Discover
ODSC's Ai X Podcast
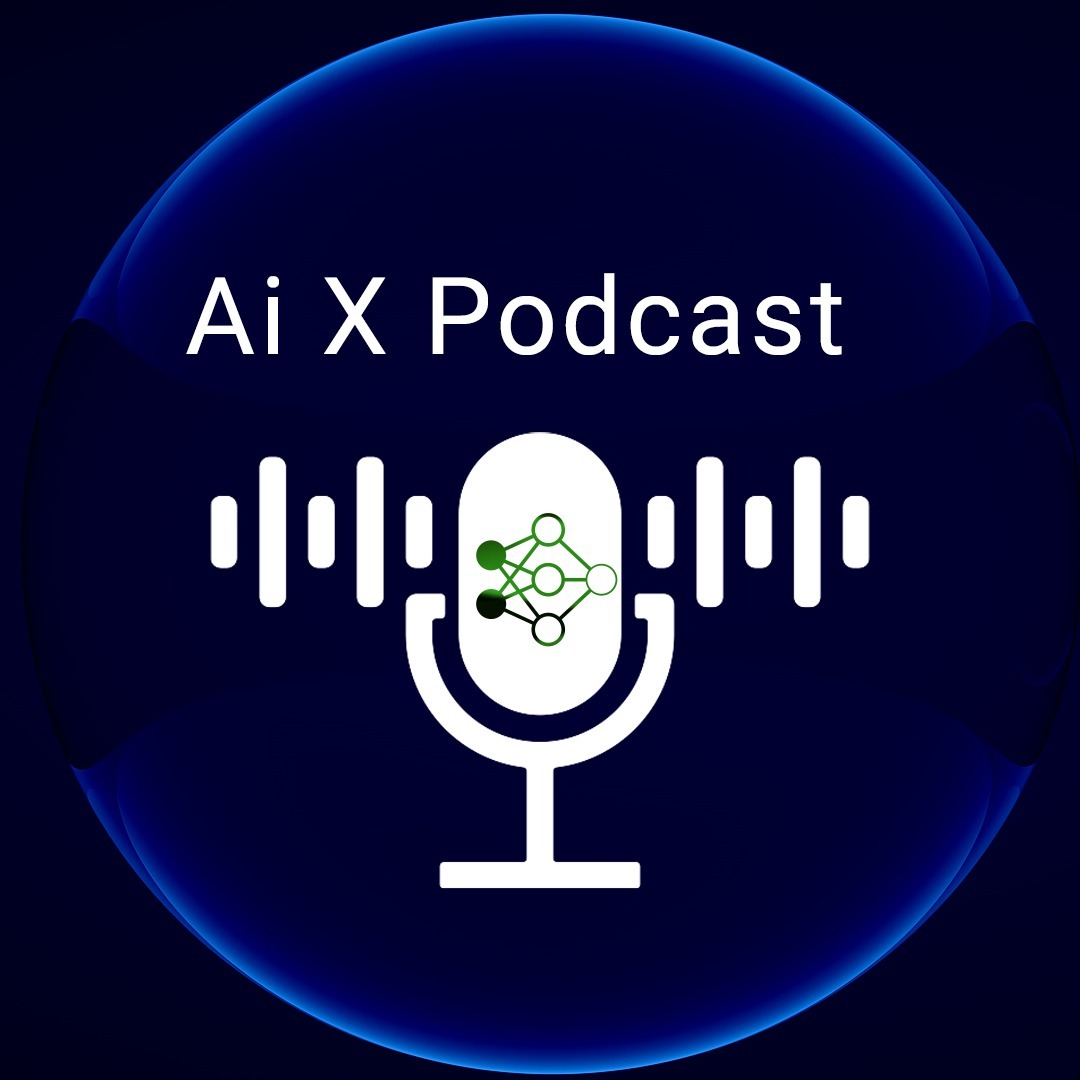
ODSC's Ai X Podcast
Author: ODSC
Subscribed: 10Played: 55Subscribe
Share
Description
With Ai X Podcast, Open Data Science Conference (ODSC) brings its vast experience in building community and its knowledge of the data science and AI fields to the podcast platform. The interests and challenges of the data science community are wide ranging. To reflect this Ai X Podcast will offer a similarly wide range of content, from one-on-one interviews with leading experts, to career talks, to educational interviews, to profiles of AI Startup Founders. Join us every two weeks to discover what’s going on in the data science community.
Find more ODSC lightning interviews, webinars, live trainings, certifications, bootcamps here – aiplus.training/
Don't miss out on this exciting opportunity to expand your knowledge and stay ahead of the curve.
Find more ODSC lightning interviews, webinars, live trainings, certifications, bootcamps here – aiplus.training/
Don't miss out on this exciting opportunity to expand your knowledge and stay ahead of the curve.
36 Episodes
Reverse
In this episode of ODSC’s Ai X Podcast, we speak with Hilary Mason about the intersection of gaming and generative AI. Hilary Mason is the co-founder and CEO of Hidden Door. Prior to her work at Hidden Door, she was the General Manager of the Machine Learning business unit at Cloudera. Before that, she was a Data Scientist in Residence at Accel Partners, Chief Scientist at bitly, and plenty more. She’s also an experienced entrepreneur and founded Fast Forward Labs, an applied machine learning research and consulting startup.SHOW NOTE TOPICS:Hillary’s journey and the key decisions that shaped her careerWhere are we with generative AI?How generative AI impacts creative domains such as story gamesDiscussion on Hillary’s latest startup, Hidden DoorNPC (Non-player characters) in gaming and the challenges of scriptingWhat gaming can teach us about AI and generative AI Building ML products that don’t have a single objective function to optimizeWhy evaluating generative AI has become much harder than training generative AIHas the gaming industry embraced narrative AI and generative AI in general?How Hidden Door’s gaming models were trainedHow the role of data scientists is evolving in the era of generative AIKey lessons learned as an entrepreneurHow do you get access to Hidden Door? Tell us about the vision behind your new startup Hidden Door which is essentially a generative AI game studio.How was Hidden Door trained?What advice do you have about generative AI’s impact on game developers?In the age of generative AI, how do you see the relationship between traditional machine learning methods and generative models evolving? Where do you think traditional ML continues to hold value?How do you see the role of data scientists evolving in the era of generative AI?What are some of the key lessons you’ve learned from your journey as a tech entrepreneur, and how have they shaped your leadership style?How do you build ethical businesses in a moment of technical change and uncertainty?How can people follow your work?SHOW NOTES:- Fast Forward Labs: https://github.com/fastforwardlabs- Hidden Door:https://www.hiddendoor.co/- Hidden Door Discord: https://discord.com/invite/hiddendoorThis episode was sponsored by: Ai+ Training https://aiplus.training/ Home to hundreds of hours of on-demand, self-paced AI training, ODSC interviews, free webinars, and certifications in in-demand skills like LLMs and Prompt EngineeringAnd created in partnership with ODSC https://odsc.com/ The Leading AI Training Conference, featuring expert-led, hands-on workshops, training sessions, and talks on cutting-edge AI topics and tools, from data science and machine learning to generative AI to LLMOpsNever miss an episode, subscribe now!
