTen talks about the intricacies of running open models in production. Philip shares his journey into AI and ML engineering, highlighting the importance of understanding product-level requirements and selecting the right model for deployment. The conversation covers the operational aspects of deploying AI models, including model evaluation, compound AI, and model serving frameworks such as TensorFlow Serving and AWS SageMaker. Philip also discusses the challenges of model quantization, rapid model evolution, and monitoring and observability in AI systems, offering valuable insights into the future trends in AI, including local inference and the competition between open source and proprietary models.AnnouncementsHello and welcome to the AI Engineering Podcast, your guide to the fast-moving world of building scalable and maintainable AI systemsYour host is Tobias Macey and today I'm interviewing Philip Kiely about running open models in productionInterviewIntroductionHow did you get involved in machine learning?Can you start by giving an overview of the major decisions to be made when planning the deployment of a generative AI model?How does the model selected in the beginning of the process influence the downstream choices?In terms of application architecture, the major patterns that I've seen are RAG, fine-tuning, multi-agent, or large model. What are the most common methods that you see? (and any that I failed to mention)How have the rapid succession of model generations impacted the ways that teams think about their overall application? (capabilities, features, architecture, etc.)In terms of model serving, I know that Baseten created Truss. What are some of the other notable options that teams are building with?What is the role of the serving framework in the context of the application?There are also a large number of inference engines that have been released. What are the major players in that arena?What are the features and capabilities that they are each basing their competitive advantage on?For someone who is new to AI Engineering, what are some heuristics that you would recommend when choosing an inference engine?Once a model (or set of models) is in production and serving traffic it's necessary to have visibility into how it is performing. What are the key metrics that are necessary to monitor for generative AI systems?In the event that one (or more) metrics are trending negatively, what are the levers that teams can pull to improve them?When running models constructed with e.g. linear regression or deep learning there was a common issue with "concept drift". How does that manifest in the context of large language models, particularly when coupled with performance optimization?What are the most interesting, innovative, or unexpected ways that you have seen teams manage the serving of open gen AI models?What are the most interesting, unexpected, or challenging lessons that you have learned while working with generative AI model serving?When is Baseten the wrong choice?What are the future trends and technology investments that you are focused on in the space of AI model serving?Contact InfoLinkedInTwitterParting QuestionFrom your perspective, what are the biggest gaps in tooling, technology, or training for AI systems today?Closing AnnouncementsThank you for listening! Don't forget to check out our other shows. The Data Engineering Podcast covers the latest on modern data management. Podcast.__init__ covers the Python language, its community, and the innovative ways it is being used.Visit the site to subscribe to the show, sign up for the mailing list, and read the show notes.If you've learned something or tried out a project from the show then tell us about it! Email hosts@aiengineeringpodcast.com with your story.To help other people find the show please leave a review on iTunes and tell your friends and co-workers.LinksBasetenPodcast EpisodeCopyleftLlama ModelsNomicOlmoAllen Institute for AIPlayground 2The Peace Dividend Of The SaaS WarsVercelNetlifyRAG == Retrieval Augmented GenerationPodcast EpisodeCompound AILangchainOutlines Structured output for AI systemsTrussChainsLlamaindexRayMLFlowCog (Replicate) containers for MLBentoMLDjangoWSGIuWSGIGunicornZapiervLLMTensorRT-LLMTensorRTQuantizationLoRA Low Rank Adaptation of Large Language ModelsPruningDistillationGrafanaSpeculative DecodingGroqRunpodLambda LabsThe intro and outro music is from Hitman's Lovesong feat. Paola Graziano by The Freak Fandango Orchestra/CC BY-SA 3.0
SummaryIn this episode of the AI Engineering podcast, Philip Rathle, CTO of Neo4J, talks about the intersection of knowledge graphs and AI retrieval systems, specifically Retrieval Augmented Generation (RAG). He delves into GraphRAG, a novel approach that combines knowledge graphs with vector-based similarity search to enhance generative AI models. Philip explains how GraphRAG works by integrating a graph database for structured data storage, providing more accurate and explainable AI responses, and addressing limitations of traditional retrieval systems. The conversation covers technical aspects such as data modeling, entity extraction, and ontology use cases, as well as the infrastructure and workflow required to support GraphRAG, setting the stage for innovative applications across various industries.AnnouncementsHello and welcome to the AI Engineering Podcast, your guide to the fast-moving world of building scalable and maintainable AI systemsYour host is Tobias Macey and today I'm interviewing Philip Rathle about the application of knowledge graphs in AI retrieval systemsInterviewIntroductionHow did you get involved in machine learning?Can you describe what GraphRAG is?What are the capabilities that graph structures offer beyond vector/similarity-based retrieval methods of prompting?What are some examples of the ways that semantic limitations of nearest-neighbor vector retrieval fail to provide relevant results?What are the technical requirements to implement graph-augmented retrieval?What are the concrete ways in which the embedding and retrieval steps of a typical RAG pipeline need to be modified to account for the addition of the graph?Many