Discover
Towards Data Science
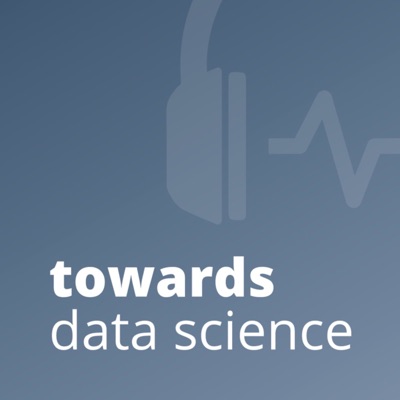
132 Episodes
Reverse
Progress in AI has been accelerating dramatically in recent years, and even months. It seems like every other day, there’s a new, previously-believed-to-be-impossible feat of AI that’s achieved by a world-leading lab. And increasingly, these breakthroughs have been driven by the same, simple idea: AI scaling.
For those who haven’t been following the AI scaling sage, scaling means training AI systems with larger models, using increasingly absurd quantities of data and processing power. So far, empirical studies by the world’s top AI labs seem to suggest that scaling is an open-ended process that can lead to more and more capable and intelligent systems, with no clear limit.
And that’s led many people to speculate that scaling might usher in a new era of broadly human-level or even superhuman AI — the holy grail AI researchers have been after for decades.
And while that might sound cool, an AI that can solve general reasoning problems as well as or better than a human might actually be an intrinsically dangerous thing to build.
At least, that’s the conclusion that many AI safety researchers have come to following the publication of a new line of research that explores how modern AI systems tend to solve problems, and whether we should expect more advanced versions of them to perform dangerous behaviours like seeking power.
This line of research in AI safety is called “power-seeking”, and although it’s currently not well understood outside the frontier of AI safety and AI alignment research, it’s starting to draw a lot of attention. The first major theoretical study of power seeking was led by Alex Turner, who’s appeared on the podcast before, and was published in NeurIPS (the world’s top AI conference), for example.
And today, we’ll be hearing from Edouard Harris, an AI alignment researcher and one of my co-founders in the AI safety company (Gladstone AI). Ed’s just completed a significant piece of AI safety research that extends Alex Turner’s original power-seeking work, and that shows what seems to be the first experimental evidence suggesting that we should expect highly advanced AI systems to seek power by default.
What does power seeking really mean though? And does all this imply for the safety of future, general-purpose reasoning systems? That’s what this episode will be all about.
***
Intro music:
- Artist: Ron Gelinas
- Track Title: Daybreak Chill Blend (original mix)
- Link to Track: https://youtu.be/d8Y2sKIgFWc
***
Chapters:
- 0:00 Intro
- 4:00 Alex Turner's research
- 7:45 What technology wants
- 11:30 Universal goals
- 17:30 Connecting observations
- 24:00 Micro power seeking behaviour
- 28:15 Ed's research
- 38:00 The human as the environment
- 42:30 What leads to power seeking
- 48:00 Competition as a default outcome
- 52:45 General concern
- 57:30 Wrap-up
It’s no secret that a new generation of powerful and highly scaled language models is taking the world by storm. Companies like OpenAI, AI21Labs, and Cohere have built models so versatile that they’re powering hundreds of new applications, and unlocking entire new markets for AI-generated text.
In light of that, I thought it would be worth exploring the applied side of language modelling — to dive deep into one specific language model-powered tool, to understand what it means to build apps on top of scaled AI systems. How easily can these models be used in the wild? What bottlenecks and challenges do people run into when they try to build apps powered by large language models? That’s what I wanted to find out.
My guest today is Amber Teng, and she’s a data scientist who recently published a blog that got quite a bit of attention, about a resume cover letter generator that she created using GPT-3, OpenAI’s powerful and now-famous language model. I thought her project would be make for a great episode, because it exposes so many of the challenges and opportunities that come with the new era of powerful language models that we’ve just entered.
So today we’ll be exploring exactly that: looking at the applied side of language modelling and prompt engineering, understanding how large language models have made new apps not only possible but also much easier to build, and the likely future of AI-powered products.
