Discover
The Nonlinear Library: LessWrong
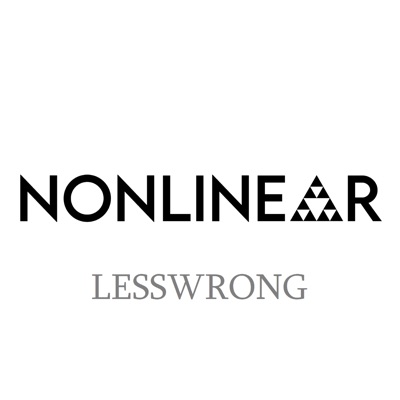
The Nonlinear Library: LessWrong
Author: The Nonlinear Fund
Subscribed: 2Played: 2,182Subscribe
Share
© 2024 The Nonlinear Fund
Description
The Nonlinear Library allows you to easily listen to top EA and rationalist content on your podcast player. We use text-to-speech software to create an automatically updating repository of audio content from the EA Forum, Alignment Forum, LessWrong, and other EA blogs. To find out more, please visit us at nonlinear.org
3123 Episodes
Reverse
Link to original articleWelcome to The Nonlinear Library, where we use Text-to-Speech software to convert the best writing from the Rationalist and EA communities into audio. This is: Why I'm doing PauseAI, published by Joseph Miller on April 30, 2024 on LessWrong.
GPT-5 training is probably starting around now. It seems very unlikely that GPT-5 will cause the end of the world. But it's hard to be sure. I would guess that GPT-5 is more likely to kill me than an asteroid, a supervolcano, a plane crash or a brain tumor. We can predict fairly well what the cross-entropy loss will be, but pretty much nothing else.
Maybe we will suddenly discover that the difference between GPT-4 and superhuman level is actually quite small. Maybe GPT-5 will be extremely good at interpretability, such that it can recursively self improve by rewriting its own weights.
Hopefully model evaluations can catch catastrophic risks before wide deployment, but again, it's hard to be sure. GPT-5 could plausibly be devious enough so circumvent all of our black-box testing. Or it may be that it's too late as soon as the model has been trained. These are small, but real possibilities and it's a significant milestone of failure that we are now taking these kinds of gambles.
How do we do better for GPT-6?
Governance efforts are mostly focussed on relatively modest goals. Few people are directly aiming at the question: how do we stop GPT-6 from being created at all? It's difficult to imagine a world where governments actually prevent Microsoft from building a $100 billion AI training data center by 2028.
In fact, OpenAI apparently fears governance so little that they just went and told the UK government that they won't give it access to GPT-5 for pre-deployment testing. And the number of safety focussed researchers employed by OpenAI is dropping rapidly.
Hopefully there will be more robust technical solutions for alignment available by the time GPT-6 training begins. But few alignment researchers actually expect this, so we need a backup plan.
Plan B: Mass protests against AI
In many ways AI is an easy thing to protest against. Climate protesters are asking to completely reform the energy system, even if it decimates the economy. Israel / Palestine protesters are trying to sway foreign policies on an issue where everyone already holds deeply entrenched views. Social justice protesters want to change people's attitudes and upend the social system.
AI protesters are just asking to ban a technology that doesn't exist yet. About 0% of the population deeply cares that future AI systems are built. Most people support pausing AI development. It doesn't feel like we're asking normal people to sacrifice anything. They may in fact be paying a large opportunity cost on the potential benefits of AI, but that's not something many people will get worked up about.
Policy-makers, CEOs and other key decision makers that governance solutions have to persuade are some of the only groups that are highly motivated to let AI development continue.
No innovation required
Protests are the most unoriginal way to prevent an AI catastrophe - we don't have to do anything new. Previous successful protesters have made detailed instructions for how to build a protest movement.
This is the biggest advantage of protests compared to other solutions - it requires no new ideas (unlike technical alignment) and no one's permission (unlike governance solutions). A sufficiently large number of people taking to the streets forces politicians to act. A sufficiently large and well organized special interest group can control an issue:
I walked into my office while this was going on and found a sugar lobbyist hanging around, trying to stay close to the action. I felt like being a smart-ass so I made some wise-crack about the sugar industry raping the taxpayers. Without another word, I walked into my private office and shut the door. I had no real plan to go after the sugar people. I was just screwing with the guy.
My phone did no...
Link to original articleWelcome to The Nonlinear Library, where we use Text-to-Speech software to convert the best writing from the Rationalist and EA communities into audio. This is: Introducing AI Lab Watch, published by Zach Stein-Perlman on April 30, 2024 on LessWrong.
I'm launching AI Lab Watch. I collected actions for frontier AI labs to improve AI safety, then evaluated some frontier labs accordingly.
It's a collection of information on what labs should do and what labs are doing. It also has some adjacent resources, including a list of other safety-ish scorecard-ish stuff.
(It's much better on desktop than mobile - don't read it on mobile.)
It's in beta
leave feedback here or comment or DM me - but I basically endorse the content and you're welcome to share and discuss it publicly.
It's unincorporated, unfunded, not affiliated with any orgs/people, and is just me.
Some clarifications and disclaimers.
How you can help:
Give feedback on how this project is helpful or how it could be different to be much more helpful
Tell me what's wrong/missing; point me to sources on what labs should do or what they are doing
Suggest better evaluation criteria
Share this
Help me find an institutional home for the project
Offer expertise on a relevant topic
Offer to collaborate
(Pitch me on new projects or offer me a job)
(Want to help and aren't sure how to? Get in touch!)
I think this project is the best existing resource for several kinds of questions, but I think it could be a lot better. I'm hoping to receive advice (and ideally collaboration) on taking it in a more specific direction. Also interested in finding an institutional home. Regardless, I plan to keep it up to date. Again, I'm interested in help but not sure what help I need.
I could expand the project (more categories, more criteria per category, more labs); I currently expect that it's more important to improve presentation stuff but I don't know how to do that; feedback will determine what I prioritize. It will also determine whether I continue spending most of my time on this or mostly drop it.
I just made a twitter account. I might use it to comment on stuff labs do.
Thanks to many friends for advice and encouragement. Thanks to Michael Keenan for doing most of the webdev. These people don't necessarily endorse this project.
Thanks for listening. To help us out with The Nonlinear Library or to learn more, please visit nonlinear.org
Link to original articleWelcome to The Nonlinear Library, where we use Text-to-Speech software to convert the best writing from the Rationalist and EA communities into audio. This is: Towards a formalization of the agent structure problem, published by Alex Altair on April 30, 2024 on LessWrong.
In Clarifying the Agent-Like Structure Problem (2022), John Wentworth describes a hypothetical instance of what he calls a selection theorem. In Scott Garrabrant's words, the question is, does agent-like behavior imply agent-like architecture? That is, if we take some class of behaving things and apply a filter for agent-like behavior, do we end up selecting things with agent-like architecture (or structure)? Of course, this question is heavily under-specified.
So another way to ask this is, under which conditions does agent-like behavior imply agent-like structure? And, do those conditions feel like they formally encapsulate a naturally occurring condition?
For the Q1 2024 cohort of AI Safety Camp, I was a Research Lead for a team of six people, where we worked a few hours a week to better understand and make progress on this idea. The teammates[1] were Einar Urdshals, Tyler Tracy, Jasmina Nasufi, Mateusz Bagiński, Amaury Lorin, and Alfred Harwood.
The AISC project duration was too short to find and prove a theorem version of the problem. Instead, we investigated questions like:
What existing literature is related to this question?
What are the implications of using different types of environment classes?
What could "structure" mean, mathematically? What could "modular" mean?
What could it mean, mathematically, for something to be a model of something else?
What could a "planning module" look like? How does it relate to "search"?
