Discover
Knowledge Graph Insights
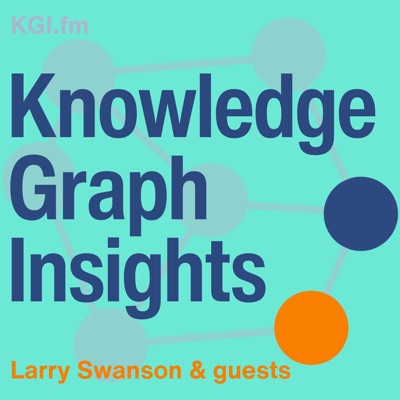
15 Episodes
Reverse
Michael Iantosca
Where content, knowledge management, and AI converge, you'll find Michael Iantosca.
As many in the AI world flock to probabilistic models like LLMs, Michael takes a deterministic approach to content management and knowledge engineering, using ontologies and knowledge graphs to ground content in a concrete facts.
This approach embodies his insight that content and the models that describe it are not static information but rather valuable, ever-evolving enterprise IP assets.
We talked about:
his 44-year career in content, knowledge management and localization/globalization roles
the three pillars of his work: content, knowledge management, and engineering
the need he sees in his work to move away from probabilistic, vector-based models to deterministic, neuro-symbolic models like knowledge graphs
how he decides which models are appropriate to use with each of the varied kinds of data he works with
his explorations of how to automatically construct a knowledge graph to use to power generative AI solutions
how he acquires and develops ontology skills in his team
how graph technology supports the "total content experiences" he builds
how the non-static nature of content makes it a poor candidate to be managed in a static system like a vector-based model
the relative merits and utility of 1) deterministic retrieval for structured content and 2) probabilistic retrieval for unstructured content
the power of combining content models, knowledge models, and ontologies and how they can become crucial enterprise IP assets
his belief that we are entering a golden age of content and knowledge engineering
Michael's bio
Michael Iantosca is the Senior Director of Knowledge Platforms and Engineering at Avalara, a sales tax automation company. With over four decades of leadership in technical content management, Michael has been a pioneer in advancing the profession, driving innovations in structured content, intelligent authoring, and scalable knowledge platforms. Renowned for bridging engineering and content teams, he has championed the adoption of AI and cutting-edge technologies to enhance user experience. A thought leader and mentor, Michael continues to shape the future of technical communication through his expertise and passion for innovation.
Connect with Michael online
LinkedIn
Medium
ThinkingDocumentation
Video
Here’s the video version of our conversation:
https://youtu.be/WG9Nl5OY3QI
Podcast intro transcript
This is the Knowledge Graph Insights podcast, episode number 16. A lot of work in the AI world these days is about vectorizing giant collections of static, unstructured content and data for LLMs. Michael Iantosca has worked for decades in a world where content is dynamic, always precisely structured, and contextualized with rich metadata. So he has a different take on architectural innovations like graph RAG, favoring knowledge-based deterministic retrieval of content over vector-based models and probabilistic methods.
Interview transcript
Larry:
Hi, everyone. Welcome to episode number 16 of the knowledge graph Insights podcast. I am really delighted today to welcome to the show, Michael Iantosca. Michael is currently the Senior Director of Knowledge Platforms and Engineering at Avalara, the big tax-compliance automation software company. He's also got a long history. He's spent a couple of decades, a few decades at IBM prior to his role at Avalara. Welcome to the show, Michael. Tell the folks a little bit more about what you're up to these days.
Michael:
Larry, thank you for having me. It's a pleasure and an honor to get a few minutes to talk to you today. Yeah, I have just started my 44th year primarily in the professional content space, but also in the knowledge management and localization globalization space as well. I have been involved with content since the early days of SGML that began the structured content revolution and wo...
Fran Alexander
When Fran Alexander looks at the current AI landscape she sees some interesting parallels between the Alien vs Predator science fiction franchise and the way RAG and other architectures are combining LLMs and knowledge graphs.
We talked about:
the analogy she draws between the Alien and Predator science fiction franchise with LLMs and knowledge graphs
how the human-esque (if malevolent) cognitive and behavioral nature of Predators aligns more with knowledge graphs and how the unpredictable and stochastic nature of Aliens aligns more with LLMs
how the eloquence of LLM outputs can deceive humans
the lack of explainability and transparency in both Alien and LLM behavior, and the opposite in knowledge graphs
the difficulty of dealing with baked-in biases in LLMs
the lack of repeatability in LLMs and the opposite in KGs
the current trend of architectures and practices like RAG that draw on the strengths of KGs and LLMs to get better results, just as the Alien and Predator media franchises combined forces
how over the past year or so investment in LLMs has overshadowed all other investments, just as Aliens are out to wipe out anything that's not an Alien
her approach to AI architectures that combine LLMs and knowledge graphs
how different kinds of people consume LLM output
how she helps enterprise decision makers choose whether to address a use case with a knowledge graph or an LLM
how taxonomists and ontologists can use LLMs in their work
the Alien Loves Predator UK Facebook group and Alien and Predator on a seesaw
Alien and Predator cosplay actors on a seesaw
Fran's bio
Fran started her career as a writer and editor of dictionaries and thesauruses in the UK, and, as technology evolved, she specialised in information architecture, search systems, and digital archives, and more recently, the use of semantics in knowledge graphs and LLM applications. Having worked on reference publications including the Collins English Dictionary, and as Taxonomy Manager for the BBC Archive, she now lives in Montreal, Canada, and is the Senior Taxonomist for Expedia Group. She was Taxonomy Bootcamp London’s Taxonomy Practitioner of the Year 2023.
Connect with Fran online
LinkedIn
Video
Here’s the video version of our conversation:
https://youtu.be/VWwDBIws6G8
Podcast intro transcript
This is the Knowledge Graph Insights podcast, episode number 15. When two impressive domains converge, amazing things can happen. When the Alien and Predator science fiction franchises joined forces, both enjoyed new commercial success. Similarly, in the AI world right now, Fran Alexander sees knowledge graphs and large language models combining forces to create retrieval augmented generation and similar architectures that work together to create systems more useful and valuable than the sum of their individual capabilities.
