Discover
AI Engineering Podcast
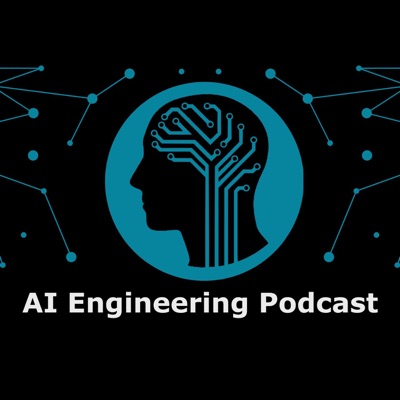
AI Engineering Podcast
Author: Tobias Macey
Subscribed: 8,800Played: 242,760Subscribe
Share
© 2024 Boundless Notions, LLC.
Description
This show is your guidebook to building scalable and maintainable AI systems. You will learn how to architect AI applications, apply AI to your work, and the considerations involved in building or customizing new models. Everything that you need to know to deliver real impact and value with machine learning and artificial intelligence.
36 Episodes
Reverse
SummaryIn this episode of the AI Engineering podcast, host Tobias Macy interviews Tammer Saleh, founder of SuperOrbital, about the potentials and pitfalls of using Kubernetes for machine learning workloads. The conversation delves into the specific needs of machine learning workflows, such as model tracking, versioning, and the use of Jupyter Notebooks, and how Kubernetes can support these tasks. Tammer emphasizes the importance of a unified API for different teams and the flexibility Kubernetes provides in handling various workloads. Finally, Tammer offers advice for teams considering Kubernetes for their machine learning workloads and discusses the future of Kubernetes in the ML ecosystem, including areas for improvement and innovation.AnnouncementsHello and welcome to the AI Engineering Podcast, your guide to the fast-moving world of building scalable and maintainable AI systemsYour host is Tobias Macey and today I'm interviewing Tammer Saleh about the potentials and pitfalls of using Kubernetes for your ML workloads.InterviewIntroductionHow did you get involved in Kubernetes?For someone who is unfamiliar with Kubernetes, how would you summarize it?For the context of this conversation, can you describe the different phases of ML that we're talking about?Kubernetes was originally designed to handle scaling and distribution of stateless processes. ML is an inherently stateful problem domain. What challenges does that add for K8s environments?What are the elements of an ML workflow that lend themselves well to a Kubernetes environment?How much Kubernetes knowledge does an ML/data engineer need to know to get their work done?What are the sharp edges of Kubernetes in the context of ML projects?What are the most interesting, unexpected, or challenging lessons that you have learned while working with Kubernetes?When is Kubernetes the wrong choice for ML?What are the aspects of Kubernetes (core or the ecosystem) that you are keeping an eye on which will help improve its utility for ML workloads?Contact InfoEmailLinkedInParting QuestionFrom your perspective, what is the biggest gap in the tooling or technology for ML workloads today?LinksSuperOrbitalCloudFoundryHeroku12 Factor ModelKubernetesDocker ComposeCore K8s ClassJupyter NotebookCrossplaneOchre JellyCNCF (Cloud Native Computing Foundation) LandscapeStateful SetRAG == Retrieval Augmented GenerationPodcast EpisodeKubeflowFlyteData Engineering Podcast EpisodePachydermData Engineering Podcast EpisodeCoreWeaveKubectl ("koob-cuddle")HelmCRD == Custom Resource DefinitionHorovodPodcast.__init__ EpisodeTemporalSlurmRayDaskInfinibandThe intro and outro music is from The Hug by The Freak Fandango Orchestra / CC BY-SA
SummaryIn this episode we're joined by Matt Zeiler, founder and CEO of Clarifai, as he dives into the technical aspects of retrieval augmented generation (RAG). From his journey into AI at the University of Toronto to founding one of the first deep learning AI companies, Matt shares his insights on the evolution of neural networks and generative models over the last 15 years. He explains how RAG addresses issues with large language models, including data staleness and hallucinations, by providing dynamic access to information through vector databases and embedding models. Throughout the conversation, Matt and host Tobias Macy discuss everything from architectural requirements to operational considerations, as well as the practical applications of RAG in industries like intelligence, healthcare, and finance. Tune in for a comprehensive look at RAG and its future trends in AI.AnnouncementsHello and welcome to the AI Engineering Podcast, your guide to the fast-moving world of building scalable and maintainable AI systemsYour host is Tobias Macey and today I'm interviewing Matt Zeiler, Founder & CEO of Clarifai, about the technical aspects of RAG, including the architectural requirements, edge cases, and evolutionary characteristicsInterviewIntroductionHow did you get involved in the area of data management?Can you describe what RAG (Retrieval Augmented Generation) is?What are the contexts in which you would want to use RAG?What are the alternatives to RAG?What are the architectural/technical components that are required for production grade RAG?Getting a quick proof-of-concept working for RAG is fairly straightforward. What are the failures modes/edge cases that start to surface as you scale the usage and complexity?The first step of building the corpus for RAG is to generate the embeddings. Can you talk through the planning and design process? (e.g. model selection for embeddings, storage capacity/latency, etc.)How does the modality of the input/output affect this and downstream decisions? (e.g. text vs. image vs. audio, etc.)What are the features of a vector store that are most critical for RAG?The set of available generative models is expanding and changing at breakneck speed. What are the foundational aspects that you look for in selecting which model(s) to use for the output?Vector databases have been gaining ground for search functionality, even without generative AI. What are some of the other ways that elements of RAG can be re-purposed?What are the most interesting, innovative, or unexpected ways that you have seen RAG used?What are the most interesting, unexpected, or challenging lessons that you have learned while working on RAG?When is RAG the wrong choice?What are the main trends that you are following for RAG and its component elements going forward?Contact InfoWebsiteLinkedInParting QuestionFrom your perspective, what is the biggest barrier to adoption of machine learning today?Closing AnnouncementsThank you for listening! Don't forget to check out our other shows. The Data Engineering Podcast covers the latest on modern data management. [Podcast.__init__]() covers the Python language, its community, and the innovative ways it is being used.Visit the site to subscribe to the show, sign up for the mailing list, and read the show notes.If you've learned something or tried out a project from the show then tell us about it! Email hosts@aiengineeringpodcast.com with your story.To help other people find the show please leave a review on iTunes and tell your friends and co-workers.LinksClarifaiGeoff HintonYann LecunNeural NetworksDeep LearningRetrieval Augmented GenerationContext WindowVector DatabasePrompt EngineeringMistralLlama 3Embedding QuantizationActive LearningGoogle GeminiAI Model AttentionRecurrent NetworkConvolutional NetworkReranking ModelStop WordsMassive Text Embedding Benchmark (MTEB)Retool State of AI ReportpgvectorMilvusQdrantPineconeOpenLLM LeaderboardSemantic SearchHashicorpThe intro and outro music is from Hitman's Lovesong feat. Paola Graziano by The Freak Fandango Orchestra/CC BY-SA 3.0
SummaryArtificial intelligence has dominated the headlines for several months due to the successes of large language models. This has prompted numerous debates about the possibility of, and timeline for, artificial general intelligence (AGI). Peter Voss has dedicated decades of his life to the pursuit of truly intelligent software through the approach of cognitive AI. In this episode he explains his approach to building AI in a more human-like fashion and the emphasis on learning rather than statistical prediction.AnnouncementsHello and welcome to the AI Engineering Podcast, your guide to the fast-moving world of building scalable and maintainable AI systemsYour host is Tobias Macey and today I'm interviewing Peter Voss about what is involved in making your AI applications more "human"InterviewIntroductionHow did you get involved in machine learning?Can you start by unpacking the idea of "human-like" AI?How does that contrast with the conception of "AGI"?The applications and limitations of GPT/LLM models have been dominating the popular conversation around AI. How do you see that impacting the overrall ecosystem of ML/AI applications and investment?The fundamental/foundational challenge of every AI use case is sourcing appropriate data. What are the strategies that you have found useful to acquire, evaluate, and prepare data at an appropriate scale to build high quality models? What are the opportunities and limitations of causal modeling techniques for generalized AI models?As AI systems gain more sophistication there is a challenge with establishing and maintaining trust. What are the risks involved in deploying more human-level AI systems and monitoring their reliability?What are the practical/architectural methods necessary to build more cognitive AI systems?How would you characterize the ecosystem of tools/frameworks available for creating, evolving, and maintaining these applications?What are the most interesting, innovative, or unexpected ways that you have seen cognitive AI applied?What are the most interesting, unexpected, or challenging lessons that you have learned while working on desiging/developing cognitive AI systems?When is cognitive AI the wrong choice?What do you have planned for the future of cognitive AI applications at Aigo?Contact InfoLinkedInWebsiteParting QuestionFrom your perspective, what is the biggest barrier to adoption of machine learning today?Closing AnnouncementsThank you for listening! Don't forget to check out our other shows. The Data Engineering Podcast covers the latest on modern data management. Podcast.