Discover
Vanishing Gradients
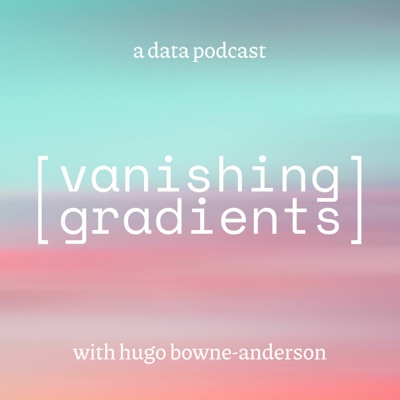
Vanishing Gradients
Author: Hugo Bowne-Anderson
Subscribed: 61Played: 813Subscribe
Share
© 2024 Hugo Bowne-Anderson
Description
A podcast about all things data, brought to you by data scientist Hugo Bowne-Anderson.
It's time for more critical conversations about the challenges in our industry in order to build better compasses for the solution space! To this end, this podcast will consist of long-format conversations between Hugo and other people who work broadly in the data science, machine learning, and AI spaces. We'll dive deep into all the moving parts of the data world, so if you're new to the space, you'll have an opportunity to learn from the experts. And if you've been around for a while, you'll find out what's happening in many other parts of the data world.
It's time for more critical conversations about the challenges in our industry in order to build better compasses for the solution space! To this end, this podcast will consist of long-format conversations between Hugo and other people who work broadly in the data science, machine learning, and AI spaces. We'll dive deep into all the moving parts of the data world, so if you're new to the space, you'll have an opportunity to learn from the experts. And if you've been around for a while, you'll find out what's happening in many other parts of the data world.
39 Episodes
Reverse
Hugo speaks with Ravin Kumar, Senior Research Data Scientist at Google Labs. Ravin’s career has taken him from building rockets at SpaceX to driving data science and technology at Sweetgreen, and now to advancing generative AI research and applications at Google Labs and DeepMind. His multidisciplinary experience gives him a rare perspective on building AI systems that combine technical rigor with practical utility.
In this episode, we dive into:
• Ravin’s fascinating career path, including the skills and mindsets needed to work effectively with AI and machine learning models at different stages of the pipeline.
• How to build generative AI systems that are scalable, reliable, and aligned with user needs.
• Real-world applications of generative AI, such as using open weight models such as Gemma to help a bakery streamline operations—an example of delivering tangible business value through AI.
• The critical role of UX in AI adoption, and how Ravin approaches designing tools like Notebook LM with the user journey in mind.
We also include a live demo where Ravin uses Notebook LM to analyze my website, extract insights, and even generate a podcast-style conversation about me. While some of the demo is visual, much can be appreciated through audio, and we’ve added a link to the video in the show notes for those who want to see it in action. We’ve also included the generated segment at the end of the episode for you to enjoy.
LINKS
The livestream on YouTube (https://www.youtube.com/live/ffS6NWqoo_k)
Google Labs (https://labs.google/)
Ravin's GenAI Handbook (https://ravinkumar.com/GenAiGuidebook/book_intro.html)
Breadboard: A library for prototyping generative AI applications (https://breadboard-ai.github.io/breadboard/)
As mentioned in the episode, Hugo is teaching a four-week course, Building LLM Applications for Data Scientists and SWEs, co-led with Stefan Krawczyk (Dagworks, ex-StitchFix). The course focuses on building scalable, production-grade generative AI systems, with hands-on sessions, $1,000+ in cloud credits, live Q&As, and guest lectures from industry experts.
Listeners of Vanishing Gradients can get 25% off the course using this special link (https://maven.com/hugo-stefan/building-llm-apps-ds-and-swe-from-first-principles?promoCode=VG25) or by applying the code VG25 at checkout.
Hugo speaks with Jason Liu, an independent AI consultant with experience at Meta and Stitch Fix. At Stitch Fix, Jason developed impactful AI systems, like a $50 million product similarity search and the widely adopted Flight recommendation framework. Now, he helps startups and enterprises design and deploy production-level AI applications, with a focus on retrieval-augmented generation (RAG) and scalable solutions.
This episode is a bit of an experiment. Instead of our usual technical deep dives, we’re focusing on the world of AI consulting and freelancing. We explore Jason’s consulting playbook, covering how he structures contracts to maximize value, strategies for moving from hourly billing to securing larger deals, and the mindset shift needed to align incentives with clients. We’ll also discuss the challenges of moving from deterministic software to probabilistic AI systems and even do a live role-playing session where Jason coaches me on client engagement and pricing pitfalls.
LINKS
The livestream on YouTube (https://youtube.com/live/9CFs06UDbGI?feature=share)
Jason's Upcoming course: AI Consultant Accelerator: From Expert to High-Demand Business (https://maven.com/indie-consulting/ai-consultant-accelerator?utm_campaign=9532cc&utm_medium=partner&utm_source=instructor)
Hugo's upcoming course: Building LLM Applications for Data Scientists and Software Engineers (https://maven.com/s/course/d56067f338)
Jason's website (https://jxnl.co/)
Jason's indie consulting newsletter (https://indieconsulting.podia.com/)
Your AI Product Needs Evals by Hamel Husain (https://hamel.dev/blog/posts/evals/)
What We’ve Learned From A Year of Building with LLMs (https://applied-llms.org/)
Dear Future AI Consultant by Jason (https://jxnl.co/writing/#dear-future-ai-consultant)
Alex Hormozi's books (https://www.acquisition.com/books)
The Burnout Society by Byung-Chul Han (https://www.sup.org/books/theory-and-philosophy/burnout-society)
Jason on Twitter (https://x.com/jxnlco)
Vanishing Gradients on Twitter (https://twitter.com/vanishingdata)
Hugo on Twitter (https://twitter.com/hugobowne)
Vanishing Gradients' lu.ma calendar (https://lu.ma/calendar/cal-8ImWFDQ3IEIxNWk)
Vanishing Gradients on YouTube (https://www.youtube.com/@vanishinggradients)
Hugo speaks with three leading figures from the world of AI research: Sander Schulhoff, a recent University of Maryland graduate and lead contributor to the Learn Prompting initiative; Philip Resnik, professor at the University of Maryland, known for his pioneering work in computational linguistics; and Dennis Peskoff, a researcher from Princeton specializing in prompt engineering and its applications in the social sciences.
