Discover
Thinking Machines: AI & Philosophy
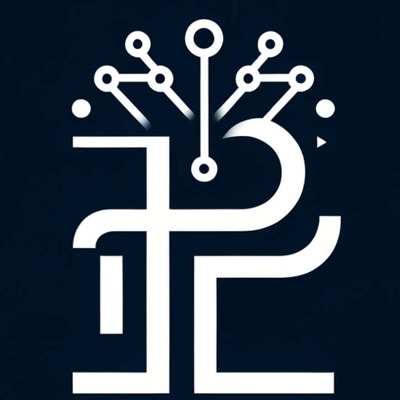
Thinking Machines: AI & Philosophy
Author: Daniel Reid Cahn
Subscribed: 2Played: 1Subscribe
Share
© 2024 Daniel Reid Cahn
Description
“Thinking Machines,” hosted by Daniel Reid Cahn, bridges the worlds of artificial intelligence and philosophy - aimed at technical audiences. Episodes explore how AI challenges our understanding of topics like consciousness, free will, and morality, featuring interviews with leading thinkers, AI leaders, founders, machine learning engineers, and philosophers. Daniel guides listeners through the complex landscape of artificial intelligence, questioning its impact on human knowledge, ethics, and the future.
We talk through the big questions that are bubbling through the AI community, covering topics like "Can AI be Creative?" and "Is the Turing Test outdated?", introduce new concepts to our vocabulary like "human washing," and only occasionally agree with each other.
Daniel is a machine learning engineer who misses his time as a philosopher at King's College London. Daniel is the cofounder and CEO of Slingshot AI, building the foundation model for psychology.
We talk through the big questions that are bubbling through the AI community, covering topics like "Can AI be Creative?" and "Is the Turing Test outdated?", introduce new concepts to our vocabulary like "human washing," and only occasionally agree with each other.
Daniel is a machine learning engineer who misses his time as a philosopher at King's College London. Daniel is the cofounder and CEO of Slingshot AI, building the foundation model for psychology.
22 Episodes
Reverse
Dev Rishi is the founder and CEO of Predibase, the company behind Ludwig and LoRAX. Predibase just released LoRA Land, a technical report showing 310 models that can outcompete GPT-4 on specific tasks through fine-tuning. In this episode, Dev tries (pretty successfully) to convince me that fine-tuning is the future, while answering a bunch of interesting questions, like:Is fine-tuning hard?If LoRAX is a competitive advantage for you, why open-source it?Is model hosting becoming commoditized? If so, how can anyone compete?What are people actually fine-tuning language models for?How worried are you about OpenAI eating your lunch?I had a ton of fun with Dev on this one. Also, check out Predibase’s newsletter called fine-tuned (great name!) and LoRA Land.
Talfan Evans is a research engineer at DeepMind, where he focuses on data curation and foundational research for pre-training LLMs and multimodal models like Gemini. I ask Talfan: Will one model rule them all?What does "high quality data" actually mean in the context of LLM training?Is language model pre-training becoming commoditized?Are companies like Google and OpenAI keeping their AI secrets to themselves?Does the startup or open source community stand a chance next to the giants?Also check out Talfan's latest paper at DeepMind, Bad Students Make Good Teachers.
"Understanding what's going on in a model is important to fine-tune it for specific tasks and to build trust."Bhavna Gopal is a PhD candidate at Duke, research intern at Slingshot with experience at Apple, Amazon and Vellum.We discussHow adversarial robustness research impacts the field of AI explainability.How do you evaluate a model's ability to generalize?What adversarial attacks should we be concerned about with LLMs?
Chris Gagne manages AI research at Hume, which just released an expressive text-to-speech model in a super impressive demo. Chris and Daniel discuss AI and emotional understanding:How does “prosody” add a dimension to human communication? What is Hume hoping to gain by adding it to Human-AI communication?Do we want to interact with AI like we interact with humans? Or should the interaction models be different?Are we entering the Uncanny Valley phase of emotionally intelligent AI?Do LLMs actually have the ability to reason about emotions? Does it matter?What do we risk, by empowering AI with emotional understanding? Are there risks from deception and manipulation? Or even a loss of human agency?
Former OpenAI Research Scientist Joel Lehman joins to discuss the non-linear nature of technological progress and the present day implications of his book, Why Greatness Cannot Be Planned.Joel co-authored the book with Kenneth Stanley back in 2015. The two did ML research at OpenAI, Uber, and the University of Central Florida and wrote the book based on insights from their work.We discuss:AI time horizons, and the Implications for investors, researchers, and entrepreneursExploration vs exploitation for AI startups and researchers, and where to fit in differential bets to avoid direct competitionThe stepping stones that will be crucial for AGIWhether startups should be training models or focusing on the AI application layerWhether to focus on scaling out transformers or to take alternative betsIs venture money going to the wrong place?
