Unlocking AI's Potential: How Retrieval-Augmented Generation Bridges Knowledge Gaps
Digest
This episode of the Beginners Guide to AI explores Retrieval Augmented Generation (RAG), a groundbreaking technique in natural language processing. RAG combines the power of retrieval-based models and generative models to create more accurate and contextually relevant responses. Generative models, like GPT-4, are trained on vast amounts of text data and learn patterns and knowledge. However, they are limited to the information available at their last training update. Retrieval-based models, on the other hand, actively search for relevant information from external databases or documents, providing access to the latest data. RAG merges these two approaches, first retrieving relevant information from external sources and then using this data to enhance the generative model's response. This hybrid approach ensures that AI responses are informed by both training data and real-time information, making them more accurate and relevant. The episode uses a simple example of baking a cake to illustrate how RAG can provide more helpful advice by incorporating the latest culinary blogs and articles. A hypothetical case study in the medical field further highlights RAG's potential, showcasing how it can assist doctors and medical staff with up-to-date treatment protocols, research findings, and drug information. RAG also reduces the occurrence of AI hallucinations by grounding the generative model with real, retrieved data. The episode concludes by emphasizing the importance of continuous learning and encourages listeners to explore RAG further.
Outlines
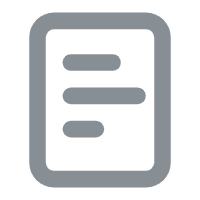
Introduction to Retrieval Augmented Generation (RAG)
This Chapter introduces the concept of Retrieval Augmented Generation (RAG) as a revolutionary technique in natural language processing. It explains how RAG combines retrieval-based models and generative models to provide more accurate and up-to-date information by accessing real-time data. The chapter also highlights the limitations of traditional generative models and how RAG addresses these limitations.
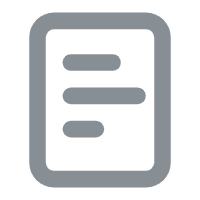
Case Study: RAG in Healthcare
This Chapter delves into a hypothetical case study showcasing the application of RAG in the medical field. It explores how a healthcare tech company, MedInfo Solutions, could use RAG to keep doctors and medical staff updated with the latest treatment protocols, research findings, and drug information. The chapter highlights the significant impact RAG could have in critical industries where access to the most current data can be a matter of life and death.
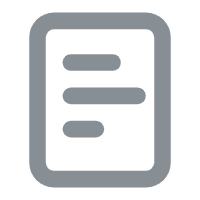
Call to Action: Explore RAG Further
This Chapter encourages listeners to explore RAG further by researching recent articles or papers on topics they are passionate about. It also suggests using AI tools that incorporate RAG and observing the difference in the responses. The chapter emphasizes the importance of continuous learning and staying updated with the latest advancements in AI.
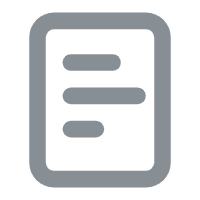
Summary and Conclusion
This Chapter summarizes the key points discussed in the episode, emphasizing the transformative potential of RAG in various fields. It highlights how RAG bridges the gap between static knowledge and dynamic real-world data, making AI-generated responses more accurate, relevant, and timely. The chapter concludes by emphasizing that RAG is not just about generating responses but about enriching them with the most relevant and current information, making AI an even more powerful tool for solving real-world problems.
Keywords
Retrieval Augmented Generation (RAG)
Retrieval Augmented Generation (RAG) is a revolutionary AI technique that combines retrieval-based models with generative models to provide more accurate and up-to-date information by accessing real-time data. It enhances the accuracy and relevance of AI-generated responses by grounding them in current information.
Generative Model
A generative model is an AI model trained on vast amounts of text data to learn patterns, structures, and common knowledge. It generates responses based on the patterns it has learned, but its knowledge is limited to the information available at its last training update.