Interview transcript
Larry:
Hi everyone. Welcome to episode number 15 of the Knowledge Graph Insights podcast. I am really delighted today to welcome to the show, Fran Alexander. Fran is an independent taxonomist and ontologist based in Montreal. And so, welcome Fran. Tell the folks a little bit more about what you're up to these days.
Fran:
Hi Larry. Well, it's nice to talk to you again. I really enjoyed talking to you on the previous podcast that we did a little while ago. And that one was kind of a bit of a general introduction to taxonomies, ontologies, thesauruses, knowledge modeling and semantics. But this time, I thought we could talk about knowledge graphs and LLMs. They're a big hot topic and I did a presentation earlier on in the year for Taxonomy Boot Camp London, Bite-sized Taxonomy Boot Camp London. That was a lot of fun and has been really popular. A lot of people have been asking me about it. I've revisited it a couple of times and that's LLMs versus knowledge graphs, Alien versus Predator.
Larry:
Okay. So why Alien versus Predator?
Andreas Blumauer
Every enterprise nowadays is awash in data, content, and knowledge, the understanding of which is all too often available only in employees' heads.
Forward-thinking businesses are moving to knowledge graphs to capture that tacit knowledge so that they can better understand and use it.
Andreas Blumauer shows how those graphs work best when they're accompanied by a domain knowledge model, creating a "semantic layer" that provides a vivid map of your business knowledge.
We talked about:
the recent merger of his company, Semantic Web Company, with Ontotext to form a new company, Graphwise, which aims to help enterprises build their semantic layer
the 20-year-old origin story behind his definition and description of a semantic layer
the emerging trend he sees of data, content, and knowledge people coming together, often around explorations of language and content structure
how getting domain knowledge out of people's heads and into multimodal AI architectures can streamline business research
how a semantic layer provides a map of your business knowledge
the importance of a domain knowledge model in a semantic layer architecture
the inadequacy of "desktop data integration," the practice of calling colleagues, consulting varied sources, and otherwise searching through ad hoc enterprise knowledge sources, and the stress it can cause
how a domain knowledge model can connect and reveal knowledge across an organization
the surprisingly small footprint of the domain knowledge model in an enterprise knowledge graph, typically just 1% or so of the semantic layer
how LLMs can help in the discovery of data and the construction of knowledge graphs
the two main elements of the semantic layer: domain knowledge models (taxonomies, ontologies, etc.) and an automatically generated enterprise knowledge graph
the different perceptions of the value of the semantic layer across data and content professionals, and how the arrival of gen AI has resulted in them talking together more to each other
how the semantic layer can facilitate the alignment of vocabularies and the understanding of data across business divisions
the benefits of a hybrid centralized-decentralized/global-local "glocalization" semantic layer strategy
Andreas' bio
Andreas Blumauer is SVP Growth at Graphwise, and CEO and founder of the Semantic Web Company (SWC), provider and developer of the PoolParty Semantic Platform and leading solution provider in the field of semantic AI and RAG. For more than 20 years, he has worked with more than 200 organizations worldwide to deliver AI and semantic search solutions, knowledge platforms, content hubs and related data modeling and integration services. Most recently, Andreas has been involved in the development of AI-powered ESG solutions for companies and investors.
A globally recognized thought leader and author in the field of semantic AI and graph technologies, Andreas has helped define and implement knowledge and AI strategies for various industries and domains. He is the author of "The Knowledge Graph Cookbook: Recipes that Work", a practical guide to building and deploying knowledge graphs in organizations. He is passionate about enabling clients to harness the power of semantic AI and graph technologies to achieve their business goals and make a social contribution.
Since 2022, Andreas has focused primarily on developing AI-based solutions that help organizations implement ESG and sustainable systems.
Connect with Andreas online
LinkedIn
Graphwise
Resources mentioned in this episode
Knowledge Graphs, LLMs and Semantic AI LinkedIn group
From Data to Trust: Leveraging Knowledge Graphs for Enterprise AI Solutions webinar
Enterprise architecture model that includes a semantic layer
Video
Here’s the video version of our conversation:
https://youtu.be/dEQh6m6zoDE
Podcast intro transcript
Jessica Talisman
Jessica Talisman is a seasoned information architect with decades of experience across a variety of domains.
She's done a lot of education and outreach around her semantic and and information architecture practices. One of the most important lessons she's learned is the crucial role of standards like the W3C SKOS model to bring structure and semantics to information and knowledge systems.
Since there are never enough information architects in any organization, she supports the democratization of IA practices, but she's also quick to highlight the unique skills that you can only get with deep study.
We talked about:
her work as a senior information architect at Adobe and previously in GLAM (galleries, libraries, art, and museums) and other domains
how her work in GLAM showed her the importance of the concept of lineage and attribution and benefits of the FRBR (Functional Requirements for Bibliographic Records) framework
how standards and rules bring discipline and structure to information and data ecosystems
how capturing knowledge via the SKOS standard can provide on its own the structure, semantics, and disambiguation your data needs, as well as set you up for future successes
the importance of focusing on semantic fundamentals and how the ensuing understanding if your data assets can improve activities like a graph RAG implementation
the importance of collaborating and sharing ideas across domains
democratization, evangelism, and other kinds of information architecture outreach
the "Golden Spike" railroad metaphor she uses to illustrate cross-functional collaboration challenges
how linked data can help span organizational silos and align stakeholders on language and terminology
the importance of understanding your unique organizational fingerprint
how applying the library science concept of "scholarly communications" can move organizations forward and promote innovation
Jessica's bio
Jessica Talisman is a Senior Information Architect at Adobe. She has been building information systems to support human and machine information retrieval for more than 25 years. Jessica has worked in a variety of domains such as e-commerce, government, AdTech, EdTech and GLAM. Jessica holds a Masters in Library and Information Science with a concentration in Informatics. She lives in Santa Cruz, California with her partner Dave, and two dogs.