“Where are the good AI products?” asks Varun Shenoy, ML engineer in his latest blog post. Varun and I talk through:What are the cool applications that exist? Why aren't there more of them?What do (the few) good AI application companies have in common?What technological or societal leaps are blocking the existence of more AI apps that matter?The optimist case and the pessimist case for the near future of AI. As Varun puts it, what if the emperor has no clothes?We'd love to hear what you think. Feel free to reach out on LinkedIn or by email. You can reach me at daniel@slingshot.xyz.
ML Engineer and tech writer Donato Riccio wrote an article entitled "The End of RAG?" discussing what might replace Retrieval Augmented Generation in the near future. The article was received as highly controversial within the AI echo chamber, so I brought Donato on the podcast to discuss RAG, why people are so obsessed with vector databases, and the upcoming research in AI that might replace it.Takeaways:RAG is necessary due to LLMs' limited context window and scalability issues, and the need to avoid hallucinations and outdated information.Larger/infinite context window models and linear-scaling models (e.g. RWKV, Eagle) may allow for learning through forward propagation, allowing for more efficient and effective knowledge acquisitionAgentic flows are likely far more powerful than RAG - and when they actually start working consistently, we may see the need for vector databases dramatically reduced.RAG libraries and abstracts can be helpful for getting off the ground but don't solve the hard problems in specific vertical LLM use cases.RAG vs Agents, and the complex ways that vertical AI approach RAG in practiceShare your thoughts with us at hello@slingshot.xyz or tweet us @slingshot_ai.
What’s going on with GPUs? We talk through the GPU bottleneck/supply gut, Meta’s apparent 600,000 H100-equivalents and the future of the GPU cloud.Neel Master is the CEO and founder of Cedana, enabling pause/migrate/resume for compute jobs. Neel is a serial entrepreneur, former founder of Engooden and angel investor. He started his career in ML research at MIT's CSAIL.Topics from this podcast include:Cedana's real-time save, migrate and resume for compute technology, enabling the migration of compute jobs across instances without interruptionWhen will there be enough GPUs?What does it mean, that the cloud can become a robot?How might compute change, as per-capita GPU supply grows massively?How much does it actually cost to run GPUs?How can startups compete with the Big Tech’s giant compute moats?Share your thoughts with us at hello@slingshot.xyz or tweet us @slingshot_ai.
Founders of Lingopal, Deven Orie and Casey Schneider, join to talk about their startup story, developing real-time translation software for enterprises.Topics include:Why is translation so hard?How are enterprise and consumer AI products different (e.g. Google Translate vs Lingopal)?Should AI product companies be doing AI research?Is it safe to rely on open source?Share your thoughts with us at hello@slingshot.xyz or tweet us @slingshot_ai.
Founder of the SafeLlama community, Enoch Kan joins us today, to talk about safety in open source and medical AI. Enoch previously worked in AI for radiology, focused on mammography at Kheiron Medical. Enoch is an open source contributor, and his substack is called Cross Validated.Key topics they discuss include:New jailbreaks for LLMs appear every day. Does it matter?How do internet firewalls compare to AI “firewalls”?Why do human radiologists still exist? Would it be safe to replace them all today?Does safety matter more or less as models become more accurate?If regulation is too intense, could we end up with illegal consumer LLMs? For example, could we stop the masses from using an illegal AI doctor that you can access from your phone?Share your thoughts with us at hello@slingshot.xyz or tweet us @slingshot_ai.
Join Daniel Cahn on another SlingTalk episode with Kristian Freed (ex-CTO at Pariti and Elder), discussing the past, present and future of AI-assisted or AI-driven software.They talked about:The Evolution of Coding Tools: From basic text editors to advanced IDEs and the integration of AI tools like Co-Pilot.The Impact of AI on Software Development Practices: How AI is reshaping the way code is written and the process of software development.AI-Generated Code and Its Potential: Exploring the current capabilities of AI in generating code and its future implications.AI as a Mentor and Learning Aid: The role of AI in guiding developers through new languages and frameworks, acting like a digital coach.Challenges and Risks of AI in Software Development: Discussing the potential increase in mediocre code, security risks, and the future role of human oversightShare your thoughts with us at hello@slingshot.xyz or tweet us @slingshot_ai.
In 1950, Alan Turing asked, “Can machines think?” He suggested the Imitation Game as a test to evaluate whether a machine can think, more commonly called the “Turing Test.” Today we ask, is the Turing Test outdated? Joining Slingtalks this week are Kristian Freed & Guilherme Freire, founding engineers at Slingshot. Guilherme argues against the Turing Test, Kristian argues in favor.Key topics they discuss include:A recent paper claims that GPT-4 comes close to passing the Turing Test. Is the paper’s result valid? How close are we to passing the Turing Test?Defining the Turing Test and understanding the various iterations on its original framing since 1950. Are there levels in passing the Turing Test?Who is the Turing Test’s interrogator? And who is the human participant?If AI could pass the Turing Test, would that necessarily mean that most remote employees would be redundant?Is an AI’s ability to emulate human-like intelligence and deceive humans sufficient for intelligence? Is it necessary?What are the moral and philosophical implications of AI passing the Turing Test? Is intelligence morally significant? Is consciousness relevant?Share your thoughts with us at hello@slingshot.xyz or tweet us @slingshot_ai.
