Discover
Training Data
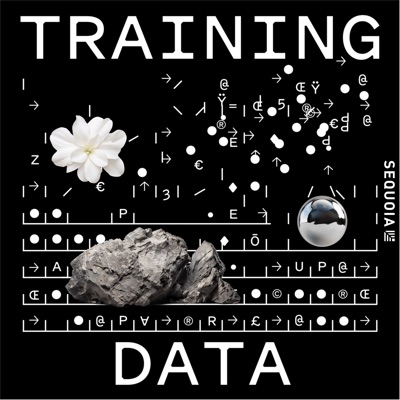
Training Data
Author: Sequoia Capital
Subscribed: 126Played: 1,453Subscribe
Share
Description
Join us as we train our neural nets on the theme of the century: AI. Sonya Huang, Pat Grady and more Sequoia Capital partners host conversations with leading AI builders and researchers to ask critical questions and develop a deeper understanding of the evolving technologies—and their implications for technology, business and society.
The content of this podcast does not constitute investment advice, an offer to provide investment advisory services, or an offer to sell or solicitation of an offer to buy an interest in any investment fund.
34 Episodes
Reverse
OpenEvidence is transforming how doctors access medical knowledge at the point of care, from the biggest medical establishments to small practices serving rural communities. Founder Daniel Nadler explains his team’s insight that training smaller, specialized AI models on peer-reviewed literature outperforms large general models for medical applications. He discusses how making the platform freely available to all physicians led to widespread organic adoption and strategic partnerships with publishers like the New England Journal of Medicine. In an industry where organizations move glacially, 10-20% of all U.S. doctors began using OpenEvidence overnight to find information buried deep in the long tail of new medical studies, to validate edge cases and improve diagnoses. Nadler emphasizes the importance of accuracy and transparency in AI healthcare applications.
Hosted by: Pat Grady, Sequoia Capital
Mentioned in this episode:
Do We Still Need Clinical Language Models?: Paper from OpenEvidence founders showing that small, specialized models outperformed large models for healthcare diagnostics
Chinchilla paper: Seminal 2022 paper about scaling laws in large language models
Understand: Ted Chiang sci-fi novella published in 1991
OpenAI’s Isa Fulford and Josh Tobin discuss how the company’s newest agent, Deep Research, represents a breakthrough in AI research capabilities by training models end-to-end rather than using hand-coded operational graphs. The product leads explain how high-quality training data and the o3 model’s reasoning abilities enable adaptable research strategies, and why OpenAI thinks Deep Research will capture a meaningful percentage of knowledge work. Key product decisions that build transparency and trust include citations and clarification flows. By compressing hours of work into minutes, Deep Research transforms what’s possible for many business and consumer use cases.
Hosted by: Sonya Huang and Lauren Reeder, Sequoia Capital
Mentioned in this episode:
Yann Lecun’s Cake: An analogy Meta AI’s leader shared in his 2016 NIPS keynote
Palo Alto Networks’s CEO Nikesh Arora dispels DeepSeek hype by detailing all of the guardrails enterprises need to have in place to give AI agents “arms and legs.” No matter the model, deploying applications for precision-use cases means superimposing better controls. Arora emphasizes that the real challenge isn’t just blocking threats but matching the accelerated pace of AI-powered attacks, requiring a fundamental shift from prevention-focused to real-time detection and response systems. CISOs are risk managers, but legacy companies competing with more risk-tolerant startups need to move quickly and embrace change.
Hosted by: Sonya Huang and Pat Grady, Sequoia Capital
Mentioned in this episode:
Cortex XSIAM: Security operations and incident remediation platform from Palo Alto Networks
MongoDB product leader Sahir Azam explains how vector databases have evolved from semantic search to become the essential memory and state layer for AI applications. He describes his view of how AI is transforming software development generally, and how combining vectors, graphs and traditional data structures enables high-quality retrieval needed for mission-critical enterprise AI use cases. Drawing from MongoDB's successful cloud transformation, Azam shares his vision for democratizing AI development by making sophisticated capabilities accessible to mainstream developers through integrated tools and abstractions.