__init__ covers the Python language, its community, and the innovative ways it is being used.Visit the site to subscribe to the show, sign up for the mailing list, and read the show notes.If you've learned something or tried out a project from the show then tell us about it! Email hosts@aiengineeringpodcast.com with your story.To help other people find the show please leave a review on iTunes and tell your friends and co-workers.LinksAigo.aiArtificial General IntelligenceCognitive AIKnowledge GraphCausal ModelingBayesian StatisticsThinking Fast & Slow by Daniel Kahneman (affiliate link)Agent-Based ModelingReinforcement LearningDARPA 3 Waves of AI presentationWhy Don't We Have AGI Yet? whitepaperConcepts Is All You Need WhitepaperHellen KellerStephen HawkingThe intro and outro music is from Hitman's Lovesong feat. Paola Graziano by The Freak Fandango Orchestra/CC BY-SA 3.0
SummaryGenerative AI promises to accelerate the productivity of human collaborators. Currently the primary way of working with these tools is through a conversational prompt, which is often cumbersome and unwieldy. In order to simplify the integration of AI capabilities into developer workflows Tsavo Knott helped create Pieces, a powerful collection of tools that complements the tools that developers already use. In this episode he explains the data collection and preparation process, the collection of model types and sizes that work together to power the experience, and how to incorporate it into your workflow to act as a second brain.AnnouncementsHello and welcome to the AI Engineering Podcast, your guide to the fast-moving world of building scalable and maintainable AI systemsYour host is Tobias Macey and today I'm interviewing Tsavo Knott about Pieces, a personal AI toolkit to improve the efficiency of developersInterviewIntroductionHow did you get involved in machine learning?Can you describe what Pieces is and the story behind it?The past few months have seen an endless series of personalized AI tools launched. What are the features and focus of Pieces that might encourage someone to use it over the alternatives?model selectionsarchitecture of Pieces applicationlocal vs. hybrid vs. online modelsmodel update/delivery processdata preparation/serving for models in context of Pieces appapplication of AI to developer workflowstypes of workflows that people are building with piecesWhat are the most interesting, innovative, or unexpected ways that you have seen Pieces used?What are the most interesting, unexpected, or challenging lessons that you have learned while working on Pieces?When is Pieces the wrong choice?What do you have planned for the future of Pieces?Contact InfoLinkedInParting QuestionFrom your perspective, what is the biggest barrier to adoption of machine learning today?Closing AnnouncementsThank you for listening! Don't forget to check out our other shows. The Data Engineering Podcast covers the latest on modern data management. Podcast.__init__ covers the Python language, its community, and the innovative ways it is being used.Visit the site to subscribe to the show, sign up for the mailing list, and read the show notes.If you've learned something or tried out a project from the show then tell us about it! Email hosts@aiengineeringpodcast.com with your story.To help other people find the show please leave a review on iTunes and tell your friends and co-workers.LinksPiecesNPU == Neural Processing UnitTensor ChipLoRA == Low Rank AdaptationGenerative Adversarial NetworksMistralEmacsVimNeoVimDartFlutterTypescriptLuaRetrieval Augmented GenerationONNXLSTM == Long Short-Term MemoryLLama 2GitHub CopilotTabninePodcast EpisodeThe intro and outro music is from Hitman's Lovesong feat. Paola Graziano by The Freak Fandango Orchestra/CC BY-SA 3.0
SummaryLarge Language Models (LLMs) have rapidly captured the attention of the world with their impressive capabilities. Unfortunately, they are often unpredictable and unreliable. This makes building a product based on their capabilities a unique challenge. Jignesh Patel is building DataChat to bring the capabilities of LLMs to organizational analytics, allowing anyone to have conversations with their business data. In this episode he shares the methods that he is using to build a product on top of this constantly shifting set of technologies.AnnouncementsHello and welcome to the Machine Learning Podcast, the podcast about machine learning and how to bring it from idea to delivery.Your host is Tobias Macey and today I'm interviewing Jignesh Patel about working with LLMs; understanding how they work and how to build your ownInterviewIntroductionHow did you get involved in machine learning?Can you start by sharing some of the ways that you are working with LLMs currently?What are the business challenges involved in building a product on top of an LLM model that you don't own or control? In the current age of business, your data is often your strategic advantage. How do you avoid losing control of, or leaking that data while interfacing with a hosted LLM API?What are the technical difficulties related to using an LLM as a core element of a product when they are largely a black box? What are some strategies for gaining visibility into the inner workings or decision making rules for these models?What are the factors, whether technical or organizational, that might motivate you to build your own LLM for a business or product? Can you unpack what it means to "build your own" when it comes to an LLM?In your work at DataChat, how has the progression of sophistication in LLM technology impacted your own product strategy?What are the most interesting, innovative, or unexpected ways that you have seen LLMs/DataChat used?What are the most interesting, unexpected, or challenging lessons that you have learned while working with LLMs?When is an LLM the wrong choice?What do you have planned for the future of DataChat?Contact InfoWebsiteLinkedInParting QuestionFrom your perspective, what is the biggest barrier to adoption of machine learning today?Closing AnnouncementsThank you for listening! Don't forget to check out our other shows. The Data Engineering Podcast covers the latest on modern data management. Podcast.__init__ covers the Python language, its community, and the innovative ways it is being used.Visit the site to subscribe to the show, sign up for the mailing list, and read the show notes.If you've learned something or tried out a project from the show then tell us about it! Email hosts@themachinelearningpodcast.com) with your story.To help other people find the show please leave a review on iTunes and tell your friends and co-workers.LinksDataChatCMU == Carnegie Mellon UniversitySVM == Support Vector MachineGenerative AIGenomicsProteomicsParquetOpenAI CodexLLamaMistralGoogle VertexLangchainRetrieval Augmented GenerationPrompt EngineeringEnsemble LearningXGBoostCatboostLinear RegressionCOGS == Cost Of Goods SoldBruce Schneier - AI And TrustThe intro and outro music is from Hitman's Lovesong feat. Paola Graziano by The Freak Fandango Orchestra/CC BY-SA 3.0
