Discover
MLOps.community
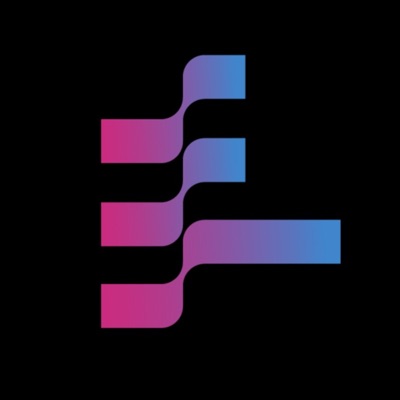
328 Episodes
Reverse
Sol Rashidi is an esteemed executive, leader, and influencer within the AI, Data, and Technology space. Having helped IBM launch Watson in 2011 as one of the earliest world applications of Artificial Intelligence, Sol has pioneered some of the early advancements of space.
Join us at our first in-person conference on June 25 all about AI Quality: https://www.aiqualityconference.com/
Huge thank you to @WeightsBiases for sponsoring this episode. WandB Free Courses - http://wandb.me/courses_mlops
MLOps podcast #227 with Sol Rashidi, CEO & Co-Founder of ExecutiveAI, Leading Enterprise Data Teams.
// Abstract
In the dynamic landscape of MLOps and data leadership, Sol shares invaluable insights on building successful teams and driving impactful projects. In this podcast episode, Sol delves into the importance of prioritizing relationships, introduces a pragmatic "Wrong Use Cases Formula" to streamline project prioritization, and emphasizes the critical role of effective communication in data leadership. Her wealth of experience and practical advice provide a roadmap for navigating the complexities of MLOps and leading data-driven initiatives to success.
// Bio
With eight (8) patents granted, 21 filed, and received awards that include:
"Top 100 AI People" 2023
"The Top 75 Innovators of 2023"
"Top 65 Most Influential Women in 2023"
"Forbes AI Maverick of the 21st Century" 2022
“Top 10 Global Women in AI & Data”, 2023
"Top AI 100 Award", 2023
“50 Most Powerful Women in Tech”, 2022
“Global 100 Power List” - 2021, 2022, 2023
“Top 20 CDOs Globally” - 2022
"Chief Analytics Officer of the Year" - 2022
"Isomer Innovators of the Year" - 2021, 2022, 2023
"Top 100 Innovators in Data & Analytics” - 2020, 2021, 2022, 2023
"Top 100 Women in Business" - 2022
Sol is an energetic business executive and a goal-oriented technologist, skilled at coupling her technical acumen with story-telling abilities to articulate business value with both startups and Fortune 100's who are leaning into data, AI, and technology as a competitive advantage while wanting to preserve the legacy in which they were founded upon. Sol has served as a C-Suite member across several Fortune 100 & Fortune 500 companies including:
Chief Analytics Officer - Estee Lauder
Chief Data & Analytics Officer - Merck Pharmaceuticals
EVP, Chief Data Officer - Sony Music
Chief Data & AI Officer - Royal Caribbean Cruise Lines
Sr. Partner leading the Digital & Innovation Practice- Ernsty & Young
Partner leading Watson Go-To-Market & Commercialization - IBM
Sol now serves as the CEO of ExecutiveAI LLC. A company dedicated to democratizing Artificial Intelligence for Humanity and is considered an outstanding and influential business leader who is influencing the space traveling the world as a keynote speaker, and serving as the bridge between established Gen1.0 markets and those evolving into 4.0.
// MLOps Jobs board
https://mlops.pallet.xyz/jobs
// MLOps Swag/Merch
https://mlops-community.myshopify.com/
// Related Links
Sol's Book will be out on April 30, 2024
Your AI Survival Guide: Scraped Knees, Bruised Elbows, and Lessons Learned from Real-World AI Deployments: https://www.amazon.com/Your-Survival-Guide-Real-World-Deployments/dp/1394272634?ref_=ast_author_mpb
--------------- ✌️Connect With Us ✌️ -------------
Join our slack community: https://go.mlops.community/slack
Follow us on Twitter: @mlopscommunity
Sign up for the next meetup: https://go.mlops.community/register
Catch all episodes, blogs, newsletters, and more: https://mlops.community/
Connect with Demetrios on LinkedIn: https://www.linkedin.com/in/dpbrinkm/
Connect with Sol on LinkedIn: https://www.linkedin.com/in/sol-rashidi-a672291/
Join us at our first in-person conference on June 25 all about AI Quality: https://www.aiqualityconference.com/
Chad Sanderson is passionate about data quality, and fixing the muddy relationship between data producers and consumers. He is a former Head of Data at Convoy, a LinkedIn writer, and a published author. He lives in Seattle, Washington, and is the Chief Operator of the Data Quality Camp.
Huge thank you to @amazonwebservices for sponsoring this episode. AWS - https://aws.amazon.com/
MLOps podcast #226 with Chad Sanderson, CEO & Co-Founder of Gable, The Rise of Modern Data Management.
// Abstract
In this session, Chad Sanderson, CEO of Gable.ai and author of the upcoming O’Reilly book: "Data Contracts," tackles the necessity of modern data management in an age of hyper iteration, experimentation, and AI. He will explore why traditional data management practices fail and how the cloud has fundamentally changed data development. The talk will cover a modern application of data management best practices, including data change detection, data contracts, observability, and CI/CD tests, and outline the roles of data producers and consumers. Attendees will leave with a clear understanding of modern data management's components and how to leverage them for better data handling and decision-making.
// Bio
Chad Sanderson, CEO of Gable.ai, is a prominent figure in the data tech industry, having held key data positions at leading companies such as Convoy, Microsoft, Sephora, Subway, and Oracle. He is also the author of the upcoming O'Reilly book, "Data Contracts” and writes about the future of data infrastructure, modeling, and contracts in his newsletter “Data Products.”
// MLOps Jobs board
https://mlops.pallet.xyz/jobs
// MLOps Swag/Merch
https://mlops-community.myshopify.com/
// Related Links
AWS Trainium and Inferentia:
https://aws.amazon.com/machine-learning/trainium/
https://aws.amazon.com/machine-learning/inferentia/
--------------- ✌️Connect With Us ✌️ -------------
Join our slack community: https://go.mlops.community/slack
Follow us on Twitter: @mlopscommunity
Sign up for the next meetup: https://go.mlops.community/register
Catch all episodes, blogs, newsletters, and more: https://mlops.community/
Connect with Demetrios on LinkedIn: https://www.linkedin.com/in/dpbrinkm/
Connect with Chad on LinkedIn: https://www.linkedin.com/in/chad-sanderson/
Patrick Beukema has a Ph.D. in neuroscience and has worked on AI models for brain decoding, which analyzes the brain's activity to decipher what people are seeing and thinking.
Join us at our first in-person conference on June 25 all about AI Quality: https://www.aiqualityconference.com/
Huge thank you to LatticeFlow for sponsoring this episode. LatticeFlow - https://latticeflow.ai/
MLOps podcast #225 with Patrick Beukema, Head / Technical Lead of the Environmental AI, Applied Science Organization at AI2, Beyond AGI, Can AI Help Save the Planet?
// Abstract
AI will play a central role in solving some of our greatest environmental challenges. The technology that we need to solve these problems is in a nascent stage -- we are just getting started. For example, the combination of remote sensing (satellites) and high-performance AI operating at a global scale in real-time unlocks unprecedented avenues to new intelligence.
