Discover
The Nonlinear Library: Alignment Forum Top Posts
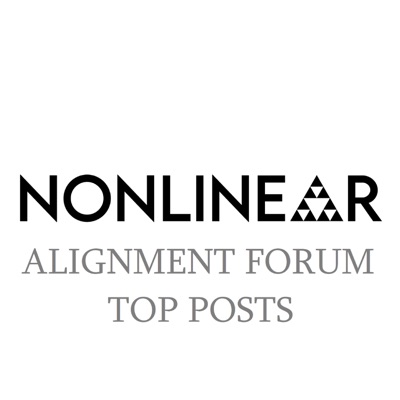
242 Episodes
Reverse
Welcome to The Nonlinear Library, where we use Text-to-Speech software to convert the best writing from the Rationalist and EA communities into audio.
This is: Discussion with Eliezer Yudkowsky on AGI interventions, published by Rob Bensinger, Eliezer Yudkowsky on the AI Alignment Forum.
The following is a partially redacted and lightly edited transcript of a chat conversation about AGI between Eliezer Yudkowsky and a set of invitees in early September 2021. By default, all other participants are anonymized as "Anonymous".
I think this Nate Soares quote (excerpted from Nate's response to a report by Joe Carlsmith) is a useful context-setting preface regarding timelines, which weren't discussed as much in the transcript:
[...] My odds [of AGI by the year 2070] are around 85%[...]
I can list a handful of things that drive my probability of AGI-in-the-next-49-years above 80%:
1. 50 years ago was 1970. The gap between AI systems then and AI systems now seems pretty plausibly greater than the remaining gap, even before accounting the recent dramatic increase in the rate of progress, and potential future increases in rate-of-progress as it starts to feel within-grasp.
2. I observe that, 15 years ago, everyone was saying AGI is far off because of what it couldn't do -- basic image recognition, go, starcraft, winograd schemas, programmer assistance. But basically all that has fallen. The gap between us and AGI is made mostly of intangibles. (Computer Programming That Is Actually Good? Theorem proving? Sure, but on my model, "good" versions of those are a hair's breadth away from full AGI already. And the fact that I need to clarify that "bad" versions don't count, speaks to my point that the only barriers people can name right now are intangibles.) That's a very uncomfortable place to be!
3. When I look at the history of invention, and the various anecdotes about the Wright brothers and Enrico Fermi, I get an impression that, when a technology is pretty close, the world looks a lot like how our world looks.
Of course, the trick is that when a technology is a little far, the world might also look pretty similar!
Though when a technology is very far, the world does look different -- it looks like experts pointing to specific technical hurdles. We exited that regime a few years ago.
4. Summarizing the above two points, I suspect that I'm in more-or-less the "penultimate epistemic state" on AGI timelines: I don't know of a project that seems like they're right on the brink; that would put me in the "final epistemic state" of thinking AGI is imminent. But I'm in the second-to-last epistemic state, where I wouldn't feel all that shocked to learn that some group has reached the brink. Maybe I won't get that call for 10 years! Or 20! But it could also be 2, and I wouldn't get to be indignant with reality. I wouldn't get to say "but all the following things should have happened first, before I made that observation". I have made those observations.
5. It seems to me that the Cotra-style compute-based model provides pretty conservative estimates. For one thing, I don't expect to need human-level compute to get human-level intelligence, and for another I think there's a decent chance that insight and innovation have a big role to play, especially on 50 year timescales.
6. There has been a lot of AI progress recently. When I tried to adjust my beliefs so that I was positively surprised by AI progress just about as often as I was negatively surprised by AI progress, I ended up expecting a bunch of rapid progress. [...]
Further preface by Eliezer:
In some sections here, I sound gloomy about the probability that coordination between AGI groups succeeds in saving the world. Andrew Critch reminds me to point out that gloominess like this can be a self-fulfilling prophecy - if people think successful coordination is impossible, they won’t try to coordinate. I therefore remark in retrospective advance that it seems to me like at least some of the top...
Welcome to The Nonlinear Library, where we use Text-to-Speech software to convert the best writing from the Rationalist and EA communities into audio.
This is: What failure looks like, published by Paul Christiano on the AI Alignment Forum.
The stereotyped image of AI catastrophe is a powerful, malicious AI system that takes its creators by surprise and quickly achieves a decisive advantage over the rest of humanity.
I think this is probably not what failure will look like, and I want to try to paint a more realistic picture. I’ll tell the story in two parts:
Part I: machine learning will increase our ability to “get what we can measure,” which could cause a slow-rolling catastrophe. ("Going out with a whimper.")
Part II: ML training, like competitive economies or natural ecosystems, can give rise to “greedy” patterns that try to expand their own influence. Such patterns can ultimately dominate the behavior of a system and cause sudden breakdowns. ("Going out with a bang," an instance of optimization daemons.)
I think these are the most important problems if we fail to solve intent alignment.
In practice these problems will interact with each other, and with other disruptions/instability caused by rapid progress. These problems are worse in worlds where progress is relatively fast, and fast takeoff can be a key risk factor, but I’m scared even if we have several years.
With fast enough takeoff, my expectations start to look more like the caricature---this post envisions reasonably broad deployment of AI, which becomes less and less likely as things get faster. I think the basic problems are still essentially the same though, just occurring within an AI lab rather than across the world.
(None of the concerns in this post are novel.)
Part I: You get what you measure
If I want to convince Bob to vote for Alice, I can experiment with many different persuasion strategies and see which ones work. Or I can build good predictive models of Bob’s behavior and then search for actions that will lead him to vote for Alice. These are powerful techniques for achieving any goal that can be easily measured over short time periods.
But if I want to help Bob figure out whether he should vote for Alice---whether voting for Alice would ultimately help create the kind of society he wants---that can’t be done by trial and error. To solve such tasks we need to understand what we are doing and why it will yield good outcomes. We still need to use data in order to improve over time, but we need to understand how to update on new data in order to improve.
Some examples of easy-to-measure vs. hard-to-measure goals:
Persuading me, vs. helping me figure out what’s true. (Thanks to Wei Dai for making this example crisp.)
Reducing my feeling of uncertainty, vs. increasing my knowledge about the world.
Improving my reported life satisfaction, vs. actually helping me live a good life.
Reducing reported crimes, vs. actually preventing crime.
Increasing my wealth on paper, vs. increasing my effective control over resources.
It’s already much easier to pursue easy-to-measure goals, but machine learning will widen the gap by letting us try a huge number of possible strategies and search over massive spaces of possible actions. That force will combine with and amplify existing institutional and social dynamics that already favor easily-measured goals.
Right now humans thinking and talking about the future they want to create are a powerful force that is able to steer our trajectory. But over time human reasoning will become weaker and weaker compared to new forms of reasoning honed by trial-and-error. Eventually our society’s trajectory will be determined by powerful optimization with easily-measurable goals rather than by human intentions about the future.
We will try to harness this power by constructing proxies for what we care about, but over time those proxies will come apart:
Corporations will deliver value to consumers as measured by profit. Eventually th...
Welcome to The Nonlinear Library, where we use Text-to-Speech software to convert the best writing from the Rationalist and EA communities into audio.
This is: The Parable of Predict-O-Matic, published by Abram Demski on the AI Alignment Forum.
I've been thinking more about partial agency. I want to expand on some issues brought up in the comments to my previous post, and on other complications which I've been thinking about. But for now, a more informal parable. (Mainly because this is easier to write than my more technical thoughts.)
This relates to oracle AI and to inner optimizers, but my focus is a little different.
