Discover
The Data Science Education Podcast
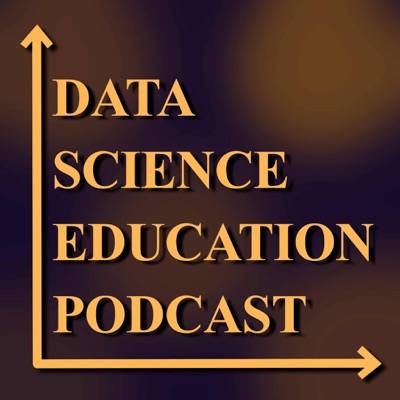
The Data Science Education Podcast
Author: Berkeley Data Science
Subscribed: 28Played: 68Subscribe
Share
© Data Science Education Program
Description
Produced by UC Berkeley's Data Science Undergraduate Studies. In this space, you will hear from a variety of distinguished Data Science educators and professionals. The individuals we’ll speak with are diverse in experience and perspective, but share the common goal of shaping the future of Data Science Education! Transcripts available at https://datascienceeducation.substack.com/
To learn more about UC Berkeley's Data Science Undergraduate Studies, visit our website at https://cdss.berkeley.edu/dsus.
datascienceeducation.substack.com
To learn more about UC Berkeley's Data Science Undergraduate Studies, visit our website at https://cdss.berkeley.edu/dsus.
datascienceeducation.substack.com
61 Episodes
Reverse
Access the full transcript for this episode“I would recommend double majoring with a different degree, because I think while data science by itself is a very, very useful and versatile degree, I think being able to apply it to a particular domain overall makes you a better statistician, or economist, or historian, right?”—Alan LiangIn the final episode of the season, we explore the pivotal role students played in shaping Berkeley’s undergraduate data science program. We sat down with three alumni — Alan Liang, Vinitra Swamy, and Gunjan Baid — who were instrumental in building the foundations of data science education at Berkeley. They reflect on their unique contributions, including developing curriculum, infrastructure, and interdisciplinary initiatives, and how those experiences shaped their career trajectories. From Alan’s insights into teaching technical concepts, to Vinitra’s innovative work on scaling Jupyter infrastructure, and Gunjan’s efforts on connector courses and technical systems, we highlight the long-lasting impact of student-led innovation.“I really loved the experience here of being a graduate student….there's a very collaborative atmosphere that people are always super excited about working on what they're working on, and that passion is what really drew me to the PhD as well. Like, the excitement to work on ideas that might be a bit too risky, that might be a little bit out there, a bit crazy, but you know, trying to get it to work and to work alongside people that are willing to put in the late nights and early mornings, because they want to, not because someone is forcing them to.”—Vinitra Swamy“Really dig deep and make sure you understand the details of a problem that you're working on. This still comes up a lot for me, but if something seems like it's off, if you're training a model and something looks funky, it's probably because something is off. And I think it's easy to kind of brush over the details and kind of gloss over that. But more often than not, really kind of getting your hands dirty, peeling back the layers, looking at the data, and going deep on a problem is how you'll make the most progress.”—Gunjan Baid This is a public episode. If you would like to discuss this with other subscribers or get access to bonus episodes, visit datascienceeducation.substack.com
Access the full transcript for this episode“We introduce a new data set to them every week, and we try and use data sets that are either themed around Illinois, or themed around things that we think that they are interested in. And so that's been something that we started doing when we first piloted the course, and have continued to do that each semester. And the students really are invested in the course, because they're using real world data that they have questions about.”—Karle FlanaganIn this episode, we explore the creation and growth of STAT 107, the University of Illinois Urbana-Champaign’s introductory data science course designed to be accessible to all students, regardless of major or background. We sat down with Karle Flanagan and Wade Fagen-Ulmschneider, the teaching professors behind STAT 107, to discuss their journey from a pilot program with 18 students to a thriving course with 1000+ students today. They detail how they built a curriculum that combines computer science and statistics, while keeping students engaged through real-world datasets, interactive live demos, and interdisciplinary collaboration. They delve into the challenges of scaling the course, the importance of co-teaching, and their broader efforts to expand data science education to high schools through initiatives like the DPI Digital Scholars Program.“So in the very beginning, we actually started by being like, there's going to be a CS day and a Stat day, and that I would give a CS lecture, and then Karle would be there, kind of just sitting in the audience, and then Karle would give a Stat lecture the next day, and they'd be inner related, but they were kind of separated. And then one day, we were just like, I want to kind of get Karle's opinion on something and let give her perspective, because I come from an engineering background, and I am obsessed with formulas. Karle, I think, really relies more on, like, tables and graphs and like, really wants to understand the story behind data, and only once you're motivated by the story do you really want to dive in deeper. And so the way we see problems are wildly different…Students just love the fact that it's back and forth.” —Wade Fagen-Ulmschneider This is a public episode. If you would like to discuss this with other subscribers or get access to bonus episodes, visit datascienceeducation.substack.com
