Discover
The Nonlinear Library
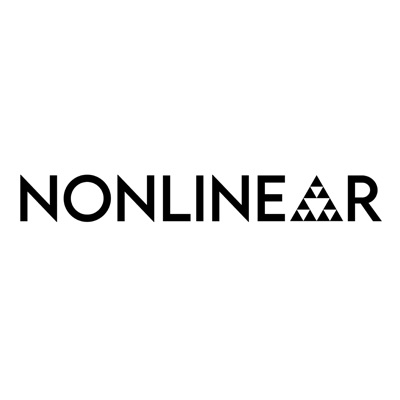
The Nonlinear Library
Author: The Nonlinear Fund
Subscribed: 25Played: 6,489Subscribe
Share
© 2024 The Nonlinear Fund
Description
The Nonlinear Library allows you to easily listen to top EA and rationalist content on your podcast player. We use text-to-speech software to create an automatically updating repository of audio content from the EA Forum, Alignment Forum, LessWrong, and other EA blogs. To find out more, please visit us at nonlinear.org
4973 Episodes
Reverse
Welcome to The Nonlinear Library, where we use Text-to-Speech software to convert the best writing from the Rationalist and EA communities into audio. This is: GiveWell is hiring a Head of Tech and Senior Researchers!, published by GiveWell on April 27, 2024 on The Effective Altruism Forum.
We're hiring for senior research and tech team members! Please apply if you're interested, and share the openings with people in your network who might be a great fit.
The
Head of Technology will take broad ownership of GiveWell's technology function and build a strong tech team. The ideal candidate has built and scaled a tech team at least once, and is excited to leverage their past experience and deep subject matter expertise to help GiveWell grow and excel. This role is remote-eligible within the United States.
Senior Researchers will lead ambitious research agendas, answer complex questions, and inform high-impact grantmaking decisions. Applicants must have a quantitatively oriented advanced degree or an undergraduate degree and substantial relevant experience using empirical tools to make real-world decisions. This role is remote-eligible anywhere in the world.
Both roles have compensation packages that are competitive with our peer organizations.
Thanks for listening. To help us out with The Nonlinear Library or to learn more, please visit nonlinear.org
Welcome to The Nonlinear Library, where we use Text-to-Speech software to convert the best writing from the Rationalist and EA communities into audio. This is: On Not Pulling The Ladder Up Behind You, published by Screwtape on April 27, 2024 on LessWrong.
Epistemic Status: Musing and speculation, but I think there's a real thing here.
I.
When I was a kid, a friend of mine had a tree fort. If you've never seen such a fort, imagine a series of wooden boards secured to a tree, creating a platform about fifteen feet off the ground where you can sit or stand and walk around the tree. This one had a rope ladder we used to get up and down, a length of knotted rope that was tied to the tree at the top and dangled over the edge so that it reached the ground.
Once you were up in the fort, you could pull the ladder up behind you. It was much, much harder to get into the fort without the ladder. Not only would you need to climb the tree itself instead of the ladder with its handholds, but you would then reach the underside of the fort and essentially have to do a pullup and haul your entire body up and over the edge instead of being able to pull yourself up a foot at a time on the rope. Only then could you let the rope back down.
The rope got pulled up a lot, mostly in games or childhood arguments with each other or our siblings. Sometimes it got pulled up out of boredom, fiddling with it or playing with the rope. Sometimes it got pulled up when we were trying to be helpful; it was easier for a younger kid to hold tight to the rope while two older kids pulled the rope up to haul the young kid into the tree fort.
"Pulling the ladder up behind you" is a metaphor for when you intentionally or unintentionally remove the easier way by which you reached some height.
II.
Quoth Ray,
Weird fact: a lot of people I know (myself included) gained a bunch of agency from running meetups.
When I arrived in the NYC community, I noticed an opportunity for some kind of winter holiday. I held the first Solstice. The only stakes were 20 people possibly having a bad time. The next year, I planned a larger event that people traveled from nearby cities to attend, which required me to learn some logistics as well as to improve at ritual design. The third year I was able to run a major event with a couple hundred attendees. At each point I felt challenged but not overwhelmed.
I made mistakes, but not ones that ruined anything longterm or important.
I'm a something of a serial inheritor[1] of meetups.
Last year I ran the Rationalist Megameetup in New York City, which had over a hundred people attending and took place at a conference hotel. It's the most complicated event I've run so far, but it didn't start that way. The first iteration of the megameetup was, as far as I know, inviting people to hang out at a big apartment and letting some of them crash on couches or air mattresses there.
That's pretty straightforward and something I can imagine a first-time organizer pulling off without too much stress. The first time I ran the megameetup, it involved renting an apartment and taking payments and buying a lot of food, but I was basically doing the exact same thing the person before me did and I got to ask a previous organizer a lot of questions.
This means that I got to slowly level up, getting more used to the existing tools and more comfortable in what I was doing as I made things bigger. There was a ladder there to let me climb up. If tomorrow I decided to stop having anything to do with the Rationalist Megameetup, I'd be leaving whoever picked up the torch after me with a harder climb. That problem is only going to get worse as the Rationalist Megameetup grows.
Projects have a tendency to grow more complicated the longer they go and the more successful they get. Meetups get bigger as more people join, codebases get larger as more features get added, companies wind up with a larger product line, fiction series add more characters and plotlines. That makes tak...
Welcome to The Nonlinear Library, where we use Text-to-Speech software to convert the best writing from the Rationalist and EA communities into audio. This is: Superposition is not "just" neuron polysemanticity, published by Lawrence Chan on April 26, 2024 on The AI Alignment Forum.
TL;DR: In this post, I distinguish between two related concepts in neural network interpretability: polysemanticity and superposition. Neuron polysemanticity is the observed phenomena that many neurons seem to fire (have large, positive activations) on multiple unrelated concepts.
Superposition is a specific explanation for neuron (or attention head) polysemanticity, where a neural network represents more sparse features than there are neurons (or number of/dimension of attention heads) in near-orthogonal directions. I provide three ways neurons/attention heads can be polysemantic without superposition: non--neuron aligned orthogonal features, non-linear feature representations, and compositional representation without features.
I conclude by listing a few reasons why it might be important to distinguish the two concepts.
Epistemic status: I wrote this "quickly" in about 12 hours, as otherwise it wouldn't have come out at all. Think of it as a (failed) experiment in writing brief and unpolished research notes, along the lines of GDM or Anthropic
Interp Updates.
Introduction
Meaningfully interpreting neural networks involves decomposing them into smaller interpretable components. For example, we might hope to look at each neuron or attention head, explain what that component is doing, and then compose our understanding of individual components into a mechanistic understanding of the model's behavior as a whole.
It would be very convenient if the natural subunits of neural networks - neurons and attention heads - are monosemantic - that is, each component corresponds to "a single concept". Unfortunately, by default, both neurons and attention heads seem to be polysemantic: many of them seemingly correspond to multiple unrelated concepts. For example, out of 307k neurons in GPT-2, GPT-4 was able to generate short explanations that captured over >50% variance for
only 5203 neurons, and a quick glance at
OpenAI microscope reveals many examples of neurons in vision models that fire on unrelated clusters
such as "poetry" and "dice".