Connect with Jessica online
LinkedIn - Jessica is working on a new book about information architecture and is looking for anecdotes and other input. If you're an IA practitioner with good stories to share, she'd love to connect.
Video
Here’s the video version of our conversation:
https://youtu.be/1tlrZTJ52Vs
Podcast intro transcript
This is the Knowledge Graph Insights podcast, episode number 12. Anyone who has tried to discern how people in a domain talk about the concepts in it, and then try to align stakeholders in an organization around those concepts and the words that describes them, and then share that information with computers so that you can scale the impact of your work, knows that you need a good system to manage your taxonomies and other terminology. Jessica Talisman argues that the W3C SKOS standard is your best friend in such endeavors.
Interview transcript
Larry:
Okay. Hi everyone. Welcome to episode number 12 of the Knowledge Graph Insights Podcast. I am really delighted today to welcome to the show Jessica Talisman. Jessica's currently a senior information architect at Adobe, but she is extremely experienced in information architecture and knowledge graph stuff, so welcome Jessica, tell the folks a little bit more about what you're up to these days.
Jessica:
Thanks, Larry. So I'm currently, as Larry said, a senior information architect at Adobe, and before this, I was information architect over at Amazon. I've worked in many different domain spaces,
Tony Seale
With ten years of semantic data experience and an endless stream of insightful posts on LinkedIn, Tony Seale has earned the moniker "The Knowledge Graph Guy."
Tony says there's precious little time for enterprises to prepare their data with the interconnectedness and semantic meaning that it needs to be ready for the coming wave of more powerful AI technology.
We talked about:
his 10-year history of applying academic knowledge graph insights to commercial work, mostly in the finance industry
the yin-yang relationship in his "neuro-symbolic loop" concept that connects creative, generative LLMs and the reliable, structured knowledge provided by knowledge graphs
the contrast in reasoning capabilities between LLMs and knowledge graphs
how neither formal logic nor probabilistic systems are rarely the right answer on their own, hence the yin-yang analogy
the crucial role of understanding and consolidating data, the gold mine on which every enterprise is sitting that describes any organization's unique value
the power of understanding the "ontological core" of your business and then projecting it, selectively and strategically, to the world
the urgent threat posed by snake oil salesmen and other opportunists coming into the graph world and derailing enterprises' chances to properly exploit their unique data advantage
the two crucial characteristics of AI-ready data: connectedness and semantic meaning
his work chairing the Data Product Ontology (DPROD) working group, an effort to provide a semantic definition of what a data product is
Tony's bio
For over a decade, Tony has been passionate about linking data. His creative vision for integrating Large Language Models (LLMs) and Knowledge Graphs in large organisations has gained widespread attention, particularly through his popular weekly LinkedIn posts, earning him the reputation of 'The Knowledge Graph Guy.'
Tony’s journey into AI and knowledge graphs began as a secret side project, working from a computer under his desk while employed at an investment bank. What started as a personal passion quickly evolved into an area of deep expertise. Over the past decade, Tony has successfully delivered several mission-critical Knowledge Graphs into production for Tier 1 investment banks, helping these institutions better organise and leverage their data.
Now, Tony has just founded The Knowledge Graph Guys, a brand-new consultancy dedicated to making knowledge graphs accessible to organisations of all sizes. Through this venture, he aims to empower businesses with the tools and strategies needed to harness this powerful technology.
Connect with Tony online
LinkedIn
The Knowledge Graph Guys
Resources mentioned in this podcast
Connected Data London conference
Knowledge Graph Conference
GraphGeeks podcast
DPROD working group
Video
Here’s the video version of our conversation:
https://youtu.be/lkNvCzwhTRY
Podcast intro transcript
This is the Knowledge Graph Insights podcast, episode number 11. Whether they realize it or not, every business on the planet is sitting on a gold mine, the precious data that uniquely positions them in their industry and market. With ten years of AI practice and an endless stream of insightful social media posts, Tony Seale has earned the moniker "The Knowledge Graph Guy." Tony argues that enterprises that fail to grasp the urgent need to consolidate and understand their data will not survive the coming wave of more powerful AI.
Interview transcript
Larry:
Hi, everyone. Welcome to episode number 11 of the Knowledge Graph Insights podcast. I am really delighted today to welcome to the show Tony Seale. Tony is the Knowledge Graph Guy. That's how everybody knows him on LinkedIn, and I think he's earned that moniker. He also does a lot of consulting and work for big investment banks and things in the financial world. But welcome, Tony.
Paco Nathan
Graph RAG is all the rage right now in the AI world. Paco Nathan is uniquely positioned to help the industry understand and contextualize this new technology.
Paco currently leads a knowledge graph practice at an AI startup, and he has been immersed in the AI community for more than 40 years.
His broad and deep understanding of the tech and business terrain, along with his "graph thinking" approach, provides executives and other decision makers a clear view of terrain that is often obfuscated by less experienced and knowledgeable advisors.
We talked about:
his work building out the knowledge graph practice at Senzing, and their focus on entity resolution
the importance of entity resolution in knowledge graph use cases like fraud detection
the high percentage of knowledge graph projects that we never hear about because of their sensitive or proprietary nature
his take on the concept of "graph thinking" and how he and colleagues illustrate it with a simple graph model of a medieval village
how graphs add structure and context to our understanding of the world
the importance of embracing complexity and the Cynefin framework in which he grounds various types of business challenges: simple, complicated, complex, and chaotic
how to apply insights discerned from a Cynefin framing in management
how knowledge graphs can help oranizations understand the complex environments in which they operate
the wide range of industries and government entities that are applying knowledge graphs to concerns like supply chains, ESG, etc.