Access the full transcript for this episode“One of the ways we incorporate ethics is by trying to expose students to a plurality of perspectives. So we want students to hear from people with different perspectives on what it means to engage with data ethically, and so we do this by hosting guest speakers. We encourage students to take classes in a variety of departments around campus. We also try to introduce students to frameworks that can help them think about how to incorporate diverse perspectives in the creation of tech products and policy.” —Mallory NoblesToday, we sit down with Dennis Sun and Mallory Nobles from Stanford University to discuss the university’s innovative approach to undergraduate data science education. Dennis and Mallory share insights into Stanford's dual-track offerings: the technical BS in Data Science and the interdisciplinary BA in Data Science & Social Systems. They dive into the origins and goals behind these programs, highlighting how they equip students with essential skills in data science, statistics, and ethics. The conversation also covers Stanford's emphasis on experiential learning through capstones, project-based courses, and partnerships with fields like neuroscience and engineering. “When I came to Stanford, one challenge that was clear to me was that there were hardly any data science and machine learning classes that were accessible to freshmen or students early on in their college careers. So many of them were gated behind probability, linear algebra, and even several computer science courses. And it's a lot to ask a student to take a bunch of theoretical courses before they get to find out what data science is really about. So that was kind of the genesis of the Principles of Data Science course. It was designed to give students a sense of what data science is about, and it gives them the practical motivation to convince them that all the theoretical courses that they'll have to take are going to be worth it in the end.” —Dennis Sun This is a public episode. If you would like to discuss this with other subscribers or get access to bonus episodes, visit datascienceeducation.substack.com
Access the full transcript for this episode“Entonces lo que yo procuro hacer con los estudiantes que son de áreas como de humanidades o ciencias sociales, es asociarlo como a situaciones cotidianas, haciendo analogías o buscando ejemplos de cosas que cualquiera ha experimentado. Eh como que se desarrolle esa intuición y ya después pues lo lo le ponemos como él la forma de de la sintaxis y ya el lenguaje específico que usemos”In the podcast’s first ever Spanish speaking episode, Eric Van Dusen and special guest host Edwin Vargas Navarro sit down with Camilo Andrés De La Cruz Arboleda from the Universidad Externado de Colombia. Camilo shares his journey from studying law to embracing data science and technology, merging the two fields to innovate legal education in Colombia. He discusses how he engages law students with data science concepts, making technical subjects accessible to those without a STEM background. Camilo also explores the challenges of teaching data science in Latin America, the importance of open data, and the role of data science in sustainability and public policy. En el primer episodio en español del podcast, Eric Van Dusen y el invitado especial Edwin Vargas Navarro conversan con Camilo Andrés De La Cruz Arboleda de la Universidad Externado en Colombia. Camilo comparte su trayectoria, desde estudiar derecho hasta abrazar la ciencia de datos y la tecnología, fusionando ambos campos para innovar la educación legal en Colombia. Habla sobre cómo involucra a los estudiantes de derecho con los conceptos de ciencia de datos, haciendo accesibles los temas técnicos para aquellos que no tienen antecedentes en STEM. Camilo también explora los desafíos de enseñar ciencia de datos en América Latina, la importancia de los datos abiertos y el papel de la ciencia de datos en la sostenibilidad y las políticas públicas.“Yo creo que históricamente el derecho ha sido una profesión que ha estado muy reacia como a a aceptar como una revolución tecnológica y por lo menos acá en Colombia, hasta incluso hace muy pocos años se permitía hacer una audiencia por una videollamada o incluso radicar documentos por un correo electrónico es que algo que existía hace miles de de años hasta ahora, hace recientemente se se pudo incorporar dentro de del día a día de la carrera de los abogados. Si uno quiere seguir siendo competitivo, tiene cuanto menos, conocer lo que puede hacer con tecnología e incorporarlo a su a su día a día. Sea un abogado que haga eso, va a estar diez veces más preparado que el que quiera seguir como la en la en la forma tradicional, pues de llevar a cabo la profesión.” This is a public episode. If you would like to discuss this with other subscribers or get access to bonus episodes, visit datascienceeducation.substack.com