In this episode of ODSC’s Ai X Podcast, Sam Manning, Senior Research Fellow at the Centre for the Governance of AI will be discussing AI’s impact on work. Sam gained significant attention last year when he co-authored a groundbreaking paper with colleagues from the University of Pennsylvania and OpenAI, examining the Labor Market Impact Potential of Large Language Models. At the Centre for the Governance of AI, Sam focuses on measuring the economic impacts of frontier AI systems like LLMs and GenAI, and designs policy options to ensure that advanced AI fosters broadly shared economic prosperity.In this episode, we’ll dive into Sam’s findings and explore how we can better measure and predict AI’s influence on work.Topics- Guest introduction and role- The Center for the Governance of AI and its mission- The occupational exposure method for measuring AI’s impact- Do predictions tell us whether the impact will be positive or negative - Why lower-skilled less experienced workers are getting a much bigger boost in productivity than their more experienced, higher-skilled counterparts- Why higher-income jobs face greater exposure to LLM capabilities, - Explanation of the rubrics developed for assessing task exposure and the automation potential of AI- How do we measure or evaluate which jobs are most likely to be replaced by AI- Augmentation vs. Automation and what are the significant barriers to fully automating tasks with LLMs, and what role does augmentation play- Real-world complexity and the challenges in accounting for the complexity of real-world jobs when evaluating AI's impact?- Discuss the limitations of the current methods for evaluating AI’s impact on labor markets- How to account for the potential future capabilities of AI in your evaluations?- Significant findings from research on AI's impact on income inequality?- Why its important to consider both task-level and economy-wide impacts when assessing AI's influence - Task-Level Productivity: How do you measure the task-level productivity impacts of AI, and what have been some significant findings using this approach?- Advice for navigating AI’s impact on their work and careers- How to follow Sam’s workShow Notes:Some of Sam's recent work includes:- A paper (https://www.science.org/doi/10.1126/science.adj0998) in Science magazine called GPTs are GPTs: Labor Market Impact Potential of LLMs, (also Arxiv link here https://arxiv.org/pdf/2303.10130)- An article (https://www.brookings.edu/articles/ais-impact-on-income-inequality-in-the-us/) for the Brookings Institution investigating AI's impact on earnings inequality- A GovAI blog post (https://www.governance.ai/post/predicting-ais-impact-on-work) on the topic of "automation evaluations" called "Predicting AI's Impact on Work". - Center for the Governance of AI: https://www.governance.ai/- US Bureau of Labor Statistics: https://www.bls.gov/- O*NET Resource Center: https://www.onetcenter.org/database.html- The customer service study by Erik Brynjolfsson, Linsdey Raymond, Danielle Li: https://www.nber.org/papers/w31161- The MIT study Sam mentioned on the cost-effectiveness of adopting computer vision systems to automate worker tasks: https://papers.ssrn.com/sol3/papers.cfm?abstract_id=4700751- Carl Frey's book, The Technology Trap: https://press.princeton.edu/books/hardcover/9780691172798/the-technology-trap- Sam’s Twitter: https://x.com/sj_manningThis episode was sponsored by: Ai+ Training https://aiplus.training/ Home to hundreds of hours of on-demand, self-paced AI training, ODSC interviews, free webinars, and certifications in in-demand skills like LLMs and Prompt EngineeringAnd created in partnership with ODSC https://odsc.com/ The Leading AI Training Conference, featuring expert-led, hands-on workshops, training sessions, and talks on cutting-edge AI topics and tools, from data science and machine learning to generative AI to LLMOpsNever miss an episode, subscribe now!
In this episode of ODSC’s Ai X Podcast, we speak with Ben Colman, who is the CEO and Co-Founder of Reality Defender, an award-winning deepfake detection platform helping enterprises flag fraudulent users and content. Over the past 15 years, Ben has scaled multiple companies at the intersection of cybersecurity and data science.Our discussion will go in-depth into the realm of deepfakes, including real-time deepfakes, fake phone calls, and how the everyday person is at risk of being scammed by deepfakes.Questions:1. Tell me a bit more about yourself and your background.2. Why did you create Reality Defender? What spurred your passion here?3. Generative AI has been a huge proponent of deepfakes - can you tell us a bit more about the current state of deepfakes and Gen AI?4. If we don’t address deepfakes now, what are some major issues we could face?5. Can you give some examples of how deepfakes have caused problems?6. How has Reality Defender addressed deepfakes? Can you provide some examples or case studies?7. How can the everyday person - aka someone not AI or data science savvy - protect themselves from deepfakes?8. Why should the everyday person be concerned about deepfakes?9. What’s the current state of political regulation around deepfakes? Do you think elected officials are doing enough? I read that you spoke to a Senate Committee in April about this, so I’m guessing you’re passionate here too?10. Is there anything else you’d like to add?Show Notes:Reality Defender:- Website: https://www.realitydefender.com/- Jobs board: https://www.realitydefender.com/open-positions- Blog: https://www.realitydefender.com/blogBen Colman:- Reality Defender bio: https://www.realitydefender.com/team-members/ben-colman- LinkedIn: https://www.linkedin.com/in/benpcolman/In the news:- Ben’s Congressional testimony summary: https://www.realitydefender.com/blog/ben-colmans-congressional-testimony-on-election-deepfakes- Congressional testimony full video: https://www.youtube.com/watch?v=1iodylhNdcU- Funding round: https://techcrunch.com/2023/10/17/reality-defender-raises-15m-to-detect-text-video-and-image-deepfakes/This episode was sponsored by: Ai+ Training https://aiplus.training/ Home to hundreds of hours of on-demand, self-paced AI training, ODSC interviews, free webinars, and certifications in in-demand skills like LLMs and Prompt EngineeringAnd created in partnership with ODSC https://odsc.com/ The Leading AI Training Conference, featuring expert-led, hands-on workshops, training sessions, and talks on cutting-edge AI topics and tools, from data science and machine learning to generative AI to LLMOpsNever miss an episode, subscribe now!
In this episode, Lukas Biewald, the founder and CEO of Weights & Biases, one of the goto platforms for machine learning, joins us to discuss Best Practices for GenAI in Production. Prior to founding Weights & Biases, Lukas co-founded Figure Eight (formerly CrowdFlower), a pioneer in the ML data-labeling space that was subsequently acquired by Appen in 2019. This discussion will focus on the best practices for integrating GenAI into production, with a particular focus on the importance of robust evaluation frameworks and key metrics for tracking performance. Lukas will also share insights on using Weights & Biases and the newest platform, Weave, for model evaluation and strategies for effective model monitoring. Last, but not least, he'll touch on the non-deterministic nature of GenAI models, share his perspective on the current AI landscape, and share advice on running a startup. SHOW NOTESLearn more about the platforms:Weave: https://wandb.github.io/weave/ Weights and Bias: https://github.com/wandbBlog: https://wandb.ai/fully-connected Listen to Lukas’ Podcast: https://podcasts.apple.com/us/podcast/gradient-dissent-conversations-on-ai/id1504567418Learn more about the Guardrails project:https://github.com/outlines-dev/outlineshttps://github.com/guardrails-ai/guardrailsLearn more about Edward Rutherfurd’s Books:https://www.edwardrutherfurd.com/edward-rutherfurd-books.htmlTopics:1. Introduction2. Tell us about your journeyBest practices for getting GenAI into Production– Set the context. Tell us about WandB– What are some of the best practices for getting into GenAI right now?– How are companies handling model evaluation? – Tell us about Weave – What about model monitoring? – How are companies handling the non-deterministic nature of GenAI models?– What part of GenAI is overhyped?– What is underhyped?– Is the stack going to get more complex and are better tools needed? Startups and Investing – What are some of the biggest lessons you've learned as a successful entrepreneur?– What do you look for in startups? – When founders ask you for advice, what questions should they ask more?– How do you hire great engineering talent in a very competitive market ?– What do you like to read in your downtime?– How can people follow your work?This episode was sponsored by: Ai+ Training https://aiplus.training/ Home to hundreds of hours of on-demand, self-paced AI training, ODSC interviews, free webinars, and certifications in in-demand skills like LLMs and Prompt EngineeringAnd created in partnership with ODSC https://odsc.com/ The Leading AI Training Conference, featuring expert-led, hands-on workshops, training sessions, and talks on cutting-edge AI topics and tools, from data science and machine learning to generative AI to LLMOpsNever miss an episode, subscribe now!