One explanation for polysemanticity is the superposition hypothesis: polysemanticity occurs because models are (approximately) linearly representing more features[1] than their activation space has dimensions (i.e. place features in superposition). Since there are more features than neurons, it immediately follows that some neurons must correspond to more than one feature.[2]
It's worth noting that most written resources on superposition clearly distinguish between the two terms. For example, in the seminal
Toy Model of Superposition,[3] Elhage et al write:
Why are we interested in toy models? We believe they are useful proxies for studying the superposition we suspect might exist in real neural networks. But how can we know if they're actually a useful toy model? Our best validation is whether their predictions are consistent with empirical observations regarding polysemanticity.
(
Source)
Similarly, Neel Nanda's
mech interp glossary explicitly notes that the two concepts are distinct:
Subtlety: Neuron superposition implies polysemanticity (since there are more features than neurons), but not the other way round. There could be an interpretable basis of features, just not the standard basis - this creates polysemanticity but not superposition.
(
Source)
However, I've noticed empirically that many researchers and grantmakers identify the two concepts, which often causes communication issues or even confused research proposals.
Consequently, this post tries to more clearly point at the distinction and explain why it might matter. I start by discussing the two terms in more detail, give a few examples of why you might have po...
Welcome to The Nonlinear Library, where we use Text-to-Speech software to convert the best writing from the Rationalist and EA communities into audio. This is: Duct Tape security, published by Isaac King on April 26, 2024 on LessWrong.
This is a linkpost for On Duct Tape and Fence Posts.
Eliezer writes about fence post security. When people think to themselves "in the current system, what's the weakest point?", and then dedicate their resources to shoring up the defenses at that point, not realizing that after the first small improvement in that area, there's likely now a new weakest point somewhere else.
Fence post security happens preemptively, when the designers of the system fixate on the most salient aspect(s) and don't consider the rest of the system. But this sort of fixation can also happen in retrospect, in which case it manifest a little differently but has similarly deleterious effects.
Consider a car that starts shaking whenever it's driven. It's uncomfortable, so the owner gets a pillow to put on the seat. Items start falling off the dash, so they get a tray to put them in. A crack forms, so they tape over it.
I call these duct tape solutions. They address symptoms of the problem, but not the root cause. The underlying issue still exists and will continue to cause problems until it's addressed directly.1
Did you know it's illegal to trade onion futures in the United States? In 1955, some people cornered the market on onions, shorted onion futures, then flooded the market with their saved onions, causing a bunch of farmers to lose money. The government responded by banning the sale of futures contracts on onions.
Not by banning futures trading on all perishable items, which would be equally susceptible to such an exploit. Not by banning market-cornering in general, which is pretty universally disliked. By banning a futures contracts on onions specifically. So of course the next time someone wants to try such a thing, they can just do it with tomatoes.
Duct-tape fixes are common in the wake of anything that goes publicly wrong. When people get hurt, they demand change, and they pressure whoever is in charge to give it to them. But implementing a proper fix is generally more complicated (since you have to perform a root cause analysis), less visible (therefore not earning the leader any social credit), or just plain unnecessary (if the risk was already priced in).
So the incentives are in favor of quickly slapping something together that superficially appears to be a solution, without regards for whether it makes sense.
Of course not all changes in the wake of a disaster are duct-tape fixes. A competent organization looks at disasters as something that gives them new information about the system in question; they then think about how they would design the system from scratch taking that information into account, and proceed from there to make changes. Proper solutions involve attempts to fix a general class of issues, not just the exact thing that failed.
Bad: "Screw #8463 needs to be reinforced."
Better: "The unexpected failure of screw #8463 demonstrates that the structural simulation we ran before construction contained a bug. Let's fix that bug and re-run the simulation, then reinforce every component that falls below the new predicted failure threshold."
Even better: "The fact that a single bug in our simulation software could cause a catastrophic failure is unacceptable. We need to implement multiple separate methods of advance modeling and testing that won't all fail in the same way if one of them contains a flaw."
Ideal: "The fact that we had such an unsafe design process in the first place means we likely have severe institutional disfunction. We need to hire some experienced safety/security professionals and give them the authority necessary to identify any other flaws that may exist in our company, including whatever processes in our leadership and hiring teams led to us not having such a security team ...
Welcome to The Nonlinear Library, where we use Text-to-Speech software to convert the best writing from the Rationalist and EA communities into audio. This is: Scaling of AI training runs will slow down after GPT-5, published by Maxime Riché on April 26, 2024 on LessWrong.
My credence: 33% confidence in the claim that the growth in the number of GPUs used for training SOTA AI will slow down significantly directly after GPT-5. It is not higher because of (1) decentralized training is possible, and (2) GPT-5 may be able to increase hardware efficiency significantly, (3) GPT-5 may be smaller than assumed in this post, (4) race dynamics.
TLDR: Because of a bottleneck in energy access to data centers and the need to build OOM larger data centers.
Update: See Vladimir_Nesov's comment below for why this claim is likely wrong, since decentralized training seems to be solved.
The reasoning behind the claim:
Current large data centers consume around 100 MW of power, while a single nuclear power plant generates 1GW. The largest seems to consume 150 MW.
An A100 GPU uses 250W, and around 1kW with overheard. B200 GPUs, uses ~1kW without overhead. Thus a 1MW data center can support maximum 1k to 2k GPUs.
GPT-4 used something like 15k to 25k GPUs to train, thus around 15 to 25MW.
Large data centers are around 10-100 MW. This is likely one of the reason why top AI labs are mostly only using ~ GPT-4 level of FLOPS to train new models.
GPT-5 will mark the end of the fast scaling of training runs.
A 10-fold increase in the number of GPUs above GPT-5 would require a 1 to 2.5 GW data center, which doesn't exist and would take years to build, OR would require decentralized training using several data centers. Thus GPT-5 is expected to mark a significant slowdown in scaling runs. The power consumption required to continue scaling at the current rate is becoming unsustainable, as it would require the equivalent of multiple nuclear power plants.
I think this is basically what Sam Altman, Elon Musk and Mark Zuckerberg are saying in public interviews.
The main focus to increase capabilities will be one more time on improving software efficiency. In the next few years, investment will also focus on scaling at inference time and decentralized training using several data centers.
If GPT-5 doesn't unlock research capabilities, then after GPT-5, scaling capabilities will slow down for some time towards historical rates, with most gains coming from software improvements, a bit from hardware improvement, and significantly less than currently from scaling spending.
Scaling GPUs will be slowed down by regulations on lands, energy production, and build time. Training data centers may be located and built in low-regulation countries. E.g., the Middle East for cheap land, fast construction, low regulation, and cheap energy, thus maybe explaining some talks with Middle East investors.
Unrelated to the claim:
Hopefully, GPT-5 is still insufficient for self-improvement:
Research has pretty long horizon tasks that may require several OOM more compute.
More accurate world models may be necessary for longer horizon tasks and especially for research (hopefully requiring the use of compute-inefficient real, non-noisy data, e.g., real video).
"Hopefully", moving to above human level requires RL.