This is Part 2 of a special two-part episode, prompted—no pun intended—by these guys being part of a team, led by Sander, that wrote a 76-page survey analyzing prompting techniques, agents, and generative AI. The survey included contributors from OpenAI, Microsoft, the University of Maryland, Princeton, and more.
In this episode, we cover:
The Prompt Report: A comprehensive survey on prompting techniques, agents, and generative AI, including advanced evaluation methods for assessing these techniques.
Security Risks and Prompt Hacking: A detailed exploration of the security concerns surrounding prompt engineering, including Sander’s thoughts on its potential applications in cybersecurity and military contexts.
AI’s Impact Across Fields: A discussion on how generative AI is reshaping various domains, including the social sciences and security.
Multimodal AI: Updates on how large language models (LLMs) are expanding to interact with images, code, and music.
Case Study - Detecting Suicide Risk: A careful examination of how prompting techniques are being used in important areas like detecting suicide risk, showcasing the critical potential of AI in addressing sensitive, real-world challenges.
The episode concludes with a reflection on the evolving landscape of LLMs and multimodal AI, and what might be on the horizon.
If you haven’t yet, make sure to check out Part 1, where we discuss the history of NLP, prompt engineering techniques, and Sander’s development of the Learn Prompting initiative.
LINKS
The livestream on YouTube (https://youtube.com/live/FreXovgG-9A?feature=share)
The Prompt Report: A Systematic Survey of Prompting Techniques (https://arxiv.org/abs/2406.06608)
Learn Prompting: Your Guide to Communicating with AI (https://learnprompting.org/)
Vanishing Gradients on Twitter (https://twitter.com/vanishingdata)
Hugo on Twitter (https://twitter.com/hugobowne)
Vanishing Gradients' lu.ma calendar (https://lu.ma/calendar/cal-8ImWFDQ3IEIxNWk)
Vanishing Gradients on YouTube (https://www.youtube.com/@vanishinggradients)
Hugo speaks with three leading figures from the world of AI research: Sander Schulhoff, a recent University of Maryland graduate and lead contributor to the Learn Prompting initiative; Philip Resnik, professor at the University of Maryland, known for his pioneering work in computational linguistics; and Dennis Peskoff, a researcher from Princeton specializing in prompt engineering and its applications in the social sciences.
This is Part 1 of a special two-part episode, prompted—no pun intended—by these guys being part of a team, led by Sander, that wrote a 76-page survey analyzing prompting techniques, agents, and generative AI. The survey included contributors from OpenAI, Microsoft, the University of Maryland, Princeton, and more.
In this first part,
* we’ll explore the critical role of prompt engineering,
* & diving into adversarial techniques like prompt hacking and
* the challenges of evaluating these techniques.
* we’ll examine the impact of few-shot learning and
* the groundbreaking taxonomy of prompting techniques from the Prompt Report.
Along the way,
* we’ll uncover the rich history of natural language processing (NLP) and AI, showing how modern prompting techniques evolved from early rule-based systems and statistical methods.
* we’ll also hear how Sander’s experimentation with GPT-3 for diplomatic tasks led him to develop Learn Prompting, and
* how Dennis highlights the accessibility of AI through prompting, which allows non-technical users to interact with AI without needing to code.
Finally, we’ll explore the future of multimodal AI, where LLMs interact with images, code, and even music creation. Make sure to tune in to Part 2, where we dive deeper into security risks, prompt hacking, and more.
LINKS
The livestream on YouTube (https://youtube.com/live/FreXovgG-9A?feature=share)
The Prompt Report: A Systematic Survey of Prompting Techniques (https://arxiv.org/abs/2406.06608)
Learn Prompting: Your Guide to Communicating with AI (https://learnprompting.org/)
Vanishing Gradients on Twitter (https://twitter.com/vanishingdata)
Hugo on Twitter (https://twitter.com/hugobowne)
Vanishing Gradients' lu.ma calendar (https://lu.ma/calendar/cal-8ImWFDQ3IEIxNWk)
Vanishing Gradients on YouTube (https://www.youtube.com/@vanishinggradients)
Hugo speaks with Dr. Chelle Gentemann, Open Science Program Scientist for NASA’s Office of the Chief Science Data Officer, about NASA’s ambitious efforts to integrate AI across the research lifecycle. In this episode, we’ll dive deeper into how AI is transforming NASA’s approach to science, making data more accessible and advancing open science practices. We explore
Measuring the Impact of Open Science: How NASA is developing new metrics to evaluate the effectiveness of open science, moving beyond traditional publication-based assessments.
The Process of Scientific Discovery: Insights into the collaborative nature of research and how breakthroughs are achieved at NASA.
** AI Applications in NASA’s Science:** From rats in space to exploring the origins of the universe, we cover how AI is being applied across NASA’s divisions to improve data accessibility and analysis.
Addressing Challenges in Open Science: The complexities of implementing open science within government agencies and research environments.
Reforming Incentive Systems: How NASA is reconsidering traditional metrics like publications and citations, and starting to recognize contributions such as software development and data sharing.