MLOPs is often overlooked on AI teams, and typically there is a lot of friction in integrating software engineering best practices into the ML/AI workflow. However, performance ML/AI depends on extremely tight feedback loops from the user back to the model that enables high iteration velocity and ultimately continual improvement.
We are making progress but environmental causes need your help. Join us fight for sustainability and conservation.
// Bio
Patrick is a machine learning engineer and scientist with a deep passion for leveraging artificial intelligence for social good. He currently leads the environmental AI team at the Allen Institute for Artificial Intelligence (AI2). His professional interests extend to enhancing scientific rigor in academia, where he is a strong advocate for the integration of professional software engineering practices to ensure reliability and reproducibility in academic research. Patrick holds a Ph.D. from the Center for Neuroscience at the University of Pittsburgh and the Center for the Neural Basis of Cognition at Carnegie Mellon University, where his research focused on neural plasticity and accelerated learning. He applied this expertise to develop state-of-the-art deep learning models for brain decoding of patient populations at a startup, later acquired by BlackRock. His earlier academic work spanned research on recurrent neural networks, causal inference, and ecology and biodiversity.
// MLOps Jobs board
https://mlops.pallet.xyz/jobs
// MLOps Swag/Merch
https://mlops-community.myshopify.com/
// Related Links
Variety of relevant papers/talks/links on Patrick's website: https://pbeukema.github.io/
--------------- ✌️Connect With Us ✌️ -------------
Join our slack community: https://go.mlops.community/slack
Follow us on Twitter: @mlopscommunity
Sign up for the next meetup: https://go.mlops.community/register
Catch all episodes, blogs, newsletters, and more: https://mlops.community/
Connect with Demetrios on LinkedIn: https://www.linkedin.com/in/dpbrinkm/
Connect with Patrick on LinkedIn: https://www.linkedin.com/in/plbeukema/
Timestamps:
[00:00] AI Quality Conference
[01:29] Patrick's preferred coffee
[02:00] Takeaways
[04:14] Learning how to learn journey
[07:04] Patrick's day to day
[08:39] Environmental AI
[11:07] Environmental AI models
[14:35] Nature Inspires Scientific Advances
[18:11] R&D
[24:58] Iterative Feedback-Driven Development
[26:37 - 28:07] LatticeFlow Ad
[33:58] Balancing Metrics for Success
[38:16] Model Retraining Pipeline
[44:11] Series Models: Versatility
[45:57] Edge Models Enhance Output
[50:22] Custom Models for Specific Data
[53:53] Wrap up
Join us at our first in-person conference on June 25 all about AI Quality: https://www.aiqualityconference.com/
Verena Weber believes that GenAI is going to transform the way we work and interact with devices. Her mission is to help companies prepare for this transformation. She has strong expertise in NLP and over 7 years of experience in Machine Learning.
Huge thank you to @zilliz for sponsoring this episode. Zilliz - https://zilliz.com/
MLOps podcast #224 with Verena Weber, Generative AI Consultant at Verena Weber, GenAI in Production - Challenges and Trends.
// Abstract
The goal of this talk is to provide insights into challenges for Generative AI in production as well as trends aiming to solve some of these challenges. The challenges and trends Verena see are:
Model size and moving towards mixture of experts architectures
context window - new breakthroughs for context lengths
from unimodality to multimodality, next step large action models?
regulation in form of the EU AI Act
Verena uses the differences between Gemini 1.0 and Gemini 1.5 to exemplify some of these trends.
// Bio
Verena leverages GenAI in natural language to elevate business competitiveness and navigate its transformative impact. Her varied experience in multiple roles and sectors underpins her ability to extract business value from AI, blending deep technical expertise with strong business acumen. Post-graduation, she consulted in Data Science at Deloitte and then advanced her skills in NLP, Deep Learning, and GenAI as a Research Scientist at Alexa team, Amazon. Passionate about gender diversity in tech, she mentors women to thrive in this field.
// MLOps Jobs board
https://mlops.pallet.xyz/jobs
// MLOps Swag/Merch
https://mlops-community.myshopify.com/
// Related Links
Website: verenaweber.de
Sign up for Verena's newsletter: https://verenas-newsletter-63558b.beehiiv.com/
Zilliz - https://zilliz.com/
--------------- ✌️Connect With Us ✌️ -------------
Join our slack community: https://go.mlops.community/slack
Follow us on Twitter: @mlopscommunity
Sign up for the next meetup: https://go.mlops.community/register
Catch all episodes, blogs, newsletters, and more: https://mlops.community/
Connect with Demetrios on LinkedIn: https://www.linkedin.com/in/dpbrinkm/
Connect with Verena on LinkedIn: https://www.linkedin.com/in/verena-weber-134178b9/
Timestamps:
[00:00] AI Quality Conference
[01:33] Verena's preferred coffee
[02:15] Takeaways
[06:33] Ski Person of Influence
[11:31] Verena's background in the last 5-10 years
[14:24] Tech Evolution: Rapid Transformation
[18:13] Working at Amazon and key challenges
[20:10] Research-inspired suggestions
[22:21] AI Updates Impact Workflows
[22:52] Alexa Query Distribution Analysis
[24:06] Innovative Solutions for Alexa
[25:27] Robust T5 Data Prompting
[27:38] Audio Data Quality Challenges
[28:21-29:28] Zilliz ad
[29:28] Alexa data transcription and data cleaning
[35:38] Considering needs, costs, and complexity
[37:44] ChatGPt is not ideal for classification
[39:32] Comparison of model building using TF, IDF
[45:08] Struggle to boost diversity in conference speakers
[47:30] Creating safe environments helps underrepresented individuals participate
[48:29] Wrap up
Join us at our first in-person conference on June 25 all about AI Quality: https://www.aiqualityconference.com/
MLOps Coffee Sessions Special episode with Databricks, Introducing DBRX: The Future of Language Models, fueled by our Premium Brand Partner, Databricks.
DBRX is designed to be especially capable of a wide range of tasks and outperforms other open LLMs on standard benchmarks. It also promises to excel at code and math problems, areas where others have struggled.
Our panel of experts will get into the technical nuances, potential applications, and implications of DBRx for businesses, developers, and the broader tech community.
This session is a great opportunity to hear from insiders about how DBRX's capabilities can benefit you.
// Bio
Denny Lee - Co-host
Denny Lee is a long-time Apache Spark™ and MLflow contributor, Delta Lake maintainer, and a Sr. Staff Developer Advocate at Databricks. A hands-on distributed systems and data sciences engineer with extensive experience developing internet-scale data platforms and predictive analytics systems. He has previously built enterprise DW/BI and big data systems at Microsoft, including Azure Cosmos DB, Project Isotope (HDInsight), and SQL Server.
Davis Blalock
Davis Blalock is a research scientist and the first employee at MosaicML. He previously worked at PocketSonics (acquired 2013) and completed his PhD at MIT, where he was advised by John Guttag. He received his M.S. from MIT and his B.S. from the University of Virginia. He is a Qualcomm Innovation Fellow, NSF Graduate Research Fellow, and Barry M. Goldwater Scholar. He is also the author of Davis Summarizes Papers, one of the most widely-read machine learning newsletters.
Bandish Shah
Bandish Shah is an Engineering Manager at MosaicML/Databricks, where he focuses on making generative AI training and inference efficient, fast, and accessible by bridging the gap between deep learning, large-scale distributed systems, and performance computing. Bandish has over a decade of experience building systems for machine learning and enterprise applications. Prior to MosaicML, Bandish held engineering and development roles at SambaNova Systems where he helped develop and ship the first RDU systems from the ground up, and Oracle where he worked as an ASIC engineer for SPARC-based enterprise servers.