Can the space of agent-like things be broken up into sub-types? What exactly is a "heuristic"?
Other posts on our progress may come out later. For this post, I'd like to simply help concretize the problem that we wish to make progress on.
What are "agent behavior" and "agent structure"?
When we say that something exhibits agent behavior, we mean that seems to make the trajectory of the system go a certain way. We mean that, instead of the "default" way that a system might evolve over time, the presence of this agent-like thing makes it go some other way. The more specific of a target it seems to hit, the more agentic we say it behaves. On LessWrong, the word "optimization" is often used for this type of system behavior. So that's the behavior that we're gesturing toward.
Seeing this behavior, one might say that the thing seems to want something, and tries to get it. It seems to somehow choose actions which steer the future toward the thing that it wants.
If it does this across a wide range of environments, then it seems like it must be paying attention to what happens around it, use that information to infer how the world around it works, and use that model of the world to figure out what actions to take that would be more likely to lead to the outcomes it wants. This is a vague description of a type of structure. That is, it's a description of a type of process happening inside the agent-like thing.
So, exactly when does the observation that something robustly optimizes imply that it has this kind of process going on inside it?
Our slightly more specific working hypothesis for what agent-like structure is consists of three parts; a world-model, a planning module, and a representation of the agent's values. The world-model is very roughly like Bayesian inference; it starts out ignorant about what world its in, and updates as observations come in. The planning module somehow identifies candidate actions, and then uses the world model to predict their outcome.
And the representation of its values is used to select which outcome is preferred. It then takes the corresponding action.
This may sound to you like an algorithm for utility maximization. But a big part of the idea behind the agent structure problem is that there is a much l...
Link to original articleWelcome to The Nonlinear Library, where we use Text-to-Speech software to convert the best writing from the Rationalist and EA communities into audio. This is: Towards Multimodal Interpretability: Learning Sparse Interpretable Features in Vision Transformers, published by hugofry on April 30, 2024 on LessWrong.
Two Minute Summary
In this post I present my results from training a Sparse Autoencoder (SAE) on a CLIP Vision Transformer (ViT) using the ImageNet-1k dataset. I have created an interactive web app, 'SAE Explorer', to allow the public to explore the visual features the SAE has learnt, found here: https://sae-explorer.streamlit.app/ (best viewed on a laptop).
My results illustrate that SAEs can identify sparse and highly interpretable directions in the residual stream of vision models, enabling inference time inspections on the model's activations. To demonstrate this, I have included a 'guess the input image' game on the web app that allows users to guess the input image purely from the SAE activations of a single layer and token of the residual stream.
I have also uploaded a (slightly outdated) accompanying talk of my results, primarily listing SAE features I found interesting: https://youtu.be/bY4Hw5zSXzQ.
The primary purpose of this post is to demonstrate and emphasise that SAEs are effective at identifying interpretable directions in the activation space of vision models. In this post I highlight a small number my favourite SAE features to demonstrate some of the abstract concepts the SAE has identified within the model's representations. I then analyse a small number of SAE features using feature visualisation to check the validity of the SAE interpretations.
Later in the post, I provide some technical analysis of the SAE. I identify a large cluster of features analogous to the 'ultra-low frequency' cluster that Anthropic identified. In line with existing research, I find that this ultra-low frequency cluster represents a single feature. I then analyse the 'neuron-alignment' of SAE features by comparing the SAE encoder matrix the MLP out matrix.
This research was conducted as part of the ML Alignment and Theory Scholars program 2023/2024 winter cohort. Special thanks to Joseph Bloom for providing generous amounts of his time and support (in addition to the SAE Lens code base) as well as LEAP labs for helping to produce the feature visualisations and weekly meetings with Jessica Rumbelow.
Example, animals eating other animals feature: (top 16 highest activating images)
Example, Italian feature: Note that the photo of the dog has a watermark with a website ending in .it (Italy's domain name). Note also that the bottom left photo is of Italian writing. The number of ambulances present is a byproduct of using ImageNet-1k.
Motivation
Frontier AI systems are becoming increasingly multimodal, and capabilities may advance significantly as multimodality increases due to transfer learning between different data modalities and tasks. As a heuristic, consider how much intuition humans gain for the world through visual reasoning; even in abstract settings such as in maths and physics, concepts are often understood most intuitively through visual reasoning.
Many cutting edge systems today such as DALL-E and Sora use ViTs trained on multimodal data. Almost by definition, AGI is likely to be multimodal. Despite this, very little effort has been made to apply and adapt our current mechanistic interpretability techniques to vision tasks or multimodal models. I believe it is important to check that mechanistic interpretability generalises to these systems in order to ensure they are future-proof and can be applied to safeguard against AGI.
In this post, I restrict the scope of my research to specifically investigating SAEs trained on multimodal models. The particular multimodal system I investigate is CLIP, a model trained on image-text pairs. CLIP consists of two encoders: a language model and a vision model that are trained to e...
Link to original articleWelcome to The Nonlinear Library, where we use Text-to-Speech software to convert the best writing from the Rationalist and EA communities into audio. This is: Ironing Out the Squiggles, published by Zack M Davis on April 29, 2024 on LessWrong.
Adversarial Examples: A Problem
The apparent successes of the deep learning revolution conceal a dark underbelly. It may seem that we now know how to get computers to (say) check whether a photo is of a bird, but this façade of seemingly good performance is belied by the existence of adversarial examples - specially prepared data that looks ordinary to humans, but is seen radically differently by machine learning models.
The differentiable nature of neural networks, which make them possible to be trained at all, are also responsible for their downfall at the hands of an adversary. Deep learning models are fit using stochastic gradient descent (SGD) to approximate the function between expected inputs and outputs.
Given an input, an expected output, and a loss function (which measures "how bad" it is for the actual output to differ from the expected output), we can calculate the gradient of the loss on the input - the derivative with respect to every parameter in our neural network - which tells us which direction to adjust the parameters in order to make the loss go down, to make the approximation better.[1]
But gradients are a double-edged sword: the same properties that make it easy to calculate how to adjust a model to make it better at classifying an image, also make it easy to calculate how to adjust an image to make the model classify it incorrectly. If we take the gradient of the loss with respect to the pixels of the image (rather than the parameters of the model), that tells us which direction to adjust the pixels to make the loss go down - or up.
(The direction of steepest increase is just the opposite of the direction of steepest decrease.) A tiny step in that direction in imagespace perturbs the pixels of an image just so - making this one the tiniest bit darker, that one the tiniest bit lighter - in a way that humans don't even notice, but which completely breaks an image classifier sensitive to that direction in the conjunction of many pixel-dimensions, making it report utmost confidence in nonsense classifications.
Some might ask: why does it matter if our image classifier fails on examples that have been mathematically constructed to fool it? If it works for the images one would naturally encounter, isn't that good enough?
One might mundanely reply that gracefully handling untrusted inputs is a desideratum for many real-world applications, but a more forward-thinking reply might instead emphasize what adversarial examples imply about our lack of understanding of the systems we're building, separately from whether we pragmatically expect to face an adversary.
It's a problem if we think we've trained our machines to recognize birds, but they've actually learned to recognize a squiggly alien set in imagespace that includes a lot of obvious non-birds and excludes a lot of obvious birds. To plan good outcomes, we need to understand what's going on, and "The loss happens to increase in this direction" is at best only the start of a real explanation.
One obvious first guess as to what's going on is that the models are overfitting. Gradient descent isn't exactly a sophisticated algorithm. There's an intuition that the first solution that you happen to find by climbing down the loss landscape is likely to have idiosyncratic quirks on any inputs it wasn't trained for.