"Hopefully", RL training to finetune agents is still several OOM less efficient than pretraining and/or is currently too noisy to improve the world model (this is different than simply shaping propensities) and doesn't work in the end.
Guessing that GPT-5 will be at expert human level on short horizon tasks but not on long horizon tasks nor on doing research (improving SOTA), and we can't scale as fast as currently above that.
How big is that effect going to be?
Using values from: https://epochai.org/blog/the-longest-training-run, we have estimates that in a year, the effective compute is increased by:
Software efficiency: x1.7/year (1 OOM in 3.9 y)
Hardware efficiency: x1.3/year ...
Welcome to The Nonlinear Library, where we use Text-to-Speech software to convert the best writing from the Rationalist and EA communities into audio. This is: Spatial attention as a "tell" for empathetic simulation?, published by Steven Byrnes on April 26, 2024 on LessWrong.
(Half-baked work-in-progress. There might be a "version 2" of this post at some point, with fewer mistakes, and more neuroscience details, and nice illustrations and pedagogy etc. But it's fun to chat and see if anyone has thoughts.)
1. Background
There's a neuroscience problem that's had me stumped since almost the very beginning of when I became interested in neuroscience at all (as a lens into AGI safety) back in 2019. But I think I might finally have "a foot in the door" towards a solution!
What is this problem? As described in my post
Symbol Grounding and Human Social Instincts, I believe the following:
(1) We can divide the brain into a "Learning Subsystem" (cortex, striatum, amygdala, cerebellum and a few other areas) on the one hand, and a "Steering Subsystem" (mostly hypothalamus and brainstem) on the other hand; and a human's "innate drives" (roughly equivalent to the reward function in reinforcement learning) are calculated by a bunch of specific, genetically-specified
"business logic" housed in the latter subsystem;
(2) Some of those "innate drives" are related to human social instincts - a suite of reactions that are upstream of things like envy and compassion;
(3) It might be helpful for AGI safety (for reasons briefly summarized
here) if we understood exactly how those particular drives worked. Ideally this would look like legible pseudocode that's simultaneously compatible with behavioral observations (including everyday experience), with evolutionary considerations, and with a neuroscience-based story of how that pseudocode is actually implemented by neurons in the brain. (
Different example of what I think it looks like to make progress towards that kind of pseudocode.)
(4) Explaining how those innate drives work is tricky in part because of the "symbol grounding problem", but it probably centrally involves "transient empathetic simulations" (see
§13.5 of the post linked at the top);
(5) …and therefore there needs to be some mechanism in the brain by which the "Steering Subsystem" (hypothalamus & brainstem) can tell whether the "Learning Subsystem" (cortex etc.) world-model is being queried for the purpose of a "transient empathetic simulation", or whether that same world-model is instead being queried for some other purpose, like recalling a memory, considering a possible plan, or perceiving what's happening right now.
As an example of (5), if Zoe is yelling at me, then when I look at Zoe, a thought might flash across my mind, for a fraction of a second, wherein I mentally simulate Zoe's angry feelings. Alternatively, I might imagine myself potentially feeling angry in the future. Both of those possible thoughts involve my cortex sending a weak but legible-to-the-brainstem ("grounded") anger-related signal to the hypothalamus and brainstem (mainly via the amygdala) (I claim).
But the hypothalamus and brainstem have presumably evolved to trigger different reactions in those two cases, because the former but not the latter calls for a specific social reaction to Zoe's anger. For example, in the former case, maybe Zoe's anger would trigger in me a reaction to feel anger back at Zoe in turn, although not necessarily because there are other inputs to the calculation as well.
So I think there has to be some mechanism by which the hypothalamus and/or brainstem can figure out whether or not a (transient) empathetic simulation was upstream of those anger-related signals. And I don't know what that mechanism is.
I came into those five beliefs above rather quickly - the first time I mentioned that I was confused about how (5) works, it was way back in my
second-ever neuroscience blog post, maybe within the first 50 hours of my trying to teach m...
Welcome to The Nonlinear Library, where we use Text-to-Speech software to convert the best writing from the Rationalist and EA communities into audio. This is: Lessons from two pioneering advocates for farmed animals, published by LewisBollard on April 26, 2024 on The Effective Altruism Forum.
Note: This post was crossposted from the Open Philanthropy Farm Animal Welfare Research Newsletter by the Forum team, with the author's permission. The author may not see or respond to comments on this post.
What would Ruth and Henry do?
How much can one person achieve for animals? Ruth Harrison (1920-2000) and Henry Spira (1927-1998) started out pessimistic. They inherited an animal welfare movement that had generated more noise than results, especially for farmed animals.
As factory farming arose in the mid 20th Century, the movement paid little attention. Moderate groups, like the ASPCA and RSPCA, were too busy sheltering lost cats and dogs - a role that had largely supplanted their original missions to win legal reforms for all animals.
Radical activists, meanwhile, were waging an endless war on animal testing. "Self-righteous antivivisection societies had been hollering, 'Abolition! All or Nothing!,'" Spira
recalled, noting that during that time animal testing had skyrocketed. "That was a pitiful track record, and it seemed a good idea to rethink strategies which have a century-long record of failure."
Harrison and Spira shook up this impasse. Harrison's 1964 book Animal Machines exposed factory farming to a mass audience and led to the world's first on-farm animal welfare laws. Spira's campaigns won the world's first corporate animal welfare policies, first for lab animals and then farmed animals.
Today's movement, which has won dozens of laws and thousands of corporate policies to protect factory farmed animals, owes much to Harrison and Spira. So how did they do it? And what can we learn from them?
Ruth-lessly effective advocacy
In 1960, an obscure grassroots group, the Crusade Against All Cruelty to Animals, pushed a leaflet against "factory farming" through Ruth Harrison's door. They got lucky. The leaflet prompted Harrison, a Quaker peace activist and life-long vegetarian, to
reflect that "in doing nothing I was allowing it to happen." She set out to study the issue.
The result was
Animal Machines, the first book to document the cruelty of factory farms. With graphic images and vivid prose, she described a system "where the animal is not allowed to live before it dies." She called for a slate of political reforms.
Harrison then expertly promoted her book. She got Rachel Carson, the author of Silent Spring, to write a foreword. Harrison leveraged Carson's endorsement to get a top publisher and to serialize the book in a London newspaper.
The book's publication sparked an outcry loud enough to force a reluctant UK Ministry of Agriculture to order a commission of inquiry. The resulting Brambell Commission called for farms to provide animals with
Five Freedoms, which guide many animal welfare policies to this day.
A few years later, the UK government passed a farm animal welfare law and established the Farm Animal Welfare Committee, on which Harrison served. These reforms partly inspired the European Convention on the Protection of Animals Kept for Farming Purposes, which led to all modern EU farm animal welfare laws.
Harrison's work also motivated the animal welfare movement, including the RSPCA, to re-engage with farmed animals. And her work helped inspire a young Australian philosopher to write an
article in the New York Review of Books entitled "Animal Liberation."
Henry for the hens
Henry Spira read that article. A former union organizer and civil rights activist, Spira would later
recall that "I decided that animal liberation was the logical extension of what my life was all about - identifying with the powerless and vulnerable."