SummaryMachine learning is a powerful set of technologies, holding the potential to dramatically transform businesses across industries. Unfortunately, the implementation of ML projects often fail to achieve their intended goals. This failure is due to a lack of collaboration and investment across technological and organizational boundaries. To help improve the success rate of machine learning projects Eric Siegel developed the six step bizML framework, outlining the process to ensure that everyone understands the whole process of ML deployment. In this episode he shares the principles and promise of that framework and his motivation for encapsulating it in his book "The AI Playbook".AnnouncementsHello and welcome to the Machine Learning Podcast, the podcast about machine learning and how to bring it from idea to delivery.Your host is Tobias Macey and today I'm interviewing Eric Siegel about how the bizML approach can help improve the success rate of your ML projectsInterviewIntroductionHow did you get involved in machine learning?Can you describe what bizML is and the story behind it? What are the key aspects of this approach that are different from the "industry standard" lifecycle of an ML project?What are the elements of your personal experience as an ML consultant that helped you develop the tenets of bizML?Who are the personas that need to be involved in an ML project to increase the likelihood of success? Who do you find to be best suited to "own" or "lead" the process?What are the organizational patterns that might hinder the work of delivering on the goals of an ML initiative?What are some of the misconceptions about the work involved in/capabilities of an ML model that you commonly encounter?What is your main goal in writing your book "The AI Playbook"?What are the most interesting, innovative, or unexpected ways that you have seen the bizML process in action?What are the most interesting, unexpected, or challenging lessons that you have learned while working on ML projects and developing the bizML framework?When is bizML the wrong choice?What are the future developments in organizational and technical approaches to ML that will improve the success rate of AI projects?Contact InfoLinkedInParting QuestionFrom your perspective, what is the biggest barrier to adoption of machine learning today?Closing AnnouncementsThank you for listening! Don't forget to check out our other shows. The Data Engineering Podcast covers the latest on modern data management. Podcast.__init__ covers the Python language, its community, and the innovative ways it is being used.Visit the site to subscribe to the show, sign up for the mailing list, and read the show notes.If you've learned something or tried out a project from the show then tell us about it! Email hosts@themachinelearningpodcast.com) with your story.To help other people find the show please leave a review on iTunes and tell your friends and co-workers.LinksThe AI Playbook: Mastering the Rare Art of Machine Learning Deployment by Eric SiegelPredictive Analytics: The Power to Predict Who Will Click, Buy, Lie, or Die by Eric SiegelColumbia UniversityMachine Learning Week ConferenceGenerative AI WorldMachine Learning Leadership and Practice CourseRexer AnalyticsKD NuggetsCRISP-DMRandom ForestGradient DescentThe intro and outro music is from Hitman's Lovesong feat. Paola Graziano by The Freak Fandango Orchestra/CC BY-SA 3.0
SummaryOne of the most time consuming aspects of building a machine learning model is feature engineering. Generative AI offers the possibility of accelerating the discovery and creation of feature pipelines. In this episode Colin Priest explains how FeatureByte is applying generative AI models to the challenge of building and maintaining machine learning pipelines.AnnouncementsHello and welcome to the Machine Learning Podcast, the podcast about machine learning and how to bring it from idea to delivery.Your host is Tobias Macey and today I'm interviewing Colin Priest about applying generative AI to the task of building and deploying AI pipelinesInterviewIntroductionHow did you get involved in machine learning?Can you start by giving the 30,000 foot view of the steps involved in an AI pipeline? Understand the problemFeature ideationFeature engineeringExperimentOptimizeProductionizeWhat are the stages of that process that are prone to repetition? What are the ways that teams typically try to automate those steps?What are the features of generative AI models that can be brought to bear on the design stage of an AI pipeline? What are the validation/verification processes that engineers need to apply to the generated suggestions?What are the opportunities/limitations for unit/integration style tests?What are the elements of developer experience that need to be addressed to make the gen AI capabilities an enhancement instead of a distraction? What are the interfaces through which the AI functionality can/should be exposed?What are the aspects of pipeline and model deployment that can benefit from generative AI functionality? What are the potential risk factors that need to be considered when evaluating the application of this functionality?What are the most interesting, innovative, or unexpected ways that you have seen generative AI used in the development and maintenance of AI pipelines?What are the most interesting, unexpected, or challenging lessons that you have learned while working on the application of generative AI to the ML workflow?When is generative AI the wrong choice?What do you have planned for the future of FeatureByte's AI copilot capabiliteis?Contact InfoLinkedInParting QuestionFrom your perspective, what is the biggest barrier to adoption of machine learning today?Closing AnnouncementsThank you for listening! Don't forget to check out our other shows. The Data Engineering Podcast covers the latest on modern data management. Podcast.__init__ covers the Python language, its community, and the innovative ways it is being used.Visit the site to subscribe to the show, sign up for the mailing list, and read the show notes.If you've learned something or tried out a project from the show then tell us about it! Email hosts@themachinelearningpodcast.com) with your story.To help other people find the show please leave a review on iTunes and tell your friends and co-workers.LinksFeatureByteGenerative AIThe Art of WarOCR == Optical Character RecognitionGenetic AlgorithmSemantic LayerPrompt EngineeringThe intro and outro music is from Hitman's Lovesong feat. Paola Graziano by The Freak Fandango Orchestra/CC BY-SA 3.0Support The Machine Learning Podcast
SummaryEvery business develops their own specific workflows to address their internal organizational needs. Not all of them are properly documented, or even visible. Workflow automation tools have tried to reduce the manual burden involved, but they are rigid and require substantial investment of time to discover and develop the routines. Boaz Hecht co-founded 8Flow to iteratively discover and automate pieces of workflows, bringing visibility and collaboration to the internal organizational processes that keep the business running.AnnouncementsHello and welcome to the Machine Learning Podcast, the podcast about machine learning and how to bring it from idea to delivery.Your host is Tobias Macey and today I'm interviewing Boaz Hecht about using AI to automate customer support at 8FlowInterviewIntroductionHow did you get involved in machine learning?Can you describe what 8Flow is and the story behind it?How does 8Flow compare to RPA tools that companies are using today? What are the opportunities for augmenting or integrating with RPA frameworks?What are the key selling points for the solution that you are building? (does AI sell? Or is it about the realized savings?)What are the sources of signal that you are relying on to build model features?Given the heterogeneity in tools and processes across customers, what are the common focal points that let you address the widest possible range of functionality?Can you describe how 8Flow is implemented? How have the design and goals evolved since you first started working on it?What are the model categories that are most relevant for process automation in your product?How have you approached the design and implementation of your MLOps workflow? (model training, deployment, monitoring, versioning, etc.)What are the open questions around product focus and system design that you are still grappling with?Given the relative recency of ML/AI as a profession and the massive growth in attention and activity, how are you addressing the challenge of obtaining and maximizing human talent?What are the most interesting, innovative, or unexpected ways that you have seen 8Flow used?What are the most interesting, unexpected, or challenging lessons that you have learned while working on 8Flow?When is 8Flow the wrong choice?What do you have planned for the future of 8Flow?Contact InfoLinkedInPersonal WebsiteParting QuestionFrom your perspective, what is the biggest barrier to adoption of machine learning today?Closing AnnouncementsThank you for listening! Don't forget to check out our other shows. The Data Engineering Podcast covers the latest on modern data management. Podcast.__init__ covers the Python language, its community, and the innovative ways it is being used.Visit the site to subscribe to the show, sign up for the mailing list, and read the show notes.If you've learned something or tried out a project from the show then tell us about it! Email hosts@themachinelearningpodcast.com) with your story.To help other people find the show please leave a review on iTunes and tell your friends and co-workers.Links8FlowRobotic Process AutomationThe intro and outro music is from Hitman's Lovesong feat. Paola Graziano by The Freak Fandango Orchestra/CC BY-SA 3.0Support The Machine Learning Podcast