(And that an AI designer from a more competent civilization would use a principled understanding of vision to come up with something much better than what we get by shoveling compute into SGD.) Similarly, a hastily cobbled-together conventional computer program that passed a test suite is going to have bugs in areas not covered by the tests.
But that explanation is in tension with other evidence, like the observati...
Link to original articleWelcome to The Nonlinear Library, where we use Text-to-Speech software to convert the best writing from the Rationalist and EA communities into audio. This is: List your AI X-Risk cruxes!, published by Aryeh Englander on April 29, 2024 on LessWrong.
[I'm posting this as a very informal community request in lieu of a more detailed writeup, because if I wait to do this in a much more careful fashion then it probably won't happen at all. If someone else wants to do a more careful version that would be great!]
By crux here I mean some uncertainty you have such that your estimate for the likelihood of existential risk from AI - your "p(doom)" if you like that term - might shift significantly if that uncertainty were resolved.
More precisely, let's define a crux as a proposition such that: (a) your estimate for the likelihood of existential catastrophe due to AI would shift a non-trivial amount depending on whether that proposition was true or false; (b) you think there's at least a non-trivial probability that the proposition is true; and (c) you also think there's at least a non-trivial probability that the proposition is false.
Note 1: It could also be a variable rather than a binary proposition, for example "year human-level AGI is achieved". In that case substitute "variable is above some number x" and "variable is below some number y" instead of proposition is true / proposition is false.
Note 2: It doesn't have to be that the proposition / variable on it's own would significantly shift your estimate. If some combination of propositions / variables would shift your estimate, then those propositions / variables are cruxes at least when combined.
For concreteness let's say that "non-trivial" here means at least 5%. So you need to think there's at least a 5% chance the proposition is true, and at least a 5% chance that it's false, and also that your estimate for p(existential catastrophe due to AI) would shift by at least 5% depending on whether the proposition is true or false.
Here are just a few examples of potential cruxes people might have (among many others!):
Year human-level AGI is achieved
How fast the transition will be from much lower-capability AI to roughly human-level AGI, or from roughly human-level AGI to vastly superhuman AI
Whether power seeking will be an instrumentally convergent goal
Whether AI will greatly upset the offense-defense balance for CBRN technologies in a way that favors malicious actors
Whether AGIs could individually or collectively defeat humanity if they wanted to
Whether the world can collectively get their collective act together to pause AGI development given a clear enough signal (in combination with the probability that we'll in fact get a clear enough signal in time
Listing all your cruxes would be the most useful, but if that is too long a list then just list the ones you find most important. Providing additional details (for example, your probability distribution for each crux and/or how exactly it would shift your p(doom) estimates) is recommended if you can but isn't necessary.
Commenting with links to other related posts on LW or elsewhere might be useful as well.
Thanks for listening. To help us out with The Nonlinear Library or to learn more, please visit nonlinear.org
Link to original articleWelcome to The Nonlinear Library, where we use Text-to-Speech software to convert the best writing from the Rationalist and EA communities into audio. This is: [Aspiration-based designs] 1. Informal introduction, published by B Jacobs on April 29, 2024 on LessWrong.
Sequence Summary. This sequence documents research by SatisfIA, an ongoing project on non-maximizing, aspiration-based designs for AI agents that fulfill goals specified by constraints ("aspirations") rather than maximizing an objective function. We aim to contribute to AI safety by exploring design approaches and their software implementations that we believe might be promising but neglected or novel.
Our approach is roughly related to but largely complementary to concepts like quantilization and satisficing (sometimes called "soft-optimization"), Decision Transformers, and Active Inference.
This post describes the purpose of the sequence, motivates the research, describes the project status, our working hypotheses and theoretical framework, and has a short glossary of terms. It does not contain results and can safely be skipped if you want to get directly into the actual research.
Epistemic status: We're still in the exploratory phase, and while the project has yielded some preliminary insights, we don't have any clear conclusions at this point. Our team holds a wide variety of opinions about the discoveries. Nothing we say is set in stone.
Purpose of the sequence
Inform: We aim to share our current ideas, thoughts, disagreements, open questions, and any results we have achieved thus far. By openly discussing the complexities and challenges we face, we seek to provide a transparent view of our project's progression and the types of questions we're exploring.
Receive Feedback: We invite feedback on our approaches, hypotheses, and findings. Constructive criticism, alternative perspectives, and further suggestions are all welcome.
Attract Collaborators: Through this sequence, we hope to resonate with other researchers and practitioners who our exploration appeals to and who are motivated by similar questions. Our goal is to expand our team with individuals who can contribute their unique expertise and insights.
Motivation
We share a general concern regarding the trajectory of Artificial General Intelligence (AGI) development, particularly the risks associated with creating AGI agents designed to maximize objective functions. We have two main concerns:
(I) AGI development might be inevitable
(We assume this concern needs no further justification)
(II) It might be impossible to implement an objective function the maximization of which would be safe
The conventional view on A(G)I agents (see, e.g., Wikipedia) is that they should aim to maximize some function of the state or trajectory of the world, often called a "utility function", sometimes also called a "welfare function". It tacitly assumes that there is such an objective function that can adequately make the AGI behave in a moral way. However, this assumption faces several significant challenges:
Moral ambiguity: The notion that a universally acceptable, safe utility function exists is highly speculative. Given the philosophical debates surrounding moral cognitivism and moral realism and similar debates in welfare economics, it is possible that there are no universally agreeable moral truths, casting doubt on the existence of a utility function that encapsulates all relevant ethical considerations.
Historical track-record: Humanity's long-standing struggle to define and agree upon universal values or ethical standards raises skepticism about our capacity to discover or construct a comprehensive utility function that safely governs AGI behavior (Outer Alignment) in time.
Formal specification and Tractability: Even if a theoretically safe and comprehensive utility function could be conceptualized, the challenges of formalizing such a function into a computable and tractable form are immense. This includes the dif...
Link to original articleWelcome to The Nonlinear Library, where we use Text-to-Speech software to convert the best writing from the Rationalist and EA communities into audio. This is: We are headed into an extreme compute overhang, published by devrandom on April 28, 2024 on LessWrong.
If we achieve AGI-level performance using an LLM-like approach, the training hardware will be capable of running ~1,000,000s concurrent instances of the model.
Definitions
Although there is some debate about the definition of compute overhang, I believe that the AI Impacts definition matches the original use, and I prefer it: "enough computing hardware to run many powerful AI systems already exists by the time the software to run such systems is developed". A large compute overhang leads to additional risk due to faster takeoff.
I use the types of superintelligence defined in Bostrom's Superintelligence book (summary here).
I use the definition of AGI in this Metaculus question. The adversarial Turing test portion of the definition is not very relevant to this post.
Thesis
Due to practical reasons, the compute requirements for training LLMs is several orders of magnitude larger than what is required for running a single inference instance. In particular, a single NVIDIA H100 GPU can run inference at a throughput of about 2000 tokens/s, while Meta trained Llama3 70B on a GPU cluster[1] of about 24,000 GPUs. Assuming we require a performance of 40 tokens/s, the training cluster can run 20004024000=1,200,000 concurrent instances of the resulting 70B model.
I will assume that the above ratios hold for an AGI level model. Considering the amount of data children absorb via the vision pathway, the amount of training data for LLMs may not be that much higher than the data humans are trained on, and so the current ratios are a useful anchor. This is explored further in the appendix.
Given the above ratios, we will have the capacity for ~1e6 AGI instances at the moment that training is complete. This will likely lead to superintelligence via "collective superintelligence" approach. Additional speed may be then available via accelerators such as GroqChip, which produces 300 tokens/s for a single instance of a 70B model. This would result in a "speed superintelligence" or a combined "speed+collective superintelligence".