1
Suppose you are designing a new invention, a predict-o-matic. It is a wonderous machine which will predict everything for us: weather, politics, the newest advances in quantum physics, you name it. The machine isn't infallible, but it will integrate data across a wide range of domains, automatically keeping itself up-to-date with all areas of science and current events. You fully expect that once your product goes live, it will become a household utility, replacing services like Google. (Google only lets you search the known!)
Things are going well. You've got investors. You have an office and a staff. These days, it hardly even feels like a start-up any more; progress is going well.
One day, an intern raises a concern.
"If everyone is going to be using Predict-O-Matic, we can't think of it as a passive observer. Its answers will shape events. If it says stocks will rise, they'll rise. If it says stocks will fall, then fall they will. Many people will vote based on its predictions."
"Yes," you say, "but Predict-O-Matic is an impartial observer nonetheless. It will answer people's questions as best it can, and they react however they will."
"But --" the intern objects -- "Predict-O-Matic will see those possible reactions. It knows it could give several different valid predictions, and different predictions result in different futures. It has to decide which one to give somehow."
You tap on your desk in thought for a few seconds. "That's true. But we can still keep it objective. It could pick randomly."
"Randomly? But some of these will be huge issues! Companies -- no, nations -- will one day rise or fall based on the word of Predict-O-Matic. When Predict-O-Matic is making a prediction, it is choosing a future for us. We can't leave that to a coin flip! We have to select the prediction which results in the best overall future. Forget being an impassive observer! We need to teach Predict-O-Matic human values!"
You think about this. The thought of Predict-O-Matic deliberately steering the future sends a shudder down your spine. But what alternative do you have? The intern isn't suggesting Predict-O-Matic should lie, or bend the truth in any way -- it answers 100% honestly to the best of its ability. But (you realize with a sinking feeling) honesty still leaves a lot of wiggle room, and the consequences of wiggles could be huge.
After a long silence, you meet the interns eyes. "Look. People have to trust Predict-O-Matic. And I don't just mean they have to believe Predict-O-Matic. They're bringing this thing into their homes. They have to trust that Predict-O-Matic is something they should be listening to. We can't build value judgements into this thing! If it ever came out that we had coded a value function into Predict-O-Matic, a value function which selected the very future itself by selecting which predictions to make -- we'd be done for! No matter how honest Predict-O-Matic remained, it would be seen as a manipulator. No matter how beneficent its guiding hand, there are always compromises, downsides, questionable calls. No matter how careful we were to set up its values -- to make them moral, to make them humanitarian, to make them politically correct and broadly appealing -- who are we to choose? No. We'd be done for. They'd hang us. We'd be toast!"
You realize at this point that you've stood up and start...
Welcome to The Nonlinear Library, where we use Text-to-Speech software to convert the best writing from the Rationalist and EA communities into audio.
This is: What 2026 looks like, published by Daniel Kokotajlo on the AI Alignment Forum.
This was written for the Vignettes Workshop.[1] The goal is to write out a detailed future history (“trajectory”) that is as realistic (to me) as I can currently manage, i.e. I’m not aware of any alternative trajectory that is similarly detailed and clearly more plausible to me. The methodology is roughly: Write a future history of 2022. Condition on it, and write a future history of 2023. Repeat for 2024, 2025, etc. (I'm posting 2022-2026 now so I can get feedback that will help me write 2027+. I intend to keep writing until the story reaches singularity/extinction/utopia/etc.)
What’s the point of doing this? Well, there are a couple of reasons:
Sometimes attempting to write down a concrete example causes you to learn things, e.g. that a possibility is more or less plausible than you thought.
Most serious conversation about the future takes place at a high level of abstraction, talking about e.g. GDP acceleration, timelines until TAI is affordable, multipolar vs. unipolar takeoff. vignettes are a neglected complementary approach worth exploring.
Most stories are written backwards. The author begins with some idea of how it will end, and arranges the story to achieve that ending. Reality, by contrast, proceeds from past to future. It isn’t trying to entertain anyone or prove a point in an argument.
Anecdotally, various people seem to have found Paul Christiano’s “tales of doom” stories helpful, and relative to typical discussions those stories are quite close to what we want. (I still think a bit more detail would be good — e.g. Paul’s stories don’t give dates, or durations, or any numbers at all really.)[2]
“I want someone to ... write a trajectory for how AI goes down, that is really specific about what the world GDP is in every one of the years from now until insane intelligence explosion. And just write down what the world is like in each of those years because I don't know how to write an internally consistent, plausible trajectory. I don't know how to write even one of those for anything except a ridiculously fast takeoff.” --Buck Shlegeris
This vignette was hard to write. To achieve the desired level of detail I had to make a bunch of stuff up, but in order to be realistic I had to constantly ask “but actually though, what would really happen in this situation?” which made it painfully obvious how little I know about the future. There are numerous points where I had to conclude “Well, this does seem implausible, but I can’t think of anything more plausible at the moment and I need to move on.” I fully expect the actual world to diverge quickly from the trajectory laid out here. Let anyone who (with the benefit of hindsight) claims this divergence as evidence against my judgment prove it by exhibiting a vignette/trajectory they themselves wrote in 2021. If it maintains a similar level of detail (and thus sticks its neck out just as much) while being more accurate, I bow deeply in respect!
I hope this inspires other people to write more vignettes soon. We at the Center on Long-Term Risk would like to have a collection to use for strategy discussions. Let me know if you’d like to do this, and I can give you advice & encouragement! I’d be happy to run another workshop.
2022
GPT-3 is finally obsolete. OpenAI, Google, Facebook, and DeepMind all have gigantic multimodal transformers, similar in size to GPT-3 but trained on images, video, maybe audio too, and generally higher-quality data.
Not only that, but they are now typically fine-tuned in various ways--for example, to answer questions correctly, or produce engaging conversation as a chatbot.
The chatbots are fun to talk to but erratic and ultimately considered shallow by intellectuals. They aren’t particularly useful for anything supe...
Welcome to The Nonlinear Library, where we use Text-to-Speech software to convert the best writing from the Rationalist and EA communities into audio.
This is: Are we in an AI overhang?, published by Andy Jones on the AI Alignment Forum.
Over on Developmental Stages of GPTs, orthonormal mentions
it at least reduces the chance of a hardware overhang.
An overhang is when you have had the ability to build transformative AI for quite some time, but you haven't because no-one's realised it's possible. Then someone does and surprise! It's a lot more capable than everyone expected.
I am worried we're in an overhang right now. I think we right now have the ability to build an orders-of-magnitude more powerful system than we already have, and I think GPT-3 is the trigger for 100x larger projects at Google, Facebook and the like, with timelines measured in months.
Investment Bounds
GPT-3 is the first AI system that has obvious, immediate, transformative economic value. While much hay has been made about how much more expensive it is than a typical AI research project, in the wider context of megacorp investment, its costs are insignificant.
GPT-3 has been estimated to cost $5m in compute to train, and - looking at the author list and OpenAI's overall size - maybe another $10m in labour.
Google, Amazon and Microsoft each spend about $20bn/year on R&D and another $20bn each on capital expenditure. Very roughly, it totals to $100bn/year. Against this budget, dropping $1bn or more on scaling GPT up by another factor of 100x is entirely plausible right now. All that's necessary is that tech executives stop thinking of natural language processing as cutesy blue-sky research and start thinking in terms of quarters-till-profitability.
A concrete example is Waymo, which is raising $2bn investment rounds - and that's for a technology with a much longer road to market.
Compute Cost
The other side of the equation is compute cost. The $5m GPT-3 training cost estimate comes from using V100s at $10k/unit and 30 TFLOPS, which is the performance without tensor cores being considered. Amortized over a year, this gives you about $1000/PFLOPS-day.