Access the full transcript for this episode“I literally collected 150 jobs on Indeed.com and parsed out all of the skills that were mentioned in all the jobs, created a graphic and said, Okay, here's the courses we already have that have these skills, and here's the skills I need to create courses for.”Today, we sat down with Crystal Wiggins, a pioneering educator in two-year college data science programs at Connecticut State Community College. Crystal shares her journey in developing Connecticut’s first two-year data science program, which has since expanded to five campuses. She discusses her innovative approach to project-based learning, teaching students to "get comfortable with the uncomfortable," and preparing them to adapt in a rapidly evolving field. Crystal also delves into her leadership role in nationwide conversations about data science in community colleges, her work with organizations like AMATYC (American Mathematical Association of Two-Year Colleges), and her vision for industry partnerships in the classroom.“Don't be afraid to dive in. You do not need to be an expert. You can learn this with your students. There's many things that students ask me, and I'm like, Well, let me show you how to find the answer. And I was actually finding the answer for myself because I didn't know, but that's what's great about the field; it's more about teaching them how to find answers than it is knowing everything yourself. So again, my slogan, be comfortable with the uncomfortable, is like the slogan for data science for me, because you're never going to know everything, and that's what I tell my students.” This is a public episode. If you would like to discuss this with other subscribers or get access to bonus episodes, visit datascienceeducation.substack.com
Access the full transcript for this episode“I developed a class called DS 100, which is in a lot of ways very similar [to Data 8], with the primary objective being, I want people to walk away from the class with saying I understand what data science is. I can do a little bit of programming, and now it's up to me whether I think it's interesting or not. I don't want anyone ever to feel like they can't do it. It's just whether or not they enjoy doing it.”In this episode, we sit down with Langdon White from Boston University to discuss his journey from software consulting to becoming a key figure in BU's growing data science program, starting off with BU Spark! He shares the challenges of expanding a data science curriculum, including the launch of new programs, and his overarching mission to make data science accessible to students of all backgrounds. Langdon also explores innovative teaching methods like experiential learning and gamification, while highlighting the importance of diversity, ethics, and inclusivity in data science education.“I continue to think that our biggest challenge in this industry is making sure that we have representation from all backgrounds, right?…Every student should be walking out of the school with an expectation of inclusion and diversity, but also ethics. And that the ethics falls to you…and you know, encouraging students to step up and represent themselves, from an ethical perspective, an inclusion perspective, and the diversity perspective.” This is a public episode. If you would like to discuss this with other subscribers or get access to bonus episodes, visit datascienceeducation.substack.com
Access the full transcript for this episode“So what we do in Data Feminism is try to synthesize a whole lot of feminist ways of thinking about the world, that have to do with questions of bias and oppression, that have to do with questions of sort of unequal power, and who gets to make choices about how to design systems — with these sort of really broad social questions, we try to apply them to data science as both a field and as a practice.”Join us as we engage in a conversation with Lauren F. Klein, Associate Professor at Emory University and Director of the Digital Humanities Lab. Klein shares her unique journey from a background in comparative literature to pioneering the field of digital humanities, where she bridges the gap between computational methods and humanistic inquiry. We delve into her innovative projects, including her influential "Data Feminism" book and the "Data by Design" project, exploring how these works challenge traditional data science perspectives and emphasize the importance of context, history, and ethics in data visualization. “The point that I'm trying to make in this project is that if we take this historicized, almost literary and critical, humanistic lens to this history, we can see how the people who were designing data visualizations were either asking very similar questions to the kinds of questions about responsible data visualization that we're asking today, or they weren't. And because of that, we can see how their visualizations — far from being some sort of neutral representation of data — in fact, represented a certain policy sort of unreflective politics that I think we also need to be able to identify again, so that we don't reproduce that in the present.” This is a public episode. If you would like to discuss this with other subscribers or get access to bonus episodes, visit datascienceeducation.substack.com
Access the full transcript for this episode“We're kind of in an early phase among most social scientists, trying to figure out what's new here, what's different, and how to integrate it with our standard social science methodological concerns, which I don't think we should abandon. Thinking about the relationship between theory, concept and measurement. For example, that's one of the things that social scientists bring to the table in data science projects: thinking about questions of representativeness, generalizability, and questions of causal inference.”Welcome to the season 8 premiere! In this episode, we sit down with David J. Harding, a professor in the sociology department at UC Berkeley. David shares his unique academic journey in sociology and data science, emphasizing the integration of social science methodologies with data science tools. He discusses his work on poverty, inequality, and incarceration, and the challenges of using administrative data in research. The conversation delves into future directions for his research on adolescents and urban communities, the importance of bridging social science and data science education, and strategies for creating inclusive classroom environments.“A standard complaint about running and estimating models in the social sciences is that we make a lot of assumptions, and then don't have the ability to test them. Then right along comes the kind of more machine learning type workflow, which allows us to learn what the model should look like from a portion of the data, and then test it and validate it on another portion of the data. I think social scientists should be building that sort of workflow into our normal work process all the time.” This is a public episode. If you would like to discuss this with other subscribers or get access to bonus episodes, visit datascienceeducation.substack.com