SummaryMachine learning and AI applications hold the promise of drastically impacting every aspect of modern life. With that potential for profound change comes a responsibility for the creators of the technology to account for the ramifications of their work. In this episode Nicholas Cifuentes-Goodbody guides us through the minefields of social, technical, and ethical considerations that are necessary to ensure that this next generation of technical and economic systems are equitable and beneficial for the people that they impact.AnnouncementsHello and welcome to the Machine Learning Podcast, the podcast about machine learning and how to bring it from idea to delivery.Your host is Tobias Macey and today I'm interviewing Nicholas Cifuentes-Goodbody about the different elements of the machine learning workflow where ethics need to be consideredInterviewIntroductionHow did you get involved in machine learning?To start with, who is responsible for addressing the ethical concerns around AI?What are the different ways that AI can have positive or negative outcomes from an ethical perspective? What is the role of practitioners/individual contributors in the identification and evaluation of ethical impacts of their work?What are some utilities that are helpful in identifying and addressing bias in training data?How can practitioners address challenges of equity and accessibility in the delivery of AI products?What are some of the options for reducing the energy consumption for training and serving AI?What are the most interesting, innovative, or unexpected ways that you have seen ML teams incorporate ethics into their work?What are the most interesting, unexpected, or challenging lessons that you have learned while working on ethical implications of ML?What are some of the resources that you recommend for people who want to invest in their knowledge and application of ethics in the realm of ML?Contact InfoWorldQuant University's Applied Data Science LabLinkedInParting QuestionFrom your perspective, what is the biggest barrier to adoption of machine learning today?Closing AnnouncementsThank you for listening! Don't forget to check out our other shows. The Data Engineering Podcast covers the latest on modern data management. Podcast.__init__ covers the Python language, its community, and the innovative ways it is being used.Visit the site to subscribe to the show, sign up for the mailing list, and read the show notes.If you've learned something or tried out a project from the show then tell us about it! Email hosts@themachinelearningpodcast.com) with your story.To help other people find the show please leave a review on iTunes and tell your friends and co-workers.LinksUNESCO Recommendation on the Ethics of Artificial IntelligenceEuropean Union AI ActHow machine learning helps advance access to human rights informationDisinformation, Team JorgeChina, AI, and Human RightsHow China Is Using A.I. to Profile a MinorityWeapons of Math DestructionFairlearnAI Fairness 360Allen Institute for AI NYTAllen Institute for AITransformersAI4ALLWorldQuant UniversityHow to Make Generative AI GreenerMachine Learning Emissions CalculatorPracticing Trustworthy Machine LearningEnergy and Policy Considerations for Deep LearningNatural Language ProcessingTrolley ProblemProtected Classesfairlearn (scikit-learn)BERT ModelThe intro and outro music is from Hitman's Lovesong feat. Paola Graziano by The Freak Fandango Orchestra/CC BY-SA 3.0
SummaryBuilding machine learning systems and other intelligent applications are a complex undertaking. This often requires retrieving data from a warehouse engine, adding an extra barrier to every workflow. The RelationalAI engine was built as a co-processor for your data warehouse that adds a greater degree of flexibility in the representation and analysis of the underlying information, simplifying the work involved. In this episode CEO Molham Aref explains how RelationalAI is designed, the capabilities that it adds to your data clouds, and how you can start using it to build more sophisticated applications on your data.AnnouncementsHello and welcome to the Machine Learning Podcast, the podcast about machine learning and how to bring it from idea to delivery.Your host is Tobias Macey and today I'm interviewing Molham Aref about RelationalAI and the principles behind it for powering intelligent applicationsInterviewIntroductionHow did you get involved in machine learning?Can you describe what RelationalAI is and the story behind it? On your site you call your product an "AI Co-processor". Can you explain what you mean by that phrase?What are the primary use cases that you address with the RelationalAI product? What are the types of solutions that teams might build to address those problems in the absence of something like the RelationalAI engine?Can you describe the system design of RelationalAI? How have the design and goals of the platform changed since you first started working on it?For someone who is using RelationalAI to address a business need, what does the onboarding and implementation workflow look like?What is your design philosophy for identifying the balance between automating the implementation of certain categories of application (e.g. NER) vs. providing building blocks and letting teams assemble them on their own?What are the data modeling paradigms that teams should be aware of to make the best use of the RKGS platform and Rel language?What are the aspects of customer education that you find yourself spending the most time on?What are some of the most under-utilized or misunderstood capabilities of the RelationalAI platform that you think deserve more attention?What are the most interesting, innovative, or unexpected ways that you have seen the RelationalAI product used?What are the most interesting, unexpected, or challenging lessons that you have learned while working on RelationalAI?When is RelationalAI the wrong choice?What do you have planned for the future of RelationalAI?Contact InfoLinkedInParting QuestionFrom your perspective, what is the biggest barrier to adoption of machine learning today?Closing AnnouncementsThank you for listening! Don't forget to check out our other shows. The Data Engineering Podcast covers the latest on modern data management. Podcast.__init__ covers the Python language, its community, and the innovative ways it is being used.Visit the site to subscribe to the show, sign up for the mailing list, and read the show notes.If you've learned something or tried out a project from the show then tell us about it! Email hosts@themachinelearningpodcast.com) with your story.To help other people find the show please leave a review on iTunes and tell your friends and co-workers.LinksRelationalAISnowflakeAI WinterBigQueryGradient DescentB-TreeNavigational DatabaseHadoopTeradataWorst Case Optimal JoinSemantic Query OptimizationRelational AlgebraHyperGraphLinear AlgebraVector DatabasePathwayData Engineering Podcast EpisodePineconeData Engineering Podcast EpisodeThe intro and outro music is from Hitman's Lovesong feat. Paola Graziano by The Freak Fandango Orchestra/CC BY-SA 3.0
SummaryMachine learning and generative AI systems have produced truly impressive capabilities. Unfortunately, many of these applications are not designed with the privacy of end-users in mind. TripleBlind is a platform focused on embedding privacy preserving techniques in the machine learning process to produce more user-friendly AI products. In this episode Gharib Gharibi explains how the current generation of applications can be susceptible to leaking user data and how to counteract those trends.AnnouncementsHello and welcome to the Machine Learning Podcast, the podcast about machine learning and how to bring it from idea to delivery.Your host is Tobias Macey and today I'm interviewing Gharib Gharibi about the challenges of bias and data privacy in generative AI modelsInterviewIntroductionHow did you get involved in machine learning?Generative AI has been gaining a lot of attention and speculation about its impact. What are some of the risks that these capabilities pose? What are the main contributing factors to their existing shortcomings?What are some of the subtle ways that bias in the source data can manifest?In addition to inaccurate results, there is also a question of how user interactions might be re-purposed and potential impacts on data and personal privacy. What are the main sources of risk?With the massive attention that generative AI has created and the perspectives that are being shaped by it, how do you see that impacting the general perception of other implementations of AI/ML? How can ML practitioners improve and convey the trustworthiness of their models to end users?What are the risks for the industry if generative models fall out of favor with the public?How does your work at Tripleblind help to encourage a conscientious approach to AI?What are the most interesting, innovative, or unexpected ways that you have seen data privacy addressed in AI applications?What are the most interesting, unexpected, or challenging lessons that you have learned while working on privacy in AI?When is TripleBlind the wrong choice?What do you have planned for the future of TripleBlind?Contact InfoLinkedInParting QuestionFrom your perspective, what is the biggest barrier to adoption of machine learning today?Closing AnnouncementsThank you for listening! Don't forget to check out our other shows. The Data Engineering Podcast covers the latest on modern data management. Podcast.