***
Intro music:
- Artist: Ron Gelinas
- Track Title: Daybreak Chill Blend (original mix)
- Link to Track: https://youtu.be/d8Y2sKIgFWc
***
Chapters:
- 0:00 Intro
- 2:30 Amber’s background
- 5:30 Using GPT-3
- 14:45 Building prompts up
- 18:15 Prompting best practices
- 21:45 GPT-3 mistakes
- 25:30 Context windows
- 30:00 End-to-end time
- 34:45 The cost of one cover letter
- 37:00 The analytics
- 41:45 Dynamics around company-building
- 46:00 Commoditization of language modelling
- 51:00 Wrap-up
Imagine you’re a big hedge fund, and you want to go out and buy yourself some data. Data is really valuable for you — it’s literally going to shape your investment decisions and determine your outcomes.
But the moment you receive your data, a cold chill runs down your spine: how do you know your data supplier gave you the data they said they would? From your perspective, you’re staring down 100,000 rows in a spreadsheet, with no way to tell if half of them were made up — or maybe more for that matter.
This might seem like an obvious problem in hindsight, but it’s one most of us haven’t even thought of. We tend to assume that data is data, and that 100,000 rows in a spreadsheet is 100,000 legitimate samples.
The challenge of making sure you’re dealing with high-quality data, or at least that you have the data you think you do, is called data observability, and it’s surprisingly difficult to solve for at scale. In fact, there are now entire companies that specialize in exactly that — one of which is Zectonal, whose co-founder Dave Hirko will be joining us for today’s episode of the podcast.
Dave has spent his career understanding how to evaluate and monitor data at massive scale. He did that first at AWS in the early days of cloud computing, and now through Zectonal, where he’s working on strategies that allow companies to detect issues with their data — whether they’re caused by intentional data poisoning, or unintentional data quality problems. Dave joined me to talk about data observability, data as a new vector for cyberattacks, and the future of enterprise data management on this episode of the TDS podcast.
***
Intro music:
- Artist: Ron Gelinas
- Track Title: Daybreak Chill Blend (original mix)
- Link to Track: https://youtu.be/d8Y2sKIgFWc
***
Chapters:
0:00 Intro
3:00 What is data observability?
10:45 “Funny business” with data providers
12:50 Data supply chains
16:50 Various cybersecurity implications
20:30 Deep data inspection
27:20 Observed direction of change
34:00 Steps the average person can take
41:15 Challenges with GDPR transitions
48:45 Wrap-up
Today, we live in the era of AI scaling. It seems like everywhere you look people are pushing to make large language models larger, or more multi-modal and leveraging ungodly amounts of processing power to do it.
But although that’s one of the defining trends of the modern AI era, it’s not the only one. At the far opposite extreme from the world of hyperscale transformers and giant dense nets is the fast-evolving world of TinyML, where the goal is to pack AI systems onto small edge devices.
My guest today is Matthew Stewart, a deep learning and TinyML researcher at Harvard University, where he collaborates with the world’s leading IoT and TinyML experts on projects aimed at getting small devices to do big things with AI. Recently, along with his colleagues, Matt co-authored a paper that introduced a new way of thinking about sensing.
The idea is to tightly integrate machine learning and sensing on one device. For example, today we might have a sensor like a camera embedded on an edge device, and that camera would have to send data about all the pixels in its field of view back to a central server that might take that data and use it to perform a task like facial recognition. But that’s not great because it involves sending potentially sensitive data — in this case, images of people’s faces — from an edge device to a server, introducing security risks.
So instead, what if the camera’s output was processed on the edge device itself, so that all that had to be sent to the server was much less sensitive information, like whether or not a given face was detected? These systems — where edge devices harness onboard AI, and share only processed outputs with the rest of the world — are what Matt and his colleagues call ML sensors.
ML sensors really do seem like they’ll be part of the future, and they introduce a host of challenging ethical, privacy, and operational questions that I discussed with Matt on this episode of the TDS podcast.
***
Intro music:
- Artist: Ron Gelinas
- Track Title: Daybreak Chill Blend (original mix)
- Link to Track: https://youtu.be/d8Y2sKIgFWc
***
Chapters:
- 3:20 Special challenges with TinyML
- 9:00 Most challenging aspects of Matt’s work
- 12:30 ML sensors
- 21:30 Customizing the technology
- 24:45 Data sheets and ML sensors
- 31:30 Customers with their own custom software
- 36:00 Access to the algorithm
- 40:30 Wrap-up
Deep learning models — transformers in particular — are defining the cutting edge of AI today. They’re based on an architecture called an artificial neural network, as you probably already know if you’re a regular Towards Data Science reader. And if you are, then you might also already know that as their name suggests, artificial neural networks were inspired by the structure and function of biological neural networks, like those that handle information processing in our brains.