tutorials for building vector-based knowledge repositories skip over considerations around data modeling. For building a graph-based knowledge repository there obviously needs to be a bit more work put in. What are the key design choices that need to be made for implementing the graph for an AI application?How does the selection of the ontology/taxonomy impact the performance and capabilities of the resulting application?Building a fully functional knowledge graph can be a significant undertaking on its own. How can LLMs and AI models help with the construction and maintenance of that knowledge repository?What are some of the validation methods that should be brought to bear to ensure that the resulting graph properly represents the knowledge domain that you are trying to model?Vector embedding and retrieval are a core building block for a majority of AI application frameworks. How much support do you see for GraphRAG in the ecosystem?For the case where someone is using a framework that does not explicitly implement GraphRAG techniques, what are some of the implementation strategies that you have seen be most effective for adding that functionality?What are some of the ways that the combination of vector search and knowledge graphs are useful independent of their combination with language models?What are the most interesting, innovative, or unexpected ways that you have seen GraphRAG used?What are the most interesting, unexpected, or challenging lessons that you have learned while working on GraphRAG applications?When is GraphRAG the wrong choice?What are the opportunities for improvement in the design and implementation of graph-based retrieval systems?Contact InfoLinkedInParting QuestionFrom your perspective, what are the biggest gaps in tooling, technology, or training for AI systems today?Closing AnnouncementsThank you for listening! Don't forget to check out our other shows. The Data Engineering Podcast covers the latest on modern data management. Podcast.__init__ covers the Python language, its community, and the innovative ways it is being used.Visit the site to subscribe to the show, sign up for the mailing list, and read the show notes.If you've learned something or tried out a project from the show then tell us about it! Email hosts@aiengineeringpodcast.com with your story.To help other people find the show please leave a review on iTunes and tell your friends and co-workers.LinksNeo4JGraphRAG ManifestoRAG == Retrieval Augmented GenerationPodcast EpisodeVLDB == Very Large DataBasesKnowledge GraphNearest Neighbor SearchPageRankThings Not Strings) Google Knowledge Graph PaperpgvectorPineconeData Engineering Podcast EpisodeTables To LabelsNLP == Natural Language ProcessingOntologyLangChainLlamaIndexRLHF == Reinforcement Learning with Human FeedbackSenzingNeoConverseCypher query languageGQL query standardAWS BedrockVertex AISequoia Training Data - Klarna episodeOuroborosThe intro and outro music is from Hitman's Lovesong feat. Paola Graziano by The Freak Fandango Orchestra/CC BY-SA 3.0
SummaryIn this episode of the AI Engineering podcast Praveen Gujar, Director of Product at LinkedIn, talks about the applications of generative AI in digital advertising. He highlights the key areas of digital advertising, including audience targeting, content creation, and ROI measurement, and delves into how generative AI is revolutionizing these aspects. Praveen shares successful case studies of generative AI in digital advertising, including campaigns by Heinz, the Barbie movie, and Maggi, and discusses the potential pitfalls and risks associated with AI-powered tools. He concludes with insights into the future of generative AI in digital advertising, highlighting the importance of cultural transformation and the synergy between human creativity and AI.AnnouncementsHello and welcome to the AI Engineering Podcast, your guide to the fast-moving world of building scalable and maintainable AI systemsYour host is Tobias Macey and today I'm interviewing Praveen Gujar about the applications of generative AI in digital advertisingInterviewIntroductionHow did you get involved in machine learning?Can you start by defining "digital advertising" for the scope of this conversation?What are the key elements/characteristics/goals of digital avertising?In the world before generative AI, what did a typical end-to-end advertising campaign workflow look like?What are the stages of that workflow where generative AI are proving to be most useful?How do the current limitations of generative AI (e.g. hallucinations, non-determinism) impact the ways in which they can be used?What are the technological and organizational systems that need to be implemented to effectively apply generative AI in public-facing applications that are so closely tied to brand/company image?What are the elements of user education/expectation setting that are necessary when working with marketing/advertising personnel to help avoid damage to the brands?What are some examples of applications for generative AI in digital advertising that have gone well?Any that have gone wrong?What are the most interesting, innovative, or unexpected ways that you have seen generative AI used in digital advertising?What are the most interesting, unexpected, or challenging lessons that you have learned while working on digital advertising applications of generative AI?When is generative AI the wrong choice?What are your future predictions for the use of generative AI in dgital advertising?Contact InfoWebsiteLinkedInParting QuestionFrom your perspective, what is the biggest barrier to adoption of machine learning today?Closing AnnouncementsThank you for listening! Don't forget to check out our other shows. The Data Engineering Podcast covers the latest on modern data management. Podcast.__init__ covers the Python language, its community, and the innovative ways it is being used.Visit the site to subscribe to the show, sign up for the mailing list, and read the show notes.If you've learned something or tried out a project from the show then tell us about it! Email hosts@aiengineeringpodcast.com with your story.To help other people find the show please leave a review on iTunes and tell your friends and co-workers.LinksGenerative AILLM == Large Language ModelDall-E)RLHF == Reinforcement Learning fHuman FeedbackThe intro and outro music is from Hitman's Lovesong feat. Paola Graziano by The Freak Fandango Orchestra/CC BY-SA 3.0
