Discover
Interconnects Audio
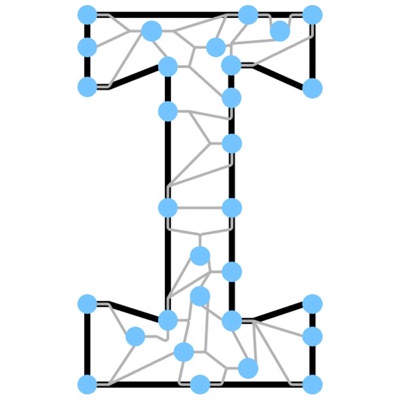
41 Episodes
Reverse
I Switched to Claude 3.5Speculations on the role of RLHF and why I love the model for people who pay attention.This is AI generated audio with Python and 11Labs.Source code: https://github.com/natolambert/interconnects-toolsOriginal post: https://www.interconnects.ai/p/switched-to-claude-from-chatgpt00:00 I Switched to Claude 3.503:57 Product priorities05:15 RLHF's peak?Fig 1: https://huggingface.co/datasets/natolambert/interconnects-figures/resolve/main/claude/img_016.pngFig 2: https://huggingface.co/datasets/natolambert/interconnects-figures/resolve/main/claude/img_018.pngFig 3: https://huggingface.co/datasets/natolambert/interconnects-figures/resolve/main/claude/img_020.pngFig 4: https://huggingface.co/datasets/natolambert/interconnects-figures/resolve/main/claude/img_022.png
I’m really excited to resume the Interconnects Interviews with Dean W. Ball from the Hyperdimensional Substack. We cover the whole stack of recent happenings in AI policy, focusing of course on California’s bill SB 1047. We cover many, many more great topics here including:What will happen in the case of a minor AI disaster,If Meta will release the 405B model, and why,The status of Chinese open-source AI,Training on model outputs,Anthropic’s recent strategy,What scaling laws actually mean,Creating content and shifting the needle of the AI discourse.View online: https://www.interconnects.ai/p/interviewing-dean-ball-on-ai-policyChapters00:00 Intro and Welcome Dean Ball 02:44 The Origins of California Bill SB1047 08:56 The Evolution of Bill SB1047 13:00 How SB1047 Affects Fine-Tuning 20:00 The Future of Bill SB1047 21:58 The Impact of AI Disasters 29:02 Meta and its 400 billion Parameter Model 32:25 Open Source AI and the Chinese Market 37:37 The Future of Open Source AI 43:35 Synthetic Data, Licenses, and Future AI Development 45:18 Anthropic's Approach to AI Safety 50:46 Scaling Laws 53:01 The Role of Audience in Influencing AI Policy
Things to be aware of if you work on language model fine-tuning.This is AI generated audio with Python and 11Labs.Source code: https://github.com/natolambert/interconnects-toolsOriginal post: https://www.interconnects.ai/p/rlhf-roundup-202400:00 RLHF Roundup: Trying to get good at PPO, charting RLHF's impact, RewardBench retrospective, and a reward model competition04:32 How big is the impact of RLHF relative to pretraining?05:54 RewardBench retrospective after 100 models and 90% peak accuracy09:19 LMSYS's reward modeling competitionFig 1: https://huggingface.co/datasets/natolambert/interconnects-figures/resolve/main/rlhf-roundup/img_009.pngFig 2: https://huggingface.co/datasets/natolambert/interconnects-figures/resolve/main/rlhf-roundup/img_012.pngFig 3: https://huggingface.co/datasets/natolambert/interconnects-figures/resolve/main/rlhf-roundup/img_017.pngFig 4: https://huggingface.co/datasets/natolambert/interconnects-figures/resolve/main/rlhf-roundup/img_026.png
Synthetic data is known to be a super powerful tool for every level of the language modeling stack. It's documented as being used for expanding vanilla pretraining data and creating large swaths of fine-tuning data. Many, many more rumors surround its use, Anthropic's pretraining-scale constitutional AI, Mistral AI's first models being pretrained on OpenAI outputs, Q-star's hopes as OpenAI's remaining moat, and much more. The diversity of use cases for synthetic data makes planning around the role of synthetic data in solving specific goals.This is AI generated audio with Python and 11Labs.Source code: https://github.com/natolambert/interconnects-toolsOriginal post: https://www.interconnects.ai/p/frontiers-in-synthetic-data00:00 Frontiers in synthetic data01:14 1. Direct distillation is still king02:54 2. Are Gemini Flash and Claude Haiku distilled?04:03 3. Filtering prevents collapse06:30 4. Synthetic data strategy taxes07:32 5. Pros and cons of training on multi-output-source synthetic datasets08:54 6. Structured synthetic data09:42 7. Weak-to-strong generalization is maybe real10:27 8. Creating synthetic prompts is overlooked again