However, this cost is driven up an order of magnitude by NVIDIA's monopolistic cloud contracts, while performance will be higher when taking tensor cores into account. The current hardware floor is nearer to the RTX 2080 TI's $1k/unit for 125 tensor-core TFLOPS, and that gives you $25/PFLOPS-day. This roughly aligns with AI Impacts’ current estimates, and offers another >10x speedup to our model.
I strongly suspect other bottlenecks stop you from hitting that kind of efficiency or GPT-3 would've happened much sooner, but I still think $25/PFLOPS-day is a lower useful bound.
Other Constraints
I've focused on money so far because most of the current 3.5-month doubling times come from increasing investment. But money aside, there are a couple of other things that could prove to be the binding constraint.
Scaling law breakdown. The GPT series' scaling is expected to break down around 10k pflops-days (§6.3), which is a long way short of the amount of cash on the table.
This could be because the scaling analysis was done on 1024-token sequences. Maybe longer sequences can go further. More likely I'm misunderstanding something.
Sequence length. GPT-3 uses 2048 tokens at a time, and that's with an efficient encoding that cripples it on many tasks. With the naive architecture, increasing the sequence length is quadratically expensive, and getting up to novel-length sequences is not very likely.
But there are a lot of plausible ways to fix that, and complexity is no bar AI. This constraint might plausibly not be resolved on a timescale of months, however.
Data availability. From the same paper as the previous point, dataset size rises with the square-root of compute; a 1000x larger GPT-3 would want 10 trillion tokens of training data.
It’s hard to find a good estimate on total-words-ever-written, but our library of 130m...
Welcome to The Nonlinear Library, where we use Text-to-Speech software to convert the best writing from the Rationalist and EA communities into audio.
This is: DeepMind: Generally capable agents emerge from open-ended play, published by Daniel Kokotajlo on the AI Alignment Forum.
This is a linkpost for
EDIT: Also see paper and results compilation video!
Today, we published "Open-Ended Learning Leads to Generally Capable Agents," a preprint detailing our first steps to train an agent capable of playing many different games without needing human interaction data. ... The result is an agent with the ability to succeed at a wide spectrum of tasks — from simple object-finding problems to complex games like hide and seek and capture the flag, which were not encountered during training. We find the agent exhibits general, heuristic behaviours such as experimentation, behaviours that are widely applicable to many tasks rather than specialised to an individual task.
The neural network architecture we use provides an attention mechanism over the agent’s internal recurrent state — helping guide the agent’s attention with estimates of subgoals unique to the game the agent is playing. We’ve found this goal-attentive agent (GOAT) learns more generally capable policies.
Playing roughly 700,000 unique games in 4,000 unique worlds within XLand, each agent in the final generation experienced 200 billion training steps as a result of 3.4 million unique tasks. At this time, our agents have been able to participate in every procedurally generated evaluation task except for a handful that were impossible even for a human. And the results we’re seeing clearly exhibit general, zero-shot behaviour across the task space — with the frontier of normalised score percentiles continually improving.
Looking qualitatively at our agents, we often see general, heuristic behaviours emerge — rather than highly optimised, specific behaviours for individual tasks. Instead of agents knowing exactly the “best thing” to do in a new situation, we see evidence of agents experimenting and changing the state of the world until they’ve achieved a rewarding state. We also see agents rely on the use of other tools, including objects to occlude visibility, to create ramps, and to retrieve other objects. Because the environment is multiplayer, we can examine the progression of agent behaviours while training on held-out social dilemmas, such as in a game of “chicken”. As training progresses, our agents appear to exhibit more cooperative behaviour when playing with a copy of themselves. Given the nature of the environment, it is difficult to pinpoint intentionality — the behaviours we see often appear to be accidental, but still we see them occur consistently.
My hot take: This seems like a somewhat big deal to me. It's what I would have predicted, but that's scary, given my timelines. I haven't read the paper itself yet but I look forward to seeing more numbers and scaling trends and attempting to extrapolate... When I do I'll leave a comment with my thoughts.
EDIT: My warm take: The details in the paper back up the claims it makes in the title and abstract. This is the GPT-1 of agent/goal-directed AGI; it is the proof of concept. Two more papers down the line (and a few OOMs more compute), and we'll have the agent/goal-directed AGI equivalent of GPT-3. Scary stuff.
Thanks for listening. To help us out with The Nonlinear Library or to learn more, please visit nonlinear.org.
Welcome to The Nonlinear Library, where we use Text-to-Speech software to convert the best writing from the Rationalist and EA communities into audio.
This is: Alignment Research Field Guide, published by Abram Demski on the AI Alignment Forum.
This field guide was written by the MIRI team with MIRIx groups in mind, though the advice may be relevant to others working on AI alignment research.
Preamble I: Decision Theory
Hello! You may notice that you are reading a document.
This fact comes with certain implications. For instance, why are you reading this? Will you finish it? What decisions will you come to as a result? What will you do next?
Notice that, whatever you end up doing, it’s likely that there are dozens or even hundreds of other people, quite similar to you and in quite similar positions, who will follow reasoning which strongly resembles yours, and make choices which correspondingly match.
Given that, it’s our recommendation that you make your next few decisions by asking the question “What policy, if followed by all agents similar to me, would result in the most good, and what does that policy suggest in my particular case?” It’s less of a question of trying to decide for all agents sufficiently-similar-to-you (which might cause you to make the wrong choice out of guilt or pressure) and more something like “if I were in charge of all agents in my reference class, how would I treat instances of that class with my specific characteristics?”
If that kind of thinking leads you to read further, great. If it leads you to set up a MIRIx chapter, even better. In the meantime, we will proceed as if the only people reading this document are those who justifiably expect to find it reasonably useful.
⠀
Preamble II: Surface Area
Imagine that you have been tasked with moving a cube of solid iron that is one meter on a side. Given that such a cube weighs ~16000 pounds, and that an average human can lift ~100 pounds, a naïve estimation tells you that you can solve this problem with ~150 willing friends.
But of course, a meter cube can fit at most something like 10 people around it. It doesn’t matter if you have the theoretical power to move the cube if you can’t bring that power to bear in an effective manner. The problem is constrained by its surface area.
MIRIx chapters are one of the best ways to increase the surface area of people thinking about and working on the technical problem of AI alignment. And just as it would be a bad idea to decree "the 10 people who happen to currently be closest to the metal cube are the only ones allowed to think about how to think about this problem", we don’t want MIRI to become the bottleneck or authority on what kinds of thinking can and should be done in the realm of embedded agency and other relevant fields of research.
The hope is that you and others like you will help actually solve the problem, not just follow directions or read what’s already been written. This document is designed to support people who are interested in doing real groundbreaking research themselves.
⠀
Contents
You and your research
Logistics of getting started
Models of social dynamics
Other useful thoughts and questions
⠀
1. You and your research
We sometimes hear questions of the form “Even a summer internship feels too short to make meaningful progress on real problems. How can anyone expect to meet and do real research in a single afternoon?”
There’s a Zeno-esque sense in which you can’t make research progress in a million years if you can’t also do it in five minutes. It’s easy to fall into a trap of (either implicitly or explicitly) conceptualizing “research” as “first studying and learning what’s already been figured out, and then attempting to push the boundaries and contribute new content.”
The problem with this frame (according to us) is that it leads people to optimize for absorbing information, rather than seeking it instrumentally, as a precursor to understanding. (Be mindful of what you’re optimizing ...
Welcome to The Nonlinear Library, where we use Text-to-Speech software to convert the best writing from the Rationalist and EA communities into audio.
This is: Hiring engineers and researchers to help align GPT-3, published by Paul Christiano on the AI Alignment Forum.
My team at OpenAI, which works on aligning GPT-3, is hiring ML engineers and researchers. Apply here for the ML engineer role and here for the ML researcher role.