SummaryIn this episode of the AI Engineering podcast, host Tobias Macy interviews Tammer Saleh, founder of SuperOrbital, about the potentials and pitfalls of using Kubernetes for machine learning workloads. The conversation delves into the specific needs of machine learning workflows, such as model tracking, versioning, and the use of Jupyter Notebooks, and how Kubernetes can support these tasks. Tammer emphasizes the importance of a unified API for different teams and the flexibility Kubernetes provides in handling various workloads. Finally, Tammer offers advice for teams considering Kubernetes for their machine learning workloads and discusses the future of Kubernetes in the ML ecosystem, including areas for improvement and innovation.AnnouncementsHello and welcome to the AI Engineering Podcast, your guide to the fast-moving world of building scalable and maintainable AI systemsYour host is Tobias Macey and today I'm interviewing Tammer Saleh about the potentials and pitfalls of using Kubernetes for your ML workloads.InterviewIntroductionHow did you get involved in Kubernetes?For someone who is unfamiliar with Kubernetes, how would you summarize it?For the context of this conversation, can you describe the different phases of ML that we're talking about?Kubernetes was originally designed to handle scaling and distribution of stateless processes. ML is an inherently stateful problem domain. What challenges does that add for K8s environments?What are the elements of an ML workflow that lend themselves well to a Kubernetes environment?How much Kubernetes knowledge does an ML/data engineer need to know to get their work done?What are the sharp edges of Kubernetes in the context of ML projects?What are the most interesting, unexpected, or challenging lessons that you have learned while working with Kubernetes?When is Kubernetes the wrong choice for ML?What are the aspects of Kubernetes (core or the ecosystem) that you are keeping an eye on which will help improve its utility for ML workloads?Contact InfoEmailLinkedInParting QuestionFrom your perspective, what is the biggest gap in the tooling or technology for ML workloads today?LinksSuperOrbitalCloudFoundryHeroku12 Factor ModelKubernetesDocker ComposeCore K8s ClassJupyter NotebookCrossplaneOchre JellyCNCF (Cloud Native Computing Foundation) LandscapeStateful SetRAG == Retrieval Augmented GenerationPodcast EpisodeKubeflowFlyteData Engineering Podcast EpisodePachydermData Engineering Podcast EpisodeCoreWeaveKubectl ("koob-cuddle")HelmCRD == Custom Resource DefinitionHorovodPodcast.__init__ EpisodeTemporalSlurmRayDaskInfinibandThe intro and outro music is from The Hug by The Freak Fandango Orchestra / CC BY-SA
SummaryIn this episode we're joined by Matt Zeiler, founder and CEO of Clarifai, as he dives into the technical aspects of retrieval augmented generation (RAG). From his journey into AI at the University of Toronto to founding one of the first deep learning AI companies, Matt shares his insights on the evolution of neural networks and generative models over the last 15 years. He explains how RAG addresses issues with large language models, including data staleness and hallucinations, by providing dynamic access to information through vector databases and embedding models. Throughout the conversation, Matt and host Tobias Macy discuss everything from architectural requirements to operational considerations, as well as the practical applications of RAG in industries like intelligence, healthcare, and finance. Tune in for a comprehensive look at RAG and its future trends in AI.AnnouncementsHello and welcome to the AI Engineering Podcast, your guide to the fast-moving world of building scalable and maintainable AI systemsYour host is Tobias Macey and today I'm interviewing Matt Zeiler, Founder & CEO of Clarifai, about the technical aspects of RAG, including the architectural requirements, edge cases, and evolutionary characteristicsInterviewIntroductionHow did you get involved in the area of data management?Can you describe what RAG (Retrieval Augmented Generation) is?What are the contexts in which you would want to use RAG?What are the alternatives to RAG?What are the architectural/technical components that are required for production grade RAG?Getting a quick proof-of-concept working for RAG is fairly straightforward. What are the failures modes/edge cases that start to surface as you scale the usage and complexity?The first step of building the corpus for RAG is to generate the embeddings. Can you talk through the planning and design process? (e.g. model selection for embeddings, storage capacity/latency, etc.)How does the modality of the input/output affect this and downstream decisions? (e.g. text vs. image vs. audio, etc.)What are the features of a vector store that are most critical for RAG?The set of available generative models is expanding and changing at breakneck speed. What are the foundational aspects that you look for in selecting which model(s) to use for the output?Vector databases have been gaining ground for search functionality, even without generative AI. What are some of the other ways that elements of RAG can be re-purposed?What are the most interesting, innovative, or unexpected ways that you have seen RAG used?What are the most interesting, unexpected, or challenging lessons that you have learned while working on RAG?When is RAG the wrong choice?What are the main trends that you are following for RAG and its component elements going forward?Contact InfoWebsiteLinkedInParting QuestionFrom your perspective, what is the biggest barrier to adoption of machine learning today?Closing AnnouncementsThank you for listening! Don't forget to check out our other shows. The Data Engineering Podcast covers the latest on modern data management. [Podcast.__init__]() covers the Python language, its community, and the innovative ways it is being used.Visit the site to subscribe to the show, sign up for the mailing list, and read the show notes.If you've learned something or tried out a project from the show then tell us about it! Email hosts@aiengineeringpodcast.com with your story.To help other people find the show please leave a review on iTunes and tell your friends and co-workers.LinksClarifaiGeoff HintonYann LecunNeural NetworksDeep LearningRetrieval Augmented GenerationContext WindowVector DatabasePrompt EngineeringMistralLlama 3Embedding QuantizationActive LearningGoogle GeminiAI Model AttentionRecurrent NetworkConvolutional NetworkReranking ModelStop WordsMassive Text Embedding Benchmark (MTEB)Retool State of AI ReportpgvectorMilvusQdrantPineconeOpenLLM LeaderboardSemantic SearchHashicorpThe intro and outro music is from Hitman's Lovesong feat. Paola Graziano by The Freak Fandango Orchestra/CC BY-SA 3.0
