Discover
Oracle University Podcast
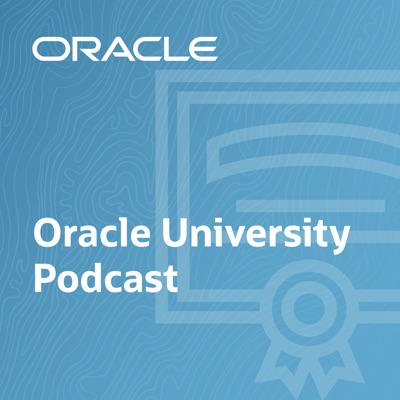
Oracle University Podcast
Author: Oracle Corporation
Subscribed: 17Played: 107Subscribe
Share
© 2023 Oracle Corporation
Description
Oracle University Podcast delivers convenient, foundational training on popular Oracle technologies such as Oracle Cloud Infrastructure, Java, Autonomous Database, and more to help you jump-start or advance your career in the cloud.
69 Episodes
Reverse
Listen to Lois Houston and Nikita Abraham, along with Senior Principal Product Manager Wes Prichard, as they explore the five core components of OCI AI services: language, speech, vision, document understanding, and anomaly detection, to help you make better sense of all that unstructured data around you. Oracle MyLearn: https://mylearn.oracle.com/ou/learning-path/become-an-oci-ai-foundations-associate-2023/127177 Oracle University Learning Community: https://education.oracle.com/ou-community LinkedIn: https://www.linkedin.com/showcase/oracle-university/ X (formerly Twitter): https://twitter.com/Oracle_Edu Special thanks to Arijit Ghosh, David Wright, Himanshu Raj, and the OU Studio Team for helping us create this episode. -------------------------------------------------------- Episode Transcript: 00:00 The world of artificial intelligence is vast and everchanging. And with all the buzz around it lately, we figured it was the perfect time to revisit our AI Made Easy series. Join us over the next few weeks as we chat about all things AI, helping you to discover its endless possibilities. Ready to dive in? Let’s go! 00:33 Welcome to the Oracle University Podcast, the first stop on your cloud journey. During this series of informative podcasts, we’ll bring you foundational training on the most popular Oracle technologies. Let’s get started! 00:46 Nikita: Welcome to the Oracle University Podcast! I’m Nikita Abraham, Principal Technical Editor with Oracle University, and with me is Lois Houston, Director of Innovation Programs. Lois: Hi there! In our last episode, we spoke about OCI AI Portfolio, including AI and ML services, and the OCI AI infrastructure. Nikita: Yeah, and in today’s episode, we’re going to continue down a similar path and take a closer look at OCI AI services. 01:16 Lois: With us today is Senior Principal Product Manager, Wes Prichard. Hi Wes! It’s lovely to have you here with us. Hemant gave us a broad overview of the various OCI AI services last week, but we’re really hoping to get into each of them with you. So, let’s jump right in and start with the OCI Language service. What can you tell us about it? Wes: OCI Language analyzes unstructured text for you. It provides models trained on industry data to perform language analysis with no data science experience needed. 01:48 Nikita: What kind of big things can it do? Wes: It has five main capabilities. First, it detects the language of the text. It recognizes 75 languages, from Afrikaans to Welsh. It identifies entities, things like names, places, dates, emails, currency, organizations, phone numbers--14 types in all. It identifies the sentiment of the text, and not just one sentiment for the entire block of text, but the different sentiments for different aspects. 02:17 Nikita: What do you mean by that, Wes? Wes: So let's say you read a restaurant review that said, the food was great, but the service sucked. You'll get food with a positive sentiment and service with a negative sentiment. And it also analyzes the sentiment for every sentence. Lois: Ah, that’s smart. Ok, so we covered three capabilities. What else? Wes: It identifies key phrases in the text that represent the important ideas or subjects. And it classifies the general topic of the text from a list of 600 categories and subcategories. 02:48 Lois: Ok, and then there’s the OCI Speech service... Wes: OCI Speech is very straightforward. It locks the data in audio tracks by converting speech to text. Developers can use Oracle's time-tested acoustic language models to provide highly accurate transcription for audio or video files across multiple languages. OCI Speech automatically transcribes audio and video files into text using advanced deep learning techniques. There's no data science experience required. It processes data directly in object storage. And it generates timestamped, grammatically accurate transcriptions. 03:22 Nikita: What are some of the main features of OCI Speech? Wes: OCI Speech supports multiple languages, specifically English, Spanish, and Portuguese, with more coming in the future. It has batching support where multiple files can be submitted with a single call. It has blazing fast processing. It can transcribe hours of audio in less than 10 minutes. It does this by chunking up your audio into smaller segments, and transcribing each segment, and then joining them all back together into a single file. It provides a confidence score, both per word and per transcription. It punctuates transcriptions to make the text more readable and to allow downstream systems to process the text with less friction. And it has SRT file support. 