Hosted by: Sonya Huang and Pat Grady, Sequoia Capital
Mentioned in this episode:
Introducing ambient agents: Blog post by Langchain on a new UX pattern where AI agents can listen to an event stream and act on it
Google Gemini Deep Research: Sahir enjoys its amazing product experience
Perplexity: AI search app that Sahir admires for its product craft
Snipd: AI powered podcast app Sahir likes
Stef Corazza leads generative AI development at Roblox after previously building Adobe’s 3D and AR platforms. His technical expertise, combined with Roblox’s unique relationship with its users, has led to the infusion of AI into its creation tools. Roblox has assembled the world’s largest multimodal dataset. Stef previews the Roblox Assistant and the company’s new 3D foundation model, while emphasizing the importance of maintaining positive experiences and civility on the platform.
Mentioned in this episode:
Driving Empire: A Roblox car racing game Stef particularly enjoys
RDC: Roblox Developer Conference
Ego.live: Roblox app to create and share synthetic worlds populated with human-like generative agents and simulated communities|
PINNs: Physics Informed Neural Networks
ControlNet: A model for controlling image diffusion by conditioning on an additional input image that Stef says can be used as a 2.5D approach to 3D generation.
Neural rendering: A combination of deep learning with computer graphics principles developed by Nvidia in its RTX platform
Hosted by: Konstantine Buhler and Sonya Huang, Sequoia Capital
Ioannis Antonoglou, founding engineer at DeepMind and co-founder of ReflectionAI, has seen the triumphs of reinforcement learning firsthand. From AlphaGo to AlphaZero and MuZero, Ioannis has built the most powerful agents in the world. Ioannis breaks down key moments in AlphaGo's game against Lee Sodol (Moves 37 and 78), the importance of self-play and the impact of scale, reliability, planning and in-context learning as core factors that will unlock the next level of progress in AI.
Hosted by: Stephanie Zhan and Sonya Huang, Sequoia Capital
Mentioned in this episode:
PPO: Proximal Policy Optimization algorithm developed by DeepMind in game environments. Also used by OpenAI for RLHF in ChatGPT.
MuJoCo: Open source physics engine used to develop PPO
Monte Carlo Tree Search: Heuristic search algorithm used in AlphaGo as well as video compression for YouTube and the self-driving system at Tesla
AlphaZero: The DeepMind model that taught itself from scratch how to master the games of chess, shogi and Go
MuZero: The DeepMind follow up to AlphaZero that mastered games without knowing the rules and able to plan winning strategies in unknown environments
AlphaChem: Chemical Synthesis Planning with Tree Search and Deep Neural Network Policies
DQN: Deep Q-Network, Introduced in 2013 paper, Playing Atari with Deep Reinforcement Learning
AlphaFold: DeepMind model for predicting protein structures for which Demis Hassabis, John Jumper and David Baker won the 2024 Nobel Prize in Chemistry
Hema Raghavan is co-founder of Kumo, a company that makes graph neural networks accessible to enterprises by connecting to their relational data stored in Snowflake and Databricks. Hema talks about how running GNNs on GPUs has led to breakthroughs in performance as well as the query language Kumo developed to help companies predict future data points. Although approachable for non-technical users, the product provides full control for data scientists who use Kumo to automate time-consuming feature engineering pipelines.
Mentioned in this episode:
Graph Neural Networks: Learning mechanism for data in graph format, the basis of the Kumo product
Graph RAG: Popular extension of retrieval-augmented generation using GNNs
LiGNN: Graph Neural Networks at LinkedIn paper
KDD: Knowledge Discovery and Data Mining Conference
Hosted by: Konstantine Buhler and Sonya Huang, Sequoia Capital
Berkeley professor Ion Stoica, co-founder of Databricks and Anyscale, transformed the open source projects Spark and Ray into successful AI infrastructure companies. He talks about what mattered most for Databricks' success -- the focus on making Spark win and making Databricks the best place to run Spark. He highlights the importance of striking key partnerships -- the Microsoft partnership in particular that accelerated Databricks' growth and contributed to Spark's dominance among data scientists and AI engineers. He also shares his perspective on finding new problems to work on, which holds lessons for aspiring founders and builders: 1) building systems in new areas that, if widely adopted, put you in the best position to understand the new problem space, and 2) focusing on a problem that is more important tomorrow than today.