SummaryArtificial intelligence has dominated the headlines for several months due to the successes of large language models. This has prompted numerous debates about the possibility of, and timeline for, artificial general intelligence (AGI). Peter Voss has dedicated decades of his life to the pursuit of truly intelligent software through the approach of cognitive AI. In this episode he explains his approach to building AI in a more human-like fashion and the emphasis on learning rather than statistical prediction.AnnouncementsHello and welcome to the AI Engineering Podcast, your guide to the fast-moving world of building scalable and maintainable AI systemsYour host is Tobias Macey and today I'm interviewing Peter Voss about what is involved in making your AI applications more "human"InterviewIntroductionHow did you get involved in machine learning?Can you start by unpacking the idea of "human-like" AI?How does that contrast with the conception of "AGI"?The applications and limitations of GPT/LLM models have been dominating the popular conversation around AI. How do you see that impacting the overrall ecosystem of ML/AI applications and investment?The fundamental/foundational challenge of every AI use case is sourcing appropriate data. What are the strategies that you have found useful to acquire, evaluate, and prepare data at an appropriate scale to build high quality models? What are the opportunities and limitations of causal modeling techniques for generalized AI models?As AI systems gain more sophistication there is a challenge with establishing and maintaining trust. What are the risks involved in deploying more human-level AI systems and monitoring their reliability?What are the practical/architectural methods necessary to build more cognitive AI systems?How would you characterize the ecosystem of tools/frameworks available for creating, evolving, and maintaining these applications?What are the most interesting, innovative, or unexpected ways that you have seen cognitive AI applied?What are the most interesting, unexpected, or challenging lessons that you have learned while working on desiging/developing cognitive AI systems?When is cognitive AI the wrong choice?What do you have planned for the future of cognitive AI applications at Aigo?Contact InfoLinkedInWebsiteParting QuestionFrom your perspective, what is the biggest barrier to adoption of machine learning today?Closing AnnouncementsThank you for listening! Don't forget to check out our other shows. The Data Engineering Podcast covers the latest on modern data management. Podcast.__init__ covers the Python language, its community, and the innovative ways it is being used.Visit the site to subscribe to the show, sign up for the mailing list, and read the show notes.If you've learned something or tried out a project from the show then tell us about it! Email hosts@aiengineeringpodcast.com with your story.To help other people find the show please leave a review on iTunes and tell your friends and co-workers.LinksAigo.aiArtificial General IntelligenceCognitive AIKnowledge GraphCausal ModelingBayesian StatisticsThinking Fast & Slow by Daniel Kahneman (affiliate link)Agent-Based ModelingReinforcement LearningDARPA 3 Waves of AI presentationWhy Don't We Have AGI Yet? whitepaperConcepts Is All You Need WhitepaperHellen KellerStephen HawkingThe intro and outro music is from Hitman's Lovesong feat. Paola Graziano by The Freak Fandango Orchestra/CC BY-SA 3.0
SummaryGenerative AI promises to accelerate the productivity of human collaborators. Currently the primary way of working with these tools is through a conversational prompt, which is often cumbersome and unwieldy. In order to simplify the integration of AI capabilities into developer workflows Tsavo Knott helped create Pieces, a powerful collection of tools that complements the tools that developers already use. In this episode he explains the data collection and preparation process, the collection of model types and sizes that work together to power the experience, and how to incorporate it into your workflow to act as a second brain.AnnouncementsHello and welcome to the AI Engineering Podcast, your guide to the fast-moving world of building scalable and maintainable AI systemsYour host is Tobias Macey and today I'm interviewing Tsavo Knott about Pieces, a personal AI toolkit to improve the efficiency of developersInterviewIntroductionHow did you get involved in machine learning?Can you describe what Pieces is and the story behind it?The past few months have seen an endless series of personalized AI tools launched. What are the features and focus of Pieces that might encourage someone to use it over the alternatives?model selectionsarchitecture of Pieces applicationlocal vs. hybrid vs. online modelsmodel update/delivery processdata preparation/serving for models in context of Pieces appapplication of AI to developer workflowstypes of workflows that people are building with piecesWhat are the most interesting, innovative, or unexpected ways that you have seen Pieces used?What are the most interesting, unexpected, or challenging lessons that you have learned while working on Pieces?When is Pieces the wrong choice?What do you have planned for the future of Pieces?Contact InfoLinkedInParting QuestionFrom your perspective, what is the biggest barrier to adoption of machine learning today?Closing AnnouncementsThank you for listening! Don't forget to check out our other shows. The Data Engineering Podcast covers the latest on modern data management. Podcast.