Signs point to a general-use Sora-like model coming very soon, maybe even with open-weights.This is AI generated audio with Python and 11Labs.Source code: https://github.com/natolambert/interconnects-toolsOriginal post: https://www.interconnects.ai/p/text-to-video-ai-is-already-abundant0:00 Text-to-video AI is already abundant5:08 What's next for the text-to-video market?6:49 Are text-to-video models good for the world?Fig 1: https://huggingface.co/datasets/natolambert/interconnects-figures/resolve/main/text-to-video/img_005.mp4Fig 2: https://huggingface.co/datasets/natolambert/interconnects-figures/resolve/main/text-to-video/img_009.mp4Fig 3: https://huggingface.co/datasets/natolambert/interconnects-figures/resolve/main/text-to-video/img_011.mp4Fig 4: https://huggingface.co/datasets/natolambert/interconnects-figures/resolve/main/text-to-video/img_013.mp4Fig 5: https://huggingface.co/datasets/natolambert/interconnects-figures/resolve/main/text-to-video/img_015.mp4Fig 6: https://huggingface.co/datasets/natolambert/interconnects-figures/resolve/main/text-to-video/img_017.mp4
Apple Intelligence makes a lot of sense when you get out of the AI bubble.This is AI generated audio with Python and 11Labs.Source code: https://github.com/natolambert/interconnects-toolsOriginal post: https://www.interconnects.ai/p/apple-intelligence00:00 AI for the rest of us02:46 Apple's technical approach03:32 Core models: What did Apple build?05:35 Alignment strategies: Some new things!10:00 Orchestrating adapters and on-device magic11:58 Light for other narratives around AIFig 1: https://huggingface.co/datasets/natolambert/interconnects-figures/resolve/main/apple-intelligence/img_005.pngFig 2: https://huggingface.co/datasets/natolambert/interconnects-figures/resolve/main/apple-intelligence/img_015.pngFig 3: https://huggingface.co/datasets/natolambert/interconnects-figures/resolve/main/apple-intelligence/img_039.pngFig 4: https://huggingface.co/datasets/natolambert/interconnects-figures/resolve/main/apple-intelligence/img_041.png
A realistic path to robotic foundation modelsNot "agents" and not "AGI." Some thoughts and excitement after revisiting the industry thanks to Physical Intelligence founders Sergey Levine and Chelsea Finn.This is AI generated audio with Python and 11Labs.Source code: https://github.com/natolambert/interconnects-toolsOriginal post: https://www.interconnects.ai/p/robotic-foundation-models0:00 A realistic path to robotic foundation models2:51 Key factors for the future of robotics6:19 Everything is a token: The transformerification of robotics
Data licensing deals, scaling, human inputs, and repeating trends in open vs. closed.This is AI generated audio with Python and 11Labs.Source code: https://github.com/natolambert/interconnects-toolsOriginal post: https://www.interconnects.ai/p/the-data-wall0:00 We aren't running out of training data, we are running out of open training data2:51 Synthetic data: 1 trillion new tokens per day4:18 Data licensing deals: High costs per token6:33 Better tokens: Search and new frontiers
Celebrity's power will only grow in the era of infinite content.This is AI generated audio with Python and 11Labs.Source code: https://github.com/natolambert/interconnects-toolsOriginal post: https://www.interconnects.ai/p/name-image-and-ai-likeness0:00 Name, image, and AI's likeness1:11 OpenAI's second terrible, horrible, no good, very bad week4:36 The expansion of name and likeness7:46 Culture and AI development