From AGI to ASI
With 1e6 AGIs, we may be able to construct an ASI, with the AGIs collaborating in a "collective superintelligence". Similar to groups of collaborating humans, a collective superintelligence divides tasks among its members for concurrent execution.
AGIs derived from the same model are likely to collaborate more effectively than humans because their weights are identical. Any fine-tune can be applied to all members, and text produced by one can be understood by all members.
Tasks that are inherently serial would benefit more from a speedup instead of a division of tasks. An accelerator such as GroqChip will be able to accelerate serial thought speed by a factor of 10x or more.
Counterpoints
It may be the case that a collective of
sub-AGI models can reach AGI capability. It would be advantageous if we could achieve AGI earlier, with sub-AGI components, at a higher hardware cost per instance. This will reduce the compute overhang at the critical point in time.
There may a paradigm change on the path to AGI resulting in smaller training clusters, reducing the overhang at the critical point.
Conclusion
A single AGI may be able to replace one human worker, presenting minimal risk. A fleet of 1,000,000 AGIs may give rise to a collective superintelligence. This capability is likely to be available immediately upon training the AGI model.
We may be able to mitigate the overhang by achieving AGI with a cluster of sub-AGI components.
Appendix - Training Data Volume
A calculation of training data processed by humans during development:
time: ~20 years, or 6e8 seconds
raw data input: ~10 mb/s = 1e7 b/s
total for human training data:
6e15 bits
Llama3 training s...
Link to original articleWelcome to The Nonlinear Library, where we use Text-to-Speech software to convert the best writing from the Rationalist and EA communities into audio. This is: So What's Up With PUFAs Chemically?, published by J Bostock on April 27, 2024 on LessWrong.
This is exploratory investigation of a new-ish hypothesis, it is not intended to be a comprehensive review of the field or even a a full investigation of the hypothesis.
I've always been skeptical of the seed-oil theory of obesity. Perhaps this is bad rationality on my part, but I've tended to retreat to the sniff test on issues as charged and confusing as diet. My response to the general seed-oil theory was basically "Really? Seeds and nuts? The things you just find growing on plants, and that our ancestors surely ate loads of?"
But a twitter thread recently made me take another look at it, and since I have a lot of chemistry experience I thought I'd take a look.
The PUFA Breakdown Theory
It goes like this:
PUFAs from nuts and seeds are fine. Deep-frying using PUFAs causes them to break down in a way other fatty acids do not, and these breakdown products are the problem.
Most of a fatty acid is the "tail". This consists of hydrogen atoms decorating a backbone of carbon atoms. Each carbon atom can make up to four bonds, of which two have to be to other carbons (except the end carbon which only bonds to one carbon) leaving space for two hydrogens. When a chain has the maximum number of hydrogen atoms, we say it's "saturated". These tails have the general formula CnH2n+1:
For a carbon which is saturated (i.e. has four single bonds) the bonds are arranged like the corners of a tetrahedron, and rotation around single bonds is permitted, meaning the overall assembly is like a floppy chain.
Instead, we can have two adjacent carbons form a double bond, each forming one bond to hydrogen, two bonds to the adjacent carbon, and one to a different carbon:
Unlike single bonds, double bonds are rigid, and if a carbon atom has a double bond, the three remaining bonds fall in a plane. This means there are two ways in which the rest of the chain can be laid out. If the carbons form a zig-zag S shape, this is a trans double bond. If they form a curved C shape, we have a cis double bond.
The health dangers of trans-fatty acids have been known for a long while. They don't occur in nature (which is probably why they're so bad for us). Cis-fatty acids are very common though, especially in vegetable and, yes, seed oils.
Of course there's no reason why we should stop at one double bond, we can just as easily have multiple. This gets us to the name poly-unsaturated fatty acids (PUFAs). I'll compare stearic acid (SA) oleic acid (OA) and linoleic acid (LA) for clarity:
Linoleic acid is the one that seed oil enthusiasts are most interested in. We can go even further and look at α-linoleic acid, which has even more double bonds, but I think LA makes the point just fine.
Three fatty acids, usually identical ones, attach to one glycerol molecule to form a triglyceride.
Isomerization
As I mentioned earlier, double bonds are rigid, so if you have a cis double bond, it stays that way. Mostly. In chemistry a reaction is never impossible, the components are just insufficiently hot. If we heat up a cis-fatty acid to a sufficient temperature, the molecules will be able to access enough energy to flip. The rate of reactions generally scales with temperature according to the Arrhenius equation:
v=Aexp(EakBT)
Where A is a general constant determining the speed, Ea is the "activation energy" of the reaction, T is temperature, and kB is a Boltzmann's constant which makes the units work out. Graphing this gives the following shape:
Suffice to say this means that reaction speed can grow very rapidly with temperature at the "right" point on this graph.
Why is this important? Well, trans-fatty acids are slightly lower energy than cis ones, so at a high enough temperature, we can see cis to trans isomerization, turning OA o...
Link to original articleWelcome to The Nonlinear Library, where we use Text-to-Speech software to convert the best writing from the Rationalist and EA communities into audio. This is: From Deep Learning to Constructability: Plainly-coded AGIs may be feasible in the near future, published by Épiphanie Gédéon on April 27, 2024 on LessWrong.
Charbel-Raphaël Segerie and Épiphanie Gédéon contributed equally to this post.
Many thanks to Davidad, Gabriel Alfour, Jérémy Andréoletti, Lucie Philippon, Vladimir Ivanov, Alexandre Variengien, Angélina Gentaz, Léo Dana and Diego Dorn for useful feedback.
TLDR: We present a new method for a safer-by design AI development. We think using plainly coded AIs may be feasible in the near future and may be safe. We also present a prototype and research ideas.
Epistemic status: Armchair reasoning style. We think the method we are proposing is interesting and could yield very positive outcomes (even though it is still speculative), but we are less sure about which safety policy would use it in the long run.
Current AIs are developed through deep learning: the AI tries something, gets it wrong, then gets backpropagated and all its weight adjusted. Then it tries again, wrong again, backpropagation again, and weights get adjusted again. Trial, error, backpropagation, trial, error, backpropagation, ad vitam eternam ad nauseam.
Of course, this leads to a severe lack of interpretability: AIs are essentially black boxes, and
we are not very optimistic about post-hoc interpretability.
We propose a different method: AI safety via pull request.[1]
By pull request, we mean that instead of modifying the neural network through successive backpropagations, we construct and design plainly-coded AIs (or hybrid systems) and explicitly modify its code using LLMs in a clear, readable, and modifiable way.
This plan may not be implementable right now, but might be as LLMs get smarter and faster. We want to outline it now so we can iterate on it early.
Overview
If the world released a powerful and autonomous agent in the wild,
white box or black box, or any color really,
humans might simply get replaced by AI.
What can we do in this context?
Don't create autonomous AGIs.
Keep your AGI controlled in a lab, and align it.
Create a minimal AGI controlled in a lab, and use it to produce safe artifacts.
This post focuses on this last path, and the specific artifacts that we want to create are plainly coded AIs (or hybrid systems)[2].
We present a method for developing such systems with a semi-automated training loop.
To do that, we start with a plainly coded system (that may also be built using LLMs) and iterate on its code, adding each feature and correction as pull requests that can be reviewed and integrated into the codebase.
This approach would allow AI systems that are, by design:
Transparent: As the system is written in plain or almost plain code, the system is more modular and understandable. As a result, it's simpler to spot backdoors, power-seeking behaviors, or inner misalignment: it is orders of magnitude simpler to refactor the system to have a part defining how it is evaluating its current situation and what it is aiming towards (if it is aiming at all). This means that if the system starts
farming cobras instead of capturing them, we would be able to see it.