So it’s a natural question to ask: how far does that analogy go? Today, deep neural networks can master an increasingly wide range of skills that were historically unique to humans — skills like creating images, or using language, planning, playing video games, and so on. Could that mean that these systems are processing information like the human brain, too?
To explore that question, we’ll be talking to JR King, a CNRS researcher at the Ecole Normale Supérieure, affiliated with Meta AI, where he leads the Brain & AI group. There, he works on identifying the computational basis of human intelligence, with a focus on language. JR is a remarkably insightful thinker, who’s spent a lot of time studying biological intelligence, where it comes from, and how it maps onto artificial intelligence. And he joined me to explore the fascinating intersection of biological and artificial information processing on this episode of the TDS podcast.
***
Intro music:
- Artist: Ron Gelinas
- Track Title: Daybreak Chill Blend (original mix)
- Link to Track: https://youtu.be/d8Y2sKIgFWc
***
Chapters:
2:30 What is JR’s day-to-day?
5:00 AI and neuroscience
12:15 Quality of signals within the research
21:30 Universality of structures
28:45 What makes up a brain?
37:00 Scaling AI systems
43:30 Growth of the human brain
48:45 Observing certain overlaps
55:30 Wrap-up
It’s no secret that the US and China are geopolitical rivals. And it’s also no secret that that rivalry extends into AI — an area both countries consider to be strategically critical.
But in a context where potentially transformative AI capabilities are being unlocked every few weeks, many of which lend themselves to military applications with hugely destabilizing potential, you might hope that the US and China would have robust agreements in place to deal with things like runaway conflict escalation triggered by an AI powered weapon that misfires. Even at the height of the cold war, the US and Russia had robust lines of communication to de-escalate potential nuclear conflicts, so surely the US and China have something at least as good in place now… right?
Well they don’t, and to understand the reason why — and what we should do about it — I’ll be speaking to Ryan Fedashuk, a Research Analyst at Georgetown University’s Center for Security and Emerging Technology and Adjunct Fellow at the Center for a New American Security. Ryan recently wrote a fascinating article for Foreign Policy Magazine, where he outlines the challenges and importance of US-China collaboration on AI safety. He joined me to talk about the U.S. and China’s shared interest in building safe AI, how reach side views the other, and what realistic China AI policy looks like on this episode of the TDs podcast.
There’s a website called thispersondoesnotexist.com. When you visit it, you’re confronted by a high-resolution, photorealistic AI-generated picture of a human face. As the website’s name suggests, there’s no human being on the face of the earth who looks quite like the person staring back at you on the page.
Each of those generated pictures are a piece of data that captures so much of the essence of what it means to look like a human being. And yet they do so without telling you anything whatsoever about any particular person. In that sense, it’s fully anonymous human face data.
That’s impressive enough, and it speaks to how far generative image models have come over the last decade. But what if we could do the same for any kind of data?
What if I could generate an anonymized set of medical records or financial transaction data that captures all of the latent relationships buried in a private dataset, without the risk of leaking sensitive information about real people? That’s the mission of Alex Watson, the Chief Product Officer and co-founder of Gretel AI, where he works on unlocking value hidden in sensitive datasets in ways that preserve privacy.
What I realized talking to Alex was that synthetic data is about much more than ensuring privacy. As you’ll see over the course of the conversation, we may well be heading for a world where most data can benefit from augmentation via data synthesis — where synthetic data brings privacy value almost as a side-effect of enriching ground truth data with context imported from the wider world.
Alex joined me to talk about data privacy, data synthesis, and what could be the very strange future of the data lifecycle on this episode of the TDS podcast.
***
Intro music:
- Artist: Ron Gelinas
- Track Title: Daybreak Chill Blend (original mix)
- Link to Track: https://youtu.be/d8Y2sKIgFWc
***
Chapters:
2:40 What is synthetic data?