Abhi Venigalla
Abhi is an NLP architect working on helping organizations build their own LLMs using Databricks. Joined as part of the MosaicML team and used to work as a researcher at Cerebras Systems.
Ajay Saini
Ajay is an engineering manager at Databricks leading the GenAI training platform team. He was one of the early engineers at MosaicML (acquired by Databricks) where he first helped build and launch Composer (an open source deep learning training framework) and afterwards led the development of the MosaicML training platform which enabled customers to train models (such as LLMs) from scratch on their own datasets at scale. Prior to MosaicML, Ajay was co-founder and CEO of Overfit, an online personal training startup (YC S20). Before that, Ajay worked on ML solutions for ransomware detection and data governance at Rubrik. Ajay has both a B.S. and MEng in computer science with a concentration in AI from MIT.
// MLOps Jobs board
https://mlops.pallet.xyz/jobs
// MLOps Swag/Merch
https://mlops-community.myshopify.com/
// Related Links
Website: https://www.databricks.com/
Databricks DBRX: https://www.databricks.com/blog/introducing-dbrx-new-state-art-open-llm
--------------- ✌️Connect With Us ✌️ -------------
Join our slack community: https://go.mlops.community/slack
Follow us on Twitter: @mlopscommunity
Sign up for the next meetup: https://go.mlops.community/register
Catch all episodes, blogs, newsletters, and more: https://mlops.community/
Join us at our first in-person conference on June 25 all about AI Quality: https://www.aiqualityconference.com/
// Abstract
Dive into the challenges of scaling AI models from Minimum Viable Product (MVP) to full production. The panel emphasizes the importance of continually updating knowledge and data, citing examples like teaching AI systems nuanced concepts and handling brand name translations.
User feedback's role in model training, alongside evaluation steps like human annotation and heuristic-based assessment, was highlighted.
The speakers stressed the necessity of tooling for user evaluation, version control, and regular performance updates. Insights on in-house and external tools for annotation and evaluation were shared, providing a comprehensive view of the complexities involved in scaling AI models.
// Bio
Alex Volkov - Moderator
Alex Volkov is an AI Evangelist at Weights & Biases, celebrated for his expertise in clarifying the complexities of AI and advocating for its beneficial uses. He is the founder and host of ThursdAI, a weekly newsletter, and podcast that explores the latest in AI, its practical applications, open-source, and innovation. With a solid foundation as an AI startup founder and 20 years in full-stack software engineering, Alex offers a deep well of experience and insight into AI innovation.
Eric Peter
Product management leader and 2x founder with experience in enterprise products, data, and machine learning. Currently building tools for generative AI @Databricks.
Donné Stevenson
Focused on building AI-powered products that give companies the tools and expertise needed to harness to power of AI in their respective fields.
Phillip Carter
Phillip is on the product team at Honeycomb where he works on a bunch of different developer tooling things. He's an OpenTelemetry maintainer -- chances are if you've read the docs to learn how to use OTel, you've read his words. He's also Honeycomb's (accidental) prompt engineering expert by virtue of building and shipping products that use LLMs. In a past life, he worked on developer tools at Microsoft, helping bring the first cross-platform version of .NET into the world and grow to 5 million active developers. When not doing computer stuff, you'll find Phillip in the mountains riding a snowboard or backpacking in the Cascades.
Andrew Hoh
Andrew Hoh is the President and Co-Founder of LastMile AI. Previously, he was a Group PM Manager at Meta AI, driving product for their AI Platform. Previously, he was the Product Manager for the Machine Learning Infrastructure team at Airbnb and a founding team member of Azure Cosmos DB, Microsoft Azure's distributed NoSQL database. He graduated with a BA in Computer Science from Dartmouth College.
A big thank you to our Premium Sponsors, @Databricks and @baseten for their generous support!
// Sign up for our Newsletter to never miss an event:
https://mlops.community/join/
// Watch all the conference videos here:
https://home.mlops.community/home/collections
// Check out the MLOps Community podcast: https://open.spotify.com/show/7wZygk3mUUqBaRbBGB1lgh?si=242d3b9675654a69
// Read our blog:
mlops.community/blog
// Join an in-person local meetup near you:
https://mlops.community/meetups/
// MLOps Swag/Merch:
https://mlops-community.myshopify.com/
// Follow us on Twitter:
https://twitter.com/mlopscommunity
//Follow us on Linkedin:
https://www.linkedin.com/company/mlopscommunity/
Shane Morris is now a Senior Executive Advisor at Devis.
Join us at our first in-person conference on June 25 all about AI Quality: https://www.aiqualityconference.com/
Huge thank you to @WeightsBiases for sponsoring this episode. WandB Free Courses - https://wandb.ai/telidavies/ml-news/reports/Introducing-W-B-MLOps-Courses-Free-Course-Effective-MLOps-Model-Development--VmlldzozMDk2ODA2
MLOps podcast #223 with Shane Morris, Senior Executive Advisor of Devis, Data Engineering in the Federal Sector.
// Abstract
Let's focus on autonomous systems rather than automation, and then super-narrow it down to smaller, cheaper, and more accessible autonomous systems.
// Bio
Former music and entertainment data and software person somehow moves into defense and national security, with hilarious and predictable results.
// MLOps Jobs board
https://mlops.pallet.xyz/jobs
// MLOps Swag/Merch
https://mlops-community.myshopify.com/
// Related Links
AI Quality in Person Conference: https://www.aiqualityconference.com/
Website: https://shanemorris.sucks
TikTok: https://www.tiktok.com/@shanemorrisdotsucks
WandB Free Courses - https://wandb.ai/telidavies/ml-news/reports/Introducing-W-B-MLOps-Courses-Free-Course-Effective-MLOps-Model-Development--VmlldzozMDk2ODA2
--------------- ✌️Connect With Us ✌️ -------------
Join our slack community: https://go.mlops.community/slack
Follow us on Twitter: @mlopscommunity
Sign up for the next meetup: https://go.mlops.community/register
Catch all episodes, blogs, newsletters, and more: https://mlops.community/
Connect with Demetrios on LinkedIn: https://www.linkedin.com/in/dpbrinkm/
Connect with Shane on LinkedIn: https://www.linkedin.com/in/shanetollmanmorris/
Join us at our first in-person conference on June 25 all about AI Quality: https://www.aiqualityconference.com/
Peter Guagenti is an accomplished business builder and entrepreneur with expertise in strategy, product development, marketing, sales, and operations. Peter has helped build multiple successful start-ups to exits, fueling high growth in each company along the way. He brings a broad perspective, deep problem-solving skills, the ability to drive innovation amongst teams, and a proven ability to convert strategy into action -- all backed up by a history of delivering results.
Huge thank you to AWS for sponsoring this episode. AWS - https://aws.amazon.com/
MLOps podcast #222 with Peter Guagenti, President & CMO of Tabnine - What Business Stakeholders Want to See from the ML Teams.
// Abstract
Peter Guagenti shares his expertise in the tech industry, discussing topics from managing large-scale tech legacy applications and data experimentation to the evolution of the Internet. He returns to his history of building and transforming businesses, such as his work in the early 90s for People magazine's website and his current involvement in AI development for software companies. Guagenti discusses the use of predictive modeling in customer management and emphasizes the importance of re-architecting solutions to fit customer needs.