__init__ covers the Python language, its community, and the innovative ways it is being used.Visit the site to subscribe to the show, sign up for the mailing list, and read the show notes.If you've learned something or tried out a project from the show then tell us about it! Email hosts@aiengineeringpodcast.com with your story.To help other people find the show please leave a review on iTunes and tell your friends and co-workers.LinksPiecesNPU == Neural Processing UnitTensor ChipLoRA == Low Rank AdaptationGenerative Adversarial NetworksMistralEmacsVimNeoVimDartFlutterTypescriptLuaRetrieval Augmented GenerationONNXLSTM == Long Short-Term MemoryLLama 2GitHub CopilotTabninePodcast EpisodeThe intro and outro music is from Hitman's Lovesong feat. Paola Graziano by The Freak Fandango Orchestra/CC BY-SA 3.0
SummaryLarge Language Models (LLMs) have rapidly captured the attention of the world with their impressive capabilities. Unfortunately, they are often unpredictable and unreliable. This makes building a product based on their capabilities a unique challenge. Jignesh Patel is building DataChat to bring the capabilities of LLMs to organizational analytics, allowing anyone to have conversations with their business data. In this episode he shares the methods that he is using to build a product on top of this constantly shifting set of technologies.AnnouncementsHello and welcome to the Machine Learning Podcast, the podcast about machine learning and how to bring it from idea to delivery.Your host is Tobias Macey and today I'm interviewing Jignesh Patel about working with LLMs; understanding how they work and how to build your ownInterviewIntroductionHow did you get involved in machine learning?Can you start by sharing some of the ways that you are working with LLMs currently?What are the business challenges involved in building a product on top of an LLM model that you don't own or control? In the current age of business, your data is often your strategic advantage. How do you avoid losing control of, or leaking that data while interfacing with a hosted LLM API?What are the technical difficulties related to using an LLM as a core element of a product when they are largely a black box? What are some strategies for gaining visibility into the inner workings or decision making rules for these models?What are the factors, whether technical or organizational, that might motivate you to build your own LLM for a business or product? Can you unpack what it means to "build your own" when it comes to an LLM?In your work at DataChat, how has the progression of sophistication in LLM technology impacted your own product strategy?What are the most interesting, innovative, or unexpected ways that you have seen LLMs/DataChat used?What are the most interesting, unexpected, or challenging lessons that you have learned while working with LLMs?When is an LLM the wrong choice?What do you have planned for the future of DataChat?Contact InfoWebsiteLinkedInParting QuestionFrom your perspective, what is the biggest barrier to adoption of machine learning today?Closing AnnouncementsThank you for listening! Don't forget to check out our other shows. The Data Engineering Podcast covers the latest on modern data management. Podcast.__init__ covers the Python language, its community, and the innovative ways it is being used.Visit the site to subscribe to the show, sign up for the mailing list, and read the show notes.If you've learned something or tried out a project from the show then tell us about it! Email hosts@themachinelearningpodcast.com) with your story.To help other people find the show please leave a review on iTunes and tell your friends and co-workers.LinksDataChatCMU == Carnegie Mellon UniversitySVM == Support Vector MachineGenerative AIGenomicsProteomicsParquetOpenAI CodexLLamaMistralGoogle VertexLangchainRetrieval Augmented GenerationPrompt EngineeringEnsemble LearningXGBoostCatboostLinear RegressionCOGS == Cost Of Goods SoldBruce Schneier - AI And TrustThe intro and outro music is from Hitman's Lovesong feat. Paola Graziano by The Freak Fandango Orchestra/CC BY-SA 3.0
SummaryMachine learning is a powerful set of technologies, holding the potential to dramatically transform businesses across industries. Unfortunately, the implementation of ML projects often fail to achieve their intended goals. This failure is due to a lack of collaboration and investment across technological and organizational boundaries. To help improve the success rate of machine learning projects Eric Siegel developed the six step bizML framework, outlining the process to ensure that everyone understands the whole process of ML deployment. In this episode he shares the principles and promise of that framework and his motivation for encapsulating it in his book "The AI Playbook".AnnouncementsHello and welcome to the Machine Learning Podcast, the podcast about machine learning and how to bring it from idea to delivery.Your host is Tobias Macey and today I'm interviewing Eric Siegel about how the bizML approach can help improve the success rate of your ML projectsInterviewIntroductionHow did you get involved in machine learning?Can you describe what bizML is and the story behind it? What are the key aspects of this approach that are different from the "industry standard" lifecycle of an ML project?What are the elements of your personal experience as an ML consultant that helped you develop the tenets of bizML?Who are the personas that need to be involved in an ML project to increase the likelihood of success? Who do you find to be best suited to "own" or "lead" the process?What are the organizational patterns that might hinder the work of delivering on the goals of an ML initiative?What are some of the misconceptions about the work involved in/capabilities of an ML model that you commonly encounter?What is your main goal in writing your book "The AI Playbook"?What are the most interesting, innovative, or unexpected ways that you have seen the bizML process in action?What are the most interesting, unexpected, or challenging lessons that you have learned while working on ML projects and developing the bizML framework?When is bizML the wrong choice?What are the future developments in organizational and technical approaches to ML that will improve the success rate of AI projects?Contact InfoLinkedInParting QuestionFrom your perspective, what is the biggest barrier to adoption of machine learning today?Closing AnnouncementsThank you for listening! Don't forget to check out our other shows. The Data Engineering Podcast covers the latest on modern data management. Podcast.