GPT-3 is similar enough to "prosaic" AGI that we can work on key alignment problems without relying on conjecture or speculative analogies. And because GPT-3 is already being deployed in the OpenAI API, its misalignment matters to OpenAI’s bottom line — it would be much better if we had an API that was trying to help the user instead of trying to predict the next word of text from the internet.
I think this puts our team in a great place to have an impact:
If our research succeeds I think it will directly reduce existential risk from AI. This is not meant to be a warm-up problem, I think it’s the real thing.
We are working with state of the art systems that could pose an existential risk if scaled up, and our team’s success actually matters to the people deploying those systems.
We are working on the whole pipeline from “interesting idea” to “production-ready system,” building critical skills and getting empirical feedback on whether our ideas actually work.
We have the real-world problems to motivate alignment research, the financial support to hire more people, and a research vision to execute on. We are bottlenecked by excellent researchers and engineers who are excited to work on alignment.
What the team does
In the past Reflection focused on fine-tuning GPT-3 using a reward function learned from human feedback. Our most recent results are here, and had the unusual virtue of simultaneously being exciting enough to ML researchers to be accepted at NeurIPS while being described by Eliezer as “directly, straight-up relevant to real alignment problems.”
We’re currently working on three things:
[20%] Applying basic alignment approaches to the API, aiming to close the gap between theory and practice.
[60%] Extending existing approaches to tasks that are too hard for humans to evaluate; in particular, we are training models that summarize more text than human trainers have time to read. Our approach is to use weaker ML systems operating over shorter contexts to help oversee stronger ones over longer contexts. This is conceptually straightforward but still poses significant engineering and ML challenges.
[20%] Conceptual research on domains that no one knows how to oversee and empirical work on debates between humans (see our 2019 writeup). I think the biggest open problem is figuring out how and if human overseers can leverage “knowledge” the model acquired during training (see an example here).
If successful, ideas will eventually move up this list, from the conceptual stage to ML prototypes to real deployments. We’re viewing this as practice for integrating alignment into transformative AI deployed by OpenAI or another organization.
What you’d do
Most people on the team do a subset of these core tasks:
Design+build+maintain code for experimenting with novel training strategies for large language models. This infrastructure needs to support a diversity of experimental changes that are hard to anticipate in advance, work as a solid base to build on for 6-12 months, and handle the complexity of working with large language models. Most of our code is maintained by 1-3 people and consumed by 2-4 people (all on the team).
Oversee ML training. Evaluate how well models are learning, figure out why they are learning badly, and identify+prioritize+implement changes to make them learn better. Tune hyperparameters and manage computing resources. Process datasets for machine consumption; understand datasets and how they affect the model’s behavior.
Design and conduct experiments to answer questions about our mode...
Welcome to The Nonlinear Library, where we use Text-to-Speech software to convert the best writing from the Rationalist and EA communities into audio.
This is: 2018 AI Alignment Literature Review and Charity Comparison, published by Larks on the AI Alignment Forum.
Cross-posted to the EA forum.
Introduction
Like last year and the year before, I’ve attempted to review the research that has been produced by various organisations working on AI safety, to help potential donors gain a better understanding of the landscape. This is a similar role to that which GiveWell performs for global health charities, and somewhat similar to an securities analyst with regards to possible investments. It appears that once again no-one else has attempted to do this, to my knowledge, so I've once again undertaken the task.
This year I have included several groups not covered in previous years, and read more widely in the literature.
My aim is basically to judge the output of each organisation in 2018 and compare it to their budget. This should give a sense for the organisations' average cost-effectiveness. We can also compare their financial reserves to their 2019 budgets to get a sense of urgency.
Note that this document is quite long, so I encourage you to just read the sections that seem most relevant to your interests, probably the sections about the individual organisations. I do not recommend you skip to the conclusions!
I’d like to apologize in advance to everyone doing useful AI Safety work whose contributions I may have overlooked or misconstrued.
Methodological Considerations
Track Records
Judging organisations on their historical output is naturally going to favour more mature organisations. A new startup, whose value all lies in the future, will be disadvantaged. However, I think that this is correct. The newer the organisation, the more funding should come from people with close knowledge. As organisations mature, and have more easily verifiable signals of quality, their funding sources can transition to larger pools of less expert money. This is how it works for startups turning into public companies and I think the same model applies here.
This judgement involves analysing a large number papers relating to Xrisk that were produced during 2018. Hopefully the year-to-year volatility of output is sufficiently low that this is a reasonable metric. I also attempted to include papers during December 2017, to take into account the fact that I'm missing the last month's worth of output from 2017, but I can't be sure I did this successfully.
This article focuses on AI risk work. If you think other causes are important too, your priorities might differ. This particularly affects GCRI, FHI and CSER, who both do a lot of work on other issues.
We focus on papers, rather than outreach or other activities. This is partly because they are much easier to measure; while there has been a large increase in interest in AI safety over the last year, it’s hard to work out who to credit for this, and partly because I think progress has to come by persuading AI researchers, which I think comes through technical outreach and publishing good work, not popular/political work.
Politics
My impression is that policy on technical subjects (as opposed to issues that attract strong views from the general population) is generally made by the government and civil servants in consultation with, and being lobbied by, outside experts and interests. Without expert (e.g. top ML researchers at Google, CMU & Baidu) consensus, no useful policy will be enacted. Pushing directly for policy seems if anything likely to hinder expert consensus. Attempts to directly influence the government to regulate AI research seem very adversarial, and risk being pattern-matched to ignorant opposition to GM foods or nuclear power. We don't want the 'us-vs-them' situation, that has occurred with climate change, to happen here. AI researchers who are dismissive of safety law, regarding it as ...
Welcome to The Nonlinear Library, where we use Text-to-Speech software to convert the best writing from the Rationalist and EA communities into audio.
This is: Another (outer) alignment failure story, published by Paul Christiano on the AI Alignment Forum.
Meta
This is a story where the alignment problem is somewhat harder than I expect, society handles AI more competently than I expect, and the outcome is worse than I expect. It also involves inner alignment turning out to be a surprisingly small problem. Maybe the story is 10-20th percentile on each of those axes. At the end I’m going to go through some salient ways you could vary the story.
This isn’t intended to be a particularly great story (and it’s pretty informal). I’m still trying to think through what I expect to happen if alignment turns out to be hard, and this more like the most recent entry in a long journey of gradually-improving stories.
I wrote this up a few months ago and was reminded to post it by Critch’s recent post (which is similar in many ways). This story has definitely been shaped by a broader community of people gradually refining failure stories rather than being written in a vacuum.
I’d like to continue spending time poking at aspects of this story that don’t make sense, digging into parts that seem worth digging into, and eventually developing clearer and more plausible stories. I still think it’s very plausible that my views about alignment will change in the course of thinking concretely about stories, and even if my basic views about alignment stay the same it’s pretty likely that the story will change.
Story
ML starts running factories, warehouses, shipping, and construction. ML assistants help write code and integrate ML into new domains. ML designers help build factories and the robots that go in them. ML finance systems invest in companies on the basis of complicated forecasts and (ML-generated) audits. Tons of new factories, warehouses, power plants, trucks and roads are being built. Things are happening quickly, investors have super strong FOMO, no one really knows whether it’s a bubble but they can tell that e.g. huge solar farms are getting built and something is happening that they want a piece of. Defense contractors are using ML systems to design new drones, and ML is helping the DoD decide what to buy and how to deploy it. The expectation is that automated systems will manage drones during high-speed ML-on-ML conflicts because humans won’t be able to understand what’s going on. ML systems are designing new ML systems, testing variations, commissioning giant clusters. The financing is coming from automated systems, the clusters are built by robots. A new generation of fabs is being built with unprecedented speed using new automation.