Hosted by: Stephanie Zhan and Sonya Huang, Sequoia Capital
Mentioned in this episode:
Spark: The open source platform for data engineering that Databricks was originally based on.
Ray: Open source framework to manage, executes and optimizes compute needs across AI workloads, now productized through Anyscale
MosaicML: Generative AI startups founded by Naveen Rao that Databricks acquired in 2023.
Unity Catalog: Data and AI governance solution from Databricks.
CIB Berkeley: Multi-strategy hedge fund at UC Berkeley that commercializes research in the UC system.
Hadoop: A long-time leading platform for large scale distributed computing.
VLLM and Chatbot Arena: Two of Ion’s students’ projects that he wanted to highlight.
Oege de Moor, the creator of GitHub Copilot, discusses how XBOW’s AI offensive security system matches and even outperforms top human penetration testers, completing security assessments in minutes instead of days. The team’s speed and focus is transforming the niche market of pen testing with an always-on service-as-a-software platform. Oege describes how he is building a large and sustainable business while also creating a product that will “protect all the software in the free world.” XBOW shows how AI is essential for protecting software systems as the amount of AI-generated code increases along with the scale and sophistication of cyber threats.
Hosted by: Konstantine Buhler and Sonya Huang, Sequoia Capital
Mentioned in this episode:
Semmle: Oege’s previous startup, a code analysis tool to secure software, acquired in 2019 by GitHub
Nico Waisman: Head of security at XBOW, previously a researcher at Semmle
The Bitter Lesson: Highly influential post by Richard Sutton
HackerOne: Cybersecurity company that runs one of the largest bug bounty programs
Suno: AI songwriting app that Oege loves
Machines of Loving Grace: Essay by Anthropic founder, Dario Amodei
When ChatGPT ushered in a new paradigm of AI in everyday use, many companies attempted to adapt to the new paradigm by rushing to add chat interfaces to their products. Eric has a different take—he doesn’t think chatbots are the right form factor for everything. He thinks “zero-touch” automation that works invisibly in the background can be more valuable in many cases. He cites self-driving cars as an analogy—or in this case, “self-driving money.” Ramp is a new kind of finance management company for businesses, offering AI-powered financial tools to help companies handle spending and expense processes. We’ll hear why Eric thinks AI that you never see is one of the most powerful instruments for reducing time spent on drudgery and unlocking more time for meaningful work.
Hosted by: Ravi Gupta and Sonya Huang, Sequoia Capital
Mentioned in this episode:
Paribus: Glyman’s previous company, acquired by Capital One in 2016
Karim Atiyeh: Cofounder and CTO at Ramp and Glyman’s cofounder at Paribus
Devin: AI agent product from Cognition Labs and Glyman’s favorite AI app
Hit Refresh: Book by Satya Nadella
Founded in early 2023 after spending years at Stripe and OpenAI, Gabriel Hubert and Stanislas Polu started Dust with the view that one model will not rule them all, and that multi-model integration will be key to getting the most value out of AI assistants. In this episode we’ll hear why they believe the proprietary data you have in silos will be key to unlocking the full power of AI, get their perspective on the evolving model landscape, and how AI can augment rather than replace human capabilities.
Hosted by: Konstantine Buhler and Pat Grady, Sequoia Capital
00:00 - Introduction
02:16 - One model will not rule them all
07:15 - Reasoning breakthroughs
11:15 - Trends in AI models
13:32 - The future of the open source ecosystem
16:16 - Model quality and performance
21:44 - “No GPUs before PMF”
27:24 - Dust in action
37:40 - How do you find “the makers”
42:36 - The beliefs Dust lives by
50:03 - Keeping the human in the loop
52:33 - Second time founders
56:15 - Lightning round
Clay is leveraging AI to help go-to-market teams unleash creativity and be more effective in their work, powering custom workflows for everything from targeted outreach to personalized landing pages. It’s one of the fastest growing AI-native applications, with over 4,500 customers and 100,000 users. Founder and CEO Kareem Amin describes Clay’s technology, and its approach to balancing imagination and automation in order to help its customers achieve new levels of go-to-market success.
Hosted by: Alfred Lin, Sequoia Capital
Can GenAI allow us to connect our imagination to what we see on our screens? Decart’s Dean Leitersdorf believes it can.