He also delves deeper into the AI tools' effectiveness in software development and the value of maintaining privacy. Guagenti sees a bright future in AI democratization and shares his company's development of AI coding assistants. Discussing successful entrepreneurship, Guagenti highlights balancing technology and go-to-market strategies and the value of failing fast.
// Bio
Peter Guagenti is the President and Chief Marketing Officer at Tabnine. Guagenti is an accomplished business leader and entrepreneur with expertise in strategy, product development, marketing, sales, and operations. He most recently served as chief marketing officer at Cockroach Labs, and he previously held leadership positions at SingleStore, NGINX (acquired by F5 Networks), and Acquia (acquired by Vista Equity Partners). Guagenti also serves as an advisor to a number of visionary AI and data companies including DragonflyDB, Memgraph, and Treeverse.
// MLOps Jobs board
https://mlops.pallet.xyz/jobs
// MLOps Swag/Merch
https://mlops-community.myshopify.com/
// Related Links
AI Quality in Person Conference: https://www.aiqualityconference.com/
Measuring the impact of GitHub Copilot Survey: https://resources.github.com/learn/pathways/copilot/essentials/measuring-the-impact-of-github-copilot/
AWS Trainium and Inferentia:
https://aws.amazon.com/machine-learning/trainium/
https://aws.amazon.com/machine-learning/inferentia/AI coding assistants: 8 features enterprises should seek: https://www.infoworld.com/article/3694900/ai-coding-assistants-8-features-enterprises-should-seek.htmlCareers at Tabnine: https://www.tabnine.com/careers
--------------- ✌️Connect With Us ✌️ -------------
Join our slack community: https://go.mlops.community/slack
Follow us on Twitter: @mlopscommunity
Sign up for the next meetup: https://go.mlops.community/register
Catch all episodes, blogs, newsletters, and more: https://mlops.community/
Connect with Demetrios on LinkedIn: https://www.linkedin.com/in/dpbrinkm/
Connect with Peter on LinkedIn: https://www.linkedin.com/in/peterguagenti/
Join us at our first in-person conference on June 25 all about AI Quality: https://www.aiqualityconference.com/
Amritha Arun Babu Mysore has been an expert in the field of consumer electronics, software products, and online marketplaces for the past 15 years. She has experience developing supply chains from the ground up, delivering AI-based products to millions of users, and advocating for ethical AI across Amazon, Wayfair, Salesforce, and NetApp.
Abhik Choudhury is a Senior Analytics Managing Consultant and Data Scientist with 11 years of experience in designing and implementing scalable data solutions for organizations across various industries.
Huge thank you to @latticeflow for sponsoring this episode. LatticeFlow - https://latticeflow.ai/
MLOps podcast #221 with Amritha Arun Babu Mysore, ML Product Leader at Klaviyo and Abhik Choudhury, Managing Consultant Analytics at IBM, MLOps - Design Thinking to Build ML Infra for ML and LLM Use Cases.
// Abstract
As machine learning (ML) and large language models (LLMs) continue permeating industries, robust ML infrastructure and operations (ML Ops) are crucial to deploying these AI systems successfully. This podcast discusses best practices for building reusable, scalable, and governable ML Ops architectures tailored to ML and LLM use cases.
// Bio
Amritha Arun Babu Mysore
Amritha is an accomplished technology leader with over 12 years of experience spearheading product innovation and strategic initiatives at both large enterprises and rapid-growth startups.
Leveraging her background in engineering, supply chain, and business, Amritha has led high-performing teams to deliver transformative solutions solving complex challenges. She has driven product road mapping, requirements analysis, system design, and launch execution for advanced platforms in domains like machine learning, logistics, and e-commerce.
Abhik Choudhury
Abhik is a Senior Analytics Managing Consultant and Data Scientist with 11 years of experience in designing and implementing scalable data solutions for organizations across various industries. Throughout his career, Abhik developed a strong understanding of AI/ML, Cloud computing, database management systems, data modeling, ETL processes, and Big Data Technologies. Abhik's expertise lies in leading cross-functional teams and collaborating with stakeholders at all levels to drive data-driven decision-making in longitudinal pharmacy and medical claims and wholesale drug distribution areas.
// MLOps Jobs board
https://mlops.pallet.xyz/jobs
// MLOps Swag/Merch
https://mlops-community.myshopify.com/
// Related Links
AI Quality in Person Conference in collaboration with Kolena: https://www.aiqualityconference.com/
LatticeFlow website: https://latticeflow.ai/
--------------- ✌️Connect With Us ✌️ -------------
Join our slack community: https://go.mlops.community/slack
Follow us on Twitter: @mlopscommunity
Sign up for the next meetup: https://go.mlops.community/register
Catch all episodes, blogs, newsletters, and more: https://mlops.community/
Connect with Demetrios on LinkedIn: https://www.linkedin.com/in/dpbrinkm/
Connect with Abhik on LinkedIn: https://www.linkedin.com/in/abhik-choudhury-35450058
Connect with Amritha on LinkedIn: https://www.linkedin.com/in/amritha-arun-babu-a2273729/
Join us at our first in-person conference on June 25 all about AI Quality: https://www.aiqualityconference.com/
Demetrios Brinkmann is the founder of the MLOps Community. Brinkmann fell into the Machine Learning Operations world, and since, has interviewed the leading names around MLOps, Data Science, and Machine Learning.
Huge thank you to Weights & Biases for sponsoring this episode. Weights & Biases - https://wandb.ai/site
MLOps podcast #220 with our very own Founder of MLOps Community, Demetrios Brinkmann, Looking Back on 4 Years of the MLOps Community.
// Abstract
In this lively podcast episode, Mihail Eric hosts Demetrios Brinkmann, the founder of the MLOps Community, discussing its origin, structure, and challenges.
Demetrios shares amusing tales of job hunting on LinkedIn and building the community despite lacking technical expertise, emphasizing the value of sharing and humor.
They delve into the practicalities of hosting events, transitioning from self-funded to sponsorship-based, and tease upcoming activities with renowned speakers.
Mihail and Demetrios explore job dynamics, the importance of sustained relationships, and diverse engagement methods like newsletters and volunteering.
Demetrios reflects on his journey to Germany post-company closure, envisioning a global hub for AI learning, embodying the community's mission.
// Bio
At the moment Demetrios is immersing himself in Machine Learning by interviewing experts from around the world in the weekly MLOps.community meetups. Demetrios is constantly learning and engaging in new activities to get uncomfortable and learn from his mistakes. He tries to bring creativity into every aspect of his life, whether that be analyzing the best paths forward, overcoming obstacles, or building Lego houses with his daughter.
// MLOps Jobs board
https://mlops.pallet.xyz/jobs
// MLOps Swag/Merch
https://mlops-community.myshopify.com/
// Related Links
AI Quality in Person Conference in collaboration with Kolena: https://www.aiqualityconference.com/
Weights & Biases Free Course: https://wandb.ai/telidavies/ml-news/reports/Introducing-W-B-MLOps-Courses-Free-Course-Effective-MLOps-Model-Development--VmlldzozMDk2ODA2What Does Best in Class AI/ML Governance Look Like in Fin Services? // Charles Radclyffe // MLOps #2: https://youtu.be/l52sRMVPVk0
--------------- ✌️Connect With Us ✌️ -------------
Join our slack community: https://go.mlops.community/slack
Follow us on Twitter: @mlopscommunity
Sign up for the next meetup: https://go.mlops.community/register
Catch all episodes, blogs, newsletters, and more: https://mlops.community/
Connect with Demetrios on LinkedIn: https://www.linkedin.com/in/dpbrinkm/
Connect with Mihail on LinkedIn: https://www.linkedin.com/in/mihaileric/
Timestamps:
[00:00] Demetrios preferred coffee and bizarre listening
[01:44] The MLOps Community Brainchild
[04:22] The MLOps Community today
[07:15] AI Quality in Person Conference on June 25th!