__init__ covers the Python language, its community, and the innovative ways it is being used.Visit the site to subscribe to the show, sign up for the mailing list, and read the show notes.If you've learned something or tried out a project from the show then tell us about it! Email hosts@themachinelearningpodcast.com) with your story.To help other people find the show please leave a review on iTunes and tell your friends and co-workers.LinksTripleBlindImageNet Geoffrey Hinton PaperBERT language modelGenerative AIGPT == Generative Pre-trained TransformerHIPAA Safe Harbor RulesFederated LearningDifferential PrivacyHomomorphic EncryptionThe intro and outro music is from Hitman's Lovesong feat. Paola Graziano by The Freak Fandango Orchestra/CC BY-SA 3.0
SummarySoftware development involves an interesting balance of creativity and repetition of patterns. Generative AI has accelerated the ability of developer tools to provide useful suggestions that speed up the work of engineers. Tabnine is one of the main platforms offering an AI powered assistant for software engineers. In this episode Eran Yahav shares the journey that he has taken in building this product and the ways that it enhances the ability of humans to get their work done, and when the humans have to adapt to the tool.AnnouncementsHello and welcome to the Machine Learning Podcast, the podcast about machine learning and how to bring it from idea to delivery.Your host is Tobias Macey and today I'm interviewing Eran Yahav about building an AI powered developer assistant at TabnineInterviewIntroductionHow did you get involved in machine learning?Can you describe what Tabnine is and the story behind it?What are the individual and organizational motivations for using AI to generate code? What are the real-world limitations of generative AI for creating software? (e.g. size/complexity of the outputs, naming conventions, etc.)What are the elements of skepticism/oversight that developers need to exercise while using a system like Tabnine?What are some of the primary ways that developers interact with Tabnine during their development workflow? Are there any particular styles of software for which an AI is more appropriate/capable? (e.g. webapps vs. data pipelines vs. exploratory analysis, etc.)For natural languages there is a strong bias toward English in the current generation of LLMs. How does that translate into computer languages? (e.g. Python, Java, C++, etc.)Can you describe the structure and implementation of Tabnine? Do you rely primarily on a single core model, or do you have multiple models with subspecialization?How have the design and goals of the product changed since you first started working on it?What are the biggest challenges in building a custom LLM for code? What are the opportunities for specialization of the model architecture given the highly structured nature of the problem domain?For users of Tabnine, how do you assess/monitor the accuracy of recommendations? What are the feedback and reinforcement mechanisms for the model(s)?What are the most interesting, innovative, or unexpected ways that you have seen Tabnine's LLM powered coding assistant used?What are the most interesting, unexpected, or challenging lessons that you have learned while working on AI assisted development at Tabnine?When is an AI developer assistant the wrong choice?What do you have planned for the future of Tabnine?Contact InfoLinkedInWebsiteParting QuestionFrom your perspective, what is the biggest barrier to adoption of machine learning today?Closing AnnouncementsThank you for listening! Don't forget to check out our other shows. The Data Engineering Podcast covers the latest on modern data management. Podcast.__init__ covers the Python language, its community, and the innovative ways it is being used.Visit the site to subscribe to the show, sign up for the mailing list, and read the show notes.If you've learned something or tried out a project from the show then tell us about it! Email hosts@themachinelearningpodcast.com) with your story.To help other people find the show please leave a review on iTunes and tell your friends and co-workers.LinksTabNineTechnion UniversityProgram SynthesisContext StuffingElixirDependency InjectionCOBOLVerilogMidJourneyThe intro and outro music is from Hitman's Lovesong feat. Paola Graziano by The Freak Fandango Orchestra/CC BY-SA 3.0
SummarySoftware systems power much of the modern world. For applications that impact the safety and well-being of people there is an extra set of precautions that need to be addressed before deploying to production. If machine learning and AI are part of that application then there is a greater need to validate the proper functionality of the models. In this episode Erez Kaminski shares the work that he is doing at Ketryx to make that validation easier to implement and incorporate into the ongoing maintenance of software and machine learning products.AnnouncementsHello and welcome to the Machine Learning Podcast, the podcast about machine learning and how to bring it from idea to delivery.Your host is Tobias Macey and today I'm interviewing Erez Kaminski about using machine learning in safety critical and highly regulated medical applicationsInterviewIntroductionHow did you get involved in machine learning?Can you start by describing some of the regulatory burdens placed on ML teams who are building solutions for medical applications? How do these requirements impact the development and validation processes of model design and development?What are some examples of the procedural and record-keeping aspects of the machine learning workflow that are required for FDA compliance? What are the opportunities for automating pieces of that overhead?Can you describe what you are doing at Ketryx to streamline the development/training/deployment of ML/AI applications for medical use cases? What are the ideas/assumptions that you had at the start of Ketryx that have been challenged/updated as you work with customers?What are the most interesting, innovative, or unexpected ways that you have seen ML used in medical applications?What are the most interesting, unexpected, or challenging lessons that you have learned while working on Ketryx?When is Ketryx the wrong choice?What do you have planned for the future of Ketryx?Contact InfoEmailLinkedInParting QuestionFrom your perspective, what is the biggest barrier to adoption of machine learning today?Closing AnnouncementsThank you for listening! Don't forget to check out our other shows. The Data Engineering Podcast covers the latest on modern data management. Podcast.__init__ covers the Python language, its community, and the innovative ways it is being used.Visit the site to subscribe to the show, sign up for the mailing list, and read the show notes.If you've learned something or tried out a project from the show then tell us about it! Email hosts@themachinelearningpodcast.com) with your story.To help other people find the show please leave a review on iTunes and tell your friends and co-workers.LinksKetryxWolfram AlphaMathematicaTensorflowSBOM == Software Bill Of MaterialsAir-gapped SystemsAlexNetShapley ValuesSHAPPodcast.__init__ EpisodeBayesian StatisticsCausal ModelingProphetFDA Principles Of Software ValidationThe intro and outro music is from Hitman's Lovesong feat. Paola Graziano by The Freak Fandango Orchestra/CC BY-SA 3.0