his overview of RAG - retrieval augmented generation and graph RAG
the wide variety of uses of the term "graph" in the current technology landscape
Microsoft's graph RAG which uses NetworkX inside their graph RAG library, not a graph database
Neo4j's approach which creates a "lexical graph" based an an NLP analysis of text
"embedding graphs"
ontology-based graphs
Google's approach to RAG, using graph neural networks
graphs that do reasoning over LLM-created facts assertions
"graph of thought" graphs based on chain-of-prompt thinking
"causal graphs" that permit causal reasoning
"graph analytics" graphs that re-rank possible answers
the evolution of graph RAG libraries and the variety of design patterns they employ
the shift in discovery dominance from search to recommender systems, most of which use knowledge graphs
examples of graph RAG from LlamaIndex and LangChain, in addition to Microsoft's graph RAG
his prediction that we'll see more reinforcement learning, graph tech, and advanced math capabilities like causality in addition to LLMs in AI systems
his reflection on his efforts to advance graph thinking over the past 4 years and the current state of LLMs, graphs, graph RAG, and the open-source software community
the need for a shift in thinking in the industry, in particular the need for cross-pollination across tech proficiencies and enterprise teams
the "10:1 ratio for the number of graph RAG experts versus the number of people we've actually worked with a library"
Paco's bio
Paco Nathan leads DevRel for the Entity Resolved Knowledge Graph practice area at Senzing.com and is a computer scientist with +40 years of tech industry experience and core expertise in data science, natural language, graph technologies, and cloud computing. He's the author of numerous books, videos, and tutorials about these topics.
Paco advises Kurve.ai, EmergentMethods.ai, KungFu.ai, DataSpartan, and Argilla.io (acq. Hugging Face), and is lead committer for the pytextrank and kglab open source projects. Formerly: Director of Learning Group at O'Reilly Media; and Director of Community Evangelism at Databricks.
Connect with Paco online
LinkedIn
Sessionize
Derwen.ai
Senzing.com
Resources mentioned in this interview
Connected Data London conference
Knowledge Graph Conference
Katariina Kari
For the past eight years, Katariina Kari has built knowledge graph teams at giant e-commerce companies like IKEA and Zalando. This practical, real-world experience puts her in an elite group of ontology and knowledge graph experts.
Knowledge graphs offer unique benefits to e-commerce merchants. From better product recommendations to more useful search results, the semantic capabilities that knowledge graphs provide routinely result in seven-figure sales increases.
The knowledge graphs that Katariina builds provide a semantic layer in the enterprise architecture that lets companies capture, use, and re-use the organization's unique domain knowledge in any number of applications.
Because knowledge graph isn't one application that does one thing. It's a paradigm shift in the way we work with data. It's a paradigm shift in the way we code, because now you don't need to put business logic into your code.
We talked about:
her work over the past eight years building knowledge graphs at companies like IKEA and Zalando
how using knowledge graphs to improve reccomendation and search routinely brings seven-figure business benefits
the set of skills and talents it takes to implement a knowledge graph project, most of which already exist in most companies
how LLMs and other AI tools can help transform structured or unstructured data into semantic data, a computable resource that captures business domain knowledge
some of the specific skills needed for KG work: ontology experts, back-end developers who understand the semantic web stack, data scientists and engineers, knowledge practitioners to capture domain knowledge, and product management
the need in each organization for a unique knowledge graph team tailored to the needs of the company and the talent available
the importance of user-centricity and use-case understanding in any knowledge graph project
the benefits of capturing business logic in a semantic layer which can be used and re-used in multiple applications
an interesting search-improvement use case that resulted in seven-figure sales increases, as well as experience-improving recommendation and info-box use cases
how capturing subject matter expertise in a knowlege graph can dramatically improve recommendation systems and deliver unexpected benefits to other
the importance of showing the benefits of knowledge graphs to organically advance enterprise adoption
her take on the difference between RDF-based knowledge graphs and labeled property graphs (LPGs) like Neo4j
the compelling case for knowledge graphs in e-commerce, which she has discovered in her eight years of practice
Katariina's bio
Katariina Kari is a leading expert in semantic web technologies, specializing in the development of ontologies and knowledge graphs. Over the past eight years, she has worked with prominent brands like IKEA and Zalando, building knowledge graphs that significantly enhance customer experiences by improving search functionalities and recommendations. Her extensive hands-on experience in creating enterprise knowledge graphs has established her as one of the global top talents in the field.
Katariina is frequently invited to speak at international events on the semantic web and knowledge graphs, sharing her insights and practical expertise with industry professionals. Her deep knowledge and passion for the semantic web have made her a sought-after keynote speaker and thought leader in the field.
Balancing a dual enthusiasm for technology and the arts, Katariina holds both a Master of Science degree and a Master of Music degree. From 2012 to 2016, she ran her own consultancy, where she worked closely with classical music organizations and artists, helping them navigate digital outreach. An art-loving and art-serving nerd, she seamlessly blends her love for music and technology in all her work, constantly pushing the boundaries of what’s possible in her field.
Vera Brozzoni
When you manage millions of digital assets, as the BBC does, you need robust metadata practices to organize and discover them.
Vera Brozzoni is a metadata manager at the BBC who focuses on classical music. She combines here academic background in music, philosophy, and the humanities with a rigorous metadata mind to help BBC systems - and ultimately viewers and listeners - discover and appreciate the music she loves so much.
We talked about:
her work as a metadata manager at the BBC and her distinctive background in classical music and philosophy and the humanities
how the complex history of music complicates her work
the role of taxonomy in her work
the meaning behind the famous quote, "Metadata is a love letter to the future"
how "music does whatever it wants" just as biological organisms don't always follow predictable rules
the importance of not being present-bound and imposing current biases on prior generations of music
her thoughts on the need for more practitioners with artistic cultural backgrounds to enter the field of metadata management
the diverse variety of intellectual talent at the BBC
how she sees her role as "bridging two completely different universes"
her thoughts on how AI could benefit her metadata work
her metadata outreach work into the music community
how to measure the effectiveness of a metadata program
her belief that the phantom of the French philosopher Blaise Pascal hovers over all cultural metadata work
Vera's bio
Vera Brozzoni was born in Italy where she studied Philosophy. She then moved to the UK where she studied History of Music and obtained a PhD in Composition at Newcastle University. She has worked in the music industry for many years, specialising in classical music metadata, devising innovative methods of schematising the history of music in all its complexities. Her aim is to evangelise metadata to classical music companies so that they can future-proof data coming from the past. Her other interests include AI, Machine Learning, cinema, literature.