[08:42] Community Quality
[10:00] Community Learnings and the Genesis
[17:55] The 600 Mark
[20:15] The Feedback form
[22:52] Demetrios' Journey and Learnings
[29:01] Building full tolerance
[29:55] Weights & Biases Free Course Ad
[34:52] Building community involvement for professional success and networking
[38:52] Balance in Community Growth
[43:56] Collection of volunteers
[49:00] Events Challenges
[53:28] The future of MLOps Community
[59:40] "Caveman" lifestyle choice
[1:00:45] Stronger Hallucinogen
[1:02:30] Wrap up
Join us at our first in-person conference on June 25 all about AI Quality: https://www.aiqualityconference.com/
Huge thank you to Databricks AI for sponsoring this episode. Databricks - http://databricks.com/
Bandish Shah is an Engineering Manager at MosaicML/Databricks, where he focuses on making generative AI training and inference efficient, fast, and accessible by bridging the gap between deep learning, large-scale distributed systems, and performance computing.
Davis Blalock is a Research Scientist and the first employee of Mosaic ML: a GenAI startup acquired for $1.3 billion by Databricks.
MLOps podcast #219 with Databricks' Engineering Manager, Bandish Shah and Research Scientist Davis Blalock, The Art and Science of Training Large Language Models.
// Abstract
What's hard about language models at scale? Turns out...everything. MosaicML's Davis and Bandish share war stories and lessons learned from pushing the limits of LLM training and helping dozens of customers get LLMs into production. They cover what can go wrong at every level of the stack, how to make sure you're building the right solution, and some contrarian takes on the future of efficient models.
// Bio
Bandish Shah
Bandish Shah is an Engineering Manager at MosaicML/Databricks, where he focuses on making generative AI training and inference efficient, fast, and accessible by bridging the gap between deep learning, large-scale distributed systems, and performance computing. Bandish has over a decade of experience building systems for machine learning and enterprise applications. Prior to MosaicML, Bandish held engineering and development roles at SambaNova Systems where he helped develop and ship the first RDU systems from the ground up, and Oracle where he worked as an ASIC engineer for SPARC-based enterprise servers.
Davis Blalock
Davis Blalock is a research scientist at MosaicML. He completed his PhD at MIT, advised by Professor John Guttag. His primary work is designing high-performance machine learning algorithms. He received his M.S. from MIT and his B.S. from the University of Virginia. He is a Qualcomm Innovation Fellow, NSF Graduate Research Fellow, and Barry M. Goldwater Scholar.
// MLOps Jobs board
https://mlops.pallet.xyz/jobs
// MLOps Swag/Merch
https://mlops-community.myshopify.com/
// Related Links
AI Quality In-person Conference: AI Quality in Person Conference: https://www.aiqualityconference.com/
Website: http://databricks.com/
Davis Summarizes Papers Newsletter signup link
Davis' Newsletters:
Learning to recognize spoken words from five unlabeled examples in under two seconds: https://arxiv.org/abs/1609.09196
Training on data at 5GB/s in a single thread: https://arxiv.org/abs/1808.02515
Nearest-neighbor searching through billions of images per second in one thread with no indexing: https://arxiv.org/abs/1706.10283
Multiplying matrices 10-100x faster than a matrix multiply (with some approximation error): https://arxiv.org/abs/2106.10860
Hidden Technical Debt in Machine Learning Systems: https://proceedings.neurips.cc/paper_files/paper/2015/file/86df7dcfd896fcaf2674f757a2463eba-Paper.pdf
--------------- ✌️Connect With Us ✌️ -------------
Join our slack community: https://go.mlops.community/slack
Follow us on Twitter: @mlopscommunity
Sign up for the next meetup: https://go.mlops.community/register
Catch all episodes, blogs, newsletters, and more: https://mlops.community/
Connect with Demetrios on LinkedIn: https://www.linkedin.com/in/dpbrinkm/
Connect with Davis on LinkedIn: https://www.linkedin.com/in/dblalock/
Connect with Bandish on LinkedIn: https://www.linkedin.com/in/bandish-shah/
// Abstract
Diego, David, Ads, and Katharine, bring to light the risks, vulnerabilities, and evolving security landscape of machine learning as we venture into the AI-driven future. They underscore the importance of education in managing AI risks and the critical role privacy engineering plays in this narrative. They explore the legal and ethical implications of AI technologies, fostering a vital conversation on the balance between utility and privacy.
// Bio
Diego Oppenheimer - Moderator
Diego Oppenheimer is a serial entrepreneur, product developer and investor with an extensive background in all things data. Currently, he is a Partner at Factory a venture fund specialized in AI investments as well as a co-founder at Guardrails AI. Previously he was an executive vice president at DataRobot, Founder and CEO at Algorithmia (acquired by DataRobot) and shipped some of Microsoft’s most used data analysis products including Excel, PowerBI and SQL Server.
Diego is active in AI/ML communities as a founding member and strategic advisor for the AI Infrastructure Alliance and MLops.Community and works with leaders to define AI industry standards and best practices. Diego holds a Bachelor's degree in Information Systems and a Masters degree in Business Intelligence and Data Analytics from Carnegie Mellon University.
Ads Dawson
A mainly self-taught, driven, and motivated proficient application, network infrastructure & cyber security professional holding over eleven years experience from start-up to large-size enterprises leading the incident response process and specializing in extensive LLM/AI Security, Web Application Security and DevSecOps protecting REST API endpoints, large-scale microservice architectures in hybrid cloud environments, application source code as well as EDR, threat hunting, reverse engineering, and forensics.
Ads have a passion for all things blue and red teams, be that offensive & API security, automation of detection & remediation (SOAR), or deep packet inspection for example.
Ads is also a networking veteran and love a good PCAP to delve into. One of my favorite things at Defcon is hunting for PWNs at the "Wall of Sheep" village and inspecting malicious payloads and binaries.
Katharine Jarmul
Katharine Jarmul is a privacy activist and data scientist whose work and research focuses on privacy and security in data science workflows. She recently authored Practical Data Privacy for O'Reilly and works as a Principal Data Scientist at Thoughtworks. Katharine has held numerous leadership and independent contributor roles at large companies and startups in the US and Germany -- implementing data processing and machine learning systems with privacy and security built in and developing forward-looking, privacy-first data strategy.
David Haber
David has started and grown several technology companies. He developed safety-critical AI in the healthcare space and for autonomous flight. David has educated thousands of people and Fortune 500 companies on the topic of AI. Outside of work, he loves to spend time with his family and enjoys training for the next Ironman.
A big thank you to our Premium Sponsors, @Databricks and @baseten for their generous support!