ChatGPT leaves the textbox, and Google is building the same, and more, as practical tools.This is AI generated audio with Python and 11Labs.Source code: https://github.com/natolambert/interconnects-toolsOriginal post: https://www.interconnects.ai/p/openai-and-her00:00 OpenAI chases Her02:10 Talking to ChatGPT03:53 GPT-4o: Toward omnimodal models08:25 Google's mirror with Gemini10:11 OpenAI's AI Safety: Have your cake and eat it tooFig 1: https://huggingface.co/datasets/natolambert/interconnects-figures/resolve/main/her/img_018.pngFig 2: https://huggingface.co/datasets/natolambert/interconnects-figures/resolve/main/her/img_023.jpg
Now we will have some grounding for when weird ChatGPT behaviors are intended or side-effects -- shrinking the Overton window of RLHF bugs.This is AI generated audio with Python and 11Labs.Source code: https://github.com/natolambert/interconnects-toolsOriginal post: https://www.interconnects.ai/p/openai-rlhf-model-spec00:00 OpenAI's Model (behavior) Spec, RLHF transparency, and personalization questions02:56 Reviewing the Model Spec08:26 Where RLHF can fail OpenAI12:23 From Model Spec's to personalizationFig 1: https://huggingface.co/datasets/natolambert/interconnects-figures/resolve/main/model-spec/img_027.pngFig 2: https://huggingface.co/datasets/natolambert/interconnects-figures/resolve/main/model-spec/img_029.pngFig 3: https://huggingface.co/datasets/natolambert/interconnects-figures/resolve/main/model-spec/img_033.pngFig 4: https://huggingface.co/datasets/natolambert/interconnects-figures/resolve/main/model-spec/img_034.pngFig 5: https://huggingface.co/datasets/natolambert/interconnects-figures/resolve/main/model-spec/img_041.webpFig 6: https://huggingface.co/datasets/natolambert/interconnects-figures/resolve/main/model-spec/img_046.webp
Many, many signs of life for preference fine-tuning beyond spoofing chat evaluation tools.This is AI generated audio with Python and 11Labs.Source code: https://github.com/natolambert/interconnects-toolsOriginal post: https://www.interconnects.ai/p/how-rlhf-works-200:00 How RLHF works, part 2: A thin line between useful and lobotomized04:27 The chattiness paradox08:09 The mechanism for making models chattier10:42 Next steps for RLHF researchFig 1: https://huggingface.co/datasets/natolambert/interconnects-figures/resolve/main/rlhf/img_012.webpFig 2: https://huggingface.co/datasets/natolambert/interconnects-figures/resolve/main/rlhf/img_018.pngFig 3: https://huggingface.co/datasets/natolambert/interconnects-figures/resolve/main/rlhf/img_025.png
Models that seem totally out of scope from recent open LLMs give us a sneak peek of where the industry will be in 6 to 18 months.This is AI generated audio with Python and 11Labs.Source code: https://github.com/natolambert/interconnects-toolsOriginal post: https://www.interconnects.ai/p/phi-3-and-arctic-llms0:00 Phi 3 and Arctic: Outlier LMs are hints1:01 Arctic & open mixture of expert trends6:10 Phi 3, synthetic data, and small modelsFig 1: https://huggingface.co/datasets/natolambert/interconnects-figures/resolve/main/phi3/img_004.pngFig 2: https://huggingface.co/datasets/natolambert/interconnects-figures/resolve/main/phi3/img_008.pngFig 3: https://huggingface.co/datasets/natolambert/interconnects-figures/resolve/main/phi3/img_018.png