Access the full transcript for this episode“You can be hoodwinked with data in the same way that you can be hoodwinked by a car salesman. And so the idea of [Calling B******t] was to step away from all the details of the black box: that's the statistical procedures, the algorithms, etc. (Not to say that we don't pay attention to what we do.) But the idea is to really pay attention to the input data that's coming in—to think about things like selection bias—to think about where that data is coming from.”Join us in our Season 7 finale as we host Jevin West, an associate professor at the University of Washington and a co-founder of the Center for an Informed Public. Dive into a deep discussion about the intersection of data science and misinformation, the challenges of big data, and the ethical considerations that come with it. Jevin shares his experiences from the early days of data science programs, his insights on combating misinformation through education, and the evolution of his course and book, "Calling B******t." Whether you're a data science professional or a student, listen in to explore how data science education can empower us to make informed decisions and foster a more truthful society.“One of the most important skills that we're going to want to enhance more and more is humaneness…things like being able to ask questions, to sort of work through logic to really tease out things, like correlation versus causation. Machines don't tend to do so well [with those things]—they don't have access to the physical world. That's one of their weaknesses. So you want to lean into your strategic advantages as humans…maintain that humaneness by doing things that machines can't do.” This is a public episode. If you would like to discuss this with other subscribers or get access to bonus episodes, visit datascienceeducation.substack.com
Access the full transcript for this episodeJoin us as we speak with three different guests, all UC Berkeley Data Science alumni, who have gone on to pursue higher education. Ranging from learning sciences to epidemiology, our guests share their experiences, challenges, and insights into how their data science education prepared them for their current paths.Ashley Quiterio, a PhD student in Learning Sciences at Northwestern University, delves into the intersection of data science and education, highlighting the transformative potential of data-driven approaches in shaping learning environments.“Try everything and try different things. I mentioned all these different roles [I did during undergrad], where I was trying to see where I fit, deciding what I like about data education. There's all these different lenses and different ways of thinking about where you fit. So I'd encourage people to try that out, early and often. Data science is such an interdisciplinary field that you're not going to be lacking opportunities.” — Ashley QuiterioAnna Nguyen, a PhD student in Epidemiology and Clinical Research at Stanford University, shares her journey from data science to public health, emphasizing the importance of interdisciplinary collaboration in addressing complex health challenges.“Regardless of what anyone says, there's no pure cut way of getting into grad school. Pursuing opportunities that allow you to really explore your interests and displaying a willingness to learn is probably the best way to prepare for a masters or a PhD program. I think I definitely overestimated how much time I had in undergrad. And the time was so limited and valuable, so it's really not worth doing things that you don't enjoy in that limited time.” — Anna NguyenRodrigo Palmaka, a Masters student in Statistics at UC Berkeley, offers perspectives on computational pathology and statistical research, illustrating the versatility of data science skills in diverse research domains.“I think I always sought to focus on the fundamentals—not overfit or pigeonhole myself too much—and give myself some flexibility to, you know, be able to adapt to the next big thing.” — Rodrigo Palmaka This is a public episode. If you would like to discuss this with other subscribers or get access to bonus episodes, visit datascienceeducation.substack.com