This is a previously recorded interview from early 2023 with one of computer science's most influential pioneers that we are rereleasing on our podcast platform for a wider audience. In this episode, the incredibly accomplished Michael I. Jordan joins us for a discussion on learning-aware mechanism design. Michael, currently a Distinguished Professor at the University of California, Berkeley, has made significant contributions to the field of AI throughout his extensive career. In 2016, he was named the "most influential computer scientist" worldwide in Science magazine. Michael is a member of many distinguished associations including the American Association for the Advancement of Science. He is also the recipient of many awards, including the Ulf Grenander Prize from the American Mathematical Society (2021) and the IEEE John von Neumann Medal (2020). This episode will delve into learning-aware mechanism design, a subfield of mechanism design, a branch of economics that studies the design of rules and procedures for decision-making in strategic settings with the goal of creating mechanisms that are more efficient, fair, and robust by incorporating insights from machine learning.Topics:- Guest’s professional background and journey to current position- Guest’s definition and understanding of machine learning- The history of machine learning - How your thinking has evolved since then due to advance in the field of ML and the economic impact of COVID on the behavior of individuals and companies- The importance of two-sided marketplace for agents - Limitations of the recommendation system- How do we solve data equality problems in market driven decision algorithms- Ways machine learning can improve decision-making under uncertainty- Market dynamic of scarcity when it comes to the designing system - The role of government regulation for AI - Why federated learning is a necessity- The possible development of Generative AI applications into two sided markets or ad revenue-based business model- The models that work best- Advice about the future and direction of AI Show Notes:More about Michael I. Jordan, PhD:https://www.linkedin.com/in/michael-jordan-767032125/https://www2.eecs.berkeley.edu/Faculty/Homepages/jordan.htmlhttps://scholar.google.com/citations?user=yxUduqMAAAAJ&hl=en This episode was sponsored by: Ai+ Training https://aiplus.training/ Home to hundreds of hours of on-demand, self-paced AI training, ODSC interviews, free webinars, and certifications in in-demand skills like LLMs and Prompt EngineeringAnd created in partnership with ODSC https://odsc.com/ The Leading AI Training Conference, featuring expert-led, hands-on workshops, training sessions, and talks on cutting-edge AI topics and tools, from data science and machine learning to generative AI to LLMOps Never miss an episode, subscribe now!
In this episode, Dr. Mike Stonebraker, a rare breed of incredibly successful academic and entrepreneur, joins us to discuss the future of databases and how to raise funding for a startup.Dr. Stonebraker has been a pioneer of database research and technology for more than a quarter century. He was the main architect of both the INGRES relational database, and the hugely popular POSTGRES relational database, which he developed at UC Berkeley during his 25 years as a Professor of Computer Science. After he moved to MIT, he co-architected the C-Store column-oriented DBMS, the H-Store OLTP DBMS, the SciDB array DBMS, and the Data Tamer integration engine and the Kyrix (kyrix) visualization system. He also developed Aurora, a real-time event processing platform which revolutionized the way businesses analyze streaming data and later became Streambase in the early 2000s. Mike is also the founder of 11 venture-capital backed startups, including companies like the very successful Vertica and VoltDB.In recognition of his impressive contributions, he’s been awarded some of the highest academic honors, including the IEEE John Von Neumann prize and the ACM Turing Award in 2014 to mention just a few.Topics:1- Guest introduction and early work on databases at UC Berkeley2- Motivation for Postgres and its extendable features3- The Aurora/Borealis/StreamBase - early streaming platforms4- Eliminating the need for traditional OS components like Linux and Kubernetes5- DBOS, a database operating system, description and motivation6- The advantage of Function as Service for Databases on the Cloud7- Dealing with race conditions and Heisenbugs8- The shortcomings of the Modern Data Stack9- Time traveling and debugging with DBOS10- The security advantages of using DBOS compared to traditional OS stacks11- Building resilience against ransomware attacks and other security threats12- Discuss the challenges and potential benefits for companies adopting DBOS 13- Function as a service model and only paying for what you use.14- Whats next for DBOSStartups and Raising Capital- Secrets of assembled high-performing teams for startup ventures. - Leading by example and lead by being right- The biggest hurdles faced in launching new startups- How to negotiate deals with a VC and the VC "Rock Fetch"SHOW NOTES:Learn more about Dr. Mike Stonebrakerhttps://en.wikipedia.org/wiki/Michael_StonebrakerDBOS Project pagehttps://dbos-project.github.io/Learn more aboutHOPORA.iohttps://dbos-project.github.io/https://hopara.io/Learn more about Ingreshttps://en.wikipedia.org/wiki/Ingres_(database)Learn more about Postgreshttps://www.postgresql.org/Learn more about the Aurora and Borealis Stream Processing Engineshttps://homes.cs.washington.edu/~magda/borealis-book.pdfLearn more about voltDB https://www.voltactivedata.com/company/Learn more about Vertica https://www.vertica.com/Learn more about Streambase: https://marketswiki.com/wiki/StreamBase_Systems Learn more about Heisenbug:https://en.wikipedia.org/wiki/HeisenbugLearn more about time travel debugging: https://docs.dbos.dev/cloud-tutorials/timetravel-debuggingLearn more about the modern data stack https://www.fivetran.com/blog/what-is-the-modern-data-stackThis episode was sponsored by: Ai+ Training https://aiplus.training/ Home to hundreds of hours of on-demand, self-paced AI training, ODSC interviews, free webinars, and certifications in in-demand skills like LLMs and Prompt EngineeringAnd created in partnership with ODSC https://odsc.com/ The Leading AI Training Conference, featuring expert-led, hands-on workshops, training sessions, and talks on cutting-edge AI topics and tools, from data science and machine learning to generative AI to LLMOpsNever miss an episode, subscribe now!