The Future of Open Science: How open science is shaping the future of research, fostering interdisciplinary collaboration, and increasing accessibility.
This conversation offers valuable insights for researchers, data scientists, and those interested in the practical applications of AI and open science. Join us as we discuss how NASA is working to make science more collaborative, reproducible, and impactful.
LINKS
The livestream on YouTube (https://youtube.com/live/VJDg3ZbkNOE?feature=share)
NASA's Open Science 101 course <-- do it to learn and also to get NASA Swag! (https://openscience101.org/)
Science Cast (https://sciencecast.org/)
NASA and IBM Openly Release Geospatial AI Foundation Model for NASA Earth Observation Data (https://www.earthdata.nasa.gov/news/impact-ibm-hls-foundation-model)
Jake VanderPlas' daily conundrum tweet from 2013 (https://x.com/jakevdp/status/408678764705378304)
Replit, "an AI-powered software development & deployment platform for building, sharing, and shipping software fast." (https://replit.com/)
Hugo speaks with Ines Montani and Matthew Honnibal, the creators of spaCy and founders of Explosion AI. Collectively, they've had a huge impact on the fields of industrial natural language processing (NLP), ML, and AI through their widely-used open-source library spaCy and their innovative annotation tool Prodigy. These tools have become essential for many data scientists and NLP practitioners in industry and academia alike.
In this wide-ranging discussion, we dive into:
• The evolution of applied NLP and its role in industry
• The balance between large language models and smaller, specialized models
• Human-in-the-loop distillation for creating faster, more data-private AI systems
• The challenges and opportunities in NLP, including modularity, transparency, and privacy
• The future of AI and software development
• The potential impact of AI regulation on innovation and competition
We also touch on their recent transition back to a smaller, more independent-minded company structure and the lessons learned from their journey in the AI startup world.
Ines and Matt offer invaluable insights for data scientists, machine learning practitioners, and anyone interested in the practical applications of AI. They share their thoughts on how to approach NLP projects, the importance of data quality, and the role of open-source in advancing the field.
Whether you're a seasoned NLP practitioner or just getting started with AI, this episode offers a wealth of knowledge from two of the field's most respected figures. Join us for a discussion that explores the current landscape of AI development, with insights that bridge the gap between cutting-edge research and real-world applications.
LINKS
The livestream on YouTube (https://youtube.com/live/-6o5-3cP0ik?feature=share)
How S&P Global is making markets more transparent with NLP, spaCy and Prodigy (https://explosion.ai/blog/sp-global-commodities)
A practical guide to human-in-the-loop distillation (https://explosion.ai/blog/human-in-the-loop-distillation)
Laws of Tech: Commoditize Your Complement (https://gwern.net/complement)
spaCy: Industrial-Strength Natural Language Processing (https://spacy.io/)
LLMs with spaCy (https://spacy.io/usage/large-language-models)
Explosion, building developer tools for AI, Machine Learning and Natural Language Processing (https://explosion.ai/)
Back to our roots: Company update and future plans, by Matt and Ines (https://explosion.ai/blog/back-to-our-roots-company-update)
Matt's detailed blog post: back to our roots (https://honnibal.dev/blog/back-to-our-roots)
Ines on twitter (https://x.com/_inesmontani)
Matt on twitter (https://x.com/honnibal)
Vanishing Gradients on Twitter (https://twitter.com/vanishingdata)
Hugo on Twitter (https://twitter.com/hugobowne)
Check out and subcribe to our lu.ma calendar (https://lu.ma/calendar/cal-8ImWFDQ3IEIxNWk) for upcoming livestreams!
Hugo speaks with Dan Becker and Hamel Husain, two veterans in the world of data science, machine learning, and AI education. Collectively, they’ve worked at Google, DataRobot, Airbnb, Github (where Hamel built out the precursor to copilot and more) and they both currently work as independent LLM and Generative AI consultants.
Dan and Hamel recently taught a course on fine-tuning large language models that evolved into a full-fledged conference, attracting over 2,000 participants. This experience gave them unique insights into the current state and future of AI education and application.
In this episode, we dive into:
* The evolution of their course from fine-tuning to a comprehensive AI conference
* The unexpected challenges and insights gained from teaching LLMs to data scientists
* The current state of AI tooling and accessibility compared to a decade ago
* The role of playful experimentation in driving innovation in the field
* Thoughts on the economic impact and ROI of generative AI in various industries
* The importance of proper evaluation in machine learning projects
* Future predictions for AI education and application in the next five years
* We also touch on the challenges of using AI tools effectively, the potential for AI in physical world applications, and the need for a more nuanced understanding of AI capabilities in the workplace.
During our conversation, Dan mentions an exciting project he's been working on, which we couldn't showcase live due to technical difficulties. However, I've included a link to a video demonstration in the show notes that you won't want to miss. In this demo, Dan showcases his innovative AI-powered 3D modeling tool that allows users to create 3D printable objects simply by describing them in natural language.