Connect with Vera online
LinkedIn
Video
Here’s the video version of our conversation:
https://www.youtube.com/watch?v=0MKWKw0x7uc
Podcast intro transcript
This is the Knowledge Graph Insights podcast, episode number 8. Adjacent to the engineering and information practices that build the semantic infrastructure we operate in, is the crucial field of metadata management. Vera Brozzoni agrees with the internet archivist Jason Scott that "metadata is a love letter to the future." In her work at the BBC, Vera combines her deep academic background in music and the humanities with her metadata expertise to help listeners and viewers discover and appreciate classical music.
Interview transcript
Larry:
Hi everyone. Welcome to episode number eight of the Knowledge Graph Insights podcast. I am really delighted today to welcome to the show, Vera Brozzoni. Vera is a metadata manager at the BBC and based in the company in the UK. Welcome, Vera. Tell the folks a little bit more about your work at BBC and ...
Vera:
Hello Larry. So, yeah, as you rightly introduced me, Larry, I'm a metadata manager. My particularity is that I'm a classical music specialist and I come from a staunchly humanities background and not a tech or library science background like most of my colleagues basically.
Vera:
The fact of being an anomaly in this field, I'm trying to use it for the good of culture, for the good of the arts, and trying to convince the classical music world of the importance of metadata, which is great fun as you can imagine.
Larry:
That's funny. I hadn't really thought about that side of it 'cause I've thought more like you, we met in London at a semantic event and you fit right in. It's like you're clearly not having any trouble integrating into the tech world and the media world, but that's interesting. Tell me more about getting your classical music coll...
Teodora Petkova
Teodora Petkova is a scholar and content marketer whose PhD dissertation explored semantic technologies and dialogical theory and how they apply in the field of digital marketing communication.
She thoughtfully combines her rigorous academic thinking with pragmatic data- and knowledge-management practices in her content-marketing work.
She's currently building a knowledge graph with marketing content at Ontotext, the RDF database and data-management platform company.
We talked about:
her work building a knowledge graph of marketing content at Ontotext
her book "Being Dialogic" and the concept of dialogic communication
the importance of metadata in dialogic communication architectures
how creating a controlled vocabulary or an ontology can support a shared understanding of the concepts stakeholders and users work with
her overview of the semantic web
her current study with the 90-year-old marketing-education legend Philip Kotler
the connections she makes between corporate knowledge management and corporate marketing
the utility of enterprise-wide controlled vocabularies
how your content efforts can help you curate your knowledge graph
the urgent need to cultivate the social practices to help us generate and curate metadata
Teodora's bio
Teodora Petkova is a philologist fascinated by the metamorphoses of text on the Web and curious about the ways the Semantic Web unfolds. She holds a PhD. in Marketing Communication, an MS in Creative writing and a Bachelor of Science in Classics. Teodora is the author of the books The Brave New Text and Being Dialogic.
Following her genuine commitment to creating dialogic moments through semantic annotations, from 2022, Teodora is part of the Ontotext Knowledge Graph team. The Ontotext Knowledge Graph is where Teodora strives to harness the potential of the Semantic Web to foster dialogic marketing communications. Driven by the fascination with the ever-evolving nature of text on the Web, Teodora is also teaching web writing to students at the Content Strategy Masters program in FH Joanneum.
Connect with Teodora online
TeodoraPetkova.com
Teodora's newsletter
LinkedIn
Teodora's books
The Brave New Text
Being Dialogic
Resources mentioned in this interview
How to Do Things with Data, Klaus Bruhn Jensen
The Semantic Web, Tim Berners-Lee, James Hendler, and Ora Lassila
Marketing 4.0: Moving from Traditional to Digital, Philip Kotler
Video
Here’s the video version of our conversation:
https://www.youtube.com/watch?v=vF5xKzXxoWM
Podcast intro transcript
This is the Knowledge Graph Insights podcast, episode number 7. When you combine deep academic curiosity, a powerful concept like the semantic web, and an intellectually innovative approach to understanding how humans, computers, and corporate knowledge interact, you get Teodora Petkova's concept of "dialogic communication." Combine this powerful idea with a nuanced appreciation for modern marketing and carefully curated enterprise metadata and you can powerfully convey your organization's value to the world.
Interview transcript
Larry:
Hi everyone. Welcome to episode number seven of the Knowledge Graph Insights podcast. I am really delighted today to welcome to the show, Teodora Petkova. Teodora is just an ordinary content writer stumbled into the knowledge graph world. So welcome to the show, Teodora. Tell the folks a little bit more about what you are doing these days.
Teodora:
Hi, Larry. Thanks for having me. I'm doing what I have been dreaming about. I'm building a knowledge graph with Ontotext, out of marketing content, and I'm doing a lot of fights, a lot of things that I imagined and envisioned, but they need to be translated into specifications and requirements. So I now have a lot of time to write, do content writing per se; however, I write in the book of life.
Larry:
Yeah,
Dean Allemang
Dean Allemang literally wrote the book on the semantic web. "Semantic Web for the Working Ontologist" is now in its third edition.
In the book, Dean and his co-authors, James Hendler and Fabien Gandon, show how to apply web standards to build a meaningful web of global, connected knowledge.
More recently, Dean has conducted research with his colleagues at data.world that shows how using knowledge graphs can triple the accuracy of LLM-based question-answering systems.
We talked about:
his role as a principal solutions architect at data.world
the meaning of the "semantic web" and its intent of sharing meaning across the web
the long history of knowledge representation and how the connectedness of the semantic web adds to it
the crucial difference between documents about things and the strings that describe them
the contrast between the persistent nature of enterprise data and the ephemerality of the applications that use the data
the power of the simple structure of RDF, its mathematical affordances, and the ease of distribution it permits
the impact of newer AI tech on knowledge graph building and querying
the research that he and Juan Sequeda have conducted that shows how using knowledge graphs can triple the accuracy of LLM-based question-answering systems
his thoughts on the yet-to-be-resolved one-way or two-way ontology question
the crucial role of trust in AI and how replacing LLMs with knowledge graphs as the point of contact in AI systems could build more trust
Dean's bio
Dean Allemang has been active in the field of Artificial Intelligence (AI) since the 1980s. With a notable emphasis on Semantic Web, he is the author of the book "Semantic Web for the Working Ontologist." His passion for understanding and implementing knowledge graphs led to a significant publication about using LLMs to answer queries over structured data, which introduced a new benchmark for evaluation.