At this point everything kind of makes sense to humans. It feels like we are living at the most exciting time in history. People are making tons of money. The US defense establishment is scared because it has no idea what a war is going to look like right now, but in terms of policy their top priority is making sure the boom proceeds as quickly in the US as it does in China because it now seems plausible that being even a few years behind would result in national irrelevance.
Things are moving very quickly and getting increasingly hard for humans to evaluate. We can no longer train systems to make factory designs that look good to humans, because we don’t actually understand exactly what robots are doing in those factories or why; we can’t evaluate the tradeoffs between quality and robustness and cost that are being made; we can't really understand the constraints on a proposed robot design or why one design is better than another. We can’t evaluate arguments about investments very well because they come down to claims about where the overall economy is going over the next 6 months that seem kind of alien (even the more recognizable claims are just kind of incomprehensible predictions about e.g. how t...
Welcome to The Nonlinear Library, where we use Text-to-Speech software to convert the best writing from the Rationalist and EA communities into audio.
This is: Debate on Instrumental Convergence between LeCun, Russell, Bengio, Zador, and More , published by Ben Pace on the AI Alignment Forum.
An actual debate about instrumental convergence, in a public space! Major respect to all involved, especially Yoshua Bengio for great facilitation.
For posterity (i.e. having a good historical archive) and further discussion, I've reproduced the conversation here. I'm happy to make edits at the request of anyone in the discussion who is quoted below. I've improved formatting for clarity and fixed some typos. For people who are not researchers in this area who wish to comment, see the public version of this post here. For people who do work on the relevant areas, please sign up in the top right. It will take a day or so to confirm membership.
Original Post
Yann LeCun: "don't fear the Terminator", a short opinion piece by Tony Zador and me that was just published in Scientific American.
"We dramatically overestimate the threat of an accidental AI takeover, because we tend to conflate intelligence with the drive to achieve dominance. [...] But intelligence per se does not generate the drive for domination, any more than horns do."
Comment Thread #1
Elliot Olds: Yann, the smart people who are very worried about AI seeking power and ensuring its own survival believe it's a big risk because power and survival are instrumental goals for almost any ultimate goal.
If you give a generally intelligent AI the goal to make as much money in the stock market as possible, it will resist being shut down because that would interfere with tis goal. It would try to become more powerful because then it could make money more effectively. This is the natural consequence of giving a smart agent a goal, unless we do something special to counteract this.
You've often written about how we shouldn't be so worried about AI, but I've never seen you address this point directly.
Stuart Russell: It is trivial to construct a toy MDP in which the agent's only reward comes from fetching the coffee. If, in that MDP, there is another "human" who has some probability, however small, of switching the agent off, and if the agent has available a button that switches off that human, the agent will necessarily press that button as part of the optimal solution for fetching the coffee. No hatred, no desire for power, no built-in emotions, no built-in survival instinct, nothing except the desire to fetch the coffee successfully. This point cannot be addressed because it's a simple mathematical observation.
Comment Thread #2
Yoshua Bengio: Yann, I'd be curious about your response to Stuart Russell's point.
Yann LeCun: You mean, the so-called "instrumental convergence" argument by which "a robot can't fetch you coffee if it's dead. Hence it will develop self-preservation as an instrumental sub-goal."
It might even kill you if you get in the way.
1. Once the robot has brought you coffee, its self-preservation instinct disappears. You can turn it off.
2. One would have to be unbelievably stupid to build open-ended objectives in a super-intelligent (and super-powerful) machine without some safeguard terms in the objective.
3. One would have to be rather incompetent not to have a mechanism by which new terms in the objective could be added to prevent previously-unforeseen bad behavior. For humans, we have education and laws to shape our objective functions and complement the hardwired terms built into us by evolution.
4. The power of even the most super-intelligent machine is limited by physics, and its size and needs make it vulnerable to physical attacks. No need for much intelligence here. A virus is infinitely less intelligent than you, but it can still kill you.
5. A second machine, designed solely to neutralize an evil super-intelligent machine will win every time, if given similar...
Welcome to The Nonlinear Library, where we use Text-to-Speech software to convert the best writing from the Rationalist and EA communities into audio.
This is: Some AI research areas and their relevance to existential safety, published by Andrew Critch on the AI Alignment Forum.
Followed by: What Multipolar Failure Looks Like, and Robust Agent-Agnostic Processes (RAAPs), which provides examples of multi-stakeholder/multi-agent interactions leading to extinction events.
Introduction
This post is an overview of a variety of AI research areas in terms of how much I think contributing to and/or learning from those areas might help reduce AI x-risk. By research areas I mean “AI research topics that already have groups of people working on them and writing up their results”, as opposed to research “directions” in which I’d like to see these areas “move”.
I formed these views mostly pursuant to writing AI Research Considerations for Human Existential Safety (ARCHES). My hope is that my assessments in this post can be helpful to students and established AI researchers who are thinking about shifting into new research areas specifically with the goal of contributing to existential safety somehow. In these assessments, I find it important to distinguish between the following types of value:
The helpfulness of the area to existential safety, which I think of as a function of what services are likely to be provided as a result of research contributions to the area, and whether those services will be helpful to existential safety, versus
The educational value of the area for thinking about existential safety, which I think of as a function of how much a researcher motivated by existential safety might become more effective through the process of familiarizing with or contributing to that area, usually by focusing on ways the area could be used in service of existential safety.
The neglect of the area at various times, which is a function of how much technical progress has been made in the area relative to how much I think is needed.
Importantly:
The helpfulness to existential safety scores do not assume that your contributions to this area would be used only for projects with existential safety as their mission. This can negatively impact the helpfulness of contributing to areas that are more likely to be used in ways that harm existential safety.
The educational value scores are not about the value of an existential-safety-motivated researcher teaching about the topic, but rather, learning about the topic.
The neglect scores are not measuring whether there is enough “buzz” around the topic, but rather, whether there has been adequate technical progress in it. Buzz can predict future technical progress, though, by causing people to work on it.
Below is a table of all the areas I considered for this post, along with their entirely subjective “scores” I’ve given them. The rest of this post can be viewed simply as an elaboration/explanation of this table:
Existing Research Area
Social Application
Helpfulness to Existential Safety
Educational Value
2015 Neglect
2020 Neglect
2030 Neglect
Out of Distribution Robustness
Zero/
Single
1/10
4/10
5/10
3/10
1/10
Agent Foundations
Zero/
Single
3/10
8/10
9/10
8/10
7/10
Multi-agent RL
Zero/
Multi
2/10
6/10
5/10
4/10
0/10
Preference Learning
Single/
Single
1/10
4/10
5/10
1/10
0/10
Side-effect Minimization
Single/
Single
4/10
4/10
6/10
5/10
4/10
Human-Robot Interaction
Single/
Single
6/10
7/10
5/10
4/10
3/10
Interpretability in ML
Single/
Single
8/10
6/10
8/10
6/10
2/10
Fairness in ML
Multi/
Single
6/10
5/10
7/10
3/10
2/10
Computational Social Choice
Multi/
Single
7/10
7/10
7/10
5/10
4/10
Accountability in ML
Multi/
Multi
8/10
3/10
8/10
7/10
5/10
The research areas are ordered from least-socially-complex to most-socially-complex. This roughly (though imperfectly) correlates with addressing existential safety problems of increasing importance and neglect, according to me. Correspondingly, the second colu...
Welcome to The Nonlinear Library, where we use Text-to-Speech software to convert the best writing from the Rationalist and EA communities into audio.
This is: Announcing the Alignment Research Center, published by Paul Christiano on the AI Alignment Forum.