Certain definitions of AGI are backing people into a pseudo-religious corner.This is AI generated audio with Python and 11Labs.Source code: https://github.com/natolambert/interconnects-toolsOriginal post: https://www.interconnects.ai/p/agi-is-what-you-want-it-to-be00:00 AGI is what you want it to be04:01 RL still rules the AGI discourse05:43 Modern AGI tests07:37 Agency and shifting goalpostsFig 1: https://huggingface.co/datasets/natolambert/interconnects-figures/resolve/main/agi/img_018.pngFig 2: https://huggingface.co/datasets/natolambert/interconnects-figures/resolve/main/agi/img_020.png
Meta shows that scaling won't be a limit for open LLM players in the near future.This is AI generated audio with Python and 11Labs.Source code: https://github.com/natolambert/interconnects-toolsOriginal post: https://www.interconnects.ai/p/llama-3-and-scaling-open-llms00:00 Llama 3; scaling open LLMs to AGI01:44 Pretraining, data, and basic evals06:06 Alignment and human evaluations10:08 Chatting with Meta AI and Llama 3 70B Instruct11:55 Same Llama license (mostly)12:52 The healthy open LLM ecosystemFig 1: https://huggingface.co/datasets/natolambert/interconnects-figures/resolve/main/llama3/img_011.jpegFig 2: https://huggingface.co/datasets/natolambert/interconnects-figures/resolve/main/llama3/img_013.pngFig 3: https://huggingface.co/datasets/natolambert/interconnects-figures/resolve/main/llama3/img_015.pngFig 4: https://huggingface.co/datasets/natolambert/interconnects-figures/resolve/main/llama3/img_020.pngFig 5: https://huggingface.co/datasets/natolambert/interconnects-figures/resolve/main/llama3/img_036.pngFig 6: https://huggingface.co/datasets/natolambert/interconnects-figures/resolve/main/llama3/img_040.pngFig 7: https://huggingface.co/datasets/natolambert/interconnects-figures/resolve/main/llama3/img_046.jpegFig 8: https://huggingface.co/datasets/natolambert/interconnects-figures/resolve/main/llama3/img_061.pngFig 9: https://huggingface.co/datasets/natolambert/interconnects-figures/resolve/main/llama3/img_063.webpFig 10: https://huggingface.co/datasets/natolambert/interconnects-figures/resolve/main/llama3/img_066.pngFig 11: https://huggingface.co/datasets/natolambert/interconnects-figures/resolve/main/llama3/img_068.jpeg
Integrating some non computing science into reinforcement learning from human feedback can give us the models we want.This is AI generated audio with Python and 11Labs.Source code: https://github.com/natolambert/interconnects-toolsOriginal post: https://www.interconnects.ai/p/reinventing-llm-alignment0:00 Stop "reinventing" everything to "solve" AI alignment2:19 Social Choice for AI Alignment: Dealing with Diverse Human Feedback7:03 OLMo 1.7 7B: A truly open model with actually good benchmarksFig 1: https://huggingface.co/datasets/natolambert/interconnects-figures/resolve/main/reinvention/img_013.pngFig 2: https://huggingface.co/datasets/natolambert/interconnects-figures/resolve/main/reinvention/img_015.pngFig 3: https://huggingface.co/datasets/natolambert/interconnects-figures/resolve/main/reinvention/img_018.pngFig 4: https://huggingface.co/datasets/natolambert/interconnects-figures/resolve/main/reinvention/img_024.pngFig 5: https://huggingface.co/datasets/natolambert/interconnects-figures/resolve/main/reinvention/img_027.png
Modeling the compute versus performance tradeoff of many open LLMs.This is AI generated audio with Python and 11Labs.Source code: https://github.com/natolambert/interconnects-toolsOriginal post: https://www.interconnects.ai/p/compute-efficient-open-llms0:00 The end of the "best open LLM"3:05 Compute efficient open LLMsFig 1: https://huggingface.co/datasets/natolambert/interconnects-figures/resolve/main/scaling/img_004.jpegFig 2: https://huggingface.co/datasets/natolambert/interconnects-figures/resolve/main/scaling/img_009.pngFig 3: https://huggingface.co/datasets/natolambert/interconnects-figures/resolve/main/scaling/img_014.pngFig 4: https://huggingface.co/datasets/natolambert/interconnects-figures/resolve/main/scaling/img_016.pngFig 5: https://huggingface.co/datasets/natolambert/interconnects-figures/resolve/main/scaling/img_018.pngFig 6: https://huggingface.co/datasets/natolambert/interconnects-figures/resolve/main/scaling/img_020.pngFig 7: https://huggingface.co/datasets/natolambert/interconnects-figures/resolve/main/scaling/img_022.pngFig 8: https://huggingface.co/datasets/natolambert/interconnects-figures/resolve/main/scaling/img_024.pngFig 9: https://huggingface.co/datasets/natolambert/interconnects-figures/resolve/main/scaling/img_028.png