In his current role as a Principal Solutions Architect at data.world, he contributes extensively to the development of the AI Context Engine product, which is inspired by his recent research (with Juan Sequeda and Bryon Jacob), and underscores his commitment to practical application of theoretical principles.
For a span of about a decode, Dean operated as an independent consultant, utilizing knowledge graph solutions to address challenges in industries such as Media, Finance, and Life Sciences. This diverse experience has cultivated a broad perspective on applying AI and Semantic Web principles.
Influenced by Sir Tim Berners-Lee's concept of linked data and data sharing, Dean Allemang's work reflects a consistent focus on these principles. His contributions have advanced the field of AI and his current interest lies in how knowledge graphs can make generative AI more effective.
Connect with Dean online
LinkedIn
Medium
Resources mentioned in this interview
Semantic Web for the Working Ontologist
A Benchmark to Understand the Role of Knowledge Graphs on Large Language Model's Accuracy for Question Answering on Enterprise SQL Databases, Juan Sequeda, Dean Allemang, Bryon Jacob
The Semantic Web, Tim Berners-Lee, James Hendler, and Ora Lassila
Video
Here’s the video version of our conversation:
https://youtu.be/29kmAc6tobU
Podcast intro transcript
This is the Knowledge Graph Insights podcast, episode number 6. Long before the introduction of the semantic web - the innovation that added meaning and metadata to documents on the web - AI pioneers like Dean Allemang had been thinking about how knowledge could be formalized to help people do their work. The web itself, along with the W3C standards that power its semantic capabilities, gave Dean and his peers the ability to scale and connect existing practices and technologies to build a more meaningful web.
Interview transcript
Larry:
Things. Hi everyone. Welcome to episode number six of the Knowledge Grap...
Alan Morrison
After 20-plus years of industry analysis, Alan Morrison has developed a keen sense for how knowledge graphs can help enterprises.
Even though he has focused on advanced tech and emerging IT practices and is deeply immersed and invested in current tech developments, much of his advice for enterprises looking to develop their data maturity involves pragmatic baby steps and basic mindset shifts.
We talked about:
his work in the consulting world and his organizing work around the knowledge graph community to improve awareness of the technology
the need to find "foxes instead of the hedgehogs" in enterprises when you're trying to promote adoption of new tech
the relationships between different AI tech, like LLMs and knowledge graphs, and the common connection they share: data
the importance of having mature data practices in any enterprise
how even simple metadata practices in common tools like spreadsheets can support better enterprise data practices
how sidestepping the formal org chart and forming guerrilla teams can advance data practice
the benefits of starting small in any knowledge graph project
how representing organization knowledge at a high level in a knowledge graph can help solve big enterprise problems
how a knowledge graph gives you a multidimensional Tinker Toys set to model and understand your org's data
the benefits of moving from tabular thinking to graph thinking
his frustration with the current framing of AI as being solely about machine learning
his observation that practices across any org - content, knowledge management, data management, business people - could benefit from long-standing standards and proven technologies (that might not be as sexy and topical as LLMs)
Alan's bio
Alan Morrison is a longtime analyst, writer, advisor and podcaster on advanced data technologies and emerging IT. For 20 years at PwC's R&D and innovation think tanks, Alan identified emerging technologies on the cusp of adoption, assessed their business impacts, and advised PwC's clients on innovation strategy.
Before PwC, he was a semiconductor industry market analyst and forecaster, a retail site location analyst, and a US Navy intelligence analyst, Russian linguist and aircrewman.
For the last five years, Alan has been a contributor on knowledge graph and related topics for Data Science Central. His writings over the years have covered dozens of different technologies.
Connect with Alan online
LinkedIn
Video
Here’s the video version of our conversation:
https://youtu.be/TXOWWjM-DBc
Podcast intro transcript
This is the Knowledge Graph Insights podcast, episode number 5. You might think that the lofty perch of multiple decades in industry-analyst roles would inspire grand visions of tech transformation with leading-edge technology. Quite the opposite in the case Alan Morrison. He shows how enterprises can advance their data maturity by cultivating basic graph thinking in their organizations and by taking small, pragmatic steps like adopting established standards for interoperability or simply adding metadata to a spreadsheet.
Interview transcript
Larry:
Hi, everyone. Welcome to episode number five of the Knowledge Graph Insights podcast. I am really happy today to welcome to the show Alan Morrison. Currently, he's a contributor at Data Science Central, a well-known publication in the field. He's a freelancer and consultant around knowledge graphs and a lot of other areas as well. His background, he comes out of the consulting world. Most recently before his current role as a freelancer and consultant, he worked for many years at PriceWaterhouseCooper, the big consultancy as a senior research fellow. So welcome, Alan. Tell the folks a little bit more about what you're up to these days.
Alan:
Hey, Larry. Great to talk with you and folks should know that you and I have some history together as a part of the Data Worthy Collective,
Ellie Young
Ellie Young effortlessly connects the human and technical elements that go into ontologies and knowledge graph building.
Ellie came to the world of knowledge graphs with backgrounds in both literature and sustainability. "If the world wasn't on fire," she says, " I would probably be writing novels."
That sense of urgency drives her work at Common Action, a platform she is creating to address climate change and advance sustainability. She also applies her knowledge graph expertise in projects like HelioWeb at NASA, which connects scientists in the field of heliophysics.