// Sign up for our Newsletter to never miss an event:
https://mlops.community/join/
// Watch all the conference videos here:
https://home.mlops.community/home/collections
// Check out the MLOps Community podcast: https://open.spotify.com/show/7wZygk3mUUqBaRbBGB1lgh?si=242d3b9675654a69
// Read our blog:
mlops.community/blog
// Join an in-person local meetup near you:
https://mlops.community/meetups/
// MLOps Swag/Merch:
https://mlops-community.myshopify.com/
// Follow us on Twitter:
https://twitter.com/mlopscommunity
//Follow us on Linkedin:
https://www.linkedin.com/company/mlopscommunity/
Frank Liu is the Director of Operations & ML Architect at Zilliz, where he serves as a maintainer for the Towhee open-source project.
Jiang Chen is the Head of AI Platform and Ecosystem at Zilliz.
Yujian Tang is a developer advocate at Zilliz. He has a background as a software engineer working on AutoML at Amazon.
MLOps Coffee Sessions Special episode with Zilliz, Why Purpose-built Vector Databases Matter for Your Use Case, fueled by our Premium Brand Partner, Zilliz.
Engineering deep-dive into the world of purpose-built databases optimized for vector data. In this live session, we explore why non-purpose-built databases fall short in handling vector data effectively and discuss real-world use cases demonstrating the transformative potential of purpose-built solutions. Whether you're a developer, data scientist, or database enthusiast, this virtual roundtable offers valuable insights into harnessing the full potential of vector data for your projects.
// Bio
Jiang Chen
Frank Liu is Head of AI & ML at Zilliz, with over eight years of industry experience in machine learning and hardware engineering. Before joining Zilliz, Frank co-founded Orion Innovations, an IoT startup based in Shanghai, and worked as an ML Software Engineer at Yahoo in San Francisco. He presents at major industry events like the Open Source Summit and writes tech content for leading publications such as Towards Data Science and DZone. His passion for ML extends beyond the workplace; in his free time, he trains ML models and experiments with unique architectures. Frank holds MS and BS degrees in Electrical Engineering from Stanford University.
Frank Liu
Jiang Chen is the Head of AI Platform and Ecosystem at Zilliz. With years of experience in data infrastructures and information retrieval, Jiang previously served as a tech lead and product manager for Search Indexing at Google. Jiang holds a Master's degree in Computer Science from the University of Michigan, Ann Arbor.
Yujian Tang
Yujian Tang is a Developer Advocate at Zilliz. He has a background as a software engineer working on AutoML at Amazon. Yujian studied Computer Science, Statistics, and Neuroscience with research papers published to conferences including IEEE Big Data. He enjoys drinking bubble tea, spending time with family, and being near water.
// MLOps Jobs board
https://mlops.pallet.xyz/jobs
// MLOps Swag/Merch
https://mlops-community.myshopify.com/
// Related Links
Website: https://zilliz.com/
Neural Priming for Sample-Efficient Adaptation: https://arxiv.org/abs/2306.10191LIMA: Less Is More for Alignment: https://arxiv.org/abs/2305.11206ColBERT: Efficient and Effective Passage Search via Contextualized Late Interaction over BERT: https://arxiv.org/abs/2004.12832
Milvus Vector Database by Zilliz: https://zilliz.com/what-is-milvus
--------------- ✌️Connect With Us ✌️ -------------
Join our slack community: https://go.mlops.community/slack
Follow us on Twitter: @mlopscommunity
Sign up for the next meetup: https://go.mlops.community/register
Catch all episodes, blogs, newsletters, and more: https://mlops.community/
Timestamps:
[00:00] Demetrios' musical intro
[04:36] Vector Databases vs. LLMs
[07:51] Relevance Over Speed
[12:55] Pipelines
[16:19] Vector Databases Integration Benefits
[26:42] Database Diversity Market
[27:38] Milus vs. Pinecone
[30:22] Vector DB for Training & Deployment
[34:32] Future proof of AI applications
[45:16] Data Size and Quality
[48:53] ColBERT Model
[54:25] Vector Data Consistency Best Practices
[57:24] Wrap up
Huge thank you to LatticeFlow AI for sponsoring this episode. LatticeFlow AI - https://latticeflow.ai/.Dr. Petar Tsankov is a researcher and entrepreneur in the field of Computer Science and Artificial Intelligence.
MLOps podcast #218 with Petar Tsankov, Co-Founder and CEO at LatticeFlow AI, A Decade of AI Safety and Trust.
// Abstract
// Bio
Co-founder & CEO at LatticeFlow AI, building the world's first product enabling organizations to build performant, safe, and trustworthy AI systems.
Before starting LatticeFlow AI, Petar was a senior researcher at ETH Zurich working on the security and reliability of modern systems, including deep learning models, smart contracts, and programmable networks.
// MLOps Jobs board
https://mlops.pallet.xyz/jobs
// MLOps Swag/Merch
https://mlops-community.myshopify.com/
// Related Links
Website: https://latticeflow.ai/
ERAN, the world's first scalable verifier for deep neural networks: https://github.com/eth-sri/eran
VerX, the world's first fully automated verifier for smart contracts: https://verx.ch
Securify, the first scalable security scanner for Ethereum smart contracts: https://securify.ch
DeGuard, de-obfuscates Android binaries: http://apk-deguard.com
SyNET, the first scalable network-wide configuration synthesis tool: https://synet.ethz.ch
--------------- ✌️Connect With Us ✌️ -------------
Join our slack community: https://go.mlops.community/slack
Follow us on Twitter: @mlopscommunity
Sign up for the next meetup: https://go.mlops.community/register
Catch all episodes, blogs, newsletters, and more: https://mlops.community/
Connect with Demetrios on LinkedIn: https://www.linkedin.com/in/dpbrinkm/
Connect with Petar on LinkedIn: https://www.linkedin.com/in/petartsankov/
Timestamps:
[00:00] Petar's preferred coffee
[00:29] Takeaways
[03:15] Shout out to LatticeFlow for sponsoring this episode!
[03:22] Please like, share, leave a review, and subscribe to our MLOps channels!
[03:42] Expansion
[05:16] Zurich ETH
[07:06] AI Safety
[09:24] Optimizing one metric, no fixed data sets
[12:19] Trust life-changing issues
[14:59] So much interest in GenAI
[16:45] Explosion of GenAI Trust and Safety
[21:14] Red Teaming
[25:22] Trustworthy AI in Industry
[27:43] DataOps Challenges
[33:42] Trusting Third-Party Models
[37:00] Testing Open Source Models
[41:41] Specialized ML for Leasing
[43:04] Regulation and Financial Incentives
[45:30] Regulations Drive Innovation Balance
[47:23] Regulations vs Certification: Voluntary Prove
[52:24] Workflow Transparency: Trust & Efficiency
[53:20] Engineers Balance Compliance Risks
[54:53] Pushing Deep Learning Limits
[57:31] Wrap up
Thank you to Zilliz our wonderful sponsors of this episode create some amazing stuff with Zilliz RAG - https://zilliz.com/vector-database-use-cases/llm-retrieval-augmented-generation
Sam Bean is a seasoned AI and machine learning expert, specializing in Large Language Models (LLMs) and search tech.
With a computer science background and a drive for innovation, Sam leads the team at Rewind AI in leveraging advanced tech to tackle complex challenges.
MLOps podcast #217 with Sam Bean, Software Engineer (Applied AI) at Rewind.ai, The Real E2E RAG Stack.
// Abstract
What does a fully operational LLM + Search stack look like when you're running your own retrieval and inference infrastructure? What does the flywheel really mean for RAG applications? How do you maintain the quality of your responses? How do you prune/dedupe documents to maintain your document quality?