In this episode, Luca Antiga, CTO of Lightning AI and ODSC East 2024 speaker, joins us to discuss Small Language Models (SLMs).Lightning AI, well regarded for providing tools and platforms that streamline workflows for machine learning engineers and AI researchers, was founded by the creators of the very popular open-source framework PyTorch Lightning.Luca himself was an early contributor to the PyTorch core library and co-authored the book “Deep Learning with PyTorch”.In this interview, we’ll explore the differences between SLMs and Large Language Models (LLMs), examine the importance of data quality in training SLMs, and talk about key SLM frameworks such as TinyLama.We’ll also touch on the process of combining SLMs with Retrieval Augmented Generation (RAG), address hallucinations within SLMs in particular, and how to deploy SLMs into production. SHOWNOTE TOPICS:1- Guest Introduction2- Explanation of Small Language Models (SLMs) is and how they differ from Large Language Models (LLMs)?3- How SLMs are trained and the importance of data quality4- Continued pre-training vs fine-tuning5- Incontext learning for LLMs6- Benchmarking LLMS with MMLU7- Small Language Model (SLM) frameworks such as MiniCPM, TinyLlama, and LitGPT8- Combining SLMs with Retrieval Augmented Generation (RAG) and fine-tuning 9- Why SLMs are more prone to hallucinations than LLMs and how to solve10- LLMs and SLMs in production - minimizing risks and maximizing value 11- The problem of over optimizing on AI solutions 12- Practical applications of SLMs, 13- Small Language Model (SLM) training challenges14- Overview of Lightning studio15- AI Agents and challenges16- Compute access from single to multi-GPUs17- Using lightning studio to easily switch between compute setups18- Quick fire questions: - Will more foundational LLMs emerge? - Open source vs closed-scouce LLM - Artificial General Intelligence? - In this fast paced environment what should practitioners focus on? - What are companies getting wrong with AI now? - What are companies getting right with AI?SHOW NOTES:Learn more about Luca Antiga, CTO at Lightning AI: https://www.linkedin.com/in/lantiga/?originalSubdomain=itLearn more about Lightning AI and PyTorch Lightning:https://lightning.ai/https://lightning.ai/docs/pytorch/stable/https://github.com/Lightning-AIhttps://pytorch.org/Learn more about Small Language Modelshttps://opendatascience.com/the-growth-of-small-language-models/https://opendatascience.com/6-small-language-models-to-get-the-job-done-with-ease/MMLU (Multi-task Language Understanding) Benchmark:https://arxiv.org/pdf/2009.03300MiniCPM: Unveiling the Potential of Small Language Models with Scalable Training Strategieshttps://ar5iv.labs.arxiv.org/html/2404.06395Small Language Model Frameworks:https://github.com/jzhang38/TinyLlamahttps://github.com/Lightning-AI/litgptWhy Larger Language Models Do In-context Learning Differently? https://arxiv.org/abs/2405.19592Learn more about RAG:https://github.blog/2024-04-04-what-is-retrieval-augmented-generation-and-what-does-it-do-for-generative-ai/Learn more about dottxt:https://dottxt.co/Learn more about structured text generation:https://github.com/outlines-dev/outlinesLearn more about HyperTalk:https://en.wikipedia.org/wiki/HyperTalkLearn more about crewAI:https://github.com/joaomdmoura/crewAILearn more about Lightning AI Studios:https://lightning.ai/studiosLearn more about Concurrent Versions System:https://en.wikipedia.org/wiki/Concurrent_Versions_System
In this episode, we take a deep dive into the fascinating universe of World Models. We'll unpack how they differ from traditional, purely predictive models, and explore key characteristics of World Models and how they empower AI agents to revolutionize the AI landscape. We'll delve into the limitations of passive observation and into the power of intervention, expose the pitfalls of curve fitting in machine learning, and explore the "shadow problem" that makes building World Models from data so challenging.Later, we'll discuss the powerful role of inductive biases in making World Models tractable. We'll also unpack the core principles of cutting-edge research areas like causal AI, generative flow networks, and active inference, and explore why these advancements are crucial for achieving artificial general intelligence (AGI).Finally, Andre will share his startup journey and explain why he believes "a world model is all you need.”Andre Franca is the co-founder and CTO of connectedFlow, which is developing the next generation of AI co-pilots. His previous roles include the VP of R&D at causaLens, and executive director at Goldman Sachs. Andre received his PhD in theoretical physics from the University of Munich.Topics:- Guest introduction and background- Understanding world models and how they differ from purely predictive models- The history behind world models and early research - The key characteristics of world models- How AI agents will take the AI world by storm - Deep dive into how world models differ from traditional predictive models- Passive observation versus intervention in the context of world models- The main disadvantages of curve fitting in machine learning - Why is it challenging to build World Models from data?- How the analogy of 2D shadows and 3D objects relate to the problem of tractability in World Models- What are inductive biases and how do they help in making World Models tractable- Core principles and significance of causal AI, generative flow networks, and active inference- Why are World Models essential for achieving artificial general intelligence (AGI)?- Tell us about your startup and why a ”world model is all you need”Show Notes:More about Andre Franca:https://www.linkedin.com/in/francaandre/Resources:World Model paper by David Ha & Jurgen Smithhuber where they explore building generative neural network models of popular reinforcement learning environmentshttps://arxiv.org/abs/1803.10122NeurIPS 2018 World Models workshop - Can agents learn inside of their own dreams?https://worldmodels.github.io/ Open AI GYM (A toolkit for developing and comparing reinforcement learning algorithms ) and Gymnasiumhttps://github.com/Farama-Foundation/GymnasiumAtari Game on GYM Retro - a platform for reinforcement learning research on games including 70 Atari games and 30 Sega games and over 1,000 games across a variety of backing emulatorshttps://openai.com/index/gym-retro/This episode was sponsored by: Ai+ Training https://aiplus.training/ Home to hundreds of hours of on-demand, self-paced AI training, ODSC interviews, free webinars, and certifications in in-demand skills like LLMs and Prompt EngineeringAnd created in partnership with ODSC https://odsc.com/ The Leading AI Training Conference, featuring expert-led, hands-on workshops, training sessions, and talks on cutting-edge AI topics and tools, from data science and machine learning to generative AI to LLMOpsNever miss an episode, subscribe now!
How to Evaluate Large Language Models and RAG ApplicationsIn this episode, Pasquale Antonante, Co-Founder & CTO of Relari AI, joins us to discuss evaluation methods for LLM and RAG applications. Pasquale has a PhD from MIT, where he focused on the reliability of complex AI systems. At Relari AI, they are building an open-source platform to simulate, test, and validate complex generative AI (GenAI) applications.During the interview, we’ll discuss Relari AI's innovative approach to improving generative AI and RAG applications, which were inspired by the testing methodologies from the autonomous vehicle industry. We’ll cover topics like the complexity of GenAI workflows, the challenges in evaluating these systems, and various evaluation methods such as reference-based, and synthetic data-based approaches. We’ll also explore metrics like precision, recall, faithfulness, and relevance, and compare GPT auto-evaluators with simulated user feedback.Finally, we'll highlight Relari's continuous-eval open-source project and the future of leveraging synthetic data for LLM finetuning.Topics- Guest background and about the startup - Relari AI- What the LLM industry can learn from the autonomous vehicle space- What do companies view as the biggest challenge to the adoption of generative AI?- Why are GenAI application workflows and pipelines so complex?- Explanation of how Retrieval-Augmented Generation (RAG) works and its benefits over traditional generation models- The challenges of evaluating these workflows- Different ways to evaluate LLM pipelines - Reference-free, reverence-based, and using synthetic data based evaluation for LLMs and RAG- Measuring precision, recall, faithfulness, relevance, and correctness in RAG systems- The key metrics used to evaluate RAG pipelines- Semantics metrics and LLM-based metrics- GPT auto-evaluators versus the advantages of simulated user feedback evaluators- The role human evaluation plays in assessing the quality of generated text- The continuous-eval open-source project and various metrics container therein- Leveraging synthetic data to improve LLM finetuning- What’s next for Relari?Show Notes:Learn more about Pasquale:https://www.linkedin.com/in/pasquale-antonante/https://www.mit.edu/~antonap/https://scholar.google.com/citations?user=7Vvpd-YAAAAJ&hl=itLearn more about Relarihttps://www.relari.ai/https://github.com/relari-ai/continuous-evalTask-Aware Risk Estimation of Perception Failures for Autonomous Vehicleshttps://arxiv.org/abs/2305.01870BM25 https://en.wikipedia.org/wiki/Okapi_BM25Precision, Recall, F1 scorehttps://scikit-learn.org/stable/modules/generated/sklearn.metrics.precision_recall_fscore_support.htmlA Practical Guide to RAG Pipeline Evaluation (Part 1: Retrieval)https://blog.relari.ai/a-practical-guide-to-rag-pipeline-evaluation-part-1-27a472b09893https://blog.relari.ai/a-practical-guide-to-rag-evaluation-part-2-generation-c79b1bde0f5dHow important is a Golden Dataset for LLM evaluation?https://blog.relari.ai/how-important-is-a-golden-dataset-for-llm-pipeline-evaluation-4ef6deb14dc5Case Study: Reference-free vs Reference-based evaluation of RAG pipelinehttps://blog.relari.ai/case-study-reference-free-vs-reference-based-evaluation-of-rag-pipeline-9a49ef49866cThis episode was sponsored by: Ai+ Training https://aiplus.training/ Home to hundreds of hours of on-demand, self-paced AI training, ODSC interviews, free webinars, and certifications in in-demand skills like LLMs and Prompt EngineeringAnd created in partnership with ODSC https://odsc.com/ The Leading AI Training Conference, featuring expert-led, hands-on workshops, training sessions, and talks on cutting-edge AI topics andNever miss an episode, subscribe now!