04:06 Lois: SRT? What’s that? Wes: SRT is the most popular closed caption output file format. And with this SRT support, users can add closed captions to their video. OCI Speech makes transcribed text more readable to resemble how humans write. This is called normalization. And the service will normalize things like addresses, times, numbers, URLs, and more. It also does profanity filtering, where it can either remove, mask, or tag profanity and output text, where removing replaces the word with asterisks, and masking does the same thing, but it retains the first letter, and tagging will leave the word in place, but it provides tagging in the output data. 04:49 Nikita: And what about OCI Vision? What are its capabilities? Wes: Vision is a computed vision service that works on images, and it provides two main capabilities-- image analysis and document AI. Image analysis analyzes photographic images. Object detection is the feature that detects objects inside an image using a bounding box and assigning a label to each object with an accuracy percentage. Object detection also locates and extracts text that appears in the scene, like on a sign. Image classification will assign classification labels to the image by identifying the major features in the scene. One of the most powerful capabilities of image analysis is that, in addition to pretrained models, users can retrain the models with their own unique data to fit their specific needs. 05:40 Lois: So object detection and image classification are features of image analysis. I think I got it! So then what’s document AI? Wes: It's used for working with document images. You can use it to understand PDFs or document image types, like JPEG, PNG, and Tiff, or photographs containing textual information. 06:01 Lois: And what are its most important features? Wes: The features of document AI are text recognition, also known as OCR or optical character recognition. And this extracts text from images, including non-trivial scenarios, like handwritten texts, plus tilted, shaded, or rotated documents. Document classification classifies documents into 10 different types based on visual appearance, high-level features, and extracted keywords. This is useful when you need to process a document, based on its classification, like an invoice, a receipt, or a resume. Language detection analyzes the visual features of text to determine the language rather than relying on the text itself. Table extraction identifies tables in docs and extracts their content in tabular form. Key value extraction finds values for 13 common fields and line items in receipts, things like merchant name and transaction date. 07:02 Want to get the inside scoop on Oracle University? Head over to the Oracle University Learning Community. Attend exclusive events. Read up on the latest news. Get first-hand access to new products. Read the OU Learning Blog. Participate in Challenges. And stay up-to-date with upcoming certification opportunities. Visit mylearn.oracle.com to get started. 07:27 Nikita: Welcome back! Wes, I want to ask you about OCI Anomaly Detection. We discussed it a bit last week and it seems like such an intelligent and efficient service. Wes: Oracle Cloud Infrastructure Anomaly Detection identifies anomalies in time series data. Equipment sensors generate time series data, but all kinds of business metrics are also time-based. The unique feature of this service is that it finds anomalies, not just in a single signal, but across many signals at once. That's important because machines often generate multiple signals at once and the signals are often related. 08:03 Nikita: Ok you need to give us an example of this! Wes: Think of a pump that has an output pressure, a flow rate, an RPM, and an electrical current draw. When a pump's going to fail, anomalies may appear across several of those signals but at different times. OCI Anomaly Detection helps you to identify anomalies in a multivariate data set by taking advantage of the interrelationship among signals. The service contains algorithms for both multi-signal, as in multivariate, single signal, as in univariate anomaly detection, and it automatically determines which algorithm to use based on the training data provided. The multivariate algorithm is called MSET-2, which stands for Multivariate State Estimation technique, and it's unique to Oracle. 08:49 Lois: And the 2? Wes: The 2 in the name refers to the patented enhancements by Oracle labs that automatically identify and fix data quality issues resulting in fewer false alarms and more accurate results. Now unlike some of the other AI services, OCI Anomaly Detection is always trained on the customer's data. It's trained using actual historical data with no anomalies, and there can be as many different trained models as needed for different sets of signals. 09:18 Nikita: So where would one use a service like this? Wes: One of the most obvious applications of this service is for predictive maintenance. Early warning of a problem provides the opportunity to deploy maintenance resources and schedule downtime to minimize disruption to the business. 09:33 Lois: How would you train an OCI Anomaly Detection model? Wes: It's a simple four-step process to prepare a model that can be used for a