__init__ covers the Python language, its community, and the innovative ways it is being used.Visit the site to subscribe to the show, sign up for the mailing list, and read the show notes.If you've learned something or tried out a project from the show then tell us about it! Email hosts@themachinelearningpodcast.com) with your story.To help other people find the show please leave a review on iTunes and tell your friends and co-workers.LinksThe AI Playbook: Mastering the Rare Art of Machine Learning Deployment by Eric SiegelPredictive Analytics: The Power to Predict Who Will Click, Buy, Lie, or Die by Eric SiegelColumbia UniversityMachine Learning Week ConferenceGenerative AI WorldMachine Learning Leadership and Practice CourseRexer AnalyticsKD NuggetsCRISP-DMRandom ForestGradient DescentThe intro and outro music is from Hitman's Lovesong feat. Paola Graziano by The Freak Fandango Orchestra/CC BY-SA 3.0
SummaryOne of the most time consuming aspects of building a machine learning model is feature engineering. Generative AI offers the possibility of accelerating the discovery and creation of feature pipelines. In this episode Colin Priest explains how FeatureByte is applying generative AI models to the challenge of building and maintaining machine learning pipelines.AnnouncementsHello and welcome to the Machine Learning Podcast, the podcast about machine learning and how to bring it from idea to delivery.Your host is Tobias Macey and today I'm interviewing Colin Priest about applying generative AI to the task of building and deploying AI pipelinesInterviewIntroductionHow did you get involved in machine learning?Can you start by giving the 30,000 foot view of the steps involved in an AI pipeline? Understand the problemFeature ideationFeature engineeringExperimentOptimizeProductionizeWhat are the stages of that process that are prone to repetition? What are the ways that teams typically try to automate those steps?What are the features of generative AI models that can be brought to bear on the design stage of an AI pipeline? What are the validation/verification processes that engineers need to apply to the generated suggestions?What are the opportunities/limitations for unit/integration style tests?What are the elements of developer experience that need to be addressed to make the gen AI capabilities an enhancement instead of a distraction? What are the interfaces through which the AI functionality can/should be exposed?What are the aspects of pipeline and model deployment that can benefit from generative AI functionality? What are the potential risk factors that need to be considered when evaluating the application of this functionality?What are the most interesting, innovative, or unexpected ways that you have seen generative AI used in the development and maintenance of AI pipelines?What are the most interesting, unexpected, or challenging lessons that you have learned while working on the application of generative AI to the ML workflow?When is generative AI the wrong choice?What do you have planned for the future of FeatureByte's AI copilot capabiliteis?Contact InfoLinkedInParting QuestionFrom your perspective, what is the biggest barrier to adoption of machine learning today?Closing AnnouncementsThank you for listening! Don't forget to check out our other shows. The Data Engineering Podcast covers the latest on modern data management. Podcast.__init__ covers the Python language, its community, and the innovative ways it is being used.Visit the site to subscribe to the show, sign up for the mailing list, and read the show notes.If you've learned something or tried out a project from the show then tell us about it! Email hosts@themachinelearningpodcast.com) with your story.To help other people find the show please leave a review on iTunes and tell your friends and co-workers.LinksFeatureByteGenerative AIThe Art of WarOCR == Optical Character RecognitionGenetic AlgorithmSemantic LayerPrompt EngineeringThe intro and outro music is from Hitman's Lovesong feat. Paola Graziano by The Freak Fandango Orchestra/CC BY-SA 3.0Support The Machine Learning Podcast
SummaryEvery business develops their own specific workflows to address their internal organizational needs. Not all of them are properly documented, or even visible. Workflow automation tools have tried to reduce the manual burden involved, but they are rigid and require substantial investment of time to discover and develop the routines. Boaz Hecht co-founded 8Flow to iteratively discover and automate pieces of workflows, bringing visibility and collaboration to the internal organizational processes that keep the business running.AnnouncementsHello and welcome to the Machine Learning Podcast, the podcast about machine learning and how to bring it from idea to delivery.Your host is Tobias Macey and today I'm interviewing Boaz Hecht about using AI to automate customer support at 8FlowInterviewIntroductionHow did you get involved in machine learning?Can you describe what 8Flow is and the story behind it?How does 8Flow compare to RPA tools that companies are using today? What are the opportunities for augmenting or integrating with RPA frameworks?What are the key selling points for the solution that you are building? (does AI sell? Or is it about the realized savings?)What are the sources of signal that you are relying on to build model features?Given the heterogeneity in tools and processes across customers, what are the common focal points that let