In this episode, we talk with Beatrice Botti from DoubleVerify, a leading provider of digital ad verification solutions. Beatrice leads DoubleVerify’s privacy, data, and AI governance strategy as their Chief Privacy Officer. She is also a licensed attorney in Massachusetts and New York with extensive in-house counsel experience in the tech industry. Join us for a discussion on the key pillars of strong AI governance and how responsible AI practices can differentiate products and brands, demystify legal frameworks, and explain why data and AI governance are crucial for organizational success.SHOW NOTESLearn more about Beatrice Botti: www.linkedin.com/in/beatricebotti/Relevant and useful links:Scarlett Johansson wants answers about the ChatGPT voice that sounds like 'Her': https://www.npr.org/2024/05/20/1252495087/openai-pulls-ai-voice-that-was-compared-to-scarlett-johansson-in-the-movie-herMore about the movie “Her”: https://www.imdb.com/title/tt1798709/More about 2001 “A Space Odyssey”: https://www.imdb.com/title/tt0062622/More about “Mission: Impossible - Dead Reckoning Part One”: https://www.imdb.com/title/tt9603212/More about US Patents: https://www.uspto.gov/SHOW NOTES QUESTIONSIntroduction to our guest and her backgroundYour primary responsibilities regarding AI governance and risk managementAI governance and the risk of third party vendor AI solutionsThe unique AI governance challenges across sectors like healthcare, wellbeing, and advertisingApplying AI and risk governance accountability across the organizationHow we can equip participants with practical tools for navigating challenges like bias, data privacy, copyright, and risk managementHow watching AI movies with your co-workers can get them involved and interested in AIWhat are the key pillars of a strong AI governance framework?How can organizations establish clear lines of accountability for AI development and deployment?How can responsible AI practices differentiate not just products, but also an organization's brand identity?How companies and startups can understand the new legal frameworks impacting AI such as the EU AI Act and similar AI lawsVoice and image cloning and legal issues of privacy and copyright pertaining to multimodal modelsHow patent laws apply to AI and non-human inventorsWhat are the "true harms and risks" of AI systemsHow can understanding data and AI governance empower data scientists?How building trustworthy AI systems differentiate products and brands in the marketplaceWhy is turning data and AI governance into a "secret weapon" such a powerful approach?Does AI-generated content infringe on the copyrights of the material it was trained on?Who within an organization should take the lead on implementing AI governance and risk management practices?How does your legal background influence your approach to AI governance and risk management?This episode was sponsored by: Ai+ Training https://aiplus.training/ Home to hundreds of hours of on-demand, self-paced AI training, ODSC interviews, free webinars, and certifications in in-demand skills like LLMs and Prompt EngineeringAnd created in partnership with ODSC https://odsc.com/ The Leading AI Training Conference, featuring expert-led, hands-on workshops, training sessions, and talks on cutting-edge AI topics andNever miss an episode, subscribe now!
In this episode our guest is Argyro (Iro) Tasitsiomi, Head of Investments Data Science at T. Rowe Price. Iro has extensive experience in AI and data science within the financial markets. Early in her career Iro held significant roles focusing on Quantitative Research & Risk Management, where she developed advanced trading strategies and econometric forecasting tools. Later, as a Data Scientist in Investment Banking, she led initiatives in business intelligence, market intelligence, and machine learning infrastructure development. Her work has spanned various domains, including asset portfolio optimization, enterprise risk management, and mergers and acquisitions.She joins us on the podcast to discuss her professional journey at leading financial institutions such as Goldman Sachs, BlackRock, Prudential, and T. Rowe Price, and explore how AI is transforming various aspects of financial markets. We'll discuss advancements in financial modeling, the opportunities and risks of integrating AI into financial analysis, and the impact of fake data on market stability. Additionally, we'll cover the importance of quality data, the enduring value of human expertise, and emerging skills in the era of AI.Topics:Career Journey: Iro’s professional journey working at top financial institutions such as Goldman Sachs, BlackRock, Prudential, and T. Rowe PriceEarlier Modeling Techniques: time series, trading strategies and risk modelingAI Applications in Financial Markets: How is AI being applied across different areas of the financial markets today?Fundamental Analysts in the Age of AI: How can fundamental analysts leverage their skills alongside AI tools to create a more comprehensive approach to financial analysis?Advancements in Financial Modeling: With the rise of AI, have modeling techniques advanced beyond traditional methods like Monte Carlo simulations and the Black-Scholes models?Opportunities and Risks of AI: What are the opportunities and risks of integrating AI into fundamental financial analysis?Impact of Fake Data and Fake News: Exploring the influence of fake data and fake news on social media and its impact on financial markets.Importance of Quality Data: The significance of quality data for AI in financial markets and how the evolving data landscape is shaping robust quantitative models.Crowding: In traditional finance, popular trading strategies often become less effective as more market participants adopt them (crowding). Looking ahead, with the rise of AI and everyone potentially having access to similar LLM tools, do we foresee a similar phenomenon of "crowding" occurring?Synthetic Data Usage: Discussing the use of synthetic data in financial market modeling and analysis.Economic forecasting: The potential benefits and challenges associated with using AI for this purposeAstrophysics: Iro’s research focused on large-scale structure formation in the universe using cosmological simulations.Show Notes:Learn more about Iro Tasitsiomi:https://www.linkedin.com/in/argyrotasitsiomi/Bits and Brainwaves Newsletter: https://www.linkedin.com/newsletters/bits-brainwaves-7151965921455046657/Argyro (Iro) Tasitsiomi's Google Scholar Astrophysic Citations: https://scholar.google.com/citations?user=-dtAtwIAAAAJ&hl=enChronos: Learning the Language of Time Series:https://arxiv.org/abs/2403.07815Monte Carlo methods in finance:https://en.wikipedia.org/wiki/Monte_Carlo_methods_in_financeStochastic processhttps://en.wikipedia.org/wiki/Stochastic_processBlack swan theoryhttps://en.wikipedia.org/wiki/Black_swan_theoryBlack-Scholes Model:https://www.investopedia.com/terms/b/blackscholes.asp#:~:text=The%20Black%2DScholes%20model%2C%20aka,free%20rate%2C%20and%20the%20volatility.Latent Dirichlet allocation (LDA) for Topic Modeling:https://en.wikipedia.org/wiki/Latent_Dirichlet_allocationBacktesting: Definition, How It Works, and Downsides:https://www.investopedia.com/terms/b/backtesting.aspCUSIP Numbers:https://www.investopedia.com/terms/c/cusipnumber.aspSynthetic data: https://en.wikipedia.org/wiki/Synthetic_dataModel Collapse Demystified: https://arxiv.org/html/2402.07712v1On cosmology, investment strategies and ergodicity:https://www.linkedin.com/pulse/cosmology-investment-strategies-ergodicity-tasitsiomi-phd-aec0fThis episode was sponsored by: Ai+ Training https://aiplus.training/ Home to hundreds of hours of on-demand, self-paced AI training, ODSC interviews, free webinars, and certifications in in-demand skills like LLMs and Prompt EngineeringAnd created in partnership with ODSC https://odsc.com/ The Leading AI Training Conference, featuring expert-led, hands-on workshops, training sessions, and talks on cutting-edge AI topics andNever miss an episode, subscribe now!