// Bio
Sam has been training, evaluating, and deploying production-grade inference solutions for language models for the past 2 years at You.com. Previous to that he built personalization algorithms at StockX.
// MLOps Jobs board
https://mlops.pallet.xyz/jobs
// MLOps Swag/Merch
https://mlops-community.myshopify.com/
// Related Links
Website: https://github.com/sam-h-bean/
REinforced Self Training (REST) - https://arxiv.org/pdf/2308.08998.pdf
REST meets REACT - https://arxiv.org/pdf/2312.10003.pdf
--------------- ✌️Connect With Us ✌️ -------------
Join our slack community: https://go.mlops.community/slack
Follow us on Twitter: @mlopscommunity
Sign up for the next meetup: https://go.mlops.community/register
Catch all episodes, blogs, newsletters, and more: https://mlops.community/
Connect with Demetrios on LinkedIn: https://www.linkedin.com/in/dpbrinkm/
Connect with Sam on LinkedIn: https://www.linkedin.com/in/samuel-h-bean/
Timestamps:
[00:00] Sam's preferred coffee
[00:11] Takeaways
[03:52] A competitive coding pinball player
[07:18] Sam's MLOps journey
[10:33] Search Challenges with ML
[15:04] Expensive evaluation
[21:04] Labeling Parties Boost Data Quality
[24:10] Zeno's Paradox of Motion
[25:51] Sam's job at Rewind AI
[29:35] Multimodal RAG
[30:59 - 32:06] Zilliz Ad
[32:07] University of Prague paper leak
[36:38] Signals behind the scenes
[39:28] Content Over Metadata Approach
[43:22] Optionality around evaluation and search
[48:35] Incremental Robustness Building
[51:33] Solid Foundations for Success
[53:42] Production RAGs
[1:00:06] Thoughts on DSPy
[1:05:40] Using DSPy in Production
[1:08:26] Wrap up
Anass Bensrhir is the Associate Partner of McKinsey & Company Casablanca. Anu Arora is the
Principal Data Engineering at McKinsey & Company.
Check out
mckinsey.com/quantumblack
MLOps podcast #214 with QuantumBlack AI by McKinsey's Principal Data Engineer, Anu Arora and Associate Partner, Anass Bensrhir, Managing Data for Effective GenAI Application brought to you by our Premium Brand Partner QuantumBlack AI by @McKinsey .
// Abstract
Generative AI is poised to bring impact across all industries and business functions across industries
While many companies pilot GenAI, only a few have deployed GenAI use cases, e.g., retailers are producing videos to answer common customer questions using ChatGPT. A majority of organizations are facing challenges to industrialize and scale, with data being one of the biggest inhibitors.
Organizations need to strengthen their data foundations given that among leading organizations, 72% noted managing data among the top challenges preventing them from scaling impact. Furthermore, leaders noticed that +31% of their staff's time is spent on non-value-added tasks due to poor data quality and availability issues.
// Bio
Anu Arora
Data architect(~12 years) and have experience in Big data technologies, API development, building scalable data pipelines including DevOps and DataOps, and building GenAI solutions.
Anass Bensrhir
Anass Leads QuantumBlack in Africa, he specializes in the Financial sector and helps organizations deliver successful large Data transformation programs.
// MLOps Jobs board
https://mlops.pallet.xyz/jobs
// MLOps Swag/Merch
https://mlops-community.myshopify.com/
// Related Links
Website: https://www.mckinsey.com/capabilities/quantumblack/how-we-help-clients
--------------- ✌️Connect With Us ✌️ -------------
Join our slack community: https://go.mlops.community/slack
Follow us on Twitter: @mlopscommunity
Sign up for the next meetup: https://go.mlops.community/register
Catch all episodes, blogs, newsletters, and more: https://mlops.community/
Connect with Demetrios on LinkedIn: https://www.linkedin.com/in/dpbrinkm/
Connect with Anu on LinkedIn: https://uk.linkedin.com/in/anu-arora-072012
Connect with Anass on LinkedIn: https://www.linkedin.com/in/abensrhir/
Timestamps:
[00:00] Anass and Anu's preferred coffee
[00:35] Takeaways
[04:02] Please like, share, leave a review, and subscribe to our MLOps channels!
[04:09] Huge shout out to our sponsor QuantumBlack!
[04:29] Anu's tech background
[06:31] Anass tech background
[07:28] The landscape of data
[10:37] Dealing with unstructured data
[15:51] Data lakes and ETL processes
[22:19] Data Engineers' Heavy Workload
[29:49] Data privacy and PII in the new LLMs paradigm
[36:13] Balancing LLM Adoption Risk
[44:06] Effective LMS Implementation Strategy
[49:00] Decisions: Create or Wait
[50:39] Wrap up
Alex Volkov serves as the AI Evangelist with
Weights & Biases, Host of ThursdAI, Founder and CEO Targum and AI Consultant GPU POOR Def. not an owl.
MLOps podcast #215 with Alex Volkov, AI Evangelist at Weights & Biases, Becoming an AI Evangelist.
// Abstract
Follow Alex's journey into the world of AI, from being interested in running his first AI models to founding an AI startup, running a successful weekly AI news podcast & newsletter, and landing a job with @WeightsBiases .
// Bio
Alex Volkov is an AI Evangelist at Weights & Biases, celebrated for his expertise in clarifying the complexities of AI and advocating for its beneficial uses. He is the founder and host of ThursdAI, a weekly newsletter and podcast that explores the latest in AI, its practical applications, open-source and innovation. With a solid foundation as an AI startup founder and 20 years in full-stack software engineering, Alex offers a deep well of experience and insight into AI innovation.
// MLOps Jobs board
https://mlops.pallet.xyz/jobs
// MLOps Swag/Merch
https://mlops-community.myshopify.com/
// Related Links
Evaluation Survey: https://hq.yougot.us/primary/WebInterview/3AW6LW5D/Start
Website: https://thursdai.news
Alex on X (+X spaces also are also there) - https://twitter.com/altryne/
ThursdAI podcast/newsletter - https://sub.thursdai.news
Denver local AI tinkerers meetup - https://denver-boulder.aitinkerers.org/
Weights & Biases Growth Team hack week review - https://www.youtube.com/watchInterview w/
Crew AI creator Joao Moura - https://sub.thursdai.news/p/jan14-sunday-special-deep-dives
--------------- ✌️Connect With Us ✌️ -------------
Join our slack community: https://go.mlops.community/slack
Follow us on Twitter: @mlopscommunity
Sign up for the next meetup: https://go.mlops.community/register
Catch all episodes, blogs, newsletters, and more: https://mlops.community/
Connect with Demetrios on LinkedIn: https://www.linkedin.com/in/dpbrinkm/
Connect with Alex on LinkedIn: https://www.linkedin.com/in/alex-volkov-/
// Abstract
From startups achieving significant value with minor capabilities to AI revolutionizing sales calls and raising sales by 30%, we explore a series of interesting real-world use cases. Understanding the objectives and complexities of various industries, exploring the challenges of launching products, and highlighting the vital integration of the human touch with technology, this episode is a treasure trove of insights.
// Bio
Greg Kamradt - Moderator
Greg has mentored thousands of developers and founders, empowering them to build AI-centric applications. By crafting tutorial-based content, Greg aims to guide everyone from seasoned builders to ambitious indie hackers. Some of his popular works: 'Introduction to LangChain Part 1, Part 2' (+145K views), and 'How To Question A Book' featuring Pinecone (+115K Views). Greg partners with companies during their product launches, feature enhancements, and funding rounds. His objective is to cultivate not just awareness, but also a practical understanding of how to optimally utilize a company's tools. He previously led Growth @ Salesforce for Sales & Service Clouds in addition to being early on at Digits, a FinTech Series-C company.