SummaryLarge language models have gained a substantial amount of attention in the area of AI and machine learning. While they are impressive, there are many applications where they are not the best option. In this episode Piero Molino explains how declarative ML approaches allow you to make the best use of the available tools across use cases and data formats.AnnouncementsHello and welcome to the Machine Learning Podcast, the podcast about machine learning and how to bring it from idea to delivery.Your host is Tobias Macey and today I'm interviewing Piero Molino about the application of declarative ML in a world being dominated by large language modelsInterviewIntroductionHow did you get involved in machine learning?Can you start by summarizing your perspective on the effect that LLMs are having on the AI/ML industry? In a world where LLMs are being applied to a growing variety of use cases, what are the capabilities that they still lack?How does declarative ML help to address those shortcomings?The majority of current hype is about commercial models (e.g. GPT-4). Can you summarize the current state of the ecosystem for open source LLMs? For teams who are investing in ML/AI capabilities, what are the sources of platform risk for LLMs?What are the comparative benefits of using a declarative ML approach?What are the most interesting, innovative, or unexpected ways that you have seen LLMs used?What are the most interesting, unexpected, or challenging lessons that you have learned while working on declarative ML in the age of LLMs?When is an LLM the wrong choice?What do you have planned for the future of declarative ML and Predibase?Contact InfoLinkedInWebsiteClosing AnnouncementsThank you for listening! Don't forget to check out our other shows. The Data Engineering Podcast covers the latest on modern data management. Podcast.__init__ covers the Python language, its community, and the innovative ways it is being used.Visit the site to subscribe to the show, sign up for the mailing list, and read the show notes.If you've learned something or tried out a project from the show then tell us about it! Email hosts@themachinelearningpodcast.com) with your story.To help other people find the show please leave a review on iTunes and tell your friends and co-workersParting QuestionFrom your perspective, what is the biggest barrier to adoption of machine learning today?LinksPredibasePodcast EpisodeLudwigPodcast.__init__ EpisodeRecommender SystemsInformation RetrievalVector DatabaseTransformer ModelBERTContext WindowsLLAMAThe intro and outro music is from Hitman's Lovesong feat. Paola Graziano by The Freak Fandango Orchestra/CC BY-SA 3.0
SummaryArtificial Intelligence is experiencing a renaissance in the wake of breakthrough natural language models. With new businesses sprouting up to address the various needs of ML and AI teams across the industry, it is a constant challenge to stay informed. Matt Turck has been compiling a report on the state of ML, AI, and Data for his work at FirstMark Capital. In this episode he shares his findings on the ML and AI landscape and the interesting trends that are developing.AnnouncementsHello and welcome to the Machine Learning Podcast, the podcast about machine learning and how to bring it from idea to delivery.As more people start using AI for projects, two things are clear: It’s a rapidly advancing field, but it’s tough to navigate. How can you get the best results for your use case? Instead of being subjected to a bunch of buzzword bingo, hear directly from pioneers in the developer and data science space on how they use graph tech to build AI-powered apps. . Attend the dev and ML talks at NODES 2023, a free online conference on October 26 featuring some of the brightest minds in tech. Check out the agenda and register today at Neo4j.com/NODES.Your host is Tobias Macey and today I'm interviewing Matt Turck about his work on the MAD (ML, AI, and Data) landscape and the insights he has gained on the ML ecosystemInterviewIntroductionHow did you get involved in machine learning?Can you describe what the MAD landscape project is and the story behind it?What are the major changes in the ML ecosystem that you have seen since you first started compiling the landscape? How have the developments in consumer-grade AI in recent years changed the business opportunities for ML/AI?What are the coarse divisions that you see as the boundaries that define the different categories for ML/AI in the landscape?For ML infrastructure products/companies, what are the biggest challenges that they face in engineering and customer acquisition?What are some of the challenges in building momentum for startups in AI (existing moats around data access, talent acquisition, etc.)? For products/companies that have ML/AI as their core offering, what are some strategies that they use to compete with "big tech" companies that already have a large corpus of data?What do you see as the societal vs. business importance of open source models as AI becomes more integrated into consumer facing products?What are the most interesting, innovative, or unexpected ways that you have seen ML/AI used in business and social contexts?What are the most interesting, unexpected, or challenging lessons that you have learned while working on the ML/AI elements of the MAD landscape?When is ML/AI the wrong choice for businesses?What are the areas of ML/AI that you are paying closest attention to in your own work?Contact InfoWebsite@mattturck on TwitterParting QuestionFrom your perspective, what is the biggest barrier to adoption of machine learning today?Closing AnnouncementsThank you for listening! Don't forget to check out our other shows. The Data Engineering Podcast covers the latest on modern data management. Podcast.__init__ covers the Python language, its community, and the innovative ways it is being used.Visit the site to subscribe to the show, sign up for the mailing list, and read the show notes.If you've learned something or tried out a project from the show then tell us about it! Email hosts@themachinelearningpodcast.com) with your story.To help other people find the show please leave a review on iTunes and tell your friends and co-workersLinksMAD LandscapeData Engineering Podcast EpisodeFirst Mark CapitalBayesian TechniquesHadoopChatGPTAutoGPTDataikuGenerative AIDatabricksMLOpsOpenAIAnthropicDeepMindBloombergGPTHuggingFaceJexi Movie"Her" MovieSynthesiaThe intro and outro music is from Hitman's Lovesong feat. Paola Graziano by The Freak Fandango Orchestra/CC BY-SA 3.0
SummaryA core challenge of machine learning systems is getting access to quality data. This often means centralizing information in a single system, but that is impractical in highly regulated industries, such as healthchare. To address this hurdle Rhino Health is building a platform for federated learning on health data, so that everyone can maintain data privacy while benefiting from AI capabilities. In this episode Ittai Dayan explains the barriers to ML in healthcare and how they have designed the Rhino platform to overcome them.AnnouncementsHello and welcome to the Machine Learning Podcast, the podcast about machine learning and how to bring it from idea to delivery.Your host is Tobias Macey and today I'm interviewing Ittai Dayan about using federated learning at Rhino Health to bring AI capabilities to the tightly regulated healthcare industryInterviewIntroductionHow did you get involved in machine learning?Can you describe what Rhino Health is and the story behind it?What is federated learning and what are the trade-offs that it introduces? What are the benefits to healthcare and pharmalogical organizations from using federated learning?What are some of the challenges that you face in validating that patient data is properly de-identified in the federated models?Can you describe what the Rhino Health platform offers and how it is implemented? How have the design and goals of the system changed since you started working on it?What are the technological capabilities that are needed for an organization to be able to start using Rhino Health to gain insights into their patient and clinical data? How have you approached the design of your product to reduce the effort to onboard new customers and solutions?What are some examples of the types of automation that you are able to provide to your customers? (e.g. medical diagnosis, radiology review, health outcome predictions, etc.)What are the ethical and regulatory challenges that you have had to address in the development of your platform?What are the most interesting, innovative, or unexpected ways that you have seen Rhino Health used?What are the most interesting, unexpected, or challenging lessons that you have learned while working on Rhino Health?When is Rhino Health the wrong choice?What do you have planned for the future of Rhino Health?Contact InfoLinkedInParting QuestionFrom your perspective, what is the biggest barrier to adoption of machine learning today?Closing AnnouncementsThank you for listening! Don't forget to check out our other shows. The Data Engineering Podcast covers the latest on modern data management. Podcast.__init__ covers the Python language, its community, and the innovative ways it is being used.Visit the site to subscribe to the show, sign up for the mailing list, and read the show notes.If you've learned something or tried out a project from the show then tell us about it! Email hosts@themachinelearningpodcast.com) with your story.To help other people find the show please leave a review on iTunes and tell your friends and co-workersLinksRhino HealthFederated LearningNvidia ClaraNvidia DGXMelloddyFlair NLPThe intro and outro music is from Hitman's Lovesong feat. Paola Graziano by The Freak Fandango Orchestra/CC BY-SA 3.0