Last minute title change from: The tech industry can't agree on what open-source AI means. That's the process.How to read what multiple people mean by the word openness and see through the PR speak.This is AI generated audio with Python and 11Labs.Source code: https://github.com/natolambert/interconnects-toolsOriginal post: https://www.interconnects.ai/p/flavors-of-open-source-ai0:00 The tech industry can't agree on what open-source AI means. That's the process.2:45 1. Effective Accelerationists, Techno-Optimists, capitalists, etc.3:39 2. Scientists, promoting understanding and transparency5:16 3. Inclusion, public interest, and fighting concentration of power6:19 4. Freedom advocates7:25 Dissecting "openness"Fig 1: https://huggingface.co/datasets/natolambert/interconnects-figures/resolve/main/openness/img_004.png
Databricks' new model is surpassing the performance of Mixtral and Llama 2 while still being in a size category that's reasonably accessible.This is AI generated audio with Python and 11Labs.Source code: https://github.com/natolambert/interconnects-toolshttps://www.interconnects.ai/p/databricks-dbrx-open-llm00:00 DBRX: The new best open model and Databricks' ML strategy03:36 The DBRX narrative07:33 Databricks' open LLM (and AI) strategy09:42 Playing with DBRX Instruct14:54 Digging for detailsFig 1: https://huggingface.co/datasets/natolambert/interconnects-figures/resolve/main/dbrx/img_007.pngFig 2: https://huggingface.co/datasets/natolambert/interconnects-figures/resolve/main/dbrx/img_012.pngFig 3: https://huggingface.co/datasets/natolambert/interconnects-figures/resolve/main/dbrx/img_023.pngFig 4: https://huggingface.co/datasets/natolambert/interconnects-figures/resolve/main/dbrx/img_045.pngFig 5: https://huggingface.co/datasets/natolambert/interconnects-figures/resolve/main/dbrx/img_047.pngFig 6: https://huggingface.co/datasets/natolambert/interconnects-figures/resolve/main/dbrx/img_059.pngFig 7: https://huggingface.co/datasets/natolambert/interconnects-figures/resolve/main/dbrx/img_066.jpegFig 8: https://huggingface.co/datasets/natolambert/interconnects-figures/resolve/main/dbrx/img_068.png
Evaluation is not only getting harder with modern LLMs, it's getting harder because it means something different.This is AI generated audio with Python and 11Labs. Music generated by Meta's MusicGen.Source code: https://github.com/natolambert/interconnects-toolsOriginal post: https://www.interconnects.ai/p/evaluations-trust-performance-and-price00:00 Evaluations: Trust, performance, and price (bonus, announcing RewardBench)03:14 The rising price of evaluation05:40 Announcing RewardBench: The First reward model evaluation tool08:37 Updates to RLHF evaluation toolsYouTube code intro: https://youtu.be/CAaHAfCqrBAFigure 1: https://huggingface.co/datasets/natolambert/interconnects-figures/resolve/main/evals/img_026.pngFigure 2: https://huggingface.co/datasets/natolambert/interconnects-figures/resolve/main/evals/img_030.pngFigure 3: https://huggingface.co/datasets/natolambert/interconnects-figures/resolve/main/evals/img_034.pngFigure 4: https://huggingface.co/datasets/natolambert/interconnects-figures/resolve/main/evals/img_040.png
Comments