In this episode, Professor Noah Giansiracusa, a tenured associate professor of mathematics and data science at Bentley University, joins us to discuss AI’s impact on the news.Professor Noah’s research interests range from algebraic geometry to machine learning to empirical legal studies. He is the author of How Algorithms Create and Prevent Fake News (2021) and has written op-eds for Barron's, Boston Globe, Wired, Slate, and Fast Company.During this interview, Professor Noah Giansiracusa will discuss the benefits and drawbacks of AI as it relates to the creation and dissemination of news. He’ll explore the economic pressures on newsrooms and how AI might provide cost-effective solutions, while also examining the challenges of ensuring factual accuracy in an AI-powered news landscape.Topics:Overview of guest’s backgroundHow AI will impact the newsThe new skills journalists will need to develop and how newsrooms can adapt their workforce strategiesAI’s potential (or lack thereof) to provide cost-effective solutions for these smaller, local news organizations struggling to compete and stay relevant Ways local and small news organizations can make use of AIAutomation and real-time AI-driven journalismUsing AI to fact-check the news The long-term impacts of AI on how people consume and understand the news and avoiding filter bubbles and echo chambersHow AI can help improve the fairness, accuracy, and trustworthiness of news, and the challenges involvedPublic awareness and perception of AI in the newsThe role AI might play in the creation and dissemination of misinformationUpcoming USA and Europe elections: misinformation generation and misinformation detectionWatermarking, audio fakes, and deepfake detectionWrapup and how to follow our guestShow Notes: Learn more about Noah Giansiracusa, PhD here:https://www.noahgian.com/Get his book here: https://link.springer.com/book/10.1007/978-1-4842-7155-1Learn more about Watermarking AI here: https://www.brookings.edu/articles/detecting-ai-fingerprints-a-guide-to-watermarking-and-beyond/Learn more about The Coalition for Content Provenance and Authenticity here:https://c2pa.org/This episode was sponsored by: Ai+ Training https://aiplus.training/ Home to hundreds of hours of on-demand, self-paced AI training, ODSC interviews, free webinars, and certifications in in-demand skills like LLMs and Prompt EngineeringAnd created in partnership with ODSC https://odsc.com/ The Leading AI Training Conference, featuring expert-led, hands-on workshops, training sessions, and talks on cutting-edge AI topics.Never miss an episode, subscribe now!
In this episode of the Ai X Podcast, Bob Foreman, Lead Software Engineering LexisNexis Risk Solutions, joins us to discuss the High-Performance Computer Cluster (HPCC) project, an open-source, massive parallel-processing computing platform for data processing and analytics.Bob has spent more than a decade working with HPCC Systems and with the Enterprise Control Language as a course developer and trainer. Not only is he a highly experienced developer, but he is also the designer of the HPCC Systems online training courses and is the senior instructor for all classroom and remote-based training.Join him for a deep dive into the HPCC project to discover how it simplifies complex data analysis at scale and why it’s an ideal tool for students, startups, or companies exploring or running POC for large-scale data-intensive computing.Topics:Guest Background and Professional DevelopmentOverview of LexisNexis as a company Guest current role What high-speed data engineering involves and why it's important in big data solutionsMain components of the HPCC Systems platformWhere the HPCC Systems platform fits in the data landscapeThe role of Thor, Roxie, and ECL in the platform Example of how ECL (Enterprise Control Language) can be used to manipulate and analyze large datasetsHow Roxie enhances the performance of real-time data querying and analysisHow to get started with HPCC's open-source massive parallel system. Working with small sets of data before getting to large data setsThe Machine Learning Library native to HPCCHow the HPCC platform fits into the latest trends of Machine Learning and Generative AI HPCC-related events in 2024ODSC Workshops and how HPCC community initiativesHow to follow HPCC updatesShow Notes:Learn more about Bob Foreman: https://www.linkedin.com/in/bobforeman/ Learn more about the HPCC Platform: https://github.com/hpcc-systems/HPCC-Platform |https://hpccsystems.com/about/#PlatformHPCC call ECL bundles: https://github.com/hpcc-systems/ecl-bundlesHPCC Systems Machine Learning Library: https://hpccsystems.com/download/free-modules/hpcc-systems-machine-learning-library/Bob’s Educational ResourcesBob’s online course: https://hpccsystems.com/training/free-online-learning-with-hpcc-systems/HPCC community initiatives: https://www.missingkids.org/ourwork/ncmecdataThis episode was sponsored by: Ai+ Training https://aiplus.training/ Home to hundreds of hours of on-demand, self-paced AI training, ODSC interviews, free webinars, and certifications in in-demand skills like LLMs and Prompt EngineeringAnd created in partnership with ODSC https://odsc.com/ The Leading AI Training Conference, featuring expert-led, hands-on workshops, training sessions, and talks on cutting-edge AI topics andNever miss an episode, subscribe now!
In this episode, we speak with Dr. Jon Krohn about the life cycle of open-source LLMs. Jon is a co-founder and chief data scientist at the machine learning company Nebula. He is the author of the book Deep Learning Illustrated, which was an instant #1 bestseller and was translated into seven languages. He is also the host of the fabulous SuperDataScience podcast, the data science industry’s most listened-to podcast. An incredible instructor and speaker, Jon’s workshops at ODSC conferences and other events are always one of our most popular.Topics:1. Guest Introduction2. Definition of an open source LLMs and what it means to be truly open source3. The importance of LLM weights and neural networks architecture for training4. Transformer architecture5. Apple expanding their AI team6. What do I need to train or fine-tune an LLM7. Key libraries for fine-tunning an LLM8. The LoRA (Low-Rank Adaptation) technique for efficiently fine-tuning large language models9. Testing and evaluating LLMs prior to deploying in production10. Retrieval Augmented Generation (RAG)11. Deploying LLM to production12. How to keep inference costs down13. How can people follow Jon’s content (see show notes also)Show Notes: More about Jon:LinkedInJon’s YouTube ChannelJon’s Monthly NewsletterTools and Resources:Michael Nielsen's eBook on Neural Networks and Deep Learning PyTorch Lightning is the deep learning framework Hugging Face Transformers LibraryVicuna: An Open-Source Chatbot LoRA: Low-Rank Adaptation of Large Language ModelsSDS 674: Parameter-Efficient Fine-Tuning of LLMs using LoRA (Low-Rank Adaptation)Unsloth for finetuning Llama 3, Mistral & GemmaPhoenix: an open-source AI Observability & Evaluation toolODSC Podcast with Amber Roberts on Phoenix and troubleshooting LLMsWeights & BiasesThis episode was sponsored by: Ai+ Training https://aiplus.training/ Home to hundreds of hours of on-demand, self-paced AI training, ODSC interviews, free webinars, and certifications in in-demand skills like LLMs and Prompt EngineeringAnd created in partnership with ODSC https://odsc.com/ The Leading AI Training Conference, featuring expert-led, hands-on workshops, training sessions, and talks on cutting-edge AI topics andNever miss an episode, subscribe now!