LINKS
The livestream on YouTube (https://youtube.com/live/hDmnwtjktsc?feature=share)
Educational resources from Dan and Hamel's LLM course (https://parlance-labs.com/education/)
Upwork Study Finds Employee Workloads Rising Despite Increased C-Suite Investment in Artificial Intelligence (https://investors.upwork.com/news-releases/news-release-details/upwork-study-finds-employee-workloads-rising-despite-increased-c)
Episode 29: Lessons from a Year of Building with LLMs (Part 1) (https://vanishinggradients.fireside.fm/29)
Episode 30: Lessons from a Year of Building with LLMs (Part 2) (https://vanishinggradients.fireside.fm/30)
Dan's demo: Creating Physical Products with Generative AI (https://youtu.be/U5J5RUOuMkI?si=_7cYLYOU1iwweQeO)
Build Great AI, Dan's boutique consulting firm helping clients be successful with large language models (https://buildgreat.ai/)
Parlance Labs, Hamel's Practical consulting that improves your AI (https://parlance-labs.com/)
Hamel on Twitter (https://x.com/HamelHusain)
Dan on Twitter (https://x.com/dan_s_becker)
Vanishing Gradients on Twitter (https://twitter.com/vanishingdata)
Hugo on Twitter (https://twitter.com/hugobowne)
Hugo speaks with Shreya Shankar, a researcher at UC Berkeley focusing on data management systems with a human-centered approach. Shreya's work is at the cutting edge of human-computer interaction (HCI) and AI, particularly in the realm of large language models (LLMs). Her impressive background includes being the first ML engineer at Viaduct, doing research engineering at Google Brain, and software engineering at Facebook.
In this episode, we dive deep into the world of LLMs and the critical challenges of building reliable AI pipelines. We'll explore:
The fascinating journey from classic machine learning to the current LLM revolution
Why Shreya believes most ML problems are actually data management issues
The concept of "data flywheels" for LLM applications and how to implement them
The intriguing world of evaluating AI systems - who validates the validators?
Shreya's work on SPADE and EvalGen, innovative tools for synthesizing data quality assertions and aligning LLM evaluations with human preferences
The importance of human-in-the-loop processes in AI development
The future of low-code and no-code tools in the AI landscape
We'll also touch on the potential pitfalls of over-relying on LLMs, the concept of "Habsburg AI," and how to avoid disappearing up our own proverbial arseholes in the world of recursive AI processes.
Whether you're a seasoned AI practitioner, a curious data scientist, or someone interested in the human side of AI development, this conversation offers valuable insights into building more robust, reliable, and human-centered AI systems.
LINKS
The livestream on YouTube (https://youtube.com/live/hKV6xSJZkB0?feature=share)
Shreya's website (https://www.sh-reya.com/)
Shreya on Twitter (https://x.com/sh_reya)
Data Flywheels for LLM Applications (https://www.sh-reya.com/blog/ai-engineering-flywheel/)
SPADE: Synthesizing Data Quality Assertions for Large Language Model Pipelines (https://arxiv.org/abs/2401.03038)
What We’ve Learned From A Year of Building with LLMs (https://applied-llms.org/)
Who Validates the Validators? Aligning LLM-Assisted Evaluation of LLM Outputs with Human Preferences (https://arxiv.org/abs/2404.12272)
Operationalizing Machine Learning: An Interview Study (https://arxiv.org/abs/2209.09125)
Vanishing Gradients on Twitter (https://twitter.com/vanishingdata)
Hugo on Twitter (https://twitter.com/hugobowne)
In the podcast, Hugo also mentioned that this was the 5th time he and Shreya chatted publicly. which is wild!
If you want to dive deep into Shreya's work and related topics through their chats, you can check them all out here:
Outerbounds' Fireside Chat: Operationalizing ML -- Patterns and Pain Points from MLOps Practitioners (https://www.youtube.com/watch?v=7zB6ESFto_U)
The Past, Present, and Future of Generative AI (https://youtu.be/q0A9CdGWXqc?si=XmaUnQmZiXL2eagS)
LLMs, OpenAI Dev Day, and the Existential Crisis for Machine Learning Engineering (https://www.youtube.com/live/MTJHvgJtynU?si=Ncjqn5YuFBemvOJ0)
Lessons from a Year of Building with LLMs (https://youtube.com/live/c0gcsprsFig?feature=share)
Check out and subcribe to our lu.ma calendar (https://lu.ma/calendar/cal-8ImWFDQ3IEIxNWk) for upcoming livestreams!
Hugo speaks with Vincent Warmerdam, a senior data professional and machine learning engineer at :probabl, the exclusive brand operator of scikit-learn. Vincent is known for challenging common assumptions and exploring innovative approaches in data science and machine learning.
In this episode, they dive deep into rethinking established methods in data science, machine learning, and AI. We explore Vincent's principled approach to the field, including:
The critical importance of exposing yourself to real-world problems before applying ML solutions
Framing problems correctly and understanding the data generating process
The power of visualization and human intuition in data analysis
Questioning whether algorithms truly meet the actual problem at hand
The value of simple, interpretable models and when to consider more complex approaches
The importance of UI and user experience in data science tools
Strategies for preventing algorithmic failures by rethinking evaluation metrics and data quality
The potential and limitations of LLMs in the current data science landscape
The benefits of open-source collaboration and knowledge sharing in the community
Throughout the conversation, Vincent illustrates these principles with vivid, real-world examples from his extensive experience in the field. They also discuss Vincent's thoughts on the future of data science and his call to action for more knowledge sharing in the community through blogging and open dialogue.
LINKS
The livestream on YouTube (https://youtube.com/live/-CD66CI1pEo?feature=share)
Vincent's blog (https://koaning.io/)
CalmCode (https://calmcode.io/)
scikit-lego (https://koaning.github.io/scikit-lego/)
Vincent's book Data Science Fiction (WIP) (https://calmcode.io/book)
The Deon Checklist, an ethics checklist for data scientists (https://deon.drivendata.org/)
Of oaths and checklists, by DJ Patil, Hilary Mason and Mike Loukides (https://www.oreilly.com/radar/of-oaths-and-checklists/)
Vincent's Getting Started with NLP and spaCy Course course on Talk Python (https://training.talkpython.fm/courses/getting-started-with-spacy)
Vincent on twitter (https://x.com/fishnets88)
:probabl. on twitter (https://x.com/probabl_ai)
Vincent's PyData Amsterdam Keynote "Natural Intelligence is All You Need [tm]" (https://www.youtube.com/watch?v=C9p7suS-NGk)
Vincent's PyData Amsterdam 2019 talk: The profession of solving (the wrong problem) (https://www.youtube.com/watch?v=kYMfE9u-lMo)
Vanishing Gradients on Twitter (https://twitter.com/vanishingdata)
Hugo on Twitter (https://twitter.com/hugobowne)
Check out and subcribe to our lu.ma calendar (https://lu.ma/calendar/cal-8ImWFDQ3IEIxNWk) for upcoming livestreams!