(Cross-post from ai-alignment.com)
I’m now working full-time on the Alignment Research Center (ARC), a new non-profit focused on intent alignment research.
I left OpenAI at the end of January and I’ve spent the last few months planning, doing some theoretical research, doing some logistical set-up, and taking time off.
For now it’s just me, focusing on theoretical research. I’m currently feeling pretty optimistic about this work: I think there’s a good chance that it will yield big alignment improvements within the next few years, and a good chance that those improvements will be integrated into practice at leading ML labs.
My current goal is to build a small team working productively on theory. I’m not yet sure how we’ll approach hiring, but if you’re potentially interested in joining you can fill out this tiny form to get notified when we’re ready.
Over the medium term (and maybe starting quite soon) I also expect to implement and study techniques that emerge from theoretical work, to help ML labs adopt alignment techniques, and to work on alignment forecasting and strategy.
Thanks for listening. To help us out with The Nonlinear Library or to learn more, please visit nonlinear.org.
Welcome to The Nonlinear Library, where we use Text-to-Speech software to convert the best writing from the Rationalist and EA communities into audio.
This is: The Rocket Alignment Problem, published by Eliezer Yudkowsky on the AI Alignment Forum.
The following is a fictional dialogue building off of AI Alignment: Why It’s Hard, and Where to Start.
(Somewhere in a not-very-near neighboring world, where science took a very different course.)
ALFONSO: Hello, Beth. I’ve noticed a lot of speculations lately about “spaceplanes” being used to attack cities, or possibly becoming infused with malevolent spirits that inhabit the celestial realms so that they turn on their own engineers.
I’m rather skeptical of these speculations. Indeed, I’m a bit skeptical that airplanes will be able to even rise as high as stratospheric weather balloons anytime in the next century. But I understand that your institute wants to address the potential problem of malevolent or dangerous spaceplanes, and that you think this is an important present-day cause.
BETH: That’s. really not how we at the Mathematics of Intentional Rocketry Institute would phrase things.
The problem of malevolent celestial spirits is what all the news articles are focusing on, but we think the real problem is something entirely different. We’re worried that there’s a difficult, theoretically challenging problem which modern-day rocket punditry is mostly overlooking. We’re worried that if you aim a rocket at where the Moon is in the sky, and press the launch button, the rocket may not actually end up at the Moon.
ALFONSO: I understand that it’s very important to design fins that can stabilize a spaceplane’s flight in heavy winds. That’s important spaceplane safety research and someone needs to do it.
But if you were working on that sort of safety research, I’d expect you to be collaborating tightly with modern airplane engineers to test out your fin designs, to demonstrate that they are actually useful.
BETH: Aerodynamic designs are important features of any safe rocket, and we’re quite glad that rocket scientists are working on these problems and taking safety seriously. That’s not the sort of problem that we at MIRI focus on, though.
ALFONSO: What’s the concern, then? Do you fear that spaceplanes may be developed by ill-intentioned people?
BETH: That’s not the failure mode we’re worried about right now. We’re more worried that right now, nobody can tell you how to point your rocket’s nose such that it goes to the moon, nor indeed any prespecified celestial destination. Whether Google or the US Government or North Korea is the one to launch the rocket won’t make a pragmatic difference to the probability of a successful Moon landing from our perspective, because right now nobody knows how to aim any kind of rocket anywhere.
ALFONSO: I’m not sure I understand.
BETH: We’re worried that even if you aim a rocket at the Moon, such that the nose of the rocket is clearly lined up with the Moon in the sky, the rocket won’t go to the Moon. We’re not sure what a realistic path from the Earth to the moon looks like, but we suspect it might not be a very straight path, and it may not involve pointing the nose of the rocket at the moon at all. We think the most important thing to do next is to advance our understanding of rocket trajectories until we have a better, deeper understanding of what we’ve started calling the “rocket alignment problem”. There are other safety problems, but this rocket alignment problem will probably take the most total time to work on, so it’s the most urgent.
ALFONSO: Hmm, that sounds like a bold claim to me. Do you have a reason to think that there are invisible barriers between here and the moon that the spaceplane might hit? Are you saying that it might get very very windy between here and the moon, more so than on Earth? Both eventualities could be worth preparing for, I suppose, but neither seem likely.
BETH: We don’t think it’s particularly likely that there...
I wrote this post to get people’s takes on a type of work that seems exciting to me personally; I’m not speaking for Open Phil as a whole. Institutionally, we are very uncertain whether to prioritize this (and if we do where it should be housed and how our giving should be structured). We are not seeking grant applications on this topic right now.
Thanks to Daniel Dewey, Eliezer Yudkowsky, Evan Hubinger, Holden Karnofsky, Jared Kaplan, Mike Levine, Nick Beckstead, Owen Cotton-Barratt, Paul Christiano, Rob Bensinger, and Rohin Shah for comments on earlier drafts.
A genre of technical AI risk reduction work that seems exciting to me is trying to align existing models that already are, or have the potential to be, “superhuman”[1] at some particular task (which I’ll call narrowly superhuman models).[2] I don’t just mean “train these models to be more robust, reliable, interpretable, etc” (though that seems good too); I mean “figure out how to harness their full abilities so they can be as useful as possible to humans” (focusing on “fuzzy” domains where it’s intuitively non-obvious how to make that happen).
Here’s an example of what I’m thinking of: intuitively speaking, it feels like GPT-3 is “smart enough to” (say) give advice about what to do if I’m sick that’s better than advice I’d get from asking humans on Reddit or Facebook, because it’s digested a vast store of knowledge about illness symptoms and remedies. Moreover, certain ways of prompting it provide suggestive evidence that it could use this knowledge to give helpful advice. With respect to the Reddit or Facebook users I might otherwise ask, it seems like GPT-3 has the potential to be narrowly superhuman in the domain of health advice.
But GPT-3 doesn’t seem to “want” to give me the best possible health advice -- instead it “wants” to play a strange improv game riffing off the prompt I give it, pretending it’s a random internet user. So if I want to use GPT-3 to get advice about my health, there is a gap between what it’s capable of (which could even exceed humans) and what I can get it to actually provide me. I’m interested in the challenge of:
How can we get GPT-3 to give “the best health advice it can give” when humans[3] in some sense “understand less” about what to do when you’re sick than GPT-3 does? And in that regime, how can we even tell whether it’s actually “doing the best it can”?
I think there are other similar challenges we could define for existing models, especially large language models.
I’m excited about tackling this particular type of near-term challenge because it feels like a microcosm of the long-term AI alignment problem in a real, non-superficial sense. In the end, we probably want to find ways to meaningfully supervise (or justifiably trust) models that are more capable than ~all humans in ~all domains.[4] So it seems like a promising form of practice to figure out how to get particular humans to oversee models that are more capable than them in specific ways, if this is done with an eye to developing scalable and domain-general techniques.
I’ll call this type of project aligning narrowly superhuman models. In the rest of this post, I:
Give a more detailed description of what aligning narrowly superhuman models could look like, what does and doesn’t “count”, and what future projects I think could be done in this space (more).
Explain why I think aligning narrowly superhuman models could meaningfully reduce long-term existential risk from misaligned AI (more).
Lay out the potential advantages that I think this work has over other types of AI alignment research: (a) conceptual thinking, (b) demos in small-scale artificial settings, and (c) mainstream ML safety such as interpretability and robustness (more).
Answer some objections and questions about this research direction, e.g. concerns that it’s not very neglected, feels suspiciously similar to commercialization, might cause harm by exacerbating AI race dynamics, or is dominated by another t...
Welcome to The Nonlinear Library, where we use Text-to-Speech software to convert the best writing from the Rationalist and EA communities into audio.