In this episode, Dean Leitersdorf breaks down how Decart is pushing the boundaries of compute in order to create AI-generated consumer experiences, from fully playable video games to immersive worlds. From achieving real-time video inference on existing hardware to building a fully vertically integrated stack, Dean explains why solving fundamental limitations rather than specific problems could lead to the next trillion-dollar company.
Hosted by: Sonya Huang and Shaun Maguire, Sequoia Capital
00:00 Introduction
03:22 About Oasis
05:25 Solving a problem vs overcoming a limitation
08:42 The role of game engines
11:15 How video real-time inference works
14:10 World model vs pixel representation
17:17 Vertical integration
34:20 Building a moat
41:35 The future of consumer entertainment
43:17 Rapid fire questions
Years before co-founding Glean, Arvind was an early Google employee who helped design the search algorithm. Today, Glean is building search and work assistants inside the enterprise, which is arguably an even harder problem. One of the reasons enterprise search is so difficult is that each individual at the company has different permissions and access to different documents and information, meaning that every search needs to be fully personalized. Solving this difficult ingestion and ranking problem also unlocks a key problem for AI: feeding the right context into LLMs to make them useful for your enterprise context. Arvind and his team are harnessing generative AI to synthesize, make connections, and turbo-change knowledge work. Hear Arvind’s vision for what kind of work we’ll do when work AI assistants reach their potential.
Hosted by: Sonya Huang and Pat Grady, Sequoia Capital
00:00 - Introduction
08:35 - Search rankings
11:30 - Retrieval-Augmented Generation
15:52 - Where enterprise search meets RAG
19:13 - How is Glean changing work?
26:08 - Agentic reasoning
31:18 - Act 2: application platform
33:36 - Developers building on Glean
35:54 - 5 years into the future
38:48 - Advice for founders
In recent years there’s been an influx of theoretical physicists into the leading AI labs. Do they have unique capabilities suited to studying large models or is it just herd behavior? To find out, we talked to our former AI Fellow (and now OpenAI researcher) Dan Roberts.
Roberts, co-author of The Principles of Deep Learning Theory, is at the forefront of research that applies the tools of theoretical physics to another type of large complex system, deep neural networks. Dan believes that DLLs, and eventually LLMs, are interpretable in the same way a large collection of atoms is—at the system level. He also thinks that emphasis on scaling laws will balance with new ideas and architectures over time as scaling asymptotes economically.
Hosted by: Sonya Huang and Pat Grady, Sequoia Capital
Mentioned in this episode:
The Principles of Deep Learning Theory: An Effective Theory Approach to Understanding Neural Networks, by Daniel A. Roberts, Sho Yaida, Boris Hanin
Black Holes and the Intelligence Explosion: Extreme scenarios of AI focus on what is logically possible rather than what is physically possible. What does physics have to say about AI risk?
Yang-Mills & The Mass Gap: An unsolved Millennium Prize problem
AI Math Olympiad: Dan is on the prize committee
NotebookLM from Google Labs has become the breakout viral AI product of the year. The feature that catapulted it to viral fame is Audio Overview, which generates eerily realistic two-host podcast audio from any input you upload—written doc, audio or video file, or even a PDF. But to describe NotebookLM as a “podcast generator” is to vastly undersell it. The real magic of the product is in offering multi-modal dimensions to explore your own content in new ways—with context that’s surprisingly additive. 200-page training manuals become synthesized into digestible chapters, turned into a 10-minute podcast—or both—and shared with the sales team, just to cite one example. Raiza Martin and Jason Speilman join us to discuss how the magic happens, and what’s next for source-grounded AI.
Hosted by: Sonya Huang and Pat Grady, Sequoia Capital
All of us as consumers have felt the magic of ChatGPT—but also the occasional errors and hallucinations that make off-the-shelf language models problematic for business use cases with no tolerance for errors. Case in point: A model deployed to help create a summary for this episode stated that Sridhar Ramaswamy previously led PyTorch at Meta. He did not. He spent years running Google’s ads business and now serves as CEO of Snowflake, which he describes as the data cloud for the AI era.