We talked about:
her work at Common Action, a platform for climate and for sustainability that uses knowledge graph technology
her work at NASA to facilitate collaboration and expose knowledge across the domain of heliophysics (the study of the sun)
how personal knowledge graphs can connect individuals and collectives of people
how her background in design, art,literature, and the humanities manifests in her knowledge graph work
her desire to leverage metadata and capabilities like her language knowledge to facilitate topical discovery
the interplay between the efficiencies that AI tech like LLMs offer and the uniquely imaginative variations that human beings create
the importance of the practice of design in advancing the productive use of information
how user experience design connects to ontologies and back-end tech
how she applies, and imagines how others might apply, a literary mindset to ontology practice
how she applied ethnographic methods from anthropology to a paper she co-authored on the NASA HelioWeb ontology
her ongoing call for volunteers to help with her Common Action program, specifically a current need for creating a "phenomena ontology"
Ellie's bio
Ellie Young brings knowledge to communities to catalyze successful, local actions to address climate/sustainability problems.
She is the founder of Common Action, an innovation network facilitating climate and sustainability action through the development of community and knowledge graph technology.
Previously she served as Head of Community and Director of Conference Operations at The Knowledge Graph Conference.
Connect with Ellie online
LinkedIn
ellie at common-action dot org
Resources
The cultural-social nucleus of an open community: A multi-level community knowledge graph and NASA application, Applied Computing and Geosciences
Common Action vision
Video
Here’s the video version of our conversation:
https://youtu.be/CL9HWocoh7I
Podcast intro transcript
This is the Knowledge Graph Insights podcast, episode number 4. In the domains of ontology engineering and the semantic web, there are plenty of people with advanced technical skills. Practitioners with social-science skills, well-developed literary instincts, and a design mindset are harder to find. Ellie Young smoothly navigates the technical and linguistic worlds that intersect in knowledge graphs, applying her humanities mindset to projects that connect scientists at NASA and address climate change and sustainability.
Interview transcript
Larry:
Okay. Hi everyone. Welcome to episode number four of the Knowledge Graph Insights podcast. I am super delighted today to welcome to the show Ellie Young. Ellie is the founder of Common Action, a sustainability and climate change activist organization. She's also ... The way I first met her, she's the former head of community for the Knowledge Graph Conference, and one of the people who really ushered a lot of people into this community. So welcome Ellie. Tell the folks a little bit more about what you're up to these days.
Ellie:
Thanks, Larry, and thank you for inviting me to be a special guest with you on this very nice community visit. So yeah. Now I've gone from KGC. I think I left about three years ago to starting Common Action, which is not really an activist organization.
George Anadiotis
Every profession has its connectors, sharers, and community organizers. In the knowledge graph world, George Anadiotis fills all of these roles.
Through his industry analysis and reporting, his conference organizing, and his writing and podcasting, George connects ideas and people across the semantic-tech landscape.
We talked about:
his work at Linked Data Orchestration and as a consultant and analyst in the knowledge graph and linked-data world
his diverse background in computing and his studies at the intersection of knowledge management, the semantic web, and distributed systems
his extensive writing experience and consulting background
his definition of a knowledge graph
the differences between RDF-based knowledge graphs and labeled property graphs (LPG)
the focus in the RDF community on standards and interoperability versus the focus in the LPG community on implementation
the variety of query languages in the LPG world and recent efforts like GQL to create a standard way of querying LPGs, as well as efforts to query across both RDF and LPG graphs
the origins of his annual Year of the Graph report
some of the reasons that knowledge graphs are positioned in the bullseye of Gartner's Impact Radar this year
where knowledge graphs fit in the AI landscape
the role of knowledge graphs in RAG architectures
the conference he organizes, Connected Data London, coming up December 11-13
George's bio
George Anadiotis has got tech, data, AI and media, and he's not afraid to use them.
He helps organizations map and understand complex domains to make better decisions; design, implement and monitor models, processes and systems to achieve goals; and craft communication strategies and outreach initiatives to grow awareness and market share.
He enjoys researching, developing, applying, writing and talking about cutting edge concepts and technology, and their implications on society and business.
Connect with George online
LinkedIn
Twitter
TikTok
Instagram
George's publications, podcasts, and conference
Connected Data London (conference roundtable recording)
The Year of the Graph
Orchestrate All the Things
Video
Here’s the video version of our conversation:
https://youtu.be/lEHj5_9y-30
Podcast intro transcript
This is the Knowledge Graph Insights podcast, episode number 3. In any domain, there are people who seem to do it all - practice and consultation, industry analysis and reporting, and community building and event organizing. In the world of knowledge graphs and the semantic web, George Anadiotis has filled all of these roles. Whether he's publishing his Year of the Graph newsletter, organizing the annual Connected Data conference, or producing the latest Orchestrate All the Things podcast, George is always connecting the dots.
Interview transcript
Larry:
Hi, everyone. Welcome to episode number three of the Knowledge Graph Insights Contest. Sorry, I'm going to redo that again. I have too many podcasts. I need a new intro for this one. Okay. Hi, everyone. Welcome to episode number three of the Knowledge Graph Insights podcast. I am really delighted today to welcome to the show George Anadiotis. George is really well known in the knowledge graph world and the graph world in general and the tech world in general, as an analyst, a consultant, a really well-developed engineer. He runs a big conference around knowledge graph and graph technology, and he is the principal at his organization called Linked Data Orchestration. So welcome, George, tell the folks a little bit more about what you're up to these days.
George:
Great. Thanks for the intro, Larry, and good to be here. Actually one of the opening, I guess, guests for this new podcast series of yours. Well, the truth is I have a long and kind of convoluted story, but I've kind of honed my skills of telling it in as simple way as possible.
Mike Dillinger
Knowledge graphs provide the digital foundation for some of most visible companies on the web.
Mike Dillinger built LinkedIn's Economic Graph, the knowledge graph that powers the social media giant's recommendation systems.
Mike now helps people understand knowledge graph technology and how it can complement and improve generative AI, whether by acting as "jet fuel" to better train LLMs or by providing "adult supervision" for their unruly, adolescent behavior.