Editable: If the system starts to learn unwanted correlations or features
such as learning to discriminate on feminine markers for a resume scorer - it is much easier to see it as a node in the AI code and remove it without retraining it.
Overseeable: We can ensure the system is well behaved by using automatic LLM reviews of the code and by using automatic unit tests of the isolated modules. In addition, we would use simulations and different settings necessary for safety, which we will describe later.
Version controlable: As all modifications are made through pull requests, we can easily trace with, e.g., git tooling where a specific modification was introduced and why.
In pract...
Link to original articleWelcome to The Nonlinear Library, where we use Text-to-Speech software to convert the best writing from the Rationalist and EA communities into audio. This is: D&D.Sci Long War: Defender of Data-mocracy, published by aphyer on April 27, 2024 on LessWrong.
This is an entry in the 'Dungeons & Data Science' series, a set of puzzles where players are given a dataset to analyze and an objective to pursue using information from that dataset.
STORY (skippable)
You have the excellent fortune to live under the governance of The People's Glorious Free Democratic Republic of Earth, giving you a Glorious life of Freedom and Democracy.
Sadly, your cherished values of Democracy and Freedom are under attack by...THE ALIEN MENACE!
Faced with the desperate need to defend Freedom and Democracy from The Alien Menace, The People's Glorious Free Democratic Republic of Earth has been forced to redirect most of its resources into the Glorious Free People's Democratic War Against The Alien Menace.
You haven't really paid much attention to the war, to be honest. Yes, you're sure it's Glorious and Free - oh, and Democratic too! - but mostly you've been studying Data Science and employing it in your Assigned Occupation as a Category Four Data Drone.
But you've grown tired of the Class Eight Habitation Module that you've been Democratically Allocated, and of your life as a Category Four Data Drone. And in order to have a voice in civic affairs (not to mention the chance to live somewhere nicer), you've enlisted with the Democratic People's Glorious Free Army in their Free Glorious People's Democratic War Against The Alien Menace.
You enlisted with the Tenth Democratic Free Glorious People's Mobilization, and were assigned to a training battalion under Sergeant Rico.
He's taught you a great deal about armed combat, unarmed combat, and how many pushups you can be forced to do before your arms give out.
You're sure the People's Glorious Free Democratic Army knows more than you about war in general. But you feel like the logistical and troop-deployment decisions being made are suboptimal, and you've been on the lookout for ways to employ your knowledge of Data Science to improve them.
So when you got your hands on a dataset of past deployments against the Alien Menace, you brought up with Sgt. Rico that you think you can use that to improve outcomes by selecting the right weapons loadout for each squad to bring.
In retrospect, when he leaned into your face and screamed: 'So you think you can do better, recruit?', that might have been intended as a rhetorical question, and you probably shouldn't have said yes.
Now you've been assigned to join a squad defending against an attack by the Alien Menace. At least he's agreed to let you choose how many soldiers to bring and how to equip them based on the data you collated (though you do rather suspect he's hoping the Alien Menace will eat you).
But with Data Science on your side, you're sure you can select a team that'll win the engagement, and hopefully he'll be more willing to listen to you after that. (Especially if you demonstrate that you can do it reliably and efficiently, without sending too large a squad that would draw manpower from other engagements).
For Glory! For The People! For Freedom! For Democracy! For The People's Glorious Free Democratic Republic of Earth! And for being allocated a larger and more pleasant Habitation Module and a higher-quality Nutrition Allotment!
DATA & OBJECTIVES
You've been assigned to repel an alien attack. The alien attack contains:
3 Arachnoid Abominations
2 Chitinous Crawlers
7 Swarming Scarabs
3 Towering Tyrants
1 Voracious Venompede
You need to select a squad of soldiers to bring with you. You may bring up to 10 soldiers, with any combination of the following weapons:
Antimatter Artillery
Fusion Flamethrower
Gluon Grenades
Laser Lance
Macross Minigun
Pulse Phaser
Rail Rifle
Thermo-Torpedos
So you could bring 10 soldiers all with Antimatter Artillery. Or you could brin...
Link to original articleWelcome to The Nonlinear Library, where we use Text-to-Speech software to convert the best writing from the Rationalist and EA communities into audio. This is: On Not Pulling The Ladder Up Behind You, published by Screwtape on April 27, 2024 on LessWrong.
Epistemic Status: Musing and speculation, but I think there's a real thing here.
I.
When I was a kid, a friend of mine had a tree fort. If you've never seen such a fort, imagine a series of wooden boards secured to a tree, creating a platform about fifteen feet off the ground where you can sit or stand and walk around the tree. This one had a rope ladder we used to get up and down, a length of knotted rope that was tied to the tree at the top and dangled over the edge so that it reached the ground.
Once you were up in the fort, you could pull the ladder up behind you. It was much, much harder to get into the fort without the ladder. Not only would you need to climb the tree itself instead of the ladder with its handholds, but you would then reach the underside of the fort and essentially have to do a pullup and haul your entire body up and over the edge instead of being able to pull yourself up a foot at a time on the rope. Only then could you let the rope back down.
The rope got pulled up a lot, mostly in games or childhood arguments with each other or our siblings. Sometimes it got pulled up out of boredom, fiddling with it or playing with the rope. Sometimes it got pulled up when we were trying to be helpful; it was easier for a younger kid to hold tight to the rope while two older kids pulled the rope up to haul the young kid into the tree fort.
"Pulling the ladder up behind you" is a metaphor for when you intentionally or unintentionally remove the easier way by which you reached some height.
II.
Quoth Ray,
Weird fact: a lot of people I know (myself included) gained a bunch of agency from running meetups.
When I arrived in the NYC community, I noticed an opportunity for some kind of winter holiday. I held the first Solstice. The only stakes were 20 people possibly having a bad time. The next year, I planned a larger event that people traveled from nearby cities to attend, which required me to learn some logistics as well as to improve at ritual design. The third year I was able to run a major event with a couple hundred attendees. At each point I felt challenged but not overwhelmed.
I made mistakes, but not ones that ruined anything longterm or important.
I'm a something of a serial inheritor[1] of meetups.
Last year I ran the Rationalist Megameetup in New York City, which had over a hundred people attending and took place at a conference hotel. It's the most complicated event I've run so far, but it didn't start that way. The first iteration of the megameetup was, as far as I know, inviting people to hang out at a big apartment and letting some of them crash on couches or air mattresses there.
That's pretty straightforward and something I can imagine a first-time organizer pulling off without too much stress. The first time I ran the megameetup, it involved renting an apartment and taking payments and buying a lot of food, but I was basically doing the exact same thing the person before me did and I got to ask a previous organizer a lot of questions.
This means that I got to slowly level up, getting more used to the existing tools and more comfortable in what I was doing as I made things bigger. There was a ladder there to let me climb up. If tomorrow I decided to stop having anything to do with the Rationalist Megameetup, I'd be leaving whoever picked up the torch after me with a harder climb. That problem is only going to get worse as the Rationalist Megameetup grows.
Projects have a tendency to grow more complicated the longer they go and the more successful they get. Meetups get bigger as more people join, codebases get larger as more features get added, companies wind up with a larger product line, fiction series add more characters and plotlines. That makes tak...
Link to original articleWelcome to The Nonlinear Library, where we use Text-to-Speech software to convert the best writing from the Rationalist and EA communities into audio. This is: Duct Tape security, published by Isaac King on April 26, 2024 on LessWrong.
This is a linkpost for On Duct Tape and Fence Posts.
Eliezer writes about fence post security. When people think to themselves "in the current system, what's the weakest point?", and then dedicate their resources to shoring up the defenses at that point, not realizing that after the first small improvement in that area, there's likely now a new weakest point somewhere else.