Agnieszka Mikołajczyk-Bareła
Senior AI Engineer@Chaptr working on LLMs. PhD, author of datasets, scientific papers, and publications with over 1800 citations, holding numerous scholarships and awards. Daily, she conducts her research on her grant "Detecting and overcoming bias in data with explainable artificial intelligence" Preludium, awarded by Polish National Centre. She is a co-organizer of PolEval2021 and PolEval 2022 tasks with punctuation prediction and restoration.
She organizes and actively contributes to the scientific community in her free time: she managed and led the team during the HearAI project focused on modeling Sign Language. A former organizer and a team leader at the open-source project. As an ML Expert, she supports the project "Susana" designed to detect and read product expiry dates to help the Blind "see".
Jason Liu
Jason is a machine learning engineer and technical advisor.
Arjun Kannan
Arjun Kannan builds products, businesses, and teams. Currently building ResiDesk, bringing AI copilots to help real estate forecast renewals, reduce turnover, and hit their budget. Arjun built and led product and engineering functions at Climb Credit (serving 100k students, doubling loan growth for 3 years straight) and at BlackRock (creating $400mm in annual revenue), and helped build multiple startups and small companies before that.
// Sign up for our Newsletter to never miss an event:
https://mlops.community/join/
// Watch all the conference videos here:
https://home.mlops.community/home/collections
// Check out the MLOps Community podcast: https://open.spotify.com/show/7wZygk3mUUqBaRbBGB1lgh?si=242d3b9675654a69
// Read our blog:
mlops.community/blog
// Join an in-person local meetup near you:
https://mlops.community/meetups/
// MLOps Swag/Merch:
https://mlops-community.myshopify.com/
// Follow us on Twitter:
https://twitter.com/mlopscommunity
//Follow us on Linkedin:
https://www.linkedin.com/company/mlopscommunity/
Daniel Svonava is the Co-Founder of Superlinked. Daniel Svonava attended the Faculty of Informatics and Information Technologies, Slovak University of Technology.
MLOps podcast #214 with Daniel Svonava, CEO & Co-founder at Superlinked, Information Retrieval & Relevance: Vector Embeddings for Semantic Search
// Abstract
In today's information-rich world, the ability to retrieve relevant information effectively is essential. This lecture explores the transformative power of vector embeddings, revolutionizing information retrieval by capturing semantic meaning and context. We'll delve into:
- The fundamental concepts of vector embeddings and their role in semantic search
- Techniques for creating meaningful vector representations of text and data
- Algorithmic approaches for efficient vector similarity search and retrieval
- Practical strategies for applying vector embeddings in information retrieval systems
// Bio
Daniel is an entrepreneurial technologist with a 20 year career starting with competitive programming and web development in highschool, algorithm research and Google & IBM Research internships during university, first entrepreneurial steps with his own computational photography startup and a 6 year tenure as a tech lead for ML infrastructure at YouTube Ads, where his ad performance forecasting engine powers the purchase of $10B of ads per year.
Presently, Daniel is a co-founder of Superlinked.com - a ML infrastructure startup that makes it easier to build information-retrieval heavy systems - from Recommender Engines to Enterprise-focused LLM apps.
// MLOps Jobs board
https://mlops.pallet.xyz/jobs
// MLOps Swag/Merch
https://mlops-community.myshopify.com/
// Related Links
--------------- ✌️Connect With Us ✌️ -------------
Join our slack community: https://go.mlops.community/slack
Follow us on Twitter: @mlopscommunity
Sign up for the next meetup: https://go.mlops.community/register
Catch all episodes, blogs, newsletters, and more: https://mlops.community/
Connect with Demetrios on LinkedIn: https://www.linkedin.com/in/dpbrinkm/
Connect with Daniel on LinkedIn: https://www.linkedin.com/in/svonava/?originalSubdomain=ch
Morgan McGuire has held a variety of roles in the past 13 years. In 2008, he completed a Research Internship at Queen Mary, University of London.
Currently, he is the Head of Growth ML and Growth ML Engineer at Weights & Biases.
Anish Shah has been working in the tech industry since 2015. In 2015, he was a Technical Support at Fox School of Business at Temple University.
In 2021, he has been an MLOps Engineer - Growth and a Tier 2 Support Machine Learning Engineer at Weights & Biases.
______________________________________________
Large Language Models have taken the world by storm. But what are the real use cases? What are the challenges in productionizing them? In this event, you will hear from practitioners about how they are dealing with things such as cost optimization, latency requirements, trust of output, and debugging. You will also get the opportunity to join workshops that will teach you how to set up your use cases and skip over all the headaches.
Join the AI in Production Conference on February 22 here: https://home.mlops.community/home/events/ai-in-production-2024-02-15
______________________________________________
MLOps podcast #213 with Weights and Biases' Growth Director, Morgan McGuire and MLE, Anish Shah, Evaluating and Integrating ML Models brought to you by our Premium Brand Partner @WeightsBiases.
// Abstract
Anish Shah and Morgan McGuire share insights on their journey into ML, the exciting work they're doing at Weights and Biases, and their thoughts on MLOps. They discuss using large language models (LLMs) for translation, pre-written code, and internal support. They discuss the challenges of integrating LLMs into products, the need for real use cases, and maintaining credibility.
They also touch on evaluating ML models collaboratively and the importance of continual improvement. They emphasize understanding retrieval and balancing novelty with precision. This episode provides a deep dive into Weights and Biases' work with LLMs and the future of ML evaluation in MLOps. It's a must-listen for anyone interested in LLMs and ML evaluation.
// Bio
Anish Shah
Anish loves turning ML ideas into ML products. He started his career working with multiple Data Science teams within SAP, working with traditional ML, deep learning, and recommendation systems before landing at Weights & Biases. With the art of programming and a little magic, Anish crafts ML projects to help better serve our customers, turning “oh nos” to “a-ha”s!
Morgan McGuire
Morgan is a Growth Director and an ML Engineer at Weights & Biases. He has a background in NLP and previously worked at Facebook on the Safety team where he helped classify and flag potentially high-severity content for removal.
// MLOps Jobs board
https://mlops.pallet.xyz/jobs
// MLOps Swag/Merch
https://mlops-community.myshopify.com/
// Related Links
AI in Production Conference: https://home.mlops.community/home/events/ai-in-production-2024-02-15
Website: https://wandb.ai/
Prompt Templates the Song: https://www.youtube.com/watch?v=g6WT85gIsE8
--------------- ✌️Connect With Us ✌️ -------------
Join our slack community: https://go.mlops.community/slack
Follow us on Twitter: @mlopscommunity
Sign up for the next meetup: https://go.mlops.community/register
Catch all episodes, blogs, newsletters, and more: https://mlops.community/
Connect with Demetrios on LinkedIn: https://www.linkedin.com/in/dpbrinkm/
Connect with Morgan on LinkedIn: https://www.linkedin.com/in/morganmcg1/
Connect with Anish on LinkedIn: https://www.linkedin.com/in/anish-shah/
"in Kaggle you normally see a 1-1 ratio of positive to negative examples" huh? has he ever done a Kaggle competition? this statement is totally off