Access the full transcript for this episode“UC Merced opened in 2005, so we were starting from a very different place than lots of campuses are. So I try very hard to be really intentional about when we think about hiring people; we want to be very aware of ways that unconscious bias plays out in in hiring. When we invite people to give seminars, we try to invite people from variety of backgrounds and campuses. And so I think that being at UC Merced—a new campus with a really strong emphasis on diversity—it's very much something that’s important to the students.”Join us in conversation with Suzanne Sindi, Professor of Applied Mathematics and Chair of the Department at UC Merced, as she shares her journey in incorporating data science concepts into her teaching, highlighting the importance of engaging students through real-world applications and interdisciplinary approaches. Suzanne discusses her involvement in diversity initiatives, such as the SIAM Activity Group in Equity, Diversity, and Inclusion, and how it shapes her teaching philosophy and fosters a more inclusive learning environment. We also touch on the challenges and opportunities of data science education in diverse settings, such as UC Merced's Central Valley location, and learn about strategies for preparing students to navigate the evolving landscape of mathematical and computational disciplines.“So something like the mean or average value, are words that, you know, have meanings outside of math. And so now you're trying to use this in a context, like in sort of a scientific context. And one of the things I hadn't appreciated is, if you're working with people who potentially don't come from homes where they speak English at home, they don't have maybe the same context for some of those words in those terms.” This is a public episode. If you would like to discuss this with other subscribers or get access to bonus episodes, visit datascienceeducation.substack.com
Access the full transcript for this episode“We are definitely a Hispanic enrolling institution, but the TIPS project is aiming to embrace that ‘serving’ term, and just the ideal of serving our Hispanic students. Through the TIPS project, there's a ton of professional development — very deep, profound professional development. We want an entire department to participate in the TIPS pathway because the department is a unit of change, meaning that the entire community and culture of that department will change, rather than just having a few people who are interested in DEI initiatives.”Join us in discussion with Dr. Omayra Ortega, a professor at Sonoma State University, as we delve into the evolving landscape of data science education. From her journey as a mathematician with a background in music to her current endeavors in mathematical epidemiology and data science, Dr. Ortega shares insights into the intersectionality between gender, ethnicity, and inclusion in the data science community. As a former president of the National Association of Mathematicians and a passionate advocate for underrepresented groups in STEM, Dr. Ortega discusses the importance of fostering diversity and equity in data science education.“If you're a data science educator, make friends with other data science educators because I'm sure they need help. They need your ideas, your models for how you run your degree program, for how you run your classes, and best practices. Go to those lovely workshops that are organized at UC Berkeley every summer and spring — if you're in California, join CADSE.” This is a public episode. If you would like to discuss this with other subscribers or get access to bonus episodes, visit datascienceeducation.substack.com
Access the full transcript for this episode“Whenever I'm trying to teach people, I try to demystify the verbiage around computer science and data science, getting people to understand that we can talk about things in a way that makes more sense to you, by using words that you're more familiar with. When we're using all these words that people aren't familiar with, that's automatically going to get people to like retreat into a shell…we have to demystify the way that we talk about technology for people to feel like it's something that can actually be understood.”In today’s episode, we sit down with Henry Bowe, the Lead Technical Instructor at Hack the Hood, an organization providing free tech education programs focused on exploring foundational technical skills through a justice lens. From Henry's personal journey into software engineering to the impactful work of Hack the Hood in empowering marginalized communities, listeners will gain insights into the intersection of technology, education, and social justice. Explore Hack the Hood's innovative programs, the incorporation of social justice into data science curriculum, and the importance of making technical concepts accessible.“And we really believe that if you can give somebody the tools to really feel like they belong in that space, to really feel like they can be comfortable in that space and they can thrive in that space, then the sky is really the limit.” This is a public episode. If you would like to discuss this with other subscribers or get access to bonus episodes, visit datascienceeducation.substack.com
Access the full transcript for this episode“Getting Python workshops, data analysis workshops…and our own Datathon, provided a lot of low stakes, low commitment opportunities [for students], and just getting in the faces of students, telling them they should try it out, has been helpful in at least generating excitement around data science for students to actually inquire about it.”In this episode, join our conversation with Denise Hum, Mathematics Engineering Science Achievement (MESA) professor from Skyline College. Delve into the journey of bringing data science education to the community college level, where Denise shares her motivations, challenges, and innovative approaches. From redefining math curriculum to fostering partnerships with four-year institutions, discover how Denise is paving the way for broader access to data science education. Gain insights into the evolving landscape of STEM education and the pivotal role data science plays in shaping the future.“I know that this is an interesting time to be in math education, with AB 1705, and the changes that that will bring. But I think that data science gives us the opportunity to really rethink math curriculum and really invigorate it. I know that data science is sort of interdisciplinary between math and computer science…I think that it invites the conversation about how we can innovate, and really an opportunity to create new courses. Yes, we will lose some courses as a result of this legislation, but at the same time, let's create some new ones.” This is a public episode. If you would like to discuss this with other subscribers or get access to bonus episodes, visit datascienceeducation.substack.com