Fence post security happens preemptively, when the designers of the system fixate on the most salient aspect(s) and don't consider the rest of the system. But this sort of fixation can also happen in retrospect, in which case it manifest a little differently but has similarly deleterious effects.
Consider a car that starts shaking whenever it's driven. It's uncomfortable, so the owner gets a pillow to put on the seat. Items start falling off the dash, so they get a tray to put them in. A crack forms, so they tape over it.
I call these duct tape solutions. They address symptoms of the problem, but not the root cause. The underlying issue still exists and will continue to cause problems until it's addressed directly.1
Did you know it's illegal to trade onion futures in the United States? In 1955, some people cornered the market on onions, shorted onion futures, then flooded the market with their saved onions, causing a bunch of farmers to lose money. The government responded by banning the sale of futures contracts on onions.
Not by banning futures trading on all perishable items, which would be equally susceptible to such an exploit. Not by banning market-cornering in general, which is pretty universally disliked. By banning a futures contracts on onions specifically. So of course the next time someone wants to try such a thing, they can just do it with tomatoes.
Duct-tape fixes are common in the wake of anything that goes publicly wrong. When people get hurt, they demand change, and they pressure whoever is in charge to give it to them. But implementing a proper fix is generally more complicated (since you have to perform a root cause analysis), less visible (therefore not earning the leader any social credit), or just plain unnecessary (if the risk was already priced in).
So the incentives are in favor of quickly slapping something together that superficially appears to be a solution, without regards for whether it makes sense.
Of course not all changes in the wake of a disaster are duct-tape fixes. A competent organization looks at disasters as something that gives them new information about the system in question; they then think about how they would design the system from scratch taking that information into account, and proceed from there to make changes. Proper solutions involve attempts to fix a general class of issues, not just the exact thing that failed.
Bad: "Screw #8463 needs to be reinforced."
Better: "The unexpected failure of screw #8463 demonstrates that the structural simulation we ran before construction contained a bug. Let's fix that bug and re-run the simulation, then reinforce every component that falls below the new predicted failure threshold."
Even better: "The fact that a single bug in our simulation software could cause a catastrophic failure is unacceptable. We need to implement multiple separate methods of advance modeling and testing that won't all fail in the same way if one of them contains a flaw."
Ideal: "The fact that we had such an unsafe design process in the first place means we likely have severe institutional disfunction. We need to hire some experienced safety/security professionals and give them the authority necessary to identify any other flaws that may exist in our company, including whatever processes in our leadership and hiring teams led to us not having such a security team ...
Link to original articleWelcome to The Nonlinear Library, where we use Text-to-Speech software to convert the best writing from the Rationalist and EA communities into audio. This is: Scaling of AI training runs will slow down after GPT-5, published by Maxime Riché on April 26, 2024 on LessWrong.
My credence: 33% confidence in the claim that the growth in the number of GPUs used for training SOTA AI will slow down significantly directly after GPT-5. It is not higher because of (1) decentralized training is possible, and (2) GPT-5 may be able to increase hardware efficiency significantly, (3) GPT-5 may be smaller than assumed in this post, (4) race dynamics.
TLDR: Because of a bottleneck in energy access to data centers and the need to build OOM larger data centers.
Update: See Vladimir_Nesov's comment below for why this claim is likely wrong, since decentralized training seems to be solved.
The reasoning behind the claim:
Current large data centers consume around 100 MW of power, while a single nuclear power plant generates 1GW. The largest seems to consume 150 MW.
An A100 GPU uses 250W, and around 1kW with overheard. B200 GPUs, uses ~1kW without overhead. Thus a 1MW data center can support maximum 1k to 2k GPUs.
GPT-4 used something like 15k to 25k GPUs to train, thus around 15 to 25MW.
Large data centers are around 10-100 MW. This is likely one of the reason why top AI labs are mostly only using ~ GPT-4 level of FLOPS to train new models.
GPT-5 will mark the end of the fast scaling of training runs.
A 10-fold increase in the number of GPUs above GPT-5 would require a 1 to 2.5 GW data center, which doesn't exist and would take years to build, OR would require decentralized training using several data centers. Thus GPT-5 is expected to mark a significant slowdown in scaling runs. The power consumption required to continue scaling at the current rate is becoming unsustainable, as it would require the equivalent of multiple nuclear power plants.
I think this is basically what Sam Altman, Elon Musk and Mark Zuckerberg are saying in public interviews.
The main focus to increase capabilities will be one more time on improving software efficiency. In the next few years, investment will also focus on scaling at inference time and decentralized training using several data centers.
If GPT-5 doesn't unlock research capabilities, then after GPT-5, scaling capabilities will slow down for some time towards historical rates, with most gains coming from software improvements, a bit from hardware improvement, and significantly less than currently from scaling spending.
Scaling GPUs will be slowed down by regulations on lands, energy production, and build time. Training data centers may be located and built in low-regulation countries. E.g., the Middle East for cheap land, fast construction, low regulation, and cheap energy, thus maybe explaining some talks with Middle East investors.
Unrelated to the claim:
Hopefully, GPT-5 is still insufficient for self-improvement:
Research has pretty long horizon tasks that may require several OOM more compute.
More accurate world models may be necessary for longer horizon tasks and especially for research (hopefully requiring the use of compute-inefficient real, non-noisy data, e.g., real video).
"Hopefully", moving to above human level requires RL.
"Hopefully", RL training to finetune agents is still several OOM less efficient than pretraining and/or is currently too noisy to improve the world model (this is different than simply shaping propensities) and doesn't work in the end.
Guessing that GPT-5 will be at expert human level on short horizon tasks but not on long horizon tasks nor on doing research (improving SOTA), and we can't scale as fast as currently above that.
How big is that effect going to be?
Using values from: https://epochai.org/blog/the-longest-training-run, we have estimates that in a year, the effective compute is increased by:
Software efficiency: x1.7/year (1 OOM in 3.9 y)
Hardware efficiency: x1.3/year ...
Link to original articleWelcome to The Nonlinear Library, where we use Text-to-Speech software to convert the best writing from the Rationalist and EA communities into audio. This is: Spatial attention as a "tell" for empathetic simulation?, published by Steven Byrnes on April 26, 2024 on LessWrong.
(Half-baked work-in-progress. There might be a "version 2" of this post at some point, with fewer mistakes, and more neuroscience details, and nice illustrations and pedagogy etc. But it's fun to chat and see if anyone has thoughts.)
1. Background
There's a neuroscience problem that's had me stumped since almost the very beginning of when I became interested in neuroscience at all (as a lens into AGI safety) back in 2019. But I think I might finally have "a foot in the door" towards a solution!
What is this problem? As described in my post
Symbol Grounding and Human Social Instincts, I believe the following:
(1) We can divide the brain into a "Learning Subsystem" (cortex, striatum, amygdala, cerebellum and a few other areas) on the one hand, and a "Steering Subsystem" (mostly hypothalamus and brainstem) on the other hand; and a human's "innate drives" (roughly equivalent to the reward function in reinforcement learning) are calculated by a bunch of specific, genetically-specified
"business logic" housed in the latter subsystem;
(2) Some of those "innate drives" are related to human social instincts - a suite of reactions that are upstream of things like envy and compassion;
(3) It might be helpful for AGI safety (for reasons briefly summarized
here) if we understood exactly how those particular drives worked. Ideally this would look like legible pseudocode that's simultaneously compatible with behavioral observations (including everyday experience), with evolutionary considerations, and with a neuroscience-based story of how that pseudocode is actually implemented by neurons in the brain. (
Different example of what I think it looks like to make progress towards that kind of pseudocode.)