SummarySatellite imagery has given us a new perspective on our world, but it is limited by the field of view for the cameras. Synthetic Aperture Radar (SAR) allows for collecting images through clouds and in the dark, giving us a more consistent means of collecting data. In order to identify interesting details in such a vast amount of data it is necessary to use the power of machine learning. ICEYE has a fleet of satellites continuously collecting information about our planet. In this episode Tapio Friberg shares how they are applying ML to that data set to provide useful insights about fires, floods, and other terrestrial phenomena.AnnouncementsHello and welcome to the Machine Learning Podcast, the podcast about machine learning and how to bring it from idea to delivery.Your host is Tobias Macey and today I'm interviewing Tapio Friberg about building machine learning applications on top of SAR (Synthetic Aperture Radar) data to generate insights about our planetInterviewIntroductionHow did you get involved in machine learning?Can you describe what ICEYE is and the story behind it?What are some of the applications of ML at ICEYE?What are some of the ways that SAR data poses a unique challenge to ML applications?What are some of the elements of the ML workflow that you are able to use "off the shelf" and where are the areas that you have had to build custom solutions?Can you share the structure of your engineering team and the role that the ML function plays in the larger organization?What does the end-to-end workflow for your ML model development and deployment look like? What are the operational requirements for your models? (e.g. batch execution, real-time, interactive inference, etc.)In the model definitions, what are the elements of the source domain that create the largest challenges? (e.g. noise from backscatter, variance in resolution, etc.)Once you have an output from an ML model how do you manage mapping between data domains to reflect insights from SAR sources onto a human understandable representation?Given that SAR data and earth imaging is still a very niche domain, how does that influence your ability to hire for open positions and the ways that you think about your contributions to the overall ML ecosystem?How can your work on using SAR as a representation of physical attributes help to improve capabilities in e.g. LIDAR, computer vision, etc.?What are the most interesting, innovative, or unexpected ways that you have seen ICEYE and SAR data used?What are the most interesting, unexpected, or challenging lessons that you have learned while working on ML for SAR data?What do you have planned for the future of ML applications at ICEYE?Contact InfoLinkedInParting QuestionFrom your perspective, what is the biggest barrier to adoption of machine learning today?Closing AnnouncementsThank you for listening! Don't forget to check out our other shows. The Data Engineering Podcast covers the latest on modern data management. Podcast.__init__ covers the Python language, its community, and the innovative ways it is being used.Visit the site to subscribe to the show, sign up for the mailing list, and read the show notes.If you've learned something or tried out a project from the show then tell us about it! Email hosts@themachinelearningpodcast.com) with your story.To help other people find the show please leave a review on iTunes and tell your friends and co-workersLinksICEYESAR == Synthetic Aperture RadarTransfer LearningThe intro and outro music is from Hitman's Lovesong feat. Paola Graziano by The Freak Fandango Orchestra/CC BY-SA 3.0
SummaryThe focus of machine learning projects has long been the model that is built in the process. As AI powered applications grow in popularity and power, the model is just the beginning. In this episode Josh Tobin shares his experience from his time as a machine learning researcher up to his current work as a founder at Gantry, and the shift in focus from model development to machine learning systems.AnnouncementsHello and welcome to the Machine Learning Podcast, the podcast about machine learning and how to bring it from idea to delivery.Your host is Tobias Macey and today I'm interviewing Josh Tobin about the state of industry best practices for designing and building ML modelsInterviewIntroductionHow did you get involved in machine learning?Can you start by describing what a "traditional" process for building a model looks like? What are the forces that shaped those "best practices"?What are some of the practices that are still necessary/useful and what is becoming outdated? What are the changes in the ecosystem (tooling, research, communal knowledge, etc.) that are forcing teams to reconsider how they think about modeling?What are the most critical practices/capabilities for teams who are building services powered by ML/AI? What systems do they need to support them in those efforts?Can you describe what you are building at Gantry and how it aids in the process of developing/deploying/maintaining models with "modern" workflows?What are the most challenging aspects of building a platform that supports ML teams in their workflows?What are the most interesting, innovative, or unexpected ways that you have seen teams approach model development/validation?What are the most interesting, unexpected, or challenging lessons that you have learned while working on Gantry?When is Gantry the wrong choice?What are some of the resources that you find most helpful to stay apprised of how modeling and ML practices are evolving?Contact InfoLinkedInWebsiteParting QuestionFrom your perspective, what is the biggest barrier to adoption of machine learning today?Closing AnnouncementsThank you for listening! Don't forget to check out our other shows. The Data Engineering Podcast covers the latest on modern data management. Podcast.__init__ covers the Python language, its community, and the innovative ways it is being used.Visit the site to subscribe to the show, sign up for the mailing list, and read the show notes.If you've learned something or tried out a project from the show then tell us about it! Email hosts@themachinelearningpodcast.com) with your story.To help other people find the show please leave a review on iTunes and tell your friends and co-workersLinksGantryFull Stack Deep LearningOpenAIKaggleNeurIPS == Neural Information Processing Systems ConferenceCaffeTheanoDeep LearningRegression Modelscikit-learnLarge Language ModelFoundation ModelsCohereFederated LearningFeature StoredbtThe intro and outro music is from Hitman's Lovesong feat. Paola Graziano by The Freak Fandango Orchestra/CC BY-SA 3.0
SummaryMachine learning models have predominantly been built and updated in a batch modality. While this is operationally simpler, it doesn't always provide the best experience or capabilities for end users of the model. Tecton has been investing in the infrastructure and workflows that enable building and updating ML models with real-time data to allow you to react to real-world events as they happen. In this episode CTO Kevin Stumpf explores they benefits of real-time machine learning and the systems that are necessary to support the development and maintenance of those models.AnnouncementsHello and welcome to the Machine Learning Podcast, the podcast about machine learning and how to bring it from idea to delivery.Your host is Tobias Macey and today I'm interviewing Kevin Stumpf about the challenges and promise of real-time ML applicationsInterviewIntroductionHow did you get involved in machine learning?Can you describe what real-time ML is and some examples of where it might be applied?What are the operational and organizational requirements for being able to adopt real-time approaches for ML projects?What are some of the ways that real-time requirements influence the scale/scope/architecture of an ML model?What are some of the failure modes for real-time vs analytical or operational ML?Given the low latency between source/input data being generated or received and a prediction being generated, how does that influence susceptibility to e.g. data drift? Data quality and accuracy also become more critical. What are some of the validation strategies that teams need to consider as they move to real-time?What are the most interesting, innovative, or unexpected ways that you have seen real-time ML applied?What are the most interesting, unexpected, or challenging lessons that you have learned while working on real-time ML systems?When is real-time the wrong choice for ML?What do you have planned for the future of real-time support for ML in Tecton?Contact InfoLinkedIn@kevinmstumpf on TwitterParting QuestionFrom your perspective, what is the biggest barrier to adoption of machine learning today?Closing AnnouncementsThank you for listening! Don't forget to check out our other shows. The Data Engineering Podcast covers the latest on modern data management. Podcast.