you address the widest possible range of functionality?Can you describe how 8Flow is implemented? How have the design and goals evolved since you first started working on it?What are the model categories that are most relevant for process automation in your product?How have you approached the design and implementation of your MLOps workflow? (model training, deployment, monitoring, versioning, etc.)What are the open questions around product focus and system design that you are still grappling with?Given the relative recency of ML/AI as a profession and the massive growth in attention and activity, how are you addressing the challenge of obtaining and maximizing human talent?What are the most interesting, innovative, or unexpected ways that you have seen 8Flow used?What are the most interesting, unexpected, or challenging lessons that you have learned while working on 8Flow?When is 8Flow the wrong choice?What do you have planned for the future of 8Flow?Contact InfoLinkedInPersonal WebsiteParting QuestionFrom your perspective, what is the biggest barrier to adoption of machine learning today?Closing AnnouncementsThank you for listening! Don't forget to check out our other shows. The Data Engineering Podcast covers the latest on modern data management. Podcast.__init__ covers the Python language, its community, and the innovative ways it is being used.Visit the site to subscribe to the show, sign up for the mailing list, and read the show notes.If you've learned something or tried out a project from the show then tell us about it! Email hosts@themachinelearningpodcast.com) with your story.To help other people find the show please leave a review on iTunes and tell your friends and co-workers.Links8FlowRobotic Process AutomationThe intro and outro music is from Hitman's Lovesong feat. Paola Graziano by The Freak Fandango Orchestra/CC BY-SA 3.0Support The Machine Learning Podcast
SummaryMachine learning and AI applications hold the promise of drastically impacting every aspect of modern life. With that potential for profound change comes a responsibility for the creators of the technology to account for the ramifications of their work. In this episode Nicholas Cifuentes-Goodbody guides us through the minefields of social, technical, and ethical considerations that are necessary to ensure that this next generation of technical and economic systems are equitable and beneficial for the people that they impact.AnnouncementsHello and welcome to the Machine Learning Podcast, the podcast about machine learning and how to bring it from idea to delivery.Your host is Tobias Macey and today I'm interviewing Nicholas Cifuentes-Goodbody about the different elements of the machine learning workflow where ethics need to be consideredInterviewIntroductionHow did you get involved in machine learning?To start with, who is responsible for addressing the ethical concerns around AI?What are the different ways that AI can have positive or negative outcomes from an ethical perspective? What is the role of practitioners/individual contributors in the identification and evaluation of ethical impacts of their work?What are some utilities that are helpful in identifying and addressing bias in training data?How can practitioners address challenges of equity and accessibility in the delivery of AI products?What are some of the options for reducing the energy consumption for training and serving AI?What are the most interesting, innovative, or unexpected ways that you have seen ML teams incorporate ethics into their work?What are the most interesting, unexpected, or challenging lessons that you have learned while working on ethical implications of ML?What are some of the resources that you recommend for people who want to invest in their knowledge and application of ethics in the realm of ML?Contact InfoWorldQuant University's Applied Data Science LabLinkedInParting QuestionFrom your perspective, what is the biggest barrier to adoption of machine learning today?Closing AnnouncementsThank you for listening! Don't forget to check out our other shows. The Data Engineering Podcast covers the latest on modern data management. Podcast.__init__ covers the Python language, its community, and the innovative ways it is being used.Visit the site to subscribe to the show, sign up for the mailing list, and read the show notes.If you've learned something or tried out a project from the show then tell us about it! Email hosts@themachinelearningpodcast.com) with your story.To help other people find the show please leave a review on iTunes and tell your friends and co-workers.LinksUNESCO Recommendation on the Ethics of Artificial IntelligenceEuropean Union AI ActHow machine learning helps advance access to human rights informationDisinformation, Team JorgeChina, AI, and Human RightsHow China Is Using A.I. to Profile a MinorityWeapons of Math DestructionFairlearnAI Fairness 360Allen Institute for AI NYTAllen Institute for AITransformersAI4ALLWorldQuant UniversityHow to Make Generative AI GreenerMachine Learning Emissions CalculatorPracticing Trustworthy Machine LearningEnergy and Policy Considerations for Deep LearningNatural Language ProcessingTrolley ProblemProtected Classesfairlearn (scikit-learn)BERT ModelThe intro and outro music is from Hitman's Lovesong feat. Paola Graziano by The Freak Fandango Orchestra/CC BY-SA 3.0