Retrieval-Based Model
A retrieval-based model is an AI model that actively searches for relevant information from external databases or documents. It provides access to the latest information, which is crucial in rapidly evolving fields or specific queries requiring up-to-date knowledge.
GPT-4
GPT-4 is a large language model developed by OpenAI. It is a powerful generative model known for its ability to generate human-like text, translate languages, write different kinds of creative content, and answer your questions in an informative way.
Natural Language Processing (NLP)
Natural Language Processing (NLP) is a field of computer science and artificial intelligence that focuses on the interaction between computers and human language. It involves enabling computers to understand, interpret, and generate human language.
AI Hallucinations
AI hallucinations refer to instances where an AI system generates incorrect or nonsensical information. This can occur when the AI model lacks sufficient training data or when it encounters unfamiliar situations. RAG helps reduce hallucinations by grounding the generative model with real, retrieved data.
PubMed
PubMed is a free search engine accessing primarily the MEDLINE database of biomedical literature. It is a valuable resource for healthcare professionals and researchers seeking the latest medical research and publications.
MedInfo Solutions
MedInfo Solutions is a hypothetical healthcare tech company used in the episode as an example of how RAG could be implemented in the medical field. It highlights the potential of RAG to improve patient outcomes by providing doctors and medical staff with up-to-date medical information.
Isaac Asimov
Isaac Asimov was a renowned science fiction writer and biochemist. He is known for his works like the Foundation series and the Robot series, which explored themes of artificial intelligence, robotics, and the future of humanity. His quote, "Self-education is, I firmly believe, the only kind of education there is," emphasizes the importance of continuous learning and staying updated, a principle that aligns with the concept of RAG.
Q&A
What is Retrieval Augmented Generation (RAG) and how does it work?
RAG is a revolutionary AI technique that combines retrieval-based models with generative models to provide more accurate and up-to-date information. It first retrieves relevant information from external sources and then uses this data to enhance the generative model's response, ensuring that AI responses are informed by both training data and real-time information.
What are the limitations of traditional generative models?
Traditional generative models, like GPT-4, are limited to the information available at their last training update. They might miss out on the most recent facts or specific details not covered in their training data.
How does RAG address the limitations of traditional generative models?
RAG addresses these limitations by incorporating retrieval-based models, which actively search for relevant information from external databases or documents. This provides access to the latest information, ensuring that AI responses are more accurate and up-to-date.
What are some real-world examples of how RAG can be used?
RAG can be used in various fields, including customer service, education, and healthcare. For example, in healthcare, RAG can assist doctors and medical staff with up-to-date treatment protocols, research findings, and drug information, improving patient outcomes.
How does RAG reduce the occurrence of AI hallucinations?
RAG reduces hallucinations by grounding the generative model with real, retrieved data. This makes the responses more factual and reliable, especially important in professional settings where the accuracy of information is paramount.
What is the importance of continuous learning in the context of RAG?
Continuous learning is crucial in the context of RAG because it ensures that AI systems can access and integrate the latest information. This is essential for providing accurate and relevant responses in rapidly evolving fields.
How can I explore RAG further?
You can explore RAG further by researching recent articles or papers on topics you are passionate about. You can also try using AI tools that incorporate RAG and observe the difference in the responses.
Show Notes
In this episode of "A Beginner's Guide to AI", Professor GePhardT delves into the fascinating world of retrieval-augmented generation (RAG). Discover how this cutting-edge technique enhances AI's ability to generate accurate and contextually relevant responses by combining the strengths of retrieval-based and generative models.
From a simple cake-baking example to a hypothetical medical case study, learn how RAG leverages real-time data to provide the most current and precise information. Join us as we explore the transformative potential of RAG and its implications for various industries.
Tune in to get my thoughts, don't forget to subscribe to our Newsletter!
Want to get in contact? Write me an email: podcast@argo.berlin
This podcast is generated with the help of ChatGPT and Claude 3. We do fact-check with human eyes but there still might be hallucinations in the output.
Music credit: "Modern Situations by Unicorn Heads"