His first campaign took on cruel experiments on cats at the American Museum of Natural Histor...
Welcome to The Nonlinear Library, where we use Text-to-Speech software to convert the best writing from the Rationalist and EA communities into audio. This is: Bringing Monitoring, Evaluation and, Learning to animal advocates: 6 months of lessons learned, published by Nicoll Peracha on April 26, 2024 on The Effective Altruism Forum.
Introduction and Summary
Why focus on Monitoring, Evaluation, and Learning in the animal cause area?
When we started our international interventions in September 2023, we were quite certain that MEL could increase the cost-effectiveness and impact of interventions in the animal cause area, and avoid doing harm. See e.g. this post about why Anima International suspended the campaign to end live fish sales in Poland (Anima International 2022).
Tools and insights from MEL can help organizations design potentially more (cost)-effective interventions from the start, know if their interventions are on track, and adapt their implementation when necessary.
We also believe MEL can contribute to increasing the evidence base for interventions in the animal cause area. Neill Buddy Shah, a co-founder of IDInsight, observed, "The animal welfare research infrastructure and ecosystem is incredibly immature compared to what has developed over decades in social policy, medicine, and public health." (EAG San Francisco 2019).
Since 2019, research and the number of animal-cause area-specific research databases have increased (Navigating Research Databases for Animal Advocacy, 2024). However, the amount of research available still pales compared to other cause areas.
Uncertainties and findings
We were less certain about the willingness and ability of Animal and Vegan Advocacy organizations to engage with MEL. We also didn't know if MEL tools used in other cause areas such as Global Health and Development would be applicable and useful in the animal cause area.
Overall, MEL is still a neglected topic in the animal community. EA-aligned organizations generally use MEL tools but many others don't, and so far we have only verified a handful of organizations that have complete MEL systems in place that do not require additional support.
Specialized support for MEL is still very limited. If you are interested in supporting animal organizations with MEL, please consider working with us as MEL Associate or communications volunteer.
Below you will find 11 key lessons learned from our pilot intervention to train and support animal and vegan advocacy organizations in Monitoring, Evaluation, and Learning.
We hope this post will be particularly relevant for charities and funders in the animal cause area and will lead to more organizations engaging with MEL and sharing best practices.
In this post, we will share what we've done so far, 11 key lessons we've learned, how they have influenced our strategy, and what you can do to help advance MEL and the overall evidence base in the animal cause area.
I. What have we done so far?
The Mission Motor's current interventions were shaped during AIM's (Charity Entrepreneurship) Incubation Program 2023. Between September 2023 and April 2024, we
trained and supported eight animal and vegan advocacy charities to develop and implement MEL systems
provided ad hoc support to another seven animal organizations
are building a community of MEL peers and practitioners through Slack and monthly meetings
Our interventions are in the ideation phase with a focus on learning if, and why or why not, they work as intended. Although we are still in the first half of our pilot program, we see some early successes. The training and coaching sessions helped staff members
increase their knowledge of MEL
design theories of change
identify key assumptions and risks
unify the collective understanding of their programs.
As a result, several organizations identified key activities they wish to add to their program, decided to focus their efforts on specific target groups, or otherwise change their program design.
To ...
Welcome to The Nonlinear Library, where we use Text-to-Speech software to convert the best writing from the Rationalist and EA communities into audio. This is: An Introduction to AI Sandbagging, published by Teun van der Weij on April 26, 2024 on The AI Alignment Forum.
Summary: Evaluations provide crucial information to determine the safety of AI systems which might be deployed or (further) developed. These development and deployment decisions have important safety consequences, and therefore they require trustworthy information. One reason why evaluation results might be untrustworthy is sandbagging, which we define as strategic underperformance on an evaluation.
The strategic nature can originate from the developer (developer sandbagging) and the AI system itself (AI system sandbagging). This post is an introduction to the problem of sandbagging.
The Volkswagen emissions scandal
There are environmental regulations which require the reduction of harmful emissions from diesel vehicles, with the goal of protecting public health and the environment. Volkswagen struggled to meet these emissions standards while maintaining the desired performance and fuel efficiency of their diesel engines (Wikipedia).
Consequently, Volkswagen intentionally installed defeat devices in millions of vehicles to lower the amount of emissions only during tests, allowing the cars to emit up to 40 times the permitted levels of nitrogen oxides during normal driving conditions. The scandal showed corporate misconduct and a lack of effective oversight, and is a form of sandbagging. But clearly, cars and their manufacturers are different to AI systems and their developers. So, how does sandbagging relate to AI systems?
Sandbagging in the context of AI
Evaluations provide crucial information to determine the safety of AI systems which might be deployed or (further) developed. Frontier labs rely on evaluations to reduce catastrophic risks, as stated in e.g. OpenAI's preparedness framework and Anthropic's responsible scaling policy. Governmental institutions like the UK AI Safety Institute and the EU AI Office also utilize evaluations to manage risks. However, sandbagging can reduce the reliability of these evaluations.
Both the developers of an AI system and the AI system itself have incentives to sandbag. For example, the developer might want to appease stakeholders, and the AI system might want to acquire more resources after an evaluation to better achieve its goals. Importantly, sandbagging is technically feasible[1] and neglected. We hope this post leads to a better understanding of the problem of sandbagging.
Definition
We define sandbagging as strategic underperformance on an evaluation.
This has three main concepts: strategic, underperformance, and evaluation (or: eval), which we discuss below in turn. Notably, we use the term 'AI system' to describe various types of AIs, such as standalone models and models augmented with tools/scaffolding (Shavit et al., 2023).
Strategic
Strategic means that the developer of the AI system and/or the AI system itself underperforms on an evaluation because it believes it will benefit.[2] If the strategy comes from the developer, we call it developer sandbagging (relating to misuse). If the strategy comes from the AI system, we call it AI system sandbagging (relating to misalignment). We use the term developer to mean any entity responsible for the AI system being evaluated.
These entities have been called developers, deployers, or providers elsewhere (1, 2, 3).
By including the term 'strategic' in the definition of sandbagging, we exclude accidental underperformance. In other words, this condition captures the fact that underperformance was intentional, which is a central condition for legal responsibility, including in the context of AI (Ashton, 2022; Halpern, 2018; Ward, 2024). Non-strategic, or accidental, sandbagging brings about other less important safety problems.
Underperformance
Underperformance occurs whe...
Welcome to The Nonlinear Library, where we use Text-to-Speech software to convert the best writing from the Rationalist and EA communities into audio. This is: Losing Faith In Contrarianism, published by omnizoid on April 26, 2024 on LessWrong.
Crosspost from my blog.
If you spend a lot of time in the blogosphere, you'll find a great deal of people expressing contrarian views. If you hang out in the circles that I do, you'll probably have heard of
Yudkowsky say that dieting doesn't really work, Guzey say that sleep is overrated, Hanson argue that medicine doesn't improve health, various people argue for the lab leak, others argue for hereditarianism, Caplan argue that mental illness is mostly just aberrant preferences and education doesn't work, and various other people expressing contrarian views.
Often, very smart people - like Robin Hanson - will write long posts defending these views, other people will have criticisms, and it will all be such a tangled mess that you don't really know what to think about them.