6:45 Large language models
11:30 Preventing data leakage
18:00 Generative versus downstream models
24:10 De-biasing and fairness
30:45 Using synthetic data
35:00 People consuming the data
41:00 Spotting correlations in the data
47:45 Generalization of different ML algorithms
51:15 Wrap-up
Two ML researchers with world-class pedigrees who decided to build a company that puts AI on the blockchain. Now to most people — myself included — “AI on the blockchain” sounds like a winning entry in some kind of startup buzzword bingo. But what I discovered talking to Jacob and Ala was that they actually have good reasons to combine those two ingredients together.
At a high level, doing AI on a blockchain allows you to decentralize AI research and reward labs for building better models, and not for publishing papers in flashy journals with often biased reviewers.
And that’s not all — as we’ll see, Ala and Jacob are taking on some of the thorniest current problems in AI with their decentralized approach to machine learning. Everything from the problem of designing robust benchmarks to rewarding good AI research and even the centralization of power in the hands of a few large companies building powerful AI systems — these problems are all in their sights as they build out Bittensor, their AI-on-the-blockchain-startup.
Ala and Jacob joined me to talk about all those things and more on this episode of the TDS podcast.
---
Intro music:
- Artist: Ron Gelinas
- Track Title: Daybreak Chill Blend (original mix)
- Link to Track: https://youtu.be/d8Y2sKIgFWc
---
Chapters:
2:40 Ala and Jacob’s backgrounds
4:00 The basics of AI on the blockchain
11:30 Generating human value
17:00 Who sees the benefit? 22:00 Use of GPUs
28:00 Models learning from each other
37:30 The size of the network
45:30 The alignment of these systems
51:00 Buying into a system
54:00 Wrap-up
As you might know if you follow the podcast, we usually talk about the world of cutting-edge AI capabilities, and some of the emerging safety risks and other challenges that the future of AI might bring. But I thought that for today’s episode, it would be fun to change things up a bit and talk about the applied side of data science, and how the field has evolved over the last year or two.
And I found the perfect guest to do that with: her name is Sadie St. Lawrence, and among other things, she’s the founder of Women in Data — a community that helps women enter the field of data and advance throughout their careers — and she’s also the host of the Data Bytes podcast, a seasoned data scientist and a community builder extraordinaire. Sadie joined me to talk about her founder’s journey, what data science looks like today, and even the possibilities that blockchains introduce for data science on this episode of the towards data science podcast.
***
Intro music:
- Artist: Ron Gelinas
- Track Title: Daybreak Chill Blend (original mix)
- Link to Track: https://youtu.be/d8Y2sKIgFWc
***
Chapters:
2:00 Founding Women in Data
6:30 Having gendered conversations
11:00 The cultural aspect
16:45 Opportunities in blockchain
22:00 The blockchain database
32:30 Data science education
37:00 GPT-3 and unstructured data
39:30 Data science as a career
42:50 Wrap-up
If the name data2vec sounds familiar, that’s probably because it made quite a splash on social and even traditional media when it came out, about two months ago. It’s an important entry in what is now a growing list of strategies that are focused on creating individual machine learning architectures that handle many different data types, like text, image and speech.
Most self-supervised learning techniques involve getting a model to take some input data (say, an image or a piece of text) and mask out certain components of those inputs (say by blacking out pixels or words) in order to get the models to predict those masked out components.
That “filling in the blanks” task is hard enough to force AIs to learn facts about their data that generalize well, but it also means training models to perform tasks that are very different depending on the input data type. Filling in blacked out pixels is quite different from filling in blanks in a sentence, for example.
So what if there was a way to come up with one task that we could use to train machine learning models on any kind of data? That’s where data2vec comes in.
For this episode of the podcast, I’m joined by Alexei Baevski, a researcher at Meta AI one of the creators of data2vec. In addition to data2vec, Alexei has been involved in quite a bit of pioneering work on text and speech models, including wav2vec, Facebook’s widely publicized unsupervised speech model. Alexei joined me to talk about how data2vec works and what’s next for that research direction, as well as the future of multi-modal learning.
***
Intro music:
- Artist: Ron Gelinas
- Track Title: Daybreak Chill Blend (original mix)
- Link to Track: https://youtu.be/d8Y2sKIgFWc
***
Chapters:
2:00 Alexei’s background
10:00 Software engineering knowledge
14:10 Role of data2vec in progression
30:00 Delta between student and teacher
38:30 Losing interpreting ability
41:45 Influence of greater abilities
49:15 Wrap-up
AI scaling has really taken off. Ever since GPT-3 came out, it’s become clear that one of the things we’ll need to do to move beyond narrow AI and towards more generally intelligent systems is going to be to massively scale up the size of our models, the amount of processing power they consume and the amount of data they’re trained on, all at the same time.