SummaryBuilding machine learning systems and other intelligent applications are a complex undertaking. This often requires retrieving data from a warehouse engine, adding an extra barrier to every workflow. The RelationalAI engine was built as a co-processor for your data warehouse that adds a greater degree of flexibility in the representation and analysis of the underlying information, simplifying the work involved. In this episode CEO Molham Aref explains how RelationalAI is designed, the capabilities that it adds to your data clouds, and how you can start using it to build more sophisticated applications on your data.AnnouncementsHello and welcome to the Machine Learning Podcast, the podcast about machine learning and how to bring it from idea to delivery.Your host is Tobias Macey and today I'm interviewing Molham Aref about RelationalAI and the principles behind it for powering intelligent applicationsInterviewIntroductionHow did you get involved in machine learning?Can you describe what RelationalAI is and the story behind it? On your site you call your product an "AI Co-processor". Can you explain what you mean by that phrase?What are the primary use cases that you address with the RelationalAI product? What are the types of solutions that teams might build to address those problems in the absence of something like the RelationalAI engine?Can you describe the system design of RelationalAI? How have the design and goals of the platform changed since you first started working on it?For someone who is using RelationalAI to address a business need, what does the onboarding and implementation workflow look like?What is your design philosophy for identifying the balance between automating the implementation of certain categories of application (e.g. NER) vs. providing building blocks and letting teams assemble them on their own?What are the data modeling paradigms that teams should be aware of to make the best use of the RKGS platform and Rel language?What are the aspects of customer education that you find yourself spending the most time on?What are some of the most under-utilized or misunderstood capabilities of the RelationalAI platform that you think deserve more attention?What are the most interesting, innovative, or unexpected ways that you have seen the RelationalAI product used?What are the most interesting, unexpected, or challenging lessons that you have learned while working on RelationalAI?When is RelationalAI the wrong choice?What do you have planned for the future of RelationalAI?Contact InfoLinkedInParting QuestionFrom your perspective, what is the biggest barrier to adoption of machine learning today?Closing AnnouncementsThank you for listening! Don't forget to check out our other shows. The Data Engineering Podcast covers the latest on modern data management. Podcast.__init__ covers the Python language, its community, and the innovative ways it is being used.Visit the site to subscribe to the show, sign up for the mailing list, and read the show notes.If you've learned something or tried out a project from the show then tell us about it! Email hosts@themachinelearningpodcast.com) with your story.To help other people find the show please leave a review on iTunes and tell your friends and co-workers.LinksRelationalAISnowflakeAI WinterBigQueryGradient DescentB-TreeNavigational DatabaseHadoopTeradataWorst Case Optimal JoinSemantic Query OptimizationRelational AlgebraHyperGraphLinear AlgebraVector DatabasePathwayData Engineering Podcast EpisodePineconeData Engineering Podcast EpisodeThe intro and outro music is from Hitman's Lovesong feat. Paola Graziano by The Freak Fandango Orchestra/CC BY-SA 3.0
Top Podcasts
The Best New Comedy Podcast Right Now – June 2024The Best News Podcast Right Now – June 2024The Best New Business Podcast Right Now – June 2024The Best New Sports Podcast Right Now – June 2024The Best New True Crime Podcast Right Now – June 2024The Best New Joe Rogan Experience Podcast Right Now – June 20The Best New Dan Bongino Show Podcast Right Now – June 20The Best New Mark Levin Podcast – June 2024
It's an informative post. You have https://www.kavyashinde.com/ shared such a unique and beneficial info with all of us.
Thanks for sharing this with so much of detailed information. https://www.westdelhiescorts.com/dwarka-escorts.html
Thanks for sharing this with so much of detailed information. https://digiclout.in/
thanks for sharing us .https://thenexturbanmillionaire.com/
Great Episode , Thanks from. https://cloutwebventures.com/
Great Episode. https://allthingsnatashaj.com/
Great Episode , Thanks from https://sarahjosbeauty.com/
Great Episode , Thanks from
Our VIP Delhi Escort Girls are renowned for their beauty and first-class services. We run a high-class escort agency and so all the girls we offer are specially selected and groomed to give the most personal and pleasurable experience for our clients. We have been in operation for a lot of years and have a wide network of independent experts who are the best in their field. Our experience has helped us understand what our clients need when they wish to hire an Delhi escorts. http://poojaescorts.in/
Every time men want to hold them and express their sexual emotions completely in front of gorgeous escorts,. For the ultimate pleasure, erotic blondes take every possible step that is full of adventure and brings happiness to their clienhttps://www.komaldas.com
Thanks from https://www.readymatrimony.com
Great Episode , Thanks from
Thanks for Your article...it gives us immense knowledge. This is a titanic mixing article. I am all around that genuinely matters content with your https://www.poonamaggarwal.co.in/city/chennai https://www.poonamaggarwal.co.in/city/hyderabad https://www.poonamaggarwal.co.in/city/indore https://www.poonamaggarwal.co.in/city/jodhpur
Book your appointment today through our website or give us a call to body massage parlour in hyderabad center, we provide best spa services with hot massage girl therapists
Our massage parlour is well cleaned, every operative has their hands and noses covered with the room sanitized after each session visit https://www.spa69.in/
Our spa working on happy and healthy therapies customer’s should get worthy service to there money this is our main aim. Safe spa in bangalore with top notch ambiance friendly therapist make a visit https://www.ishaspa.com/
The masseuse then uses their entire body to massage the recipient, sliding and gliding along their body in a rhythmic and sensual manner. body to body massage in chennai can be highly intimate and erotic, focusing on relaxation and pleasure https://vipbodyspa.com/
Very interesting post you have crafted hear. https://www.kolkatadolls.com/ I am blessed to have come across this resolutions post. Thanks.
Very interesting post you have crafted hear. I am blessed to have come across this resolutions post. Thanks. https://www.kolkatadolls.com/
nice post thanks for sharing, visit us: https://bodymassageinchennai.in/