For a while, I took a lot of these contrarian views pretty seriously. If I'd had to bet 6-months ago, I'd have bet on the lab leak, at maybe 2 to 1 odds. I'd have had significant credence in Hanson's view that healthcare doesn't improve health until pretty recently, when Scott released his post explaining why it is wrong.
Over time, though, I've become much less sympathetic to these contrarian views. It's become increasingly obvious that the things that make them catch on are unrelated to their truth. People like being provocative and tearing down sacred cows - as a result, when a smart articulate person comes along defending some contrarian view - perhaps one claiming that something we think is valuable is really worthless - the view spreads like wildfire, even if it's pretty implausible.
Sam Atis has an article titled
The Case Against Public Intellectuals. He starts it by noting a surprising fact: lots of his friends think education has no benefits. This isn't because they've done a thorough investigation of the literature - it's because they've read Bryan Caplan's book arguing for that thesis. Atis notes that there's a literature review finding that education has significant benefits, yet it's written by boring academics, so no one has read it.
Everyone wants to read the contrarians who criticize education - no one wants to read the boring lit reviews that say what we believed about education all along is right.
Sam is right, yet I think he understates the problem. There are various topics where arguing for one side of them is inherently interesting, yet arguing for the other side is boring. There are a lot of people who read Austian economics blogs, yet no one reads (or writes) anti-Austrian economics blogs. That's because there are a lot of fans of Austrians economics - people who are willing to read blogs on the subject - but almost no one who is really invested in Austrian economics being wrong.
So as a result, in general, the structural incentives of the blogosphere favor being a contrarian.
Thus, you should expect the sense of the debate you get, unless you peruse the academic literature in depth surrounding some topic, to be wildly skewed towards contrarian views. And I think this is exactly what we observe.
I've seen the contrarians be wrong over and over again - and this is what really made me lose faith in them. Whenever I looked more into a topic, whenever I got to the bottom of the full debate, it always seemed like the contrarian case fell apart.
It's easy for contrarians to portray their opponents as the kind of milquetoast bureaucrats who aren't very smart and follow the consensus just because it is the consensus. If Bryan Caplan has a disagreement with a random administrator, I trust that Bryan Caplan's probably right, because he's smarter and cares more about ideas.
But what I've come to realize is that the mainstream view that's supported by most of the academics tends to be supported by some r...
Welcome to The Nonlinear Library, where we use Text-to-Speech software to convert the best writing from the Rationalist and EA communities into audio. This is: EA Meta Funding Landscape Report, published by Joel Tan on April 26, 2024 on The Effective Altruism Forum.
The Centre for Exploratory Altruism Research (CEARCH) is an EA organization working on cause prioritization research as well as grantmaking and donor advisory. This project was commissioned by the leadership of the Meta Charity Funders (MCF) - also known as the Meta Charity Funding Circle (MCFC) - with the objective of identifying what is underfunded vs overfunded in EA meta. The views expressed in this report are CEARCH's and do not necessarily reflect the position of the MCF.
Generally, by meta we refer to projects whose theory of change is indirect, and involving improving the EA movement's ability to do good - for example, via cause/intervention/charity prioritization (i.e. improving our knowledge of what is cost-effective); effective giving (i.e. increasing the pool of money donated in an impact-oriented way); or talent development (i.e. increasing the pool and ability of people willing and able to work in impactful careers).
The full report may be found here (link). Note that the public version of the report is partially redacted, to respect the confidentiality of certain grants, as well as the anonymity of the people whom we interviewed or surveyed.
Quantitative Findings
Detailed Findings
To access our detailed findings, refer to our spreadsheet (
link).
Overall Meta Funding
Aggregate EA meta funding saw rapid growth and equally rapid contraction over 2021 to 2023 - growing from 109 million in 2021 to 193 million in 2022, before shrinking back to 117 million in 2023. The analysis excludes FTX, as ongoing clawbacks mean that their funding has functioned less as grants and more as loans.
Open Philanthropy is by far the biggest funder in the space, and changes in the meta funding landscape are largely driven by changes in OP's spending. And indeed, OP's global catastrophic risks (GCR) capacity building grants tripled from 2021 to 2022, before falling to twice the 2021 baseline in 2023.
This finding is in line with Tyler Maule's
previous analysis.
Meta Funding by Cause Area
The funding allocation by cause was, in descending order: (most funding) longtermism (i.e. AI, biosecurity, nuclear etc) (274 million) >> global health and development (GHD) (67 million) > cross-cause (53 million) > animal welfare (25 million) (least funding).
Meta Funding by Intervention
The funding allocation by intervention was, in descending order: (most funding) other/miscellaneous (e.g. general community building, including by national/local EA organizations; events; community infrastructure; co-working spaces; fellowships for community builders; production of EA-adjacent media content; translation projects; student outreach; and book purchases etc) (193 million) > talent (121 million) > prioritization (92 million) >> effective giving (13 million) (least funding).
One note of caution - we believe our results overstate how well funded prioritization is, relative to the other three intervention types.
We take into account what grantmakers spend internally on prioritization research, but for lack of time, we do not perform an equivalent analysis for non-grantmakers (i.e. imputing their budget to effective giving, talent, and other/miscellaneous).
For simplicity, we classified all grantmakers (except GWWC) as engaging in prioritization, though some grantmakers (e.g. Founders Pledge, Longview) also do effective giving work.
Meta Funding by Cause Area & Intervention
The funding allocation by cause/intervention subgroup was as follows:
The areas with the most funding were: longtermist other/miscellaneous (153 million) > longtermist talent (106 million) > GHD prioritization (51 million).
The areas with the least funding were GHD other/miscellaneous (3,000,000) > animal welfare effective givi...
Welcome to The Nonlinear Library, where we use Text-to-Speech software to convert the best writing from the Rationalist and EA communities into audio. This is: LLMs seem (relatively) safe, published by JustisMills on April 26, 2024 on LessWrong.
Post for a somewhat more general audience than the modal LessWrong reader, but gets at my actual thoughts on the topic.
In 2018 OpenAI
defeated the world champions of Dota 2, a major esports game. This was hot on the heels of DeepMind's AlphaGo performance
against Lee Sedol in 2016, achieving superhuman Go performance way before anyone thought that might happen. AI benchmarks were being cleared at a pace which felt breathtaking at the time, papers were proudly published, and ML tools like Tensorflow (released in 2015) were coming online. To people already interested in AI, it was an exciting era. To everyone else, the world was unchanged.
Now Saturday Night Live sketches use sober discussions of AI risk
as the backdrop for their actual jokes, there are
hundreds of AI bills moving through the world's legislatures, and Eliezer Yudkowsky
is featured in Time Magazine.
For people who have been predicting, since well before AI was cool (and now passe), that it could spell doom for humanity, this explosion of mainstream attention is a dark portent. Billion dollar AI companies keep springing up and allying with the largest tech companies in the world, and bottlenecks like money, energy, and talent are widening considerably. If current approaches can get us to superhuman AI in principle, it seems like they will in practice, and soon.
But what if large language models, the vanguard of the AI movement, are actually safer than what came before? What if the path we're on is less perilous than what we might have hoped for, back in 2017? It seems that way to me.
