Discover
Python Bytes
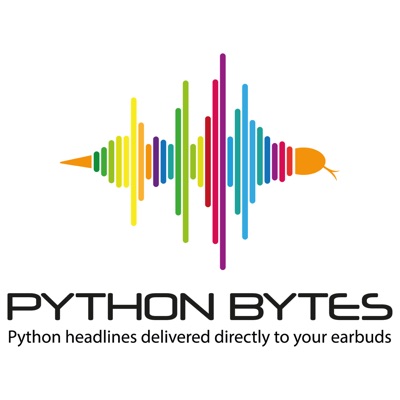
Python Bytes
Author: Michael Kennedy and Brian Okken
Subscribed: 7,956Played: 219,555Subscribe
Share
© Copyright 2016-2024
Description
Python Bytes is a weekly podcast hosted by Michael Kennedy and Brian Okken. The show is a short
discussion on the headlines and noteworthy news in the Python, developer, and data science space.
discussion on the headlines and noteworthy news in the Python, developer, and data science space.
475 Episodes
Reverse
Topics include Marimo: of Notebooks”, pytest 8.3.0 & 8.3.1 are out, Python Language Summit 2024, and bash-dungeon.
Topics include 2024 PSF Board Election & Proposed Bylaw Change Results, SATYRN: A modern Jupyter client for Mac, Incident Report: Leaked GitHub Personal Access Token, and.
Topics include Vendorize packages from PyPI, A Guide to Python's Weak References Using weakref Module, Making Time Speak, and How Should You Test Your Machine Learning Project? A Beginner’s Guide.
Topics include Joining Strings in Python: A Moment, 10 hard-to-swallow truths they won't tell you about software engineer job, My thoughts on Python in Excel, and.
Topics include Solara UI Framework, Coverage at a crossroads, and “Virtual” methods in Python classes.
Topics include , Cloud engineer gets 2 years for wiping ex-employer’s code repos, Python: Import by string with pkgutil.resolve_name(), and DuckDB goes 1.0.
Topics include Dataherald, Python's many command-line utilities, Distroless Python, and functools.cache cachetools cachebox.
Topics include NumPy 2.0 release date is June 16, Uvicorn adds multiprocess workers, pixi, and JupyterLab 4.2 and Notebook 7.2.
Topics include PostgresREST, How Python Asyncio Works: Recreating it from Scratch, Bend, and The Smartest Way to Learn Python Regular Expressions.
Topics include Git: Force push safely with and, , Being friendly: Strategies for friendly fork management, and tach.
Topics include I asked 100 devs why they aren’t shipping faster. Here’s what I learned, Python 3.13.0 beta 1 released, A theme editor for JupyterLab, and rich-argparse.
Topics include act: Run your GitHub Actions locally!, portr, Annotating args and kwargs in Python, and github badges.
Topics include Announcing py2wasm: A Python to Wasm compiler, Oven PyPI Browser, PyCharm Local LLM, and.
Topics include NumFOCUS concerns, leaping pytest debugger llm, , and PyPI has completed its first security audit.
Topics include How to Set Up Pre-Commit Hooks A step-by-step guide to installing and configuring pre-commit hooks on your project, difftastic, Quarto, and constable.
Topics include pacemaker, PyPI suspends new user registration to block malware campaign, Python Project-Local Virtualenv Management Redux, and Python Edge Workers at Cloudflare.
Topics include justpath, , LPython, and dramatic.
Topics include 🤖 On Robots.txt, niquests, Every dunder method in Python, and Lockbox.
Topics include pycountry, Does Python have pointers?, ingestr, and Make your terminal nice.
Topics include 6 ways to improve the architecture of your Python project (using import-linter), Mountaineer, Why Python's Integer Division Floors, and Hatchet.
I don't work with python, but I know that for many people developing their own solutions, apps and products and managing those products is more than relevant. I assume pip https://setapp.com/how-to/install-pip-on-mac you use as well, and it's great that there are such solutions for Mac to manage all products as easily and efficiently as possible.
Very good podcast!
sorry, but I can't with so many yawning 😂
I get that str.strip() needs some work. However, for the time being (and to ensure backwards compatibility) surely re.sub() is a solid choice for some of the use cases you guys are discussed no?
can't believe it
Author: Jukka Lehtosalo Sponsor: Guido van Rossum Status: Accepted Version: 3.8 PEP 484 defines the type Dict[K, V] for uniform dictionaries, where each value has the same type, and arbitrary key values are supported. It doesn't properly support the common pattern where the type of a dictionary value depends on the string value of the key. Core idea: Consider creating a type to validate an arbitrary JSON document with a fixed schema Proposed syntax: https://icetutor.com
I think the methodology for the calculation of language popularity is specifically under representative of both R and python. if you check out trends for dplyr (R) or pandas (python) packages for data manipulation, both dwarf the overall language specific searches. I wonder if that bias also partially led to the declining interest in Ruby on Rails.
fgr Dr rhh
Thanks for the kubernetes example, and overall good episode
ypf
As usual, perfect!
I think you missed to highlight all the nice work of realphlython and your podcasts, these are key stuffs for Python in 2018!
The jokes are good but let brian do it. 😂
Congrats Python Bytes. This episode was really great 😎
Joel Grus talk can be found here: https://youtu.be/7jiPeIFXb6U
víbora means in Spanish: snake. umm, just thinking about Phyton
It's intetesting the title is flask but you guys spoke more about Django? kidding? hahaha please dont mess with us《Mico framework fans Thanks
nice, another super good Python postcast