__init__ covers the Python language, its community, and the innovative ways it is being used.Visit the site to subscribe to the show, sign up for the mailing list, and read the show notes.If you've learned something or tried out a project from the show then tell us about it! Email hosts@themachinelearningpodcast.com) with your story.To help other people find the show please leave a review on iTunes and tell your friends and co-workersLinksTectonPodcast EpisodeData Engineering Podcast EpisodeUber MichelangeloReinforcement LearningOnline LearningRandom ForestChatGPTXGBoostLinear RegressionTrain-Serve SkewFlinkData Engineering Podcast EpisodeThe intro and outro music is from Hitman's Lovesong feat. Paola Graziano by The Freak Fandango Orchestra/CC BY-SA 3.0
SummaryShopify uses machine learning to power multiple features in their platform. In order to reduce the amount of effort required to develop and deploy models they have invested in building an opinionated platform for their engineers. They have gone through multiple iterations of the platform and their most recent version is called Merlin. In this episode Isaac Vidas shares the use cases that they are optimizing for, how it integrates into the rest of their data platform, and how they have designed it to let machine learning engineers experiment freely and safely.AnnouncementsHello and welcome to the Machine Learning Podcast, the podcast about machine learning and how to bring it from idea to delivery.Your host is Tobias Macey and today I'm interviewing Isaac Vidas about his work on the ML platform used by ShopifyInterviewIntroductionHow did you get involved in machine learning?Can you describe what Shopify is and some of the ways that you are using ML at Shopify? What are the challenges that you have encountered as an organization in applying ML to your business needs?Can you describe how you have designed your current technical platform for supporting ML workloads? Who are the target personas for this platform?What does the workflow look like for a given data scientist/ML engineer/etc.?What are the capabilities that you are trying to optimize for in your current platform? What are some of the previous iterations of ML infrastructure and process that you have built?What are the most useful lessons that you gathered from those previous experiences that informed your current approach?How have the capabilities of the Merlin platform influenced the ways that ML is viewed and applied across Shopify?What are the most interesting, innovative, or unexpected ways that you have seen Merlin used?What are the most interesting, unexpected, or challenging lessons that you have learned while working on Merlin?When is Merlin the wrong choice?What do you have planned for the future of Merlin?Contact Info@kazuaros on TwitterLinkedInkazuar on GitHubParting QuestionFrom your perspective, what is the biggest barrier to adoption of machine learning today?Closing AnnouncementsThank you for listening! Don't forget to check out our other shows. The Data Engineering Podcast covers the latest on modern data management. Podcast.__init__ covers the Python language, its community, and the innovative ways it is being used.Visit the site to subscribe to the show, sign up for the mailing list, and read the show notes.If you've learned something or tried out a project from the show then tell us about it! Email hosts@themachinelearningpodcast.com) with your story.To help other people find the show please leave a review on iTunes and tell your friends and co-workersLinksShopifyShopify MerlinVertex AIscikit-learnXGBoostRayPodcast.__init__ EpisodePySparkGPT-3ChatGPTGoogle AIPyTorchPodcast.__init__ EpisodeDaskModinPodcast.__init__ EpisodeFlinkData Engineering Podcast EpisodeFeast Feature StoreKubernetesThe intro and outro music is from Hitman's Lovesong feat. Paola Graziano by The Freak Fandango Orchestra/CC BY-SA 3.0
Top Podcasts
The Best New Comedy Podcast Right Now – June 2024The Best News Podcast Right Now – June 2024The Best New Business Podcast Right Now – June 2024The Best New Sports Podcast Right Now – June 2024The Best New True Crime Podcast Right Now – June 2024The Best New Joe Rogan Experience Podcast Right Now – June 20The Best New Dan Bongino Show Podcast Right Now – June 20The Best New Mark Levin Podcast – June 2024
Our VIP Delhi Escort Girls are renowned for their beauty and first-class services. We run a high-class escort agency and so all the girls we offer are specially selected and groomed to give the most personal and pleasurable experience for our clients. We have been in operation for a lot of years and have a wide network of independent experts who are the best in their field. Our experience has helped us understand what our clients need when they wish to hire an Delhi escorts. http://poojaescorts.in/
Every time men want to hold them and express their sexual emotions completely in front of gorgeous escorts,. For the ultimate pleasure, erotic blondes take every possible step that is full of adventure and brings happiness to their clienhttps://www.komaldas.com
Thanks from https://www.readymatrimony.com
Great Episode , Thanks from
Thanks for Your article...it gives us immense knowledge. This is a titanic mixing article. I am all around that genuinely matters content with your https://www.poonamaggarwal.co.in/city/chennai https://www.poonamaggarwal.co.in/city/hyderabad https://www.poonamaggarwal.co.in/city/indore https://www.poonamaggarwal.co.in/city/jodhpur
Book your appointment today through our website or give us a call to body massage parlour in hyderabad center, we provide best spa services with hot massage girl therapists
Our massage parlour is well cleaned, every operative has their hands and noses covered with the room sanitized after each session visit https://www.spa69.in/
Our spa working on happy and healthy therapies customer’s should get worthy service to there money this is our main aim. Safe spa in bangalore with top notch ambiance friendly therapist make a visit https://www.ishaspa.com/
The masseuse then uses their entire body to massage the recipient, sliding and gliding along their body in a rhythmic and sensual manner. body to body massage in chennai can be highly intimate and erotic, focusing on relaxation and pleasure https://vipbodyspa.com/
Very interesting post you have crafted hear. https://www.kolkatadolls.com/ I am blessed to have come across this resolutions post. Thanks.
Very interesting post you have crafted hear. I am blessed to have come across this resolutions post. Thanks. https://www.kolkatadolls.com/
nice post thanks for sharing, visit us: https://bodymassageinchennai.in/
Awesome
✨✨
Our hot massage girls are always to assist you, they can bring your evening a joy, and you are able to avail all these services at our body-to-body massage at chennai center
nice post thanks for sharing, visit us: https://ginisharma.in
My name Gini Sharma from Chandigarh. I'm sweet , warm , lovely and amicable, free escort young lady. My body is attractive and regular. Every one of my photos are late and genuine. Incall and outcalls also offer class and great assistance. Make sure to me, message me on WhatsApp. I'm sitting tight for you? https://ginisharma.in
If you are looking for a body to body massage in chennai centre in my area, flipbody spa is the top massage centre in Chennai. so come and take best services
As you settle onto the plush massage table, skilled hands expertly knead away tension and tightness, unraveling knots and restoring balance to your body. The therapist's rhythmic motions and precise techniques coax muscles into a state of deep relaxation, releasing built-up stress and promoting circulation. https://www.spasweety.com/
As you settle onto the plush massage table, skilled hands expertly knead away tension and tightness, unraveling knots and restoring balance to your body. The therapist's rhythmic motions and precise techniques coax muscles into a state of deep relaxation, releasing built-up stress and promoting circulation. With each stroke, you feel tension dissipate, and a sense of rejuvenation washes over you, leaving you feeling renewed and invigorated. https://www.spasweety.com/