LLMs are self limiting
To train a large language model, you need an absolutely massive amount of data. The core thing these models are doing is predicting the next few letters of text, over and over again, and they need to be trained on billions and billions of words of human-generated text to get good at it.
Compare this process to
AlphaZero, DeepMind's algorithm that superhumanly masters Chess, Go, and Shogi. AlphaZero trains by playing against itself. While older chess engines bootstrap themselves by observing the records of countless human games, AlphaZero simply learns by doing. Which means that the only bottleneck for training it is computation - given enough energy, it can just play itself forever, and keep getting new data.
Not so with LLMs: their source of data is human-produced text, and human-produced text is a finite resource.
The precise datasets used to train cutting-edge LLMs are secret, but let's suppose that they include a fair bit of the low hanging fruit: maybe 5% of publicly available text that is in principle available and not garbage. You can schlep your way to a 20x bigger dataset in that case, though you'll hit diminishing returns as you have to, for example, generate transcripts of random videos and filter old mailing list threads for metadata and spam.
But nothing you do is going to get you 1,000x the training data, at least not in the short run.
Scaling laws are among the watershed discoveries of ML research in the last decade; basically, these are equations that project how much oomph you get out of increasing the size, training time, and dataset that go into a model. And as it turns out, the amount of high quality data is extremely important, and often becomes the bottleneck.
It's easy to take this fact for granted now, but it wasn't always obvious! If computational power or model size was usually the bottleneck, we could just make bigger and bigger computers and reliably get smarter and smarter AIs. But that only works to a point, because it turns out we need high quality data too, and high quality data is finite (and, as the political apparatus wakes up to what's going on,
legally fraught).
There are rumbling...
Welcome to The Nonlinear Library, where we use Text-to-Speech software to convert the best writing from the Rationalist and EA communities into audio. This is: Animals in Cost-Benefit Analysis, published by Vasco Grilo on April 25, 2024 on The Effective Altruism Forum.
This is a linkpost for Animals in Cost-Benefit Analysis by Andrew Stawasz. The article is forthcoming in the University of Michigan Journal of Law Reform.
Abstract
Federal agencies' cost-benefit analyses do not capture nonhuman animals' ("animals'") interests. This omission matters. Cost-benefit analysis drives many regulatory decisions that substantially affect many billions of animals. That omission creates a regulatory blind spot that is untenable as a matter of morality and of policy.
This Article advances two claims related to valuing animals in cost-benefit analyses. The Weak Claim argues that agencies typically may do so. No legal prohibitions usually exist, and such valuation is within agencies' legitimate discretion. The Strong Claim argues that agencies often must do so if a policy would substantially affect animals. Cost-benefit analysis is concerned with improving welfare, and no argument for entirely omitting animals' welfare holds water.
Agencies have several options to implement this vision. These options include, most preferably, human-derived valuations (albeit in limited circumstances), interspecies comparisons, direct estimates of animals' preferences, and, at a minimum, breakeven analysis. Agencies could deal with uncertainty by conducting sensitivity analyses or combining methods.
For any method, agencies should consider what happens when a policy would save animals from some bad outcomes and what form a mandate to value animals should take.
Valuing animals could have mattered for many cost-benefit analyses, including those for pet-food safety regulations and a rear backup camera mandate. As a sort of "proof of concept," this Article shows that even a simple breakeven analysis from affected animals' perspective paints even the thoroughly investigated policy decision at issue in Entergy Corp. v. Riverkeeper, Inc. in an informative new light.
Table of contents
Thanks for listening. To help us out with The Nonlinear Library or to learn more, please visit nonlinear.org
Welcome to The Nonlinear Library, where we use Text-to-Speech software to convert the best writing from the Rationalist and EA communities into audio. This is: WSJ: Inside Amazon's Secret Operation to Gather Intel on Rivals, published by trevor on April 25, 2024 on LessWrong.
The operation, called Big River Services International, sells around $1 million a year of goods through e-commerce marketplaces including
eBay,
Shopify,
Walmart and
Amazon AMZN 1.49%increase; green up pointing triangle.com under brand names such as Rapid Cascade and Svea Bliss. "We are entrepreneurs, thinkers, marketers and creators," Big River says on its website. "We have a passion for customers and aren't afraid to experiment."
What the website doesn't say is that Big River is an arm of Amazon that surreptitiously gathers intelligence on the tech giant's competitors.
Born out of a 2015 plan code named "Project Curiosity," Big River uses its sales across multiple countries to obtain pricing data, logistics information and other details about rival e-commerce marketplaces,
logistics operations and payments services, according to people familiar with Big River and corporate documents viewed by The Wall Street Journal. The team then shared that information with Amazon to incorporate into decisions about its own business.
...
The story of Big River offers new insight into Amazon's
elaborate efforts to stay ahead of rivals. Team members attended their rivals' seller conferences and met with competitors identifying themselves only as employees of Big River Services, instead of disclosing that they worked for Amazon.
They were given non-Amazon email addresses to use externally - in emails with people at Amazon, they used Amazon email addresses - and took other extraordinary measures to keep the project secret. They disseminated their reports to Amazon executives using printed, numbered copies rather than email. Those who worked on the project weren't even supposed to discuss the relationship internally with most teams at Amazon.
An internal crisis-management paper gave advice on what to say if discovered. The response to questions should be: "We make a variety of products available to customers through a number of subsidiaries and online channels." In conversations, in the event of a leak they were told to focus on the group being formed to improve the seller experience on Amazon, and say that such research is normal, according to people familiar with the discussions.
Senior Amazon executives, including Doug Herrington, Amazon's current CEO of Worldwide Amazon Stores, were regularly briefed on the Project Curiosity team's work, according to one of the people familiar with Big River.
...
Virtually all companies research their competitors, reading public documents for information, buying their products or shopping their stores. Lawyers say there is a difference between such corporate intelligence gathering of publicly available information, and what is known as corporate or industrial espionage.
Companies can get into legal trouble for actions such as hiring a rival's former employee to obtain trade secrets or hacking a rival. Misrepresenting themselves to competitors to gain proprietary information can lead to suits on trade secret misappropriation, said Elizabeth Rowe, a professor at the University of Virginia School of Law who specializes in trade secret law.
...
The benchmarking team pitched "Project Curiosity" to senior management and got the approval to buy inventory, use a shell company and find warehouses in the U.S., Germany, England, India and Japan so they could pose as sellers on competitors' websites.
...
Once launched, the focus of the project quickly started shifting to gathering information about rivals, the people said.
...
The team presented its findings from being part of the FedEx program to senior Amazon logistics leaders. They used the code name "OnTime Inc." to refer to FedEx. Amazon made changes to its Fulfillment by Amazon service to ...
Welcome to The Nonlinear Library, where we use Text-to-Speech software to convert the best writing from the Rationalist and EA communities into audio. This is: "Why I Write" by George Orwell (1946), published by Arjun Panickssery on April 25, 2024 on LessWrong.
People have been posting great essays so that they're "fed through the standard LessWrong algorithm." This essay is in the public domain in the UK but not the US.