Ramaswamy discusses how smart systems design helped Snowflake create reliable "talk-to-your-data" applications with over 90% accuracy, compared to around 45% for out-of-the-box solutions using off the shelf LLMs. He describes Snowflake's commitment to making reliable AI simple for their customers, turning complex software engineering projects into straightforward tasks.
Finally, he stresses that even as frontier models progress, there is significant value to be unlocked from current models by applying them more effectively across various domains.
Hosted by: Sonya Huang and Pat Grady, Sequoia Capital
Mentioned in this episode:
Cortex Analyst: Snowflake’s talk-to-your-data API
Document AI: Snowflake feature that extracts in structured information from documents
Combining LLMs with AlphaGo-style deep reinforcement learning has been a holy grail for many leading AI labs, and with o1 (aka Strawberry) we are seeing the most general merging of the two modes to date. o1 is admittedly better at math than essay writing, but it has already achieved SOTA on a number of math, coding and reasoning benchmarks.
Deep RL legend and now OpenAI researcher Noam Brown and teammates Ilge Akkaya and Hunter Lightman discuss the ah-ha moments on the way to the release of o1, how it uses chains of thought and backtracking to think through problems, the discovery of strong test-time compute scaling laws and what to expect as the model gets better.
Hosted by: Sonya Huang and Pat Grady, Sequoia Capital
Mentioned in this episode:
Learning to Reason with LLMs: Technical report accompanying the launch of OpenAI o1.
Generator verifier gap: Concept Noam explains in terms of what kinds of problems benefit from more inference-time compute.
Agent57: Outperforming the human Atari benchmark, 2020 paper where DeepMind demonstrated “the first deep reinforcement learning agent to obtain a score that is above the human baseline on all 57 Atari 2600 games.”
Move 37: Pivotal move in AlphaGo’s second game against Lee Sedol where it made a move so surprising that Sedol thought it must be a mistake, and only later discovered he had lost the game to a superhuman move.
IOI competition: OpenAI entered o1 into the International Olympiad in Informatics and received a Silver Medal.
System 1, System 2: The thesis if Danial Khaneman’s pivotal book of behavioral economics, Thinking, Fast and Slow, that positied two distinct modes of thought, with System 1 being fast and instinctive and System 2 being slow and rational.
AlphaZero: The predecessor to AlphaGo which learned a variety of games completely from scratch through self-play. Interestingly, self-play doesn’t seem to have a role in o1.
Solving Rubik’s Cube with a robot hand: Early OpenAI robotics paper that Ilge Akkaya worked on.
The Last Question: Science fiction story by Isaac Asimov with interesting parallels to scaling inference-time compute.
Strawberry: Why?
O1-mini: A smaller, more efficient version of 1 for applications that require reasoning without broad world knowledge.
00:00 - Introduction
01:33 - Conviction in o1
04:24 - How o1 works
05:04 - What is reasoning?
07:02 - Lessons from gameplay
09:14 - Generation vs verification
10:31 - What is surprising about o1 so far
11:37 - The trough of disillusionment
14:03 - Applying deep RL
14:45 - o1’s AlphaGo moment?
17:38 - A-ha moments
21:10 - Why is o1 good at STEM?
24:10 - Capabilities vs usefulness
25:29 - Defining AGI
26:13 - The importance of reasoning
28:39 - Chain of thought
30:41 - Implication of inference-time scaling laws
35:10 - Bottlenecks to scaling test-time compute
38:46 - Biggest misunderstanding about o1?
41:13 - o1-mini
42:15 - How should founders think about o1?
Adding code to LLM training data is a known method of improving a model’s reasoning skills. But wouldn’t math, the basis of all reasoning, be even better? Up until recently, there just wasn’t enough usable data that describes mathematics to make this feasible.
A few years ago, Vlad Tenev (also founder of Robinhood) and Tudor Achim noticed the rise of the community around an esoteric programming language called Lean that was gaining traction among mathematicians. The combination of that and the past decade’s rise of autoregressive models capable of fast, flexible learning made them think the time was now and they founded Harmonic. Their mission is both lofty—mathematical superintelligence—and imminently practical, verifying all safety-critical software.