Hugo speaks about Lessons Learned from a Year of Building with LLMs with Eugene Yan from Amazon, Bryan Bischof from Hex, Charles Frye from Modal, Hamel Husain from Parlance Labs, and Shreya Shankar from UC Berkeley.
These five guests, along with Jason Liu who couldn't join us, have spent the past year building real-world applications with Large Language Models (LLMs). They've distilled their experiences into a report of 42 lessons across operational, strategic, and tactical dimensions (https://applied-llms.org/), and they're here to share their insights.
We’ve split this roundtable into 2 episodes and, in this second episode, we'll explore:
An inside look at building end-to-end systems with LLMs;
The experimentation mindset: Why it's the key to successful AI products;
Building trust in AI: Strategies for getting stakeholders on board;
The art of data examination: Why looking at your data is more crucial than ever;
Evaluation strategies that separate the pros from the amateurs.
Although we're focusing on LLMs, many of these insights apply broadly to data science, machine learning, and product development, more generally.
LINKS
The livestream on YouTube (https://www.youtube.com/live/c0gcsprsFig)
The Report: What We’ve Learned From A Year of Building with LLMs (https://applied-llms.org/)
About the Guests/Authors (https://applied-llms.org/about.html) <-- connect with them all on LinkedIn, follow them on Twitter, subscribe to their newsletters! (Seriously, though, the amount of collective wisdom here is 🤑
Your AI product needs evals by Hamel Husain (https://hamel.dev/blog/posts/evals/)
Prompting Fundamentals and How to Apply them Effectively by Eugene Yan (https://eugeneyan.com/writing/prompting/)
Fuck You, Show Me The Prompt by Hamel Husain (https://hamel.dev/blog/posts/prompt/)
Vanishing Gradients on YouTube (https://www.youtube.com/channel/UC_NafIo-Ku2loOLrzm45ABA)
Vanishing Gradients on Twitter (https://x.com/vanishingdata)
Vanishing Gradients on Lu.ma (https://lu.ma/calendar/cal-8ImWFDQ3IEIxNWk)
Hugo speaks about Lessons Learned from a Year of Building with LLMs with Eugene Yan from Amazon, Bryan Bischof from Hex, Charles Frye from Modal, Hamel Husain from Parlance Labs, and Shreya Shankar from UC Berkeley.
These five guests, along with Jason Liu who couldn't join us, have spent the past year building real-world applications with Large Language Models (LLMs). They've distilled their experiences into a report of 42 lessons across operational, strategic, and tactical dimensions (https://applied-llms.org/), and they're here to share their insights.
We’ve split this roundtable into 2 episodes and, in this first episode, we'll explore:
The critical role of evaluation and monitoring in LLM applications and why they're non-negotiable, including "evals" - short for evaluations, which are automated tests for assessing LLM performance and output quality;
Why data literacy is your secret weapon in the AI landscape;
The fine-tuning dilemma: when to do it and when to skip it;
Real-world lessons from building LLM applications that textbooks won't teach you;
The evolving role of data scientists and AI engineers in the age of AI.
Although we're focusing on LLMs, many of these insights apply broadly to data science, machine learning, and product development, more generally.
LINKS
The livestream on YouTube (https://www.youtube.com/live/c0gcsprsFig)
The Report: What We’ve Learned From A Year of Building with LLMs (https://applied-llms.org/)
About the Guests/Authors (https://applied-llms.org/about.html) <-- connect with them all on LinkedIn, follow them on Twitter, subscribe to their newsletters! (Seriously, though, the amount of collective wisdom here is 🤑
Your AI product needs evals by Hamel Husain (https://hamel.dev/blog/posts/evals/)
Prompting Fundamentals and How to Apply them Effectively by Eugene Yan (https://eugeneyan.com/writing/prompting/)
Fuck You, Show Me The Prompt by Hamel Husain (https://hamel.dev/blog/posts/prompt/)
Vanishing Gradients on YouTube (https://www.youtube.com/channel/UC_NafIo-Ku2loOLrzm45ABA)
Vanishing Gradients on Twitter (https://x.com/vanishingdata)
Vanishing Gradients on Lu.ma (https://lu.ma/calendar/cal-8ImWFDQ3IEIxNWk)
Hugo speaks with Alan Nichol, co-founder and CTO of Rasa, where they build software to enable developers to create enterprise-grade conversational AI and chatbot systems across industries like telcos, healthcare, fintech, and government.
What's super cool is that Alan and the Rasa team have been doing this type of thing for over a decade, giving them a wealth of wisdom on how to effectively incorporate LLMs into chatbots - and how not to. For example, if you want a chatbot that takes specific and important actions like transferring money, do you want to fully entrust the conversation to one big LLM like ChatGPT, or secure what the LLMs can do inside key business logic?
In this episode, they also dive into the history of conversational AI and explore how the advent of LLMs is reshaping the field. Alan shares his perspective on how supervised learning has failed us in some ways and discusses what he sees as the most overrated and underrated aspects of LLMs.