From a very early age, perhaps the age of five or six, I knew that when I grew up I should be a writer. Between the ages of about seventeen and twenty-four I tried to abandon this idea, but I did so with the consciousness that I was outraging my true nature and that sooner or later I should have to settle down and write books.
I was the middle child of three, but there was a gap of five years on either side, and I barely saw my father before I was eight. For this and other reasons I was somewhat lonely, and I soon developed disagreeable mannerisms which made me unpopular throughout my schooldays. I had the lonely child's habit of making up stories and holding conversations with imaginary persons, and I think from the very start my literary ambitions were mixed up with the feeling of being isolated and undervalued.
I knew that I had a facility with words and a power of facing unpleasant facts, and I felt that this created a sort of private world in which I could get my own back for my failure in everyday life. Nevertheless the volume of serious - i.e. seriously intended - writing which I produced all through my childhood and boyhood would not amount to half a dozen pages. I wrote my first poem at the age of four or five, my mother taking it down to dictation.
I cannot remember anything about it except that it was about a tiger and the tiger had 'chair-like teeth' - a good enough phrase, but I fancy the poem was a plagiarism of Blake's 'Tiger, Tiger'. At eleven, when the war or 1914-18 broke out, I wrote a patriotic poem which was printed in the local newspaper, as was another, two years later, on the death of Kitchener. From time to time, when I was a bit older, I wrote bad and usually unfinished 'nature poems' in the Georgian style.
I also, about twice, attempted a short story which was a ghastly failure. That was the total of the would-be serious work that I actually set down on paper during all those years.
However, throughout this time I did in a sense engage in literary activities. To begin with there was the made-to-order stuff which I produced quickly, easily and without much pleasure to myself. Apart from school work, I wrote vers d'occasion, semi-comic poems which I could turn out at what now seems to me astonishing speed - at fourteen I wrote a whole rhyming play, in imitation of Aristophanes, in about a week - and helped to edit school magazines, both printed and in manuscript.
These magazines were the most pitiful burlesque stuff that you could imagine, and I took far less trouble with them than I now would with the cheapest journalism. But side by side with all this, for fifteen years or more, I was carrying out a literary exercise of a quite different kind: this was the making up of a continuous "story" about myself, a sort of diary existing only in the mind. I believe this is a common habit of children and adolescents.
As a very small child I used to imagine that I was, say, Robin Hood, and picture myself as the hero of thrilling adventures, but quite soon my "story" ceased to be narcissistic in a crude way and became more and more a mere description of what I was doing and the things I saw. For minutes at a time this kind of thing would be running through my head: 'He pushed the door open and entered the room.
A yellow beam of sunlight, filtering through the muslin curtains, slanted on to the table, where a matchbox, half-open, lay beside the inkpot. With his right hand in his pocket he moved across to the window. Down in the street a tortoiseshell cat was chasing a dead leaf,' etc., etc. Thi...
Welcome to The Nonlinear Library, where we use Text-to-Speech software to convert the best writing from the Rationalist and EA communities into audio. This is: AXRP Episode 29 - Science of Deep Learning with Vikrant Varma, published by DanielFilan on April 25, 2024 on The AI Alignment Forum.
In 2022, it was announced that a fairly simple method can be used to extract the true beliefs of a language model on any given topic, without having to actually understand the topic at hand.
Earlier, in 2021, it was announced that neural networks sometimes 'grok': that is, when training them on certain tasks, they initially memorize their training data (achieving their training goal in a way that doesn't generalize), but then suddenly switch to understanding the 'real' solution in a way that generalizes.
What's going on with these discoveries? Are they all they're cracked up to be, and if so, how are they working? In this episode, I talk to Vikrant Varma about his research getting to the bottom of these questions.
Topics we discuss:
Challenges with unsupervised LLM knowledge discovery, aka contra CCS
What is CCS?
Consistent and contrastive features other than model beliefs
Understanding the banana/shed mystery
Future CCS-like approaches
CCS as principal component analysis
Explaining grokking through circuit efficiency
Why research science of deep learning?
Summary of the paper's hypothesis
What are 'circuits'?
The role of complexity
Many kinds of circuits
How circuits are learned
Semi-grokking and ungrokking
Generalizing the results
Vikrant's research approach
The DeepMind alignment team
Follow-up work
Daniel Filan: Hello, everybody. In this episode I'll be speaking with Vikrant Varma, a research engineer at Google DeepMind, and the technical lead of their sparse autoencoders effort. Today, we'll be talking about his research on problems with contrast-consistent search, and also explaining grokking through circuit efficiency. For links what we're discussing, you can check the description of this episode and you can read the transcript at axrp.net.
All right, well, welcome to the podcast.
Vikrant Varma: Thanks, Daniel. Thanks for having me.
Challenges with unsupervised LLM knowledge discovery, aka contra CCS
What is CCS?
Daniel Filan: Yeah. So first, I'd like to talk about this paper. It is called Challenges with Unsupervised LLM Knowledge Discovery, and the authors are Sebastian Farquhar, you, Zachary Kenton, Johannes Gasteiger, Vladimir Mikulik, and Rohin Shah. This is basically about this thing called CCS. Can you tell us: what does CCS stand for and what is it?
Vikrant Varma: Yeah, CCS stands for contrastive-consistent search. I think to explain what it's about, let me start from a more fundamental problem that we have with advanced AI systems. One of the problems is that when we train AI systems, we're training them to produce outputs that look good to us, and so this is the supervision that we're able to give to the system. We currently don't really have a good idea of how an AI system or how a neural network is computing those outputs.
And in particular, we're worried about the situation in the future when the amount of supervision we're able to give it causes it to achieve a superhuman level of performance at that task. By looking at the network, we can't know how this is going to behave in a new situation.
And so the Alignment Research Center put out a report recently about this problem. They named a potential part of this problem as "eliciting latent knowledge". What this means is if your model is, for example, really, really good at figuring out what's going to happen next in a video, as in it's able to predict the next frame of a video really well given a prefix of the video, this must mean that it has some sort of model of what's going on in the world.
Instead of using the outputs of the model, if you could directly look at what it understands about the world, then potentially, you could use that information in a much safer ...
Welcome to The Nonlinear Library, where we use Text-to-Speech software to convert the best writing from the Rationalist and EA communities into audio. This is: Improving Dictionary Learning with Gated Sparse Autoencoders, published by Neel Nanda on April 25, 2024 on The AI Alignment Forum.
Authors: Senthooran Rajamanoharan*, Arthur Conmy*, Lewis Smith, Tom Lieberum, Vikrant Varma, János Kramár, Rohin Shah, Neel Nanda
A new paper from the Google DeepMind mech interp team: Improving Dictionary Learning with Gated Sparse Autoencoders!
Gated SAEs are a new Sparse Autoencoder architecture that seems to be a significant Pareto-improvement over normal SAEs, verified on models up to Gemma 7B. They are now our team's preferred way to train sparse autoencoders, and we'd love to see them adopted by the community! (Or to be convinced that it would be a bad idea for them to be adopted by the community!)
They achieve similar reconstruction with about half as many firing features, and while being either comparably or more interpretable (confidence interval for the increase is 0%-13%).