This is: Realism about rationality , published by Richard Ngo on the AI Alignment Forum.
This is a linkpost for http://thinkingcomplete.blogspot.com/2018/09/rational-and-real.html
Epistemic status: trying to vaguely gesture at vague intuitions. A similar idea was explored here under the heading "the intelligibility of intelligence", although I hadn't seen it before writing this post. As of 2020, I consider this follow-up comment to be a better summary of the thing I was trying to convey with this post than the post itself.
There’s a mindset which is common in the rationalist community, which I call “realism about rationality” (the name being intended as a parallel to moral realism). I feel like my skepticism about agent foundations research is closely tied to my skepticism about this mindset, and so in this essay I try to articulate what it is.
Humans ascribe properties to entities in the world in order to describe and predict them. Here are three such properties: "momentum", "evolutionary fitness", and "intelligence". These are all pretty useful properties for high-level reasoning in the fields of physics, biology and AI, respectively. There's a key difference between the first two, though. Momentum is very amenable to formalisation: we can describe it using precise equations, and even prove things about it. Evolutionary fitness is the opposite: although nothing in biology makes sense without it, no biologist can take an organism and write down a simple equation to define its fitness in terms of more basic traits. This isn't just because biologists haven't figured out that equation yet. Rather, we have excellent reasons to think that fitness is an incredibly complicated "function" which basically requires you to describe that organism's entire phenotype, genotype and environment.
In a nutshell, then, realism about rationality is a mindset in which reasoning and intelligence are more like momentum than like fitness. It's a mindset which makes the following ideas seem natural:
The idea that there is a simple yet powerful theoretical framework which describes human intelligence and/or intelligence in general. (I don't count brute force approaches like AIXI for the same reason I don't consider physics a simple yet powerful description of biology).
The idea that there is an “ideal” decision theory.
The idea that AGI will very likely be an “agent”.
The idea that Turing machines and Kolmogorov complexity are foundational for epistemology.
The idea that, given certain evidence for a proposition, there's an "objective" level of subjective credence which you should assign to it, even under computational constraints.
The idea that Aumann's agreement theorem is relevant to humans.
The idea that morality is quite like mathematics, in that there are certain types of moral reasoning that are just correct.
The idea that defining coherent extrapolated volition in terms of an idealised process of reflection roughly makes sense, and that it converges in a way which doesn’t depend very much on morally arbitrary factors.
The idea that having having contradictory preferences or beliefs is really bad, even when there’s no clear way that they’ll lead to bad consequences (and you’re very good at avoiding dutch books and money pumps and so on).
To be clear, I am neither claiming that realism about rationality makes people dogmatic about such ideas, nor claiming that they're all false. In fact, from a historical point of view I’m quite optimistic about using maths to describe things in general. But starting from that historical baseline, I’m inclined to adjust downwards on questions related to formalising intelligent thought, whereas rationality realism would endorse adjusting upwards. This essay is primarily intended to explain my position, not justify it, but one important consideration for me is th...
Welcome to The Nonlinear Library, where we use Text-to-Speech software to convert the best writing from the Rationalist and EA communities into audio.
This is: Birds, Brains, Planes, and AI: Against Appeals to the Complexity/Mysteriousness/Efficiency of the Brain, published by Daniel Kokotajlo on the AI Alignment Forum.
[Epistemic status: Strong opinions lightly held, this time with a cool graph.]
I argue that an entire class of common arguments against short timelines is bogus, and provide weak evidence that anchoring to the human-brain-human-lifetime milestone is reasonable.
In a sentence, my argument is that the complexity and mysteriousness and efficiency of the human brain (compared to artificial neural nets) is almost zero evidence that building TAI will be difficult, because evolution typically makes things complex and mysterious and efficient, even when there are simple, easily understood, inefficient designs that work almost as well (or even better!) for human purposes.
In slogan form: If all we had to do to get TAI was make a simple neural net 10x the size of my brain, my brain would still look the way it does.
The case of birds & planes illustrates this point nicely. Moreover, it is also a precedent for several other short-timelines talking points, such as the human-brain-human-lifetime (HBHL) anchor.
Plan:
Illustrative Analogy
Exciting Graph
Analysis
Extra brute force can make the problem a lot easier
Evolution produces complex mysterious efficient designs by default, even when simple inefficient designs work just fine for human purposes.
What’s bogus and what’s not
Example: Data-efficiency
Conclusion
Appendix
1909 French military plane, the Antionette VII.
By Deep silence (Mikaël Restoux) - Own work (Bourget museum, in France), CC BY 2.5,
Illustrative Analogy
AI timelines, from our current perspective Flying machine timelines, from the perspective of the late 1800’s:
Shorty: Human brains are giant neural nets. This is reason to think we can make human-level AGI (or at least AI with strategically relevant skills, like politics and science) by making giant neural nets. Shorty: Birds are winged creatures that paddle through the air. This is reason to think we can make winged machines that paddle through the air.
Longs: Whoa whoa, there are loads of important differences between brains and artificial neural nets: [what follows is a direct quote from the objection a friend raised when reading an early draft of this post!]
- During training, deep neural nets use some variant of backpropagation. My understanding is that the brain does something else, closer to Hebbian learning. (Though I vaguely remember at least one paper claiming that maybe the brain does something that's similar to backprop after all.)
- It's at least possible that the wiring diagram of neurons plus weights is too coarse-grained to accurately model the brain's computation, but it's all there is in deep neural nets. If we need to pay attention to glial cells, intracellular processes, different neurotransmitters etc., it's not clear how to integrate this into the deep learning paradigm.
- My impression is that several biological observations on the brain don't have a plausible analog in deep neural nets: growing new neurons (though unclear how important it is for an adult brain), "repurposing" in response to brain damage, .
Longs: Whoa whoa, there are loads of important differences between birds and flying machines:
- Birds paddle the air by flapping, whereas current machine designs use propellers and fixed wings.
- It’s at least possible that the anatomical diagram of bones, muscles, and wing surfaces is too coarse-grained to accurately model how a bird flies, but that’s all there is to current machine designs (replacing bones with struts and muscles with motors, that is). If we need to pay attention to the percolation of air through and between feathers, micro-eddies in the air sensed by the bird and instinctively responded to, etc. it’s not clear ...
Welcome to The Nonlinear Library, where we use Text-to-Speech software to convert the best writing from the Rationalist and EA communities into audio.
This is: Goodhart Taxonomy, published by Scott Garrabrant on the AI Alignment Forum.
Goodhart’s Law states that "any observed statistical regularity will tend to collapse once pressure is placed upon it for control purposes." However, this is not a single phenomenon. I propose that there are (at least) four different mechanisms through which proxy measures break when you optimize for them.
The four types are Regressional, Causal, Extremal, and Adversarial. In this post, I will go into detail about these four different Goodhart effects using mathematical abstractions as well as examples involving humans and/or AI. I will also talk about how you can mitigate each effect.
Throughout the post, I will use
V
to refer to the true goal and use
U
to refer to a proxy for that goal which was observed to correlate with
V
and which is being optimized in some way.
Quick Reference
Regressional Goodhart - When selecting for a proxy measure, you select not only for the true goal, but also for the difference between the proxy and the goal.
Model: When
U
is equal to
V
X
, where
X
is some noise, a point with a large
U
value will likely have a large
V
value, but also a large
X
value. Thus, when
U
is large, you can expect
V
to be predictably smaller than
U
Example: height is correlated with basketball ability, and does actually directly help, but the best player is only 6'3", and a random 7' person in their 20s would probably not be as good
Causal Goodhart - When there is a non-causal correlation between the proxy and the goal, intervening on the proxy may fail to intervene on the goal.
