Discover
Build a Career in Data Science
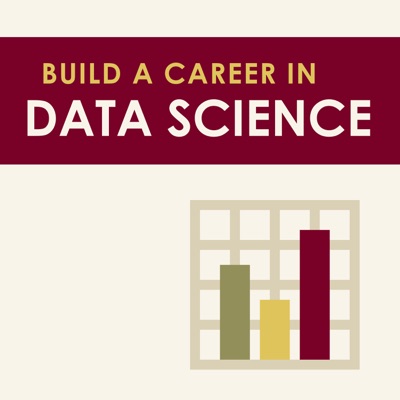
Build a Career in Data Science
Author: Jacqueline Nolis and Emily Robinson
Subscribed: 173Played: 1,777Subscribe
Share
© Jacqueline Nolis and Emily Robinson
Description
Build a Career in Data Science teaches you what data science courses leave out: from how to land your first job to the lifecycle of a data science project and even how to become a manager. This is a true how-to on obtaining and then navigating a data science career--filled with real stories from data scientists. This podcast is an extension of the similarly named book: Build a Career in Data Science.
22 Episodes
Reverse
This very surprise bonus episode was made after Emily and Jacqueline found themselves simultaneously unemployed. Here Emily will chat about being part of a 15% company-wide labor reduction while Jacqueline walks through the steps she's been doing as she interviews with new companies. Join them for some vulnerable conversation that are relevant to this current wave of layoffs.
In this special live episode recorded at PyLadies London, Emily and Jacqueline discuss those little subfields of data science like experimentation and fraud. They ponder the benefits of becoming more specialized in your career and how different fields have different cultures. The episode ends with a Q&A using live audience questions!
In the final episode of Season 1, Emily and Jacqueline take a moment to reflect on all they've learned in writing a book and making a podcast about data science careers.
In this special live episode recorded at the Data Science DC, Emily and Jacqueline talk about data scientists' relationships with their managers. They discuss how you should communicate with your manager, how much you should be doing what your manager asks vs what you think is best for the company, and other topics. Several times in the episode Jacqueline and Emily disagree, adding drama and intrigue.
After you are a senior data scientist for a few years, what is next? In this episode Jacqueline and Emily talk about three possible career moves for seasoned data scientists: becoming a manager, a technical lead, or an independent consultant. They discuss the pros and cons of the different types of jobs and strategies for getting into those roles.
"You can't fire me I quit!" --a great example of not leaving a job gracefully (but hey it happens!). In this week's episode Emily and Jacqueline discuss how to decide when the right moment is for you to leave a job, and what steps you should take. They also talk about the realities of the situation, like managers who don't seem to listen when you discuss why you are unhappy, walking out of job, and getting a last minute counter offer.
In this special live episode recorded at the ODSC East conference, Emily and Jacqueline discuss what to think about it you can't seem to find that data science job you want. They talk about the different reasons why job hunts don't work, what you can do about it, and answer listener questions from the audience.
Social media, conferences, blogs, and meetups--there are many places where data scientists congregate outside of work. But how does a data scientist become a part of these communities and contribute? In this episode Jacqueline and Emily discuss speaking at conferences, awkwardly attending your first meetup, and many other moments that can happen when a data scientist starts to join the community.
You're not a failure! The field of data science is overflowing with projects that just didn't work out. This episode we talk about these projects and why they fail. We also discuss how to handle them emotionally even though it might feel like you're solely responsible. In the game, Emily makes Jacqueline try and discern true stories of data science mistakes from her twisted deceptions.
Stakeholders! The people who data scientists help by creating analyses and models. Stakeholders have so many needs, and keeping them satisfied is one of the most important parts of being a successful data scientist. In this episode Jacqueline and Emily discuss how to make your stakeholders happy with good communication and empathy.
Putting data science into production can mean a ton of things: from customer-facing models run millions of times a day to continuously live dashboards for stakeholders. But writing code for production and getting it to work can be intimidating for many data scientists, and lots of us have never tried. In this episode we talk about production, why it can be scary, and what you can do to get started! We also have a game that just might rip off a much more popular TV show.
An analysis is the work of taking data and turning it into a PowerPoint presentation (more or less), and in this episode we talk all about it. What makes an analysis sharp, how to sell data stories to stakeholders, and the useful tool of an "analysis plan" are all discussed. The episode ends with Emily giving Jacqueline an analysis game that tangentially relates to puppies.
Welcome to your new data science role! You just started a new data science job, maybe your first one, and wow there is a lot to do! In this episode, we cover what to expect in the first few months of the job and what to do if it’s not what you hoped. We also discuss how to handle the inevitable heartbreak as you find technical skeletons in the closet like production databases that are just csv files on a SharePoint.
Technically still just in time for the last of the holidays - a special bonus episode! Here we cover three small mini-topics. First, whether you should get a PhD to become a data scientist (spoiler alert: almost certainly not), delving deep into the culture of academia and our own PhD experiences. Then we share how we decide if we should learn a new topic/technology. Finally, we talk about how to design good data science interviews as a hiring manager. Do we advocate for white boarding, take-homes, and unstructured interviews? Listen to hear our hot takes!
Stop! Don’t just accept that coveted data science job offer right off the bat. Instead, listen to this episode where we discuss negotiating: what’s on the table, what salaries to expect at different levels of data scientist, what the heck RSUs and stock options are, and much more. By reflecting on what’s important to you and how to ask for it, you’ll start your new job in the best way. Includes stories from Jacqueline and Emily's pasts!
The interview: possibly the most nerve-wracking part of a data science job search. In this episode, we’ll help you prepare by covering the full interview process, including the different types of interviews, what questions you might be asked, and what the interviewers are looking for. And since interviewing is a two-way street, we also do a mock interview where Emily as the interviewee tries to see if there are red flags in a few jobs.
“You only get one chance at a first impression.” When applying for data science jobs, that’s usually your resume (and sometimes a cover letter). So how can you give your data science resume the polish it deserves? In this episode, we discuss what hiring managers and recruiters are looking for in a resume, how and why to customize it for different jobs, and weigh in on the always hotly contested: “how many pages should it be?”
Before you can get a data science job, you need to find one! In this episode, we discuss how to decode job descriptions, use your network, and find jobs that don’t need 3+ years of experience. We share how we’ve found our previous jobs and we do some on-the-spot judging of job posts that Emily found on LinkedIn.
Perhaps the most common piece of advice for aspiring data scientists is to make a project portfolio. Despite this, so few data scientists do so! In this episode, we discuss what exactly a portfolio is, the benefits, and the common reasons people don’t do it and how to overcome them. Spoiler: it's just as much psychological as it is about time and skills.
It seems there are so many “required” skills for a data science job—how can someone possibly learn them? In this episode, we discuss four possible ways to do so: a formal degree program, a boot camp, learning on the job, and teaching yourself. We also share our own very different backgrounds: Jacqueline's math master's and engineering PhD versus Emily's statistics minor, master's in organizational behavior, and a boot camp.
Your post is very nice. But eInblick's advanced progressive computation engine delivers predictable results within seconds, even on very large datasets. Whether you're building a simple histogram, a run of AutoML, or a chunk of Python code, you'll never have to wait. We keep adding new samples and refining the answer in the background as you move forward with analysis and estimation. You can visit this site https://www.einblick.ai/ to know more.
i am new here https://castbox.fm/episode/Chapter-16%3A-Moving-up-the-ladder-id3232615-id380053092?country=us
classification of a data scientist