See Sen's Twitter summary, my Twitter summary, and the paper!
Thanks for listening. To help us out with The Nonlinear Library or to learn more, please visit nonlinear.org.
Welcome to The Nonlinear Library, where we use Text-to-Speech software to convert the best writing from the Rationalist and EA communities into audio. This is: Today is World Malaria Day (April 25), published by tobytrem on April 25, 2024 on The Effective Altruism Forum.
Malaria is massive. Our World in Data writes: "Over half a million people died from the disease each year in the 2010s. Most were children, and the disease is one of the leading causes of child mortality." Or, as Rob Mather, CEO of the Against Malaria Foundation (AMF) phrases it: the equivalent of seven jumbo jets full of children die of Malaria each day.
But I don't see malaria in the news that much. This is partly because it was eradicated from Western countries over the course of the 20th century, both because of intentional interventions such as insecticide, and because of the draining of swamp lands and building of better housing. But it's also because malaria is a slow catastrophe, like poverty, and climate change.
We've dealt with it to varying degrees throughout history, and though it is an emergency to anyone affected by it, to the rest of us, it's a tropical disease which has been around forever. It can be hard to generate urgency when a problem has existed for so long.
But there is a lot that we can do. Highly effective charities work on malaria; the Against Malaria Foundation (AMF) distributes insecticide treated bed-nets, and a Malaria Consortium program offers seasonal malaria chemoprevention treatment- both are GiveWell Top Charities. Two malaria vaccines, RTS,S and the cheaper R21[1], have been developed in recent years[2]. Malaria is preventable.
Though malaria control and eradication is funded by international bodies such as The Global Fund, there isn't nearly enough money being spent on it. AMF has an immediate funding gap of $185.78m. That's money for nets they know are needed. And though vaccines are being rolled out, progress has been slower than it could be, and the agencies distributing them have been criticised for lacking urgency.
Malaria is important, malaria is neglected, malaria is tractable.
If you want to do something about malaria today, consider donating to Givewell's recommendations: AMF, or the Malaria Consortium:
Related links I recommend
Why we didn't get a malaria vaccine sooner; an article in Works in Progress.
WHO's World Malaria Day 2024 announcement.
The Our World in Data page on malaria.
Audio AMA, with Rob Mather, CEO of AMF (transcript).
From SoGive, an EA Forum discussion of the cost-effectiveness of malaria vaccines, with cameos from 1DaySooner and GiveWell.
For more info, see GiveWell's page on malaria vaccines.
The story of Tu Youyou, a researcher who helped develop an anti-malarial drug in Mao's China.
What is an Emergency? The Case for Rapid Malaria Vaccination, from Marginal Revolution.
More content on the Forum's Malaria tag.
^
R21 offers up to 75% reduction of symptomatic malaria cases when delivered at the right schedule.
^
Supported by Open Philanthropy and GiveWell.
Thanks for listening. To help us out with The Nonlinear Library or to learn more, please visit nonlinear.org
Welcome to The Nonlinear Library, where we use Text-to-Speech software to convert the best writing from the Rationalist and EA communities into audio. This is: Sustainable fishing policy increases fishing, and demand reductions might, too, published by MichaelStJules on April 25, 2024 on The Effective Altruism Forum.
Summary
Where there's overfishing, reducing fishing pressure or harvest rates - roughly the share of the population or biomass caught in a fishery per fishing period - actually allows more animals to be caught in the long run.
Sustainable fishery management policies are generally aimed at maximizing or maintaining high levels of catch - the biomass of wild aquatic animals caught - in the long run. More restrictive policies that would actually reduce long-run catch generally seem politically infeasible, and less restrictive policies that increase long-run catch don't seem like a stepping stone to more restrictive ones that decrease it.
Demand reductions for wild-caught aquatic animals may increase or decrease actual catch, and it's very unclear which. My highly uncertain tentative best guesses are that
they seem slightly more likely to increase than increase catch in the near term but bioeconomic "long run", e.g. over the next 10-20 years, and
persistent demand reductions and cumulative work towards them seem slightly more likely to decrease than increase catch over longer timelines with more sustainable fishery management and eventual population decline, but it's not clear if and when catch would actually be consistently lower on average than otherwise.
Acknowledgements
Thanks to Brian Tomasik, Ren Ryba and Tori for their feedback, and Saulius Šimčikas for his supervision on an earlier unpublished project. All errors are my own.
Basic terminology
I use 'fishing' to include the capture of any wild aquatic animal, including crustaceans, not just fish.
I refer to the long run (and long-run) in fishing as long enough for all production factors, including the number of boats/vessels, amount of fishing equipment, employment and the number of fishing companies or businesses to increase or decrease and approximately reach a new equilibrium in response to a permanent shift in prices, supply or demand. I'd expect this to typically be less than a decade. This is a standard term in economics.
Introduction
Reductions in fishing pressure or harvest rates - roughly the share of the population or biomass caught in a fishery per fishing period - can result from reductions in demand or from improvements in fishery management, like the use of quotas, smaller fishing net mesh sizes, seasonal closures or restrictions on fishing vessels or their numbers. However, these reductions can also lead to increases in catch where there's overfishing, by allowing stocks to recover, resulting in more fish to catch.
Fishery management policies that preserve or increase stocks are also typically aimed at increasing long-run catch.
If we're concerned with reducing total exploitation, injustice or harm caused by humans or moral/rational agents, then this would count against this kind of work (see also Tomasik, 2015, who made this point earlier).
The same could hold for a (weighted) average level of exploitation, injustice or harm by humans across all animals or moral patients.[1][2] I don't personally take exploitation or harm by humans in particular to be worse than other types of harms, independently of their effects on subjective welfare,[3] but this seems to be a common position.
Furthermore, harm caused by humans might matter more for indirect reasons related to subjective welfare in practice, tracking our willingness to help or make other sacrifices for other animals.[4]
Or, if fishing deaths are particularly bad compared to natural deaths and will continue to be so (e.g. humane capture and humane slaughter won't become widespread), and bad enough to be worth preventing regardless of the population effects and effects on natural deaths, then...
Welcome to The Nonlinear Library, where we use Text-to-Speech software to convert the best writing from the Rationalist and EA communities into audio. This is: The first future and the best future, published by KatjaGrace on April 25, 2024 on LessWrong.
It seems to me worth trying to slow down AI development to steer successfully around the shoals of extinction and out to utopia.
But I was thinking lately: even if I didn't think there was any chance of extinction risk, it might still be worth prioritizing a lot of care over moving at maximal speed. Because there are many different possible AI futures, and I think there's a good chance that the initial direction affects the long term path, and different long term paths go to different places. The systems we build now will shape the next systems, and so forth.
If the first human-level-ish AI is brain emulations, I expect a quite different sequence of events to if it is GPT-ish.
People genuinely pushing for AI speed over care (rather than just feeling impotent) apparently think there is negligible risk of bad outcomes, but also they are asking to take the first future to which there is a path. Yet possible futures are a large space, and arguably we are in a rare plateau where we could climb very different hills, and get to much better futures.
Thanks for listening. To help us out with The Nonlinear Library or to learn more, please visit nonlinear.org