Access the full transcript for this episode“For a long time, I didn't want to write a book about statistics…But I felt that the two things that I could add, based on my BBC experience, was, one, a kind of psychological realism: a recognition that a lot of what we think is not really about, oh, you got confused between correlation and causation or something like that. The problem is you believe something because you wanted to believe it. The second thing that I wanted to introduce was just the idea that statistics can be a really positive thing, your data can be a positive thing…Even among people who are advocates for data science, it's very easy to fall into the trap of only talking about things going wrong, only talking about misinformation…I wanted to push back against that.”In this compelling episode, we engage in a dynamic conversation with Tim Harford, renowned economist, author of “The Data Detective,” and host of BBC’s “More or Less” podcast. Harford shares his journey from economist to BBC presenter, unveiling the inspiration behind "The Data Detective" and his distinctive approach to the subject. Delving into the challenges of building trust in statistics amid contemporary skepticism, Harford underscores the importance of trustworthy data connected to real-world issues. The conversation extends to the role of educators in promoting data literacy for society, with Harford advocating for the integration of statistical thinking across academic disciplines to highlight the positive impact of data.“So to educate us, I would say, are you teaching the three C's? Are you encouraging your students to be calm? Are you educating them in the importance of context, as well as all the technical stuff? Like all the things around the technical stuff that make all the difference? And above all, are you fostering a sense of curiosity in your students? I'm sure most educators hope to do that. But it's always a good idea to remind ourselves.” This is a public episode. If you would like to discuss this with other subscribers or get access to bonus episodes, visit datascienceeducation.substack.com
Access the full transcript for this episode“Our focus is very balanced across foundations and applications, we feel that they're hand in hand. But the Northstar of what we're building is a new discipline...We understand that Data Science is going to not just take a bunch of disciplines together to form a new discipline, but it's actually going to take things that are not even at the university.”Hello and welcome back to the seventh season of the Data Science Education Podcast! In this episode, we’re chatting with David Uminsky, Executive Director of the Data Science Institute at the University of Chicago. We begin by exploring Uminsky’s career evolution from a mathematician to a key player in the Data Science education sphere, and then shift to insights into the innovative initiatives happening at the University of Chicago, including the development of intentional doctoral programs and the groundbreaking preceptorship program that bridges the gap between academia and community colleges.“When we're having these conversations with the community colleges, I was thinking: Wait a minute, there's a real thing here that they want. And what they wanted was to make sure that there were 100,000+ students being served by these incredible seven campuses plus, that were at risk of being left out of the data and AI revolution, the workforce training, and the educational pathways. And they wanted to form a partnership around that with UChicago.” This is a public episode. If you would like to discuss this with other subscribers or get access to bonus episodes, visit datascienceeducation.substack.com
Access the full transcript for this episodeWelcome to the final episode of Season 8! Like every season, we’re spending our last episode talking to three recent data science graduates about navigating post-grad life and what it means to enter the industry. We start with Rebecca Hayes, a recent graduate of the City College of San Francisco, who current works as a data analyst and emphasizes the importance of SQL, interpersonal skills, and project management. We then listen to Jacob Cavanaugh from Cal Poly San Luis Obispo, where he shares his experiences in location analytics, highlighting the impact of introductory courses and adaptability. We end with Yash Potdar, a recent UC San Diego graduate and current software engineer at Rivian, who discusses critical thinking, problem-solving, and the role of a product design elective. Talk to you all next season!“Do something that makes you passionate. If you love dogs, or ice cream, or music, think about how you can learn something and create something in data science using that type of data.” — Rebecca Hayes (CCSF)“Don't forget to exercise your people skills. The technical ones are important, but at the end of the day, your employers, your coworkers, they're gonna remember the people that connected with them.” — Jacob Cavanaugh (Cal Poly SLO)“Don't pigeonhole yourself into data science. Don't second guess yourself. If you believe that you have the basic skills and can put in the effort to learn, just apply. Don't be discouraged.” — Yash Potdar (UC San Diego) This is a public episode. If you would like to discuss this with other subscribers or get access to bonus episodes, visit datascienceeducation.substack.com