Alan offers advice for those looking to work with LLMs and conversational AI, emphasizing the importance of not sleeping on proven techniques and looking beyond the latest hype. In a live demo, he showcases Rasa's Calm (Conversational AI with Language Models), which allows developers to define business logic declaratively and separate it from the LLM, enabling reliable execution of conversational flows.
LINKS
The livestream on YouTube (https://www.youtube.com/live/kMFBYC2pB30?si=yV5sGq1iuC47LBSi)
Alan's Rasa CALM Demo: Building Conversational AI with LLMs (https://youtu.be/4UnxaJ-GcT0?si=6uLY3GD5DkOmWiBW)
Alan on twitter.com (https://x.com/alanmnichol)
Rasa (https://rasa.com/)
CALM, an LLM-native approach to building reliable conversational AI (https://rasa.com/docs/rasa-pro/calm/)
Task-Oriented Dialogue with In-Context Learning (https://arxiv.org/abs/2402.12234)
'We don’t know how to build conversational software yet' by Alan Nicol (https://medium.com/rasa-blog/we-don-t-know-how-to-build-conversational-software-yet-a18301db0e4b)
Vanishing Gradients on Twitter (https://twitter.com/vanishingdata)
Hugo on Twitter (https://twitter.com/hugobowne)
Upcoming Livestreams
Lessons from a Year of Building with LLMs (https://lu.ma/e8huz3s6?utm_source=vgan)
VALIDATING THE VALIDATORS with Shreya Shanker (https://lu.ma/zz3qic45?utm_source=vgan)
Hugo speaks with Jason Liu, an independent consultant who uses his expertise in recommendation systems to help fast-growing startups build out their RAG applications. He was previously at Meta and Stitch Fix is also the creator of Instructor, Flight, and an ML and data science educator.
They talk about how Jason approaches consulting companies across many industries, including construction and sales, in building production LLM apps, his playbook for getting ML and AI up and running to build and maintain such apps, and the future of tooling to do so.
They take an inverted thinking approach, envisaging all the failure modes that would result in building terrible AI systems, and then figure out how to avoid such pitfalls.
LINKS
The livestream on YouTube (https://youtube.com/live/USTG6sQlB6s?feature=share)
Jason's website (https://jxnl.co/)
PyDdantic is all you need, Jason's Keynote at AI Engineer Summit, 2023 (https://youtu.be/yj-wSRJwrrc?si=JIGhN0mx0i50dUR9)
How to build a terrible RAG system by Jason (https://jxnl.co/writing/2024/01/07/inverted-thinking-rag/)
To express interest in Jason's Systematically improving RAG Applications course (https://q7gjsgfstrp.typeform.com/ragcourse?typeform-source=vg)
Vanishing Gradients on Twitter (https://twitter.com/vanishingdata)
Hugo on Twitter (https://twitter.com/hugobowne)
Upcoming Livestreams
Good Riddance to Supervised Learning with Alan Nichol (CTO and co-founder, Rasa) (https://lu.ma/gphzzyyn?utm_source=vgj)
Lessons from a Year of Building with LLMs (https://lu.ma/e8huz3s6?utm_source=vgj)
Hugo speaks with Sebastian Raschka, a machine learning & AI researcher, programmer, and author. As Staff Research Engineer at Lightning AI, he focuses on the intersection of AI research, software development, and large language models (LLMs).
How do you build LLMs? How can you use them, both in prototype and production settings? What are the building blocks you need to know about?
In this episode, we’ll tell you everything you need to know about LLMs, but were too afraid to ask: from covering the entire LLM lifecycle, what type of skills you need to work with them, what type of resources and hardware, prompt engineering vs fine-tuning vs RAG, how to build an LLM from scratch, and much more.
The idea here is not that you’ll need to use an LLM you’ve built from scratch, but that we’ll learn a lot about LLMs and how to use them in the process.
Near the end we also did some live coding to fine-tune GPT-2 in order to create a spam classifier!
LINKS
The livestream on YouTube (https://youtube.com/live/qL4JY6Y5pmA)
Sebastian's website (https://sebastianraschka.com/)
Machine Learning Q and AI: 30 Essential Questions and Answers on Machine Learning and AI by Sebastian (https://nostarch.com/machine-learning-q-and-ai)
Build a Large Language Model (From Scratch) by Sebastian (https://www.manning.com/books/build-a-large-language-model-from-scratch)
PyTorch Lightning (https://lightning.ai/docs/pytorch/stable/)
Lightning Fabric (https://lightning.ai/docs/fabric/stable/)
LitGPT (https://github.com/Lightning-AI/litgpt)
Sebastian's notebook for finetuning GPT-2 for spam classification! (https://github.com/rasbt/LLMs-from-scratch/blob/main/ch06/01_main-chapter-code/ch06.ipynb)
The end of fine-tuning: Jeremy Howard on the Latent Space Podcast (https://www.latent.space/p/fastai)
Our next livestream: How to Build Terrible AI Systems with Jason Liu (https://lu.ma/terrible-ai-systems?utm_source=vg)
Vanishing Gradients on Twitter (https://twitter.com/vanishingdata)
Hugo on Twitter (https://twitter.com/hugobowne)
Hugo speaks with Omoju Miller, a machine learning guru and founder and CEO of Fimio, where she is building 21st century dev tooling. In the past, she was Technical Advisor to the CEO at GitHub, spent time co-leading non-profit investment in Computer Science Education for Google, and served as a volunteer advisor to the Obama administration’s White House Presidential Innovation Fellows.
We need open tools, open data, provenance, and the ability to build fully reproducible, transparent machine learning workflows. With the advent of closed-source, vendor-based APIs and compute becoming a form of gate-keeping, developer tools are at the risk of becoming commoditized and developers becoming consumers.