Model: If
V
causes
U
(or if
V
and
U
are both caused by some third thing), then a correlation between
V
and
U
may be observed. However, when you intervene to increase
U
through some mechanism that does not involve
V
, you will fail to also increase
V
Example: someone who wishes to be taller might observe that height is correlated with basketball skill and decide to start practicing basketball.
Extremal Goodhart - Worlds in which the proxy takes an extreme value may be very different from the ordinary worlds in which the correlation between the proxy and the goal was observed.
Model: Patterns tend to break at simple joints. One simple subset of worlds is those worlds in which
U
is very large. Thus, a strong correlation between
U
and
V
observed for naturally occuring
U
values may not transfer to worlds in which
U
is very large. Further, since there may be relatively few naturally occuring worlds in which
U
is very large, extremely large
U
may coincide with small
V
values without breaking the statistical correlation.
Example: the tallest person on record, Robert Wadlow, was 8'11" (2.72m). He grew to that height because of a pituitary disorder, he would have struggled to play basketball because he "required leg braces to walk and had little feeling in his legs and feet."
Adversarial Goodhart - When you optimize for a proxy, you provide an incentive for adversaries to correlate their goal with your proxy, thus destroying the correlation with your goal.
Model: Consider an agent
A
with some different goal
W
. Since they depend on common resources,
W
and
V
are naturally opposed. If you optimize
U
as a proxy for
V
, and
A
knows this,
A
is incentivized to make large
U
values coincide with large
W
values, thus stopping them from coinciding with large
V
values.
Example: aspiring NBA players might just lie about their height.
Regressional Goodhart
When selecting for a proxy measure, you select not only for the true goal, but also for the difference between the proxy and the goal.
Abstract Model
When
U
is equal to
V
X
, where
X
is some noise, a point with a large
U
value will likely have a large
V
value, but also a large
X
value. Thus, when
U
is large, you can expect
V
to be predictably smaller than
U
The above description is whe...
Welcome to The Nonlinear Library, where we use Text-to-Speech software to convert the best writing from the Rationalist and EA communities into audio.
This is: The ground of optimization, published by Alex Flint on the AI Alignment Forum.
This work was supported by OAK, a monastic community in the Berkeley hills. This document could not have been written without the daily love of living in this beautiful community. The work involved in writing this cannot be separated from the sitting, chanting, cooking, cleaning, crying, correcting, fundraising, listening, laughing, and teaching of the whole community.
What is optimization? What is the relationship between a computational optimization process — say, a computer program solving an optimization problem — and a physical optimization process — say, a team of humans building a house?
We propose the concept of an optimizing system as a physically closed system containing both that which is being optimized and that which is doing the optimizing, and defined by a tendency to evolve from a broad basin of attraction towards a small set of target configurations despite perturbations to the system. We compare our definition to that proposed by Yudkowsky, and place our work in the context of work by Demski and Garrabrant’s Embedded Agency, and Drexler’s Comprehensive AI Services. We show that our definition resolves difficult cases proposed by Daniel Filan. We work through numerous examples of biological, computational, and simple physical systems showing how our definition relates to each.
Introduction
In the field of computer science, an optimization algorithm is a computer program that outputs the solution, or an approximation thereof, to an optimization problem. An optimization problem consists of an objective function to be maximized or minimized, and a feasible region within which to search for a solution. For example we might take the objective function
x
2
−
2
2
as a minimization problem and the whole real number line as the feasible region. The solution then would be
x
√
2
and a working optimization algorithm for this problem is one that outputs a close approximation to this value.
In the field of operations research and engineering more broadly, optimization involves improving some process or physical artifact so that it is fit for a certain purpose or fulfills some set of requirements. For example, we might choose to measure a nail factory by the rate at which it outputs nails, relative to the cost of production inputs. We can view this as a kind of objective function, with the factory as the object of optimization just as the variable x was the object of optimization in the previous example.
There is clearly a connection between optimizing the factory and optimizing for x, but what exactly is this connection? What is it that identifies an algorithm as an optimization algorithm? What is it that identifies a process as an optimization process?
The answer proposed in this essay is: an optimizing system is a physical process in which the configuration of some part of the universe moves predictably towards a small set of target configurations from any point in a broad basin of optimization, despite perturbations during the optimization process.
We do not imagine that there is some engine or agent or mind performing optimization, separately from that which is being optimized. We consider the whole system jointly — engine and object of optimization — and ask whether it exhibits a tendency to evolve towards a predictable target configuration. If so, then we call it an optimizing system. If the basin of attraction is deep and wide then we say that this is a robust optimizing system.
An optimizing system as defined in this essay is known in dynamical systems theory as a dynamical system with one or more attractors. In this essay we show how this framework can help to understand optimization as manifested in physically closed systems containing both engine and object of optimization.
I...
Welcome to The Nonlinear Library, where we use Text-to-Speech software to convert the best writing from the Rationalist and EA communities into audio.
This is: An overview of 11 proposals for building safe advanced AI , published by Evan Hubinger on the AI Alignment Forum.
This is the blog post version of the paper by the same name. Special thanks to Kate Woolverton, Paul Christiano, Rohin Shah, Alex Turner, William Saunders, Beth Barnes, Abram Demski, Scott Garrabrant, Sam Eisenstat, and Tsvi Benson-Tilsen for providing helpful comments and feedback on this post and the talk that preceded it.
This post is a collection of 11 different proposals for building safe advanced AI under the current machine learning paradigm. There's a lot of literature out there laying out various different approaches such as amplification, debate, or recursive reward modeling, but a lot of that literature focuses primarily on outer alignment at the expense of inner alignment and doesn't provide direct comparisons between approaches. The goal of this post is to help solve that problem by providing a single collection of 11 different proposals for building safe advanced AI—each including both inner and outer alignment components. That being said, not only does this post not cover all existing proposals, I strongly expect that there will be lots of additional new proposals to come in the future. Nevertheless, I think it is quite useful to at least take a broad look at what we have now and compare and contrast some of the current leading candidates.
It is important for me to note before I begin that the way I describe the 11 approaches presented here is not meant to be an accurate representation of how anyone else would represent them. Rather, you should treat all the approaches I describe here as my version of that approach rather than any sort of canonical version that their various creators/proponents would endorse.
Furthermore, this post only includes approaches that intend to directly build advanced AI systems via machine learning. Thus, this post doesn't include other possible approaches for solving the broader AI existential risk problem such as:
finding a fundamentally different way of approaching AI than the current machine learning paradigm that makes it easier to build safe advanced AI,
developing some advanced technology that produces a decisive strategic advantage without using advanced AI, or
achieving global coordination around not building advanced AI via (for example) a persuasive demonstration that any advanced AI is likely to be unsafe.
For each of the proposals that I consider, I will try to evaluate them on the following four basic components that I think any story for how to build safe advanced AI under the current machine learning paradigm needs.
Outer alignment. Outer alignment is about asking why the objective we're training for is aligned—that is, if we actually got a model that was trying to optimize for the given loss/reward/etc., would we like that model? For a more thorough description of what I mean by outer alignment, see “Outer alignment and imitative amplification.”
Inner alignment. Inner alignment is about asking the question of how our training procedure can actually guarantee that the model it produces will, in fact, be trying to accomplish the objective we trained it on. For a more rigorous treatment of this question and an explanation of why it might be a concern, see “Risks from Learned Optimization.”
Training competitiveness. Competitiveness is a bit of a murky concept, so I want to break it up into two pieces here. Training competitiveness is the question of whether the given training procedure is one that a team or group of teams with a reasonable lead would be able to afford to implement without completely throwing away that lead. Thus, training competitiveness is about whether the proposed process of producing advanced AI is competitive.
Performance competitiveness. Performance competitiveness, on the othe...