Access the full transcript for this episode“One of the things that I tell my students is like, you are learning how to learn as well. And being able to provide students with the guardrails, provide them with the support that they need to realize what they find interesting. I think that's one of the things I tried to do in these large classes.”In this episode of the Data Science Education podcast, join us in our conversation with Lisa Yan, an assistant teaching professor in Electrical Engineering and Computer Science at UC Berkeley. Discover the interdisciplinary nature of data science education, the challenges of teaching large classes, and the importance of creating a supportive community for students. Lisa Yan shares her experiences in teaching gateway classes like Data 100 and Data 101, emphasizing the need to empower students and cultivate problem-solving skills. Explore the intersection of technology, society, and power as Lisa discusses her seminars on social implications of computer technology and technology, society, and power.“I have the realization everyday that the study of data science is not just a technical one, but that it's applicable to pretty much anything and everything because we are living in the world of data. And so understanding not just as a citizen, how the data flows around us, but also understanding how we as data scientists can change the world around us with the way that we analyze data and understand data and share our findings from data. I think that's really, really important that we continue to make such a field interdisciplinary and open to many, many different students.” This is a public episode. If you would like to discuss this with other subscribers or get access to bonus episodes, visit datascienceeducation.substack.com
Access the full transcript for this episode“So we need teachers, ones that are interested in learning data science and willing to go through the growing pains of being able to learn something new. And we need pathways. We need pathways to get students interested in things, to know about data science and know what it is, into high schools.”In this episode, we engage in a conversation with Professor Solomon Russell from El Camino College, a community college in Southern California. Professor Russell shares his journey from teaching computer science to his dedicated focus on advancing data science education, discussing the challenges and successes of introducing data science at the college level. He reflects on the diversity among students entering the data science classes and the disparities in completion rates, highlighting strategies to bridge the knowledge gap and ensure student success. Delving into his ongoing work, including the development of an intermediate data science class for community college students and securing grants to expand data science education, he underscores the importance of ethics, equity, and community building in this field.“I really think education is about liberation, right? Liberating, like different ways that you thought in the past, liberating other people, liberating your future, like the people that come after you. So to be able to make people more conscious through data science—I think that's a huge win. But ultimately, even beyond data science, I just want people to find what they're passionate about…like, I'm a dancer, so I always think that everybody has a dance.” This is a public episode. If you would like to discuss this with other subscribers or get access to bonus episodes, visit datascienceeducation.substack.com
Access the full transcript for this episode“Just like how doctors take the Hippocratic Oath, I would hope data scientists would take a similar oath and have the mantra 'first do no harm.'”In this episode, we sit down with Professor R. Uma to discuss her work in broadening participation in data science for social justice. Professor Uma shares her journey from teaching computer science at a historically black university to using data science as a tool to make STEM education more inclusive. She talks about her innovative approaches to teaching data science to students from diverse backgrounds, her recent NSF grant to develop a data analytics certificate for non-computing majors, and the importance of ethics and inclusivity in the field of data science. Join us for an insightful conversation on the future of data science education and its potential to create a more equitable and just society!“Many of [students] come from high schools where they had no exposure to computer science courses. And the only exposure to computers that they've had is to create some PowerPoint presentation or a Word document. They come here, they take the first CS course, which for us is a C+ class, and they're like, “No, this is not what I thought computer science was about.” And so that obviously led to huge attrition and retention problems.” This is a public episode. If you would like to discuss this with other subscribers or get access to bonus episodes, visit datascienceeducation.substack.com
Comments
Top Podcasts
The Best New Comedy Podcast Right Now – June 2024The Best News Podcast Right Now – June 2024The Best New Business Podcast Right Now – June 2024The Best New Sports Podcast Right Now – June 2024The Best New True Crime Podcast Right Now – June 2024The Best New Joe Rogan Experience Podcast Right Now – June 20The Best New Dan Bongino Show Podcast Right Now – June 20The Best New Mark Levin Podcast – June 2024