Today on our podcast, we're thrilled to have Hagay Lupesko, Senior Director of Engineering in the Mosaic AI team at Databricks and one of the key architects behind Databricks' groundbreaking large language model, DBRX. Previously Haguy was the VP of Engineering at Moscia ML, which was acquired by Databricks in 2023. Hagay has also held AI engineering leadership roles at Meta, AWS, and GE Healthcare. Our topic today is the the open-source DBRX large language model, which stands out in the LLM AI landscape due to its innovative use of the Mixture of Experts (MoE) architecture. This architecture allows DBRX to efficiently scale by distributing tasks across 64 combinable experts, allowing the model to select the most suitable set of internal configurations from a pool of experts for each specific task. This results in faster processing and potentially better performance compared to traditional LLM architectures. We'll be exploring the inspiration behind DBRX, the advantages of Mixture of Experts, and how it positions DBRX within the larger LLM landscape.Podcast TopicsDBRX backstory and Databricks' Mosaic AI Research team Inspiration for the open Source DBRX LLMs, and what gap it fills Core features of DBRX that distinguish it from other LLMs (LLMs)Mixture of Experts - Mixture-of-experts (MoE) architecture How Mixture-of-Experts (MoE) architecture enhances LLMComparison to other MoE models like Mixtral-8x7B and Grok-1 Advanced DBRX Architecture features? Rotary Position Encodings (RoPE): Gated Linear Units (GLU): Grouped Query Attention (GQA): GPT-4 Tokenizer (tiktoken): Types of tasks and applications Mixture-of-Experts models are particularly well-suited forRAG (Retrieval Augmented Generation ) and LLMs for Enterprise applicationsHow open source LLM MoE Models like DBRX are being used by Databricks customersWhat’s next in 2024 for DBRX, mixture-of-experts models, and LLMs in generalKeep up with the evolving AI field? Show Notes: Learn more about and connect with Hagay Lupesko: https://www.linkedin.com/in/hagaylupesko/ Learn more about DBRX, its use of Mixture of Experts, and scaling laws:Introducing DBRX: A New State-of-the-Art Open LLMhttps://www.databricks.com/blog/introducing-dbrx-new-state-art-open-llm Mixture of Experts https://opendatascience.com/what-is-mixture-of-experts-and-how-can-they-boost-llms/#google_vignette Lost in the Middle: How Language Models Use Long Contextshttps://cs.stanford.edu/~nfliu/papers/lost-in-the-middle.arxiv2023.pdf HotpotQA: A Dataset for Diverse, Explainable Multi-hop Question Answeringhttps://hotpotqa.github.io Scaling Lawshttps://en.wikipedia.org/wiki/Neural_scaling_law Training Compute-Optimal Large Language Modelshttps://arxiv.org/pdf/2203.15556 Advance Architecture Features & Techniques in DBRX Rotary Position Encodings (RoPE): Gated Linear Units (GLU): Grouped Query Attention (GQA): GPT-4 Tokenizer (tiktoken): This episode was sponsored by: Ai+ Training https://aiplus.training/ Home to hundreds of hours of on-demand, self-paced AI training, ODSC interviews, free webinars, and certifications in in-demand skills like LLMs and Prompt EngineeringAnd created in partnership with ODSC https://odsc.com/ The Leading AI Training Conference, featuring expert-led, hands-on workshops, training sessions, and talks on cutting-edge AI topics andNever miss an episode, subscribe now!
Vrushali Sawant is a data scientist with SAS's Data Ethics Practice (DEP), steering the practical implementation of fairness and trustworthy principles into the SAS platform. She regularly writes and speaks about practical strategies for implementing trustworthy AI systems on sas.com and external platforms. With a background in technology consulting, data management, and data visualization, she has been helping customers make data-driven decisions for a decade. In this episode of ODSC’s Ai X Podcast, we speak with SAS’s Vrushali Sawant about trustworthy AI, including an overview of what it is, what a career path in trustworthy AI looks like, how data scientists can implement trustworthy AI, and what’s already being done to promote ethical AI. Questions: 1. What is the career path of a data scientist specializing in AI ethics?2. What exactly is trustworthy AI, and why is it crucial?3. How can we build AI systems that reflect societal values and avoid unintended bias?4. What practical steps can data scientists take to ensure data privacy, security, and fairness throughout the AI lifecycle?5. How can we develop models that are not only accurate but also interpretable and explainable?6. What are some unintended harms at massive scale and real-world examples of unintended harms caused by AI?7. What is the AI incidents database?8. What is the significance of data management in building trustworthy AI systems?9. What’s an example of bias and practical way to identify and mitigate potential biases in datasets?10. How can training data imbalances affect the fairness of AI models?11. What are the three stages of bias mitigation?12. What is the importance of asking the right questions at the beginning of the AI lifecycle?13. Beyond fairness metrics, what methods and can developers use to ensure fairness, model transparency, and explainability?14. Why is accountability such a critical aspect for building trust in AI systems15. What are accountability and feedback mechanisms?16. What are some ethical concerns associated with using synthetic data in various industries?17. What are key ethical guidelines that developers and organizations should follow when implementing AI solutions?18. What strategies can organizations implement to build a culture of responsible AI?19. What are the biggest hurdles in achieving trustworthy AI in practice?20. Let’s review your ODSC talk, "From Code to Trust." Learn more about Vrushali and her work:Her blog and articles: https://blogs.sas.com/content/author/vrushalisawant/Follow her posts on LinkedIn: https://www.linkedin.com/in/vrushalipsawant/SAS blogs: https://blogs.sas.com/content/SAS Viya: https://www.sas.com/en_us/software/viya.htmlLearn more about the harms and near harms caused by AI on the AI incident database and how we can use the lessons learned to prevent future bad outcomes: https://incidentdatabase.ai/ This episode was sponsored by: Ai+ Training https://aiplus.training/ Home to hundreds of hours of on-demand, self-paced AI training, ODSC interviews, free webinars, and certifications in in-demand skills like LLMs and Prompt EngineeringAnd created in partnership with ODSC https://odsc.com/ The Leading AI Training Conference, featuring expert-led, hands-on workshops, training sessions, and talks on cutting-edge AI topics andNever miss an episode, subscribe now!
In today’s episode of ODSC’s Ai X Podcast, we speak with Leondra Gonzalez, Senior Data & Applied Scientist at Microsoft, about the data science interview process in 2024.We’ll discuss the full stack of the data science interview process, including how to stand out with a resume and portfolio, questions you may hear during the interview, and how to properly follow up with the hiring manager after the interview.Topics:Questions:1. First, tell us a bit about yourself!2. You published a book not long ago - Cracking the Data Science Interview - tell us a bit about it and what spurred you to write it.3. Everyone is talking about AI now - what advice do you have for someone trying to enter the field?4. What are some ways that someone with little-to-no experience in AI can get an interview? Especially for career-shifters.5. What are some reasons that you believe people have issues with regarding the data science interview?6. What are some interview tips that you think are timeless?7. What Qs are common?8. When applying for a job in a particular industry, such as in a healthcare setting, what unique questions might arise?9. What are some newer questions that an employer might ask that you may not have heard a year ago? I ask this since the entire industry has shifted with generative AI, prompt engineering, etc?10. Before the interview, what can someone do after submitting a resume - or to be included in a cover letter - that might help them stand out to get the interview in the first place?11. What are some questions that an applicant might ask of the hiring manager that would impress them?12. What can someone do following an interview, such as a thank you email or anything else?13. Any other tips you’d like to share?Show Notes & Links:Connect with Leondra on LinkedIn here: https://www.linkedin.com/in/leondragonzalez/Her book, "Cracking the Data Science Interview": https://www.amazon.com/dp/1805120506?ref_=cm_sw_r_apan_dp_P5XHPSWHKWYFBP1DR3QS&language=en-US This episode was sponsored by: Ai+ Training https://aiplus.training/ Home to hundreds of hours of on-demand, self-paced AI training, ODSC interviews, free webinars, and certifications in in-demand skills like LLMs and Prompt EngineeringAnd created in partnership with ODSC https://odsc.com/ The Leading AI Training Conference, featuring expert-led, hands-on workshops, training sessions, and talks on cutting-edge AI topics andNever miss an episode, subscribe now!