That’s led to a huge wave of highly scaled models that are incredibly expensive to train, largely because of their enormous compute budgets. But what if there was a more flexible way to scale AI — one that allowed us to decouple model size from compute budgets, so that we can track a more compute-efficient course to scale?
That’s the promise of so-called mixture of experts models, or MoEs. Unlike more traditional transformers, MoEs don’t update all of their parameters on every training pass. Instead, they route inputs intelligently to sub-models called experts, which can each specialize in different tasks. On a given training pass, only those experts have their parameters updated. The result is a sparse model, a more compute-efficient training process, and a new potential path to scale.
Google has been pushing the frontier of research on MoEs, and my two guests today in particular have been involved in pioneering work on that strategy (among many others!). Liam Fedus and Barrett Zoph are research scientists at Google Brain, and they joined me to talk about AI scaling, sparsity and the present and future of MoE models on this episode of the TDS podcast.
***
Intro music:
- Artist: Ron Gelinas
- Track Title: Daybreak Chill Blend (original mix)
- Link to Track: https://youtu.be/d8Y2sKIgFWc
***
Chapters:
2:15 Guests’ backgrounds
8:00 Understanding specialization
13:45 Speculations for the future
21:45 Switch transformer versus dense net
27:30 More interpretable models
33:30 Assumptions and biology
39:15 Wrap-up
There’s an idea in machine learning that most of the progress we see in AI doesn’t come from new algorithms of model architectures. instead, some argue, progress almost entirely comes from scaling up compute power, datasets and model sizes — and besides those three ingredients, nothing else really matters.
Through that lens the history of AI becomes the history f processing power and compute budgets. And if that turns out to be true, then we might be able to do a decent job of predicting AI progress by studying trends in compute power and their impact on AI development.
And that’s why I wanted to talk to Jaime Sevilla, an independent researcher and AI forecaster, and affiliate researcher at Cambridge University’s Centre for the Study of Existential Risk, where he works on technological forecasting and understanding trends in AI in particular. His work’s been cited in a lot of cool places, including Our World In Data, who used his team’s data to put together an exposé on trends in compute. Jaime joined me to talk about compute trends and AI forecasting on this episode of the TDS podcast.
***
Intro music:
- Artist: Ron Gelinas
- Track Title: Daybreak Chill Blend (original mix)
- Link to Track: https://youtu.be/d8Y2sKIgFWc
***
Chapters:
2:00 Trends in compute
4:30 Transformative AI
13:00 Industrial applications
19:00 GPT-3 and scaling
25:00 The two papers
33:00 Biological anchors
39:00 Timing of projects
43:00 The trade-off
47:45 Wrap-up
Generating well-referenced and accurate Wikipedia articles has always been an important problem: Wikipedia has essentially become the Internet's encyclopedia of record, and hundreds of millions of people use it do understand the world.
But over the last decade Wikipedia has also become a critical source of training data for data-hungry text generation models. As a result, any shortcomings in Wikipedia’s content are at risk of being amplified by the text generation tools of the future. If one type of topic or person is chronically under-represented in Wikipedia’s corpus, we can expect generative text models to mirror — or even amplify — that under-representation in their outputs.
Through that lens, the project of Wikipedia article generation is about much more than it seems — it’s quite literally about setting the scene for the language generation systems of the future, and empowering humans to guide those systems in more robust ways.
That’s why I wanted to talk to Meta AI researcher Angela Fan, whose latest project is focused on generating reliable, accurate, and structured Wikipedia articles. She joined me to talk about her work, the implications of high-quality long-form text generation, and the future of human/AI collaboration on this episode of the TDS podcast.