We talked about:
how he describes knowledge graphs
how the richness of information in a knowledge graph helps computers better understand the things in a system
the differences between knowledge graphs and LLMs
how LinkedIn's Economic Graph, which Mike's team built, works
how LLMs can help build knowledge graphs, and how knowledge graphs can act as "jet fuel" to train LLMs
the RDF "triples" that are at the foundation of knowledge graphs
the importance of distinguishing between unique concepts in a knowledge graph and how practitioners do this
the two main crafts needed to build knowledge graphs: linguistic expertise and software engineering
the job opportunities for language professionals in the LLM and knowledge graph worlds
the propensity of tech companies to staff knowledge graph efforts with engineers while there is actually a need for a variety of talent, as well as better collaboration skills
his assertion that "language professionals aren't janitors," put on teams only to clean up data for software engineers
how knowledge graphs provide "adult supervision" for unruly, adolescent LLMs
his hypothesis that using KGs as a separate modality of data rather than as training data for LLMs will advance AI
Mike's bio
Mike Dillinger, PhD is a technical advisor, consultant, and thought leader who champions the importance of capturing and leveraging reusable, explicit human knowledge to enable more reliable machine intelligence. He was Technical Lead for Knowledge Graphs in the AI Division at LinkedIn and for LinkedIn’s and eBay’s first machine translation systems. He was also an independent consultant specialized in deploying translation technologies for Fortune 500 companies, and Director of Linguistics at two machine translation software companies where he led development of the first commercial MT-TM integration. He was President of the Association for Machine Translation in the Americas and has two MT-related patents. Dr. Dillinger has also taught at more than a dozen universities in several countries, has been a visiting researcher on four continents, and has a weekly blog on Knowledge Architecture.
Connect with Mike online
LinkedIn
Video
Here’s the video version of our conversation:
https://youtu.be/wX2C3DwiWG4
Podcast intro transcript
This is the Knowledge Graph Insights podcast, episode number 2. If you've ever looked for a job or recruited talent on LinkedIn, you've seen Mike Dillinger's work. His team built LinkedIn's Economic Graph, the knowledge graph that powers the social media platform's recommendation system. These days, Mike thinks a lot about how knowledge graph technology can work with generative AI, seeing opportunities for the technologies to help the other, like the ability of knowledge graphs to act as "jet fuel" to train large language models.
Interview transcript
Larry:
Hi everyone. Welcome to Episode Number 2 of the Knowledge Graph Insights podcast. I am really delighted today to welcome to the program Mike Dillinger. Mike is a cage-free consultant based in San Jose. He's been doing knowledge graph and other technical things for many years. So welcome, Mike. Tell the folks a little bit more about what you're up to these days.
Mike:
Thanks a lot, Larry. What am I up to? I'm trying to help people wrap their heads around knowledge graphs and how we can make AI, transform GenAI into Next-GenAI by leveraging more explicit content and human knowledge.
François Scharffe and Thomas Deely
The Knowledge Graph Conference is one of the premier events in the semantic technology space.
François Scharffe and Thomas Deeley started the conference to bridge the gap between academic researchers and industry practitioners. The community they have built around the conference and the conference programming - a mix of workshops, classes, presentations, and demos - reflect this purpose.
They also intend to democratize knowledge graph use, and toward that end are participating in a number of efforts to develop education programs - both professional certifications and academic curricula.
We talked about:
the origins of the Knowledge Graph Conference, and its balanced inclusion of both academic and industry entities
how generative AI is propelling interest in knowledge graphs
their mission to broaden awareness of knowledge graph technology and practice
the importance of education and the need for a practical approach
the origins of the Open Knowledge Network, part of the National Science Foundation's Proto-Open Knowledge Network program, and KGC's role in it
their ambition to build an educational institute around KG technology
how to help enterprise executives understand the benefits of knowledge graphs
how modeling an enterprise's knowledge and capturing it in a knowledge graph can help organizations address complex challenges
the advantages of knowledge graphs over LLMs and GenAI, which have yet to prove their reliability
how LLMs can assist in the construction and use of knowledge graphs
how study of the human brain illustrates how GenAI and KGs can work together
the community that has arisen around the Knowledge Graph Conference
François' bio
François Scharffe is a hands-on technology executive with a track record of improving decision making in complex data environments. His career as a technical leader and entrepreneur has led him to perform engineering, product management and leadership roles in various organizations.
François has also worked as a lecturer and researcher, most recently at Columbia University (New York) and at the University of Montpellier (France). François is the founder and chief executive of The Data Chefs, a data management consulting firm, and the founder of the Knowledge Graph Conference, the leading event on knowledge-centric AI technologies.
Thomas' bio
Thomas Deely is co-founder of The Knowledge Graph Conference and Community, a global community bridging research and industry on Knowledge Graphs, AI, and related technologies. Thomas is also the Customer Community Manager at Box, the leading cloud content management platform.
Thomas started his career as an engineer at JPMorgan in London, before joining Goldman Sachs where he advanced to become a senior engineer in the NY office. Thomas launched an Applied Analytics program and executive education initiatives at Columbia University, before venturing into the customer experience, product, and community domain at companies such as Unqork, where he launched the Community, and Stack Overflow, where he helped grow and develop the StackOverflow for Teams product and business, as part of the customer success team, before joining Box.
Thomas has an electronic engineering undergraduate degree from University College, Dublin, and a Masters in Science in Technology Management from Columbia University, and lives in NY where he is married with two children.
Connect with François and Thomas online
François at LinkedIn
Thomas at LinkedIn
Video
Here’s the video version of our conversation:
https://youtu.be/gjtoPXY3Ka8
Podcast intro transcript
This is the Knowledge Graph Insights podcast, episode number 1. I'm really happy to launch this new podcast with a conversation with François Scharffe and Thomas Deeley, the founders of The Knowledge Graph Conference. Their annual gathering in New York City attracts knowledge graph practitioners,
Comments
Top Podcasts
The Best New Comedy Podcast Right Now – June 2024The Best News Podcast Right Now – June 2024The Best New Business Podcast Right Now – June 2024The Best New Sports Podcast Right Now – June 2024The Best New True Crime Podcast Right Now – June 2024The Best New Joe Rogan Experience Podcast Right Now – June 20The Best New Dan Bongino Show Podcast Right Now – June 20The Best New Mark Levin Podcast – June 2024