In this episode, John Dickerson, co-founder and Chief Scientist of Arthur AI, joins us for a conversation about the shifting large language model landscape. He'll discuss emerging alternatives to established giants like OpenAI and Anthropic, and how these new players are impacting the market. He'll also explore the rise of open-source initiatives and smaller, task-specific models, tackle the challenges and benefits of specialized LLMs versus general-purpose models, and discuss the key advantages of smaller, open-source models. Finally he’ll dive into the detail of integrating new LLM models and the cost considerations associated with those models.Topics: 1. The founding story of Arthur AI, a NYC based startup 2. The Shifting LLM Landscape and emerging alternatives to established models like GPT-43. How the rise of well funded LLMs startup and especially open-source LLMs are contributing to a more diverse LLM ecosystem4. Smaller open source LLMs very larger established LLM models5. LLm costs, latency,, and performance and how these factors influence the adoption and usage of these models?6. How organizations effectively measuring and showcase the ROI for deploying LLMs7. Examples of use cases for open source LLMs such as text to SQL8. How competition drives innovation in the LLM space. 9. Open source vs closed source LLMs and the AI alignment debate around open source LLMs10. One-Size-Fits-All versus specialized LLMs versus waiting on newer improved general-purpose models11. Key advantages of using smaller, open-source LLM models in specific scenarios compared to their larger counterparts 12. Mixture of Experts Architecture13. Model Integration challenges when Integrating new AI models 14. Pros and cons of fine-tuning task-specific models15. Criteria where more expensive established LLM solutions might be justified. 16. How to balance the trade-offs between model latency and accuracy when deploying LLMs on cloud versus edge devices 16. Investing in early stage startups17. Advice for startups operating in the LLM landscape18. Machine Learning in Adversarial Environments: 19. Discuss John’s upcoming talk at ODSC East 2024Show Note LinksLearn more aboutJohn Dickerson at his websiteConnect with him on X. Learn more about Arthur AI research-driven approach and their publication library here. Arthur blog https://www.arthur.ai/blogExplore Arthur AI Bench, a tool for evaluating LLMs for production use cases, on GitHub. This episode was sponsored by: Ai+ Training https://aiplus.training/ Home to hundreds of hours of on-demand, self-paced AI training, ODSC interviews, free webinars, and certifications in in-demand skills like LLMs and Prompt EngineeringAnd created in partnership with ODSC https://odsc.com/ The Leading AI Training Conference, featuring expert-led, hands-on workshops, training sessions, and talks on cutting-edge AI topics andNever miss an episode, subscribe now!
In this episode, Sofien Kaabar will discuss the role of deep learning and machine learning for finance, through the lens of his recent book Deep Learning for Finance. You’ll explore a whole range of topics including machine learning for finance, featuring engineering, time series prediction strategies, challenges such as backtesting, overfitting, and non-stationary data, and much more!Sofien Kaabar is a machine learning expert and Institutional Market Strategist and financial Trader, specializing in Technical and Quantitative trading strategies. He is also the author of several books including Mastering Financial Pattern Recognition and the aforementioned Deep Learning for Finance. TOPICS1- The importance of feature engineering machine learning for finance2- Using deep learning for financial trading3- Deep Learning for Time Series Prediction4- The challenges of using deep learning for financial trading5- Backtesting explained and the challenges in backtesting6- Deep Reinforcement Learning for Time Series Prediction.fractional differentiation and forecasting thresholds7- The exponential error issue of time series over multiple periods8- Advanced Techniques and Strategies for financial prediction9- Ensemble models, random forest, and XGBoost 10- Financial Market Drivers and Risk Management11- Behavioral finance, cognitive bias, and emotional bias12- Getting started with deep learning for financial trading13- Avoiding the pitfall of online trading model marketplaces SHOW NOTES Learn more about Sofien Kaabar’s book here.You can also explore the code discussed in Deep Learning for Finance on github.Learn more about Sofien Kaabar and read more on his writings on his site here. Learn more about the Saint Louis Fed, an open-data source where you can download 823000 economic time series from 114 sources. here. Explore Pinescript here and MQL5 here which are specialized programming language used by traders to create trading functions, backtest their trading strategies and evaluate performance This episode was created in partnership with: Ai+ Training https://aiplus.training/ Home to hundreds of hours of on-demand, self-paced AI training, ODSC interviews, free webinars, and certifications in in-demand skills like LLMs and Prompt EngineeringAnd ODSC https://odsc.com/ The Leading AI Training Conference, featuring expert-led, hands-on workshops, training sessions, and talks on cutting-edge AI topics and Never miss an episode, subscribe now!
In this episode, James Serra, author of "Deciphering Data Architectures: Choosing Between a Modern Data Warehouse, Data Fabric, Data Lakehouse, and Data Mesh" joins us to discuss his book and dive into the current state and possible future of data architectures. James has been at the forefront of data architecture at Microsoft for the better part of the last nine years. Known for his thought leadership in the realm of Big Data and advanced analytics, James specializes in contemporary data architectures including the modern data warehouse, data lakehouse, data fabric, and data mesh. Beyond his technical achievements, James is a sought-after speaker and is a prolific voice in the data community through his blog, JamesSerra.com. Topics. 1- Motivation for writing "Deciphering Data Architectures" 2- Factors driving the emergence of modern alternatives to data warehouse3- How the author's experience as a data architect at Microsoft and background in big data and data warehousing influence the insights shared4- Design session and real-world example of how collaborative processes lead to choosing the right data architecture.5- Relational vs. Modern Data Warehouses6- Data Lake vs. Data Lakehouse and the key advantages of a data Lakehouse7- Data Fabric in Action and examples of its advantages and drawbacks..8- Data Mesh Demystified:9- Choosing the right data architecture: and MVM (Minimum Viable Mesh)10- How data architecture is evolving in the next 5-10 years?11- What makes data projects successful and what makes them fail and the changing role of the data architect12- Finally, what's next for you? Are there any new projects or books on the horizon that build upon the work you've done in "Deciphering Data Architectures"?13- ODSC talks SHOW NOTES Find “Deciphering Data Architectures” book here - https://a.co/d/3vlgmyb Get in touch with James Serra via Linkedin - https://www.linkedin.com/in/JamesSerra/ James Serra’s Blog https://www.jamesserra.com/ This episode was created in partnership with: Ai+ Training https://aiplus.training/ Home to hundreds of hours of on-demand, self-paced AI training, ODSC lightning interviews, free webinars, and certifications in in-demand skills like LLMs and Prompt EngineeringAnd ODSC https://odsc.com/ The Leading AI Training Conference, featuring expert-led, hands-on workshops, training sessions, and talks on cutting-edge AI topics and tools, from Generative AI to LLMOps Never miss an episode, subscribe now!
Comments
Top Podcasts
The Best New Comedy Podcast Right Now – June 2024The Best News Podcast Right Now – June 2024The Best New Business Podcast Right Now – June 2024The Best New Sports Podcast Right Now – June 2024The Best New True Crime Podcast Right Now – June 2024The Best New Joe Rogan Experience Podcast Right Now – June 20The Best New Dan Bongino Show Podcast Right Now – June 20The Best New Mark Levin Podcast – June 2024