---
Intro music:
- Artist: Ron Gelinas
- Track Title: Daybreak Chill Blend (original mix)
- Link to Track: https://youtu.be/d8Y2sKIgFWc
---
Chapters:
1:45 Journey into Meta AI
5:45 Transition to Wikipedia
11:30 How articles are generated
18:00 Quality of text
21:30 Accuracy metrics
25:30 Risk of hallucinated facts
30:45 Keeping up with changes
36:15 UI/UX problems
45:00 Technical cause of gender imbalance
51:00 Wrap-up
Trustworthy AI is one of today’s most popular buzzwords. But although everyone seems to agree that we want AI to be trustworthy, definitions of trustworthiness are often fuzzy or inadequate. Maybe that shouldn’t be surprising: it’s hard to come up with a single set of standards that add up to “trustworthiness”, and that apply just as well to a Netflix movie recommendation as a self-driving car.
So maybe trustworthy AI needs to be thought of in a more nuanced way — one that reflects the intricacies of individual AI use cases. If that’s true, then new questions come up: who gets to define trustworthiness, and who bears responsibility when a lack of trustworthiness leads to harms like AI accidents, or undesired biases?
Through that lens, trustworthiness becomes a problem not just for algorithms, but for organizations. And that’s exactly the case that Beena Ammanath makes in her upcoming book, Trustworthy AI, which explores AI trustworthiness from a practical perspective, looking at what concrete steps companies can take to make their in-house AI work safer, better and more reliable. Beena joined me to talk about defining trustworthiness, explainability and robustness in AI, as well as the future of AI regulation and self-regulation on this episode of the TDS podcast.
Intro music:
- Artist: Ron Gelinas
- Track Title: Daybreak Chill Blend (original mix)
- Link to Track: https://youtu.be/d8Y2sKIgFWc
Chapters:
1:55 Background and trustworthy AI
7:30 Incentives to work on capabilities
13:40 Regulation at the level of application domain
16:45 Bridging the gap
23:30 Level of cognition offloaded to the AI
25:45 What is trustworthy AI?
34:00 Examples of robustness failures
36:45 Team diversity
40:15 Smaller companies
43:00 Application of best practices
46:30 Wrap-up
Until recently, very few people were paying attention to the potential malicious applications of AI. And that made some sense: in an era where AIs were narrow and had to be purpose-built for every application, you’d need an entire research team to develop AI tools for malicious applications. Since it’s more profitable (and safer) for that kind of talent to work in the legal economy, AI didn’t offer much low-hanging fruit for malicious actors.
But today, that’s all changing. As AI becomes more flexible and general, the link between the purpose for which an AI was built and its potential downstream applications has all but disappeared. Large language models can be trained to perform valuable tasks, like supporting writers, translating between languages, or write better code. But a system that can write an essay can also write a fake news article, or power an army of humanlike text-generating bots.
More than any other moment in the history of AI, the move to scaled, general-purpose foundation models has shown how AI can be a double-edged sword. And now that these models exist, we have to come to terms with them, and figure out how to build societies that remain stable in the face of compelling AI-generated content, and increasingly accessible AI-powered tools with malicious use potential.
That’s why I wanted to speak with Katya Sedova, a former Congressional Fellow and Microsoft alumna who now works at Georgetown University’s Center for Security and Emerging Technology, where she recently co-authored some fascinating work exploring current and likely future malicious uses of AI. If you like this conversation I’d really recommend checking out her team’s latest report — it’s called “AI and the future of disinformation campaigns”.
Katya joined me to talk about malicious AI-powered chatbots, fake news generation and the future of AI-augmented influence campaigns on this episode of the TDS podcast.
***
Intro music:
➞ Artist: Ron Gelinas
➞ Track Title: Daybreak Chill Blend (original mix)
➞ Link to Track: https://youtu.be/d8Y2sKIgFWc
***
Chapters:
2:40 Malicious uses of AI
4:30 Last 10 years in the field
7:50 Low handing fruit of automation
14:30 Other analytics functions
25:30 Authentic bots
30:00 Influences of service businesses
36:00 Race to the bottom
42:30 Automation of systems
50:00 Manufacturing norms
52:30 Interdisciplinary conversations
54:00 Wrap-up
Imagine, for example, an AI that’s trained to identify cows in images. Ideally, we’d want it to learn to detect cows based on their shape and colour. But what if the cow pictures we put in the training dataset always show cows standing on grass?
In that case, we have a spurious correlation between grass and cows, and if we’re not careful, our AI might learn to become a grass detector rather than a cow detector. Even worse, we could only realize that’s happened once we’ve deployed it in the real world and it runs into a cow that isn’t standing on grass for the first time.