(4) Explaining how those innate drives work is tricky in part because of the "symbol grounding problem", but it probably centrally involves "transient empathetic simulations" (see
§13.5 of the post linked at the top);
(5) …and therefore there needs to be some mechanism in the brain by which the "Steering Subsystem" (hypothalamus & brainstem) can tell whether the "Learning Subsystem" (cortex etc.) world-model is being queried for the purpose of a "transient empathetic simulation", or whether that same world-model is instead being queried for some other purpose, like recalling a memory, considering a possible plan, or perceiving what's happening right now.
As an example of (5), if Zoe is yelling at me, then when I look at Zoe, a thought might flash across my mind, for a fraction of a second, wherein I mentally simulate Zoe's angry feelings. Alternatively, I might imagine myself potentially feeling angry in the future. Both of those possible thoughts involve my cortex sending a weak but legible-to-the-brainstem ("grounded") anger-related signal to the hypothalamus and brainstem (mainly via the amygdala) (I claim).
But the hypothalamus and brainstem have presumably evolved to trigger different reactions in those two cases, because the former but not the latter calls for a specific social reaction to Zoe's anger. For example, in the former case, maybe Zoe's anger would trigger in me a reaction to feel anger back at Zoe in turn, although not necessarily because there are other inputs to the calculation as well.
So I think there has to be some mechanism by which the hypothalamus and/or brainstem can figure out whether or not a (transient) empathetic simulation was upstream of those anger-related signals. And I don't know what that mechanism is.
I came into those five beliefs above rather quickly - the first time I mentioned that I was confused about how (5) works, it was way back in my
second-ever neuroscience blog post, maybe within the first 50 hours of my trying to teach m...
Link to original articleWelcome to The Nonlinear Library, where we use Text-to-Speech software to convert the best writing from the Rationalist and EA communities into audio. This is: Losing Faith In Contrarianism, published by omnizoid on April 26, 2024 on LessWrong.
Crosspost from my blog.
If you spend a lot of time in the blogosphere, you'll find a great deal of people expressing contrarian views. If you hang out in the circles that I do, you'll probably have heard of
Yudkowsky say that dieting doesn't really work, Guzey say that sleep is overrated, Hanson argue that medicine doesn't improve health, various people argue for the lab leak, others argue for hereditarianism, Caplan argue that mental illness is mostly just aberrant preferences and education doesn't work, and various other people expressing contrarian views.
Often, very smart people - like Robin Hanson - will write long posts defending these views, other people will have criticisms, and it will all be such a tangled mess that you don't really know what to think about them.
For a while, I took a lot of these contrarian views pretty seriously. If I'd had to bet 6-months ago, I'd have bet on the lab leak, at maybe 2 to 1 odds. I'd have had significant credence in Hanson's view that healthcare doesn't improve health until pretty recently, when Scott released his post explaining why it is wrong.
Over time, though, I've become much less sympathetic to these contrarian views. It's become increasingly obvious that the things that make them catch on are unrelated to their truth. People like being provocative and tearing down sacred cows - as a result, when a smart articulate person comes along defending some contrarian view - perhaps one claiming that something we think is valuable is really worthless - the view spreads like wildfire, even if it's pretty implausible.
Sam Atis has an article titled
The Case Against Public Intellectuals. He starts it by noting a surprising fact: lots of his friends think education has no benefits. This isn't because they've done a thorough investigation of the literature - it's because they've read Bryan Caplan's book arguing for that thesis. Atis notes that there's a literature review finding that education has significant benefits, yet it's written by boring academics, so no one has read it.
Everyone wants to read the contrarians who criticize education - no one wants to read the boring lit reviews that say what we believed about education all along is right.
Sam is right, yet I think he understates the problem. There are various topics where arguing for one side of them is inherently interesting, yet arguing for the other side is boring. There are a lot of people who read Austian economics blogs, yet no one reads (or writes) anti-Austrian economics blogs. That's because there are a lot of fans of Austrians economics - people who are willing to read blogs on the subject - but almost no one who is really invested in Austrian economics being wrong.
So as a result, in general, the structural incentives of the blogosphere favor being a contrarian.
Thus, you should expect the sense of the debate you get, unless you peruse the academic literature in depth surrounding some topic, to be wildly skewed towards contrarian views. And I think this is exactly what we observe.
I've seen the contrarians be wrong over and over again - and this is what really made me lose faith in them. Whenever I looked more into a topic, whenever I got to the bottom of the full debate, it always seemed like the contrarian case fell apart.
It's easy for contrarians to portray their opponents as the kind of milquetoast bureaucrats who aren't very smart and follow the consensus just because it is the consensus. If Bryan Caplan has a disagreement with a random administrator, I trust that Bryan Caplan's probably right, because he's smarter and cares more about ideas.
But what I've come to realize is that the mainstream view that's supported by most of the academics tends to be supported by some r...
Link to original articleWelcome to The Nonlinear Library, where we use Text-to-Speech software to convert the best writing from the Rationalist and EA communities into audio. This is: LLMs seem (relatively) safe, published by JustisMills on April 26, 2024 on LessWrong.
Post for a somewhat more general audience than the modal LessWrong reader, but gets at my actual thoughts on the topic.
In 2018 OpenAI
defeated the world champions of Dota 2, a major esports game. This was hot on the heels of DeepMind's AlphaGo performance
against Lee Sedol in 2016, achieving superhuman Go performance way before anyone thought that might happen. AI benchmarks were being cleared at a pace which felt breathtaking at the time, papers were proudly published, and ML tools like Tensorflow (released in 2015) were coming online. To people already interested in AI, it was an exciting era. To everyone else, the world was unchanged.
Now Saturday Night Live sketches use sober discussions of AI risk
as the backdrop for their actual jokes, there are
hundreds of AI bills moving through the world's legislatures, and Eliezer Yudkowsky
is featured in Time Magazine.
For people who have been predicting, since well before AI was cool (and now passe), that it could spell doom for humanity, this explosion of mainstream attention is a dark portent. Billion dollar AI companies keep springing up and allying with the largest tech companies in the world, and bottlenecks like money, energy, and talent are widening considerably. If current approaches can get us to superhuman AI in principle, it seems like they will in practice, and soon.
But what if large language models, the vanguard of the AI movement, are actually safer than what came before? What if the path we're on is less perilous than what we might have hoped for, back in 2017? It seems that way to me.
LLMs are self limiting
To train a large language model, you need an absolutely massive amount of data. The core thing these models are doing is predicting the next few letters of text, over and over again, and they need to be trained on billions and billions of words of human-generated text to get good at it.
Compare this process to
AlphaZero, DeepMind's algorithm that superhumanly masters Chess, Go, and Shogi. AlphaZero trains by playing against itself. While older chess engines bootstrap themselves by observing the records of countless human games, AlphaZero simply learns by doing. Which means that the only bottleneck for training it is computation - given enough energy, it can just play itself forever, and keep getting new data.
Not so with LLMs: their source of data is human-produced text, and human-produced text is a finite resource.
The precise datasets used to train cutting-edge LLMs are secret, but let's suppose that they include a fair bit of the low hanging fruit: maybe 5% of publicly available text that is in principle available and not garbage. You can schlep your way to a 20x bigger dataset in that case, though you'll hit diminishing returns as you have to, for example, generate transcripts of random videos and filter old mailing list threads for metadata and spam.
But nothing you do is going to get you 1,000x the training data, at least not in the short run.
Scaling laws are among the watershed discoveries of ML research in the last decade; basically, these are equations that project how much oomph you get out of increasing the size, training time, and dataset that go into a model. And as it turns out, the amount of high quality data is extremely important, and often becomes the bottleneck.