Hosted by: Sonya Huang and Pat Grady, Sequoia Capital
Mentioned in this episode:
IMO and the Millennium Prize: Two significant global competitions Harmonic hopes to win (soon)
Riemann hypothesis: One of the most difficult unsolved math conjectures (and a Millenium Prize problem) most recently in the sights of MIT mathematician Larry Guth
Terry Tao: perhaps the greatest living mathematician and Vlad’s professor at UCLA
Lean: an open source functional language for code verification launched by Leonardo de Moura when at Microsoft Research in 2013 that powers the Lean Theorem Prover
mathlib: the largest math textbook in the world, all written in Lean
Metaculus: online prediction platform that tracks and scores thousands of forecasters
Minecraft Beaten in 20 Seconds: The video Vlad references as an analogy to AI math
Navier-Stokes equations: another important Millenium Prize math problem. Vlad considers this more tractable that Riemann
John von Neumann: Hungarian mathematician and polymath that made foundational contributions to computing, the Manhattan Project and game theory
Gottfried Wilhelm Leibniz: co-inventor of calculus and (remarkably) creator of the “universal characteristic,” a system for reasoning through a language of symbols and calculations—anticipating Lean and Harmonic by 350 years!
00:00 - Introduction
01:42 - Math is reasoning
06:16 - Studying with the world's greatest living mathematician
10:18 - What does the math community think of AI math?
15:11 - Recursive self-improvement
18:31 - What is Lean?
21:05 - Why now?
22:46 - Synthetic data is the fuel for the model
27:29 - How fast will your model get better?
29:45 - Exploring the frontiers of human knowledge
34:11 - Lightning round
AI researcher Jim Fan has had a charmed career. He was OpenAI’s first intern before he did his PhD at Stanford with “godmother of AI,” Fei-Fei Li. He graduated into a research scientist position at Nvidia and now leads its Embodied AI “GEAR” group. The lab’s current work spans foundation models for humanoid robots to agents for virtual worlds.
Jim describes a three-pronged data strategy for robotics, combining internet-scale data, simulation data and real world robot data. He believes that in the next few years it will be possible to create a “foundation agent” that can generalize across skills, embodiments and realities—both physical and virtual. He also supports Jensen Huang’s idea that “Everything that moves will eventually be autonomous.”
Hosted by: Stephanie Zhan and Sonya Huang, Sequoia Capital
Mentioned in this episode:
World of Bits: Early OpenAI project Jim worked on as an intern with Andrej Karpathy. Part of a bigger initiative called Universe
Fei-Fei Li: Jim’s PhD advisor at Stanford who founded the ImageNet project in 2010 that revolutionized the field of visual recognition, led the Stanford Vision Lab and just launched her own AI startup, World Labs
Project GR00T: Nvidia’s “moonshot effort” at a robotic foundation model, premiered at this year’s GTC
Thinking Fast and Slow: Influential book by Daniel Kahneman that popularized some of his teaching from behavioral economics
Jetson Orin chip: The dedicated series of edge computing chips Nvidia is developing to power Project GR00T
Eureka: Project by Jim’s team that trained a five finger robot hand to do pen spinning
MineDojo: A project Jim did when he first got to Nvidia that developed a platform for general purpose agents in the game of Minecraft. Won NeurIPS 2022 Outstanding Paper Award
ADI: artificial dog intelligence
Mamba: Selective State Space Models, an alternative architecture to Transformers that Jim is interested in (original paper here)
00:00 Introduction
01:35 Jim’s journey to embodied intelligence
04:53 The GEAR Group
07:32 Three kinds of data for robotics
10:32 A GPT-3 moment for robotics
16:05 Choosing the humanoid robot form factor
19:37 Specialized generalists
21:59 GR00T gets its own chip
23:35 Eureka and Issac Sim
25:23 Why now for robotics?
28:53 Exploring virtual worlds
36:28 Implications for games
39:13 Is the virtual world in service of the physical world?
42:10 Alternative architectures to Transformers
44:15 Lightning round
Comments
Top Podcasts
The Best New Comedy Podcast Right Now – June 2024The Best News Podcast Right Now – June 2024The Best New Business Podcast Right Now – June 2024The Best New Sports Podcast Right Now – June 2024The Best New True Crime Podcast Right Now – June 2024The Best New Joe Rogan Experience Podcast Right Now – June 20The Best New Dan Bongino Show Podcast Right Now – June 20The Best New Mark Levin Podcast – June 2024