So how do you build AI systems that can learn robust, general concepts that remain valid outside the context of their training data?
That’s the problem of out-of-distribution generalization, and it’s a central part of the research agenda of Irina Rish, a core member of the Mila— Quebec AI Research institute, and the Canadian Excellence Research Chair in Autonomous AI. Irina’s research explores many different strategies that aim to overcome the out-of-distribution problem, from empirical AI scaling efforts to more theoretical work, and she joined me to talk about just that on this episode of the podcast.
***
Intro music:
- Artist: Ron Gelinas
- Track Title: Daybreak Chill Blend (original mix)
- Link to Track: https://youtu.be/d8Y2sKIgFWc
***
Chapters:
2:00 Research, safety, and generalization
8:20 Invariant risk minimization
15:00 Importance of scaling
21:35 Role of language
27:40 AGI and scaling
32:30 GPT versus ResNet 50
37:00 Potential revolutions in architecture
42:30 Inductive bias aspect
46:00 New risks
49:30 Wrap-up
Google the phrase “AI over-hyped”, and you’ll find literally dozens of articles from the likes of Forbes, Wired, and Scientific American, all arguing that “AI isn’t really as impressive at it seems from the outside,” and “we still have a long way to go before we come up with *true* AI, don’t you know.”
Amusingly, despite the universality of the “AI is over-hyped” narrative, the statement that “We haven’t made as much progress in AI as you might think™️” is often framed as somehow being an edgy, contrarian thing to believe.
All that pressure not to over-hype AI research really gets to people — researchers included. And they adjust their behaviour accordingly: they over-hedge their claims, cite outdated and since-resolved failure modes of AI systems, and generally avoid drawing straight lines between points that clearly show AI progress exploding across the board. All, presumably, to avoid being perceived as AI over-hypers.
Why does this matter? Well for one, under-hyping AI allows us to stay asleep — to delay answering many of the fundamental societal questions that come up when widespread automation of labour is on the table. But perhaps more importantly, it reduces the perceived urgency of addressing critical problems in AI safety and AI alignment.
Yes, we need to be careful that we’re not over-hyping AI. “AI startups” that don’t use AI are a problem. Predictions that artificial general intelligence is almost certainly a year away are a problem. Confidently prophesying major breakthroughs over short timescales absolutely does harm the credibility of the field.
But at the same time, we can’t let ourselves be so cautious that we’re not accurately communicating the true extent of AI’s progress and potential. So what’s the right balance?
That’s where Sam Bowman comes in. Sam is a professor at NYU, where he does research on AI and language modeling. But most important for today’s purposes, he’s the author of a paper titled, “When combating AI hype, proceed with caution,” in which he explores a trend he calls under-claiming — a common practice among researchers that consists of under-stating the extent of current AI capabilities, and over-emphasizing failure modes in ways that can be (unintentionally) deceptive.
Sam joined me to talk about under-claiming and what it means for AI progress on this episode of the Towards Data Science podcast.
***
Intro music:
- Artist: Ron Gelinas
- Track Title: Daybreak Chill Blend (original mix)
- Link to Track: https://youtu.be/d8Y2sKIgFWc
***
Chapters:
2:15 Overview of the paper
8:50 Disappointing systems
13:05 Potential double standard
19:00 Moving away from multi-modality
23:50 Overall implications
28:15 Pressure to publish or perish
32:00 Announcement discrepancies
36:15 Policy angle
41:00 Recommendations
47:20 Wrap-up
It’s no secret that AI systems are being used in more and more high-stakes applications. As AI eats the world, it’s becoming critical to ensure that AI systems behave robustly — that they don’t get thrown off by unusual inputs, and start spitting out harmful predictions or recommending dangerous courses of action. If we’re going to have AI drive us to work, or decide who gets bank loans and who doesn’t, we’d better be confident that our AI systems aren’t going to fail because of a freak blizzard, or because some intern missed a minus sign.
We’re now past the point where companies can afford to treat AI development like a glorified Kaggle competition, in which the only thing that matters is how well models perform on a testing set. AI-powered screw-ups aren’t always life-or-death issues, but they can harm real users, and cause brand damage to companies that don’t anticipate them.