We’ll talk about how ideas for escaping these burgeoning walled gardens. We’ll dive into
What fully reproducible ML workflows would look like, including git for the workflow build process,
The need for loosely coupled and composable tools that embrace a UNIX-like philosophy,
What a much more scientific toolchain would look like,
What a future open sources commons for Generative AI could look like,
What an open compute ecosystem could look like,
How to create LLMs and tooling so everyone can use them to build production-ready apps,
And much more!
LINKS
The livestream on YouTube (https://www.youtube.com/live/n81PWNsHSMk?si=pgX2hH5xADATdJMu)
Omoju on Twitter (https://twitter.com/omojumiller)
Hugo on Twitter (https://twitter.com/hugobowne)
Vanishing Gradients on Twitter (https://twitter.com/vanishingdata)
Lu.ma Calendar that includes details of Hugo's European Tour for Outerbounds (https://lu.ma/Outerbounds)
Blog post that includes details of Hugo's European Tour for Outerbounds (https://outerbounds.com/blog/ob-on-the-road-2024-h1/)
Hugo speaks with Johno Whitaker, a Data Scientist/AI Researcher doing R&D with answer.ai. His current focus is on generative AI, flitting between different modalities. He also likes teaching and making courses, having worked with both Hugging Face and fast.ai in these capacities.
Johno recently reminded Hugo how hard everything was 10 years ago: “Want to install TensorFlow? Good luck. Need data? Perhaps try ImageNet. But now you can use big models from Hugging Face with hi-res satellite data and do all of this in a Colab notebook. Or think ecology and vision models… or medicine and multimodal models!”
We talk about where we’ve come from regarding tooling and accessibility for foundation models, ML, and AI, where we are, and where we’re going. We’ll delve into
What the Generative AI mindset is, in terms of using atomic building blocks, and how it evolved from both the data science and ML mindsets;
How fast.ai democratized access to deep learning, what successes they had, and what was learned;
The moving parts now required to make GenAI and ML as accessible as possible;
The importance of focusing on UX and the application in the world of generative AI and foundation models;
The skillset and toolkit needed to be an LLM and AI guru;
What they’re up to at answer.ai to democratize LLMs and foundation models.
LINKS
The livestream on YouTube (https://youtube.com/live/hxZX6fBi-W8?feature=share)
Zindi, the largest professional network for data scientists in Africa (https://zindi.africa/)
A new old kind of R&D lab: Announcing Answer.AI (http://www.answer.ai/posts/2023-12-12-launch.html)
Why and how I’m shifting focus to LLMs by Johno Whitaker (https://johnowhitaker.dev/dsc/2023-07-01-why-and-how-im-shifting-focus-to-llms.html)
Applying AI to Immune Cell Networks by Rachel Thomas (https://www.fast.ai/posts/2024-01-23-cytokines/)
Replicate -- a cool place to explore GenAI models, among other things (https://replicate.com/explore)
Hands-On Generative AI with Transformers and Diffusion Models (https://www.oreilly.com/library/view/hands-on-generative-ai/9781098149239/)
Johno on Twitter (https://twitter.com/johnowhitaker)
Hugo on Twitter (https://twitter.com/hugobowne)
Vanishing Gradients on Twitter (https://twitter.com/vanishingdata)
SciPy 2024 CFP (https://www.scipy2024.scipy.org/#CFP)
Escaping Generative AI Walled Gardens with Omoju Miller, a Vanishing Gradients Livestream (https://lu.ma/xonnjqe4)
Hugo speaks with Allen Downey, a curriculum designer at Brilliant, Professor Emeritus at Olin College, and the author of Think Python, Think Bayes, Think Stats, and other computer science and data science books. In 2019-20 he was a Visiting Professor at Harvard University. He previously taught at Wellesley College and Colby College and was a Visiting Scientist at Google. He is also the author of the upcoming book Probably Overthinking It!
They discuss Allen's new book and the key statistical and data skills we all need to navigate an increasingly data-driven and algorithmic world. The goal was to dive deep into the statistical paradoxes and fallacies that get in the way of using data to make informed decisions.
For example, when it was reported in 2021 that “in the United Kingdom, 70-plus percent of the people who die now from COVID are fully vaccinated,” this was correct but the implication was entirely wrong. Their conversation jumps into many such concrete examples to get to the bottom of using data for more than “lies, damned lies, and statistics.” They cover
Information and misinformation around pandemics and the base rate fallacy;
The tools we need to comprehend the small probabilities of high-risk events such as stock market crashes, earthquakes, and more;
The many definitions of algorithmic fairness, why they can't all be met at once, and what we can do about it;
Public health, the need for robust causal inference, and variations on Berkson’s paradox, such as the low-birthweight paradox: an influential paper found that that the mortality rate for children of smokers is lower for low-birthweight babies;
Why none of us are normal in any sense of the word, both in physical and psychological measurements;
The Inspection paradox, which shows up in the criminal justice system and distorts our perception of prison sentences and the risk of repeat offenders.
LINKS
The livestream on YouTube (https://youtube.com/live/G8LulD72kzs?feature=share)
Allen Downey on Github (https://github.com/AllenDowney)
Allen's new book Probably Overthinking It! (https://greenteapress.com/wp/probably-overthinking-it/)
Allen on Twitter (https://twitter.com/AllenDowney)
Prediction-Based Decisions and Fairness: A Catalogue of Choices, Assumptions, and Definitions by Mitchell et al. (https://arxiv.org/abs/1811.07867)
Jeremy Howard (Fast.ai), Shreya Shankar (UC Berkeley), and Hamel Husain (Parlance Labs) join Hugo Bowne-Anderson to talk about how LLMs and OpenAI are changing the worlds of data science, machine learning, and machine learning engineering.