It's easy to take this fact for granted now, but it wasn't always obvious! If computational power or model size was usually the bottleneck, we could just make bigger and bigger computers and reliably get smarter and smarter AIs. But that only works to a point, because it turns out we need high quality data too, and high quality data is finite (and, as the political apparatus wakes up to what's going on,
legally fraught).
There are rumbling...
Link to original articleWelcome to The Nonlinear Library, where we use Text-to-Speech software to convert the best writing from the Rationalist and EA communities into audio. This is: WSJ: Inside Amazon's Secret Operation to Gather Intel on Rivals, published by trevor on April 25, 2024 on LessWrong.
The operation, called Big River Services International, sells around $1 million a year of goods through e-commerce marketplaces including
eBay,
Shopify,
Walmart and
Amazon AMZN 1.49%increase; green up pointing triangle.com under brand names such as Rapid Cascade and Svea Bliss. "We are entrepreneurs, thinkers, marketers and creators," Big River says on its website. "We have a passion for customers and aren't afraid to experiment."
What the website doesn't say is that Big River is an arm of Amazon that surreptitiously gathers intelligence on the tech giant's competitors.
Born out of a 2015 plan code named "Project Curiosity," Big River uses its sales across multiple countries to obtain pricing data, logistics information and other details about rival e-commerce marketplaces,
logistics operations and payments services, according to people familiar with Big River and corporate documents viewed by The Wall Street Journal. The team then shared that information with Amazon to incorporate into decisions about its own business.
...
The story of Big River offers new insight into Amazon's
elaborate efforts to stay ahead of rivals. Team members attended their rivals' seller conferences and met with competitors identifying themselves only as employees of Big River Services, instead of disclosing that they worked for Amazon.
They were given non-Amazon email addresses to use externally - in emails with people at Amazon, they used Amazon email addresses - and took other extraordinary measures to keep the project secret. They disseminated their reports to Amazon executives using printed, numbered copies rather than email. Those who worked on the project weren't even supposed to discuss the relationship internally with most teams at Amazon.
An internal crisis-management paper gave advice on what to say if discovered. The response to questions should be: "We make a variety of products available to customers through a number of subsidiaries and online channels." In conversations, in the event of a leak they were told to focus on the group being formed to improve the seller experience on Amazon, and say that such research is normal, according to people familiar with the discussions.
Senior Amazon executives, including Doug Herrington, Amazon's current CEO of Worldwide Amazon Stores, were regularly briefed on the Project Curiosity team's work, according to one of the people familiar with Big River.
...
Virtually all companies research their competitors, reading public documents for information, buying their products or shopping their stores. Lawyers say there is a difference between such corporate intelligence gathering of publicly available information, and what is known as corporate or industrial espionage.
Companies can get into legal trouble for actions such as hiring a rival's former employee to obtain trade secrets or hacking a rival. Misrepresenting themselves to competitors to gain proprietary information can lead to suits on trade secret misappropriation, said Elizabeth Rowe, a professor at the University of Virginia School of Law who specializes in trade secret law.
...
The benchmarking team pitched "Project Curiosity" to senior management and got the approval to buy inventory, use a shell company and find warehouses in the U.S., Germany, England, India and Japan so they could pose as sellers on competitors' websites.
...
Once launched, the focus of the project quickly started shifting to gathering information about rivals, the people said.
...
The team presented its findings from being part of the FedEx program to senior Amazon logistics leaders. They used the code name "OnTime Inc." to refer to FedEx. Amazon made changes to its Fulfillment by Amazon service to ...
Link to original articleWelcome to The Nonlinear Library, where we use Text-to-Speech software to convert the best writing from the Rationalist and EA communities into audio. This is: "Why I Write" by George Orwell (1946), published by Arjun Panickssery on April 25, 2024 on LessWrong.
People have been posting great essays so that they're "fed through the standard LessWrong algorithm." This essay is in the public domain in the UK but not the US.
From a very early age, perhaps the age of five or six, I knew that when I grew up I should be a writer. Between the ages of about seventeen and twenty-four I tried to abandon this idea, but I did so with the consciousness that I was outraging my true nature and that sooner or later I should have to settle down and write books.
I was the middle child of three, but there was a gap of five years on either side, and I barely saw my father before I was eight. For this and other reasons I was somewhat lonely, and I soon developed disagreeable mannerisms which made me unpopular throughout my schooldays. I had the lonely child's habit of making up stories and holding conversations with imaginary persons, and I think from the very start my literary ambitions were mixed up with the feeling of being isolated and undervalued.
I knew that I had a facility with words and a power of facing unpleasant facts, and I felt that this created a sort of private world in which I could get my own back for my failure in everyday life. Nevertheless the volume of serious - i.e. seriously intended - writing which I produced all through my childhood and boyhood would not amount to half a dozen pages. I wrote my first poem at the age of four or five, my mother taking it down to dictation.
I cannot remember anything about it except that it was about a tiger and the tiger had 'chair-like teeth' - a good enough phrase, but I fancy the poem was a plagiarism of Blake's 'Tiger, Tiger'. At eleven, when the war or 1914-18 broke out, I wrote a patriotic poem which was printed in the local newspaper, as was another, two years later, on the death of Kitchener. From time to time, when I was a bit older, I wrote bad and usually unfinished 'nature poems' in the Georgian style.
I also, about twice, attempted a short story which was a ghastly failure. That was the total of the would-be serious work that I actually set down on paper during all those years.
However, throughout this time I did in a sense engage in literary activities. To begin with there was the made-to-order stuff which I produced quickly, easily and without much pleasure to myself. Apart from school work, I wrote vers d'occasion, semi-comic poems which I could turn out at what now seems to me astonishing speed - at fourteen I wrote a whole rhyming play, in imitation of Aristophanes, in about a week - and helped to edit school magazines, both printed and in manuscript.
These magazines were the most pitiful burlesque stuff that you could imagine, and I took far less trouble with them than I now would with the cheapest journalism. But side by side with all this, for fifteen years or more, I was carrying out a literary exercise of a quite different kind: this was the making up of a continuous "story" about myself, a sort of diary existing only in the mind. I believe this is a common habit of children and adolescents.
As a very small child I used to imagine that I was, say, Robin Hood, and picture myself as the hero of thrilling adventures, but quite soon my "story" ceased to be narcissistic in a crude way and became more and more a mere description of what I was doing and the things I saw. For minutes at a time this kind of thing would be running through my head: 'He pushed the door open and entered the room.
A yellow beam of sunlight, filtering through the muslin curtains, slanted on to the table, where a matchbox, half-open, lay beside the inkpot. With his right hand in his pocket he moved across to the window. Down in the street a tortoiseshell cat was chasing a dead leaf,' etc., etc. Thi...
Link to original articleWelcome to The Nonlinear Library, where we use Text-to-Speech software to convert the best writing from the Rationalist and EA communities into audio. This is: The first future and the best future, published by KatjaGrace on April 25, 2024 on LessWrong.
It seems to me worth trying to slow down AI development to steer successfully around the shoals of extinction and out to utopia.
But I was thinking lately: even if I didn't think there was any chance of extinction risk, it might still be worth prioritizing a lot of care over moving at maximal speed. Because there are many different possible AI futures, and I think there's a good chance that the initial direction affects the long term path, and different long term paths go to different places. The systems we build now will shape the next systems, and so forth.
If the first human-level-ish AI is brain emulations, I expect a quite different sequence of events to if it is GPT-ish.
People genuinely pushing for AI speed over care (rather than just feeling impotent) apparently think there is negligible risk of bad outcomes, but also they are asking to take the first future to which there is a path. Yet possible futures are a large space, and arguably we are in a rare plateau where we could climb very different hills, and get to much better futures.
Thanks for listening. To help us out with The Nonlinear Library or to learn more, please visit nonlinear.org