Fortunately, AI risk is starting to get more attention these days, and new companies — like Robust Intelligence — are stepping up to develop strategies that anticipate AI failures, and mitigate their effects. Joining me for this episode of the podcast was Yaron Singer, a former Googler, professor of computer science and applied math at Harvard, and now CEO and co-founder of Robust Intelligence. Yaron has the rare combination of theoretical and engineering expertise required to understand what AI risk is, and the product intuition to know how to integrate that understanding into solutions that can help developers and companies deal with AI risk.
---
Intro music:
➞ Artist: Ron Gelinas
➞ Track Title: Daybreak Chill Blend (original mix)
➞ Link to Track: https://youtu.be/d8Y2sKIgFWc
---
Chapters:
0:00 Intro
2:30 Journey into AI risk
5:20 Guarantees of AI systems
11:00 Testing as a solution
15:20 Generality and software versus custom work
18:55 Consistency across model types
24:40 Different model failures
30:25 Levels of responsibility
35:00 Wrap-up
Until very recently, the study of human disease involved looking at big things — like organs or macroscopic systems — and figuring out when and how they can stop working properly. But that’s all started to change: in recent decades, new techniques have allowed us to look at disease in a much more detailed way, by examining the behaviour and characteristics of single cells.
One class of those techniques now known as single-cell genomics — the study of gene expression and function at the level of single cells. Single-cell genomics is creating new, high-dimensional datasets consisting of tens of millions of cells whose gene expression profiles and other characteristics have been painstakingly measured. And these datasets are opening up exciting new opportunities for AI-powered drug discovery — opportunities that startups are now starting to tackle head-on.
Joining me for today’s episode is Tali Raveh, Senior Director of Computational Biology at Immunai, a startup that’s using single-cell level data to perform high resolution profiling of the immune system at industrial scale. Tali joined me to talk about what makes the immune system such an exciting frontier for modern medicine, and how single-cell data and AI might be poised to generate unprecedented breakthroughs in disease treatment on this episode of the TDS podcast.
---
Intro music:
➞ Artist: Ron Gelinas
➞ Track Title: Daybreak Chill Blend (original mix)
➞ Link to Track: https://youtu.be/d8Y2sKIgFWc
---
Chapters:
0:00 Intro
2:00 Tali’s background
4:00 Immune systems and modern medicine
14:40 Data collection technology
19:00 Exposing cells to different drugs
24:00 Labeled and unlabelled data
27:30 Dataset status
31:30 Recent algorithmic advances
36:00 Cancer and immunology
40:00 The next few years
41:30 Wrap-up
If you were scrolling through your newsfeed in late September 2021, you may have caught this splashy headline from The Times of London that read, “Can this man save the world from artificial intelligence?”. The man in question was Mo Gawdat, an entrepreneur and senior tech executive who spent several years as the Chief Business Officer at GoogleX (now called X Development), Google’s semi-secret research facility, that experiments with moonshot projects like self-driving cars, flying vehicles, and geothermal energy. At X, Mo was exposed to the absolute cutting edge of many fields — one of which was AI. His experience seeing AI systems learn and interact with the world raised red flags for him — hints of the potentially disastrous failure modes of the AI systems we might just end up with if we don’t get our act together now.
Mo writes about his experience as an insider at one of the world’s most secretive research labs and how it led him to worry about AI risk, but also about AI’s promise and potential in his new book, Scary Smart: The Future of Artificial Intelligence and How You Can Save Our World. He joined me to talk about just that on this episode of the TDS podcast.
Top Podcasts
The Best New Comedy Podcast Right Now – June 2024The Best News Podcast Right Now – June 2024The Best New Business Podcast Right Now – June 2024The Best New Sports Podcast Right Now – June 2024The Best New True Crime Podcast Right Now – June 2024The Best New Joe Rogan Experience Podcast Right Now – June 20The Best New Dan Bongino Show Podcast Right Now – June 20The Best New Mark Levin Podcast – June 2024
Always make yourself comfortable for doing your assignment because a cool and calm mind works better but if you are facing any difficulty then you can take Mechanical Engineering Assignment Help from Great Assignment Helper. https://greatassignmenthelper.com/mechanical-engineering-assignment-help/
The GPT-2 language model is an interesting subject for the research. I just want to say thank you for the article. I am going to pay to write research paper on https://studyclerk.com/pay-for-research-paper and will share this podcast with my expert. That's so helpful.