Join Daniel Cahn on SlingTalks as he welcomes Jonathan Pedoeem (Founder of PromptLayer) to talk through Prompt Engineering. This episode offers an in-depth look into the past, present, and future of prompt engineering and the intricacies of crafting effective AI prompts.Key topics they discuss include:Is prompt engineering more art or more science?The role of “prompt engineer” and whether prompt engineering is a highly specialized skill or a skill as common as GooglingApproaches to evaluating prompts - in theory and in practiceTechniques for AI-driven prompt engineeringOpenAI’s new “GPTs” feature, and how it will change the landscape of prompt-engineered botsPromptLayer and dev tools for prompt engineeringShare your thoughts with us at hello@slingshot.xyz or tweet us @slingshot_ai.
Adam Kirsh (Head of Product & Engineering, Stealth Startup) joins Slingshot to talk about how AI is transforming investment due diligence.Beyond AI in diligence, we discuss:“Horizontal” and “vertical” business models, that start from a point solutionBuilding products vs. building relationships, and on being an AI partner for the enterpriseAI-native startups and the reinvention of business models for the AI ageMaking incremental progress as a startup vs. visionary top-down redesigns of entire processes or even industriesConsumer vs. enterprise applications of LLMs (analogy: iPhones vs Blackberrys)We wrap up with some thought-provoking reflections and an open invitation for further discussion. We'd love to hear them! Drop an email at hello@slingshot.xyz or reach out on Twitter: @slingshot_ai.
Ex-Datadog Founding PM, Ayush Kapur, joins Daniel Cahn on SlingTalks to talk through the overloaded term, "Human in the Loop". They hone in on the impact of both, emotional and philosophical aspects of human interactions, for instance, your interaction with a doctor, and how those services can be considered irreplaceable by AI.Key topics include:Human-in-the-loop as human review vs. partially automated processesUse cases where human-in-the-loop makes automation useless because humans have to repeat the exact same steps as the AI.Liability and “human-washing,” where a human-in-the-loop is used for masking decisions effectively made by AI.The psychological aspects of trusting a decision that's viewed as human vs. AIFuture scenarios where decision-making is fully delegated to AIGot something to say about AI and the human touch? Share your thoughts and reach out at hello@slingshot.xyz or tweet us @slingshot_ai. We want to hear from you!
AI is increasingly doing the heavy lifting in our communications and content generation. On this episode, Guilherme Freire, Founding ML Engineer at Slingshot, joins the podcast to discuss the impact of AI-generated content.Some of the topics discussed:“Proof of Work” for humans, when AI makes personalization and connection too easyPotential for subpar AI-generated to put high-quality content creation out of businessHyper-personalized AI leading to greater divisiveness and bias; or on the flip side, for AI to serve as a "benevolent dictator" nudging us towards better habits and more diverse thinkingThe changing meaning of “free speech” in the age of AIThe “Boring Utopia” eventuality, where AI processes scientific information at scale to generate new insightsHave thoughts? We'd love to hear them! Drop an email at hello@slingshot.xyz or reach out on Twitter: @slingshot_ai.
Daniel hosts our machine learning research intern and Cambridge Masters student, Andy Lo, to talk about the present and future of ML programming. Topics include:PyTorch vs. TensorFlow vs. Jax vs MoJoNo-code, low-code and pro-code for ML engineersThe (frustrating) world of debugging ML codeHave thoughts? We'd love to hear them! Drop an email at hello@slingshot.xyz or reach out on Twitter: @slingshot_ai.
In this episode, Daniel shares his perspective on the opportunities for the next wave of AI-native startups.Machine Learning isn’t just about sentiment classification, churn prediction, and revenue forecasting anymore. Generative models can simulate real intelligence. But hard problems continue to require hard solutions, and prompt engineering with retrieval augmented generation won’t be nearly enough.Have thoughts? We'd love to hear them! Drop an email at hello@slingshot.xyz or reach out on Twitter: @slingshot_ai.
Daniel hosts our Founding Engineer, Edwin Zhang to unravel the balance in Design Driven Developments. Key things they cover:The conundrums faced when balancing user wants with real, valuable needs - showcasing our stance on "Doing what people need, not just what they want."A peek into the futuristic vision of browsers like Arc and how we regard the Browser Company.The harmonious dance between engineering-driven, customer-driven, data-driven, and design-driven developments.The delicate art of prioritising features based on feasibility, utility, and the tech's cool factor.The transformative power of machine learning in reshaping user interactions and breaking new grounds.Have thoughts? We'd love to hear them! Drop an email at hello@slingshot.xyz or reach out on Twitter: @slingshot_ai.
In this tech talk, we dive deep into the technical specifics around LLM inference.The big question is: Why are LLMs slow? How can they be faster? And might slow inference affect UX in the next generation of AI-powered software?We jump into:Is fast model inference the real moat for LLM companies?What are the implications of slow model inference on the future of decentralized and edge model inference?As demand rises, what will the latency/throughput tradeoff look like?What innovations on the horizon might massively speed up model inference?
Comments