Jeremy Howard (https://twitter.com/jeremyphoward) is co-founder of fast.ai, an ex-Chief Scientist at Kaggle, and creator of the ULMFiT approach on which all modern language models are based. Shreya Shankar (https://twitter.com/sh_reya) is at UC Berkeley, ex Google brain, Facebook, and Viaduct. Hamel Husain (https://twitter.com/HamelHusain) has his own generative AI and LLM consultancy Parlance Labs (https://parlance-labs.com/) and was previously at Outerbounds, Github, and Airbnb.
They talk about
How LLMs shift the nature of the work we do in DS and ML,
How they change the tools we use,
The ways in which they could displace the role of traditional ML (e.g. will we stop using xgboost any time soon?),
How to navigate all the new tools and techniques,
The trade-offs between open and closed models,
Reactions to the recent Open Developer Day and the increasing existential crisis for ML.
LINKS
The panel on YouTube (https://youtube.com/live/MTJHvgJtynU?feature=share)
Hugo and Jeremy's upcoming livestream on what the hell happened recently at OpenAI, among many other things (https://lu.ma/byxyzfrr?utm_source=vg)
Vanishing Gradients on YouTube (https://www.youtube.com/channel/UC_NafIo-Ku2loOLrzm45ABA)
Vanishing Gradients on twitter (https://twitter.com/VanishingData)
Hugo speaks with Hamel Husain, a machine learning engineer who loves building machine learning infrastructure and tools 👷. Hamel leads and contributes to many popular open-source machine learning projects. He also has extensive experience (20+ years) as a machine learning engineer across various industries, including large tech companies like Airbnb and GitHub. At GitHub, he led CodeSearchNet (https://github.com/github/CodeSearchNet), a large language model for semantic search that was a precursor to CoPilot. Hamel is the founder of Parlance-Labs (https://parlance-labs.com/), a research and consultancy focused on LLMs.
They talk about generative AI, large language models, the business value they can generate, and how to get started.
They delve into
Where Hamel is seeing the most business interest in LLMs (spoiler: the answer isn’t only tech);
Common misconceptions about LLMs;
The skills you need to work with LLMs and GenAI models;
Tools and techniques, such as fine-tuning, RAGs, LoRA, hardware, and more!
Vendor APIs vs OSS models.
LINKS
Our upcoming livestream LLMs, OpenAI Dev Day, and the Existential Crisis for Machine Learning Engineering with Jeremy Howard (Fast.ai), Shreya Shankar (UC Berkeley), and Hamel Husain (Parlance Labs): Sign up for free! (https://lu.ma/m81oepqe/utm_source=vghh)
Our recent livestream Data and DevOps Tools for Evaluating and Productionizing LLMs (https://youtube.com/live/B_DMMlDuJB0) with Hamel and Emil Sedgh, Lead AI engineer at Rechat -- in it, we showcase an actual industrial use case that Hamel and Emil are working on with Rechat, a real estate CRM, taking you through LLM workflows and tools.
Extended Guide: Instruction-tune Llama 2 (https://www.philschmid.de/instruction-tune-llama-2) by Philipp Schmid
The livestream recoding of this episode! (https://youtube.com/live/l7jJhL9geZQ?feature=share)
Hamel on twitter (https://twitter.com/HamelHusain)
Hugo speaks with Chris Wiggins (Columbia, NYTimes) and Matthew Jones (Princeton) about their recent book How Data Happened, and the Columbia course it expands upon, data: past, present, and future.
Chris is an associate professor of applied mathematics at Columbia University and the New York Times’ chief data scientist, and Matthew is a professor of history at Princeton University and former Guggenheim Fellow.
From facial recognition to automated decision systems that inform who gets loans and who receives bail, we all now move through a world determined by data-empowered algorithms. These technologies didn’t just appear: they are part of a history that goes back centuries, from the census enshrined in the US Constitution to the birth of eugenics in Victorian Britain to the development of Google search.
DJ Patil, former U.S. Chief Data Scientist, said of the book "This is the first comprehensive look at the history of data and how power has played a critical role in shaping the history. It’s a must read for any data scientist about how we got here and what we need to do to ensure that data works for everyone."
If you’re a data scientist, machine learning engineer, or work with data in any way, it’s increasingly important to know more about the history and future of the work that you do and understand how your work impacts society and the world.
Among other things, they'll delve into
* the history of human use of data;
* how data are used to reveal insight and support decisions;
* how data and data-powered algorithms shape, constrain, and manipulate our commercial, civic, and personal transactions and experiences; and
* how exploration and analysis of data have become part of our logic and rhetoric of communication and persuasion.
You can also sign up for our next livestreamed podcast recording here (https://www.eventbrite.com/e/data-science-past-present-and-future-tickets-695643357007?aff=kjvg)!
LINKS
How Data Happened, the book! (https://wwnorton.com/books/how-data-happened)
data: past, present, and future, the course (https://data-ppf.github.io/)
Race After Technology, by Ruha Benjamin (https://www.ruhabenjamin.com/race-after-technology)
The problem with metrics is a big problem for AI by Rachel Thomas (https://www.ruhabenjamin.com/race-after-technology)
Vanishing Gradients on YouTube (https://www.youtube.com/channel/UC_NafIo-Ku2loOLrzm45ABA)
Comments
Top Podcasts
The Best New Comedy Podcast Right Now – June 2024The Best News Podcast Right Now – June 2024The Best New Business Podcast Right Now – June 2024The Best New Sports Podcast Right Now – June 2024The Best New True Crime Podcast Right Now – June 2024The Best New Joe Rogan Experience Podcast Right Now – June 20The Best New Dan Bongino Show Podcast Right Now – June 20The Best New Mark Levin Podcast – June 2024