Oracle has been actively focusing on bringing AI to the enterprise at every layer of its tech stack, be it SaaS apps, AI services, infrastructure, or data. In this episode, hosts Lois Houston and Nikita Abraham, along with senior instructors Hemant Gahankari and Himanshu Raj, discuss OCI AI and Machine Learning services. They also go over some key OCI Data Science concepts and responsible AI principles. Oracle MyLearn: https://mylearn.oracle.com/ou/learning-path/become-an-oci-ai-foundations-associate-2023/127177 Oracle University Learning Community: https://education.oracle.com/ou-community LinkedIn: https://www.linkedin.com/showcase/oracle-university/ X (formerly Twitter): https://twitter.com/Oracle_Edu Special thanks to Arijit Ghosh, David Wright, Himanshu Raj, and the OU Studio Team for helping us create this episode. -------------------------------------------------------- Episode Transcript: 00:00 The world of artificial intelligence is vast and everchanging. And with all the buzz around it lately, we figured it was the perfect time to revisit our AI Made Easy series. Join us over the next few weeks as we chat about all things AI, helping you to discover its endless possibilities. Ready to dive in? Let’s go! 00:33 Welcome to the Oracle University Podcast, the first stop on your cloud journey. During this series of informative podcasts, we’ll bring you foundational training on the most popular Oracle technologies. Let’s get started! 00:46 Lois: Welcome to the Oracle University Podcast! I’m Lois Houston, Director of Innovation Programs with Oracle University, and with me is Nikita Abraham, Principal Technical Editor. Nikita: Hey everyone! In our last episode, we dove into Generative AI and Language Learning Models. Lois: Yeah, that was an interesting one. But today, we’re going to discuss the AI and machine learning services offered by Oracle Cloud Infrastructure, and we’ll look at the OCI AI infrastructure. Nikita: I’m also going to try and squeeze in a couple of questions on a topic I’m really keen about, which is responsible AI. To take us through all of this, we have two of our colleagues, Hemant Gahankari and Himanshu Raj. Hemant is a Senior Principal OCI Instructor and Himanshu is a Senior Instructor on AI/ML. So, let’s get started! 01:36 Lois: Hi Hemant! We’re so excited to have you here! We know that Oracle has really been focusing on bringing AI to the enterprise at every layer of our stack. Hemant: It all begins with data and infrastructure layers. OCI AI services consume data, and AI services, in turn, are consumed by applications. This approach involves extensive investment from infrastructure to SaaS applications. Generative AI and massive scale models are the more recent steps. Oracle AI is the portfolio of cloud services for helping organizations use the data they may have for the business-specific uses. Business applications consume AI and ML services. The foundation of AI services and ML services is data. AI services contain pre-built models for specific uses. Some of the AI services are pre-trained, and some can be additionally trained by the customer with their own data. AI services can be consumed by calling the API for the service, passing in the data to be processed, and the service returns a result. There is no infrastructure to be managed for using AI services. 02:58 Nikita: How do I access OCI AI services? Hemant: OCI AI services provide multiple methods for access. The most common method is the OCI Console. The OCI Console provides an easy to use, browser-based interface that enables access to notebook sessions and all the features of all the data science, as well as AI services. The REST API provides access to service functionality but requires programming expertise. And API reference is provided in the product documentation. OCI also provides programming language SDKs for Java, Python, TypeScript, JavaScript, .Net, Go, and Ruby. The command line interface provides both quick access and full functionality without the need for scripting. 03:52 Lois: Hemant, what are the types of OCI AI services that are available? Hemant: OCI AI services is a collection of services with pre-built machine learning models that make it easier for developers to build a variety of business applications. The models can also be custom trained for more accurate business results. The different services provided are digital assistant, language, vision, speech, document understanding, anomaly detection. 04:24 Lois: I know we’re going to talk about them in more detail in the next episode, but can you introduce us to OCI Language, Vision, and Speech? Hemant: OCI Language allows you to perform sophisticated text analysis at scale. Using the pre-trained and custom models, you can process unstructured text to extract insights without data science expertise. Pre-trained models include language detection, sentiment analysis, key phrase extraction, text classification, named entity recognition, and personal identifiable information detection. Custom models can be trained for named entity recognition and text classification with domain-specific data sets. In text translation, natural machine translation is used to translate text across numerous languages. Using OCI Vision, you can upload images to detect and classify objects in them. Pre-trained models and custom models are supported. In image analysis, pre-trained models perform object detection, image classification, and optical character recognition. In image analysis, custom models can perform custom object detection by detecting the location of custom objects in an image and providing a bounding box. The OCI Speech service is used to convert media files to readable texts that's stored in JSON and SRT format. Speech enables you to easily convert media files containing human speech into highly exact text transcriptions. 06:12 Nikita: That’s great. And what about document understanding and anomaly detection? Hemant: Using OCI document understanding, you can upload documents to detect and classify text and objects in them. You can process individual files or batches of documents. In OCR, document understanding can detect and recognize text in a document. In text extraction, document understanding provides the word level and line level text, and the bounding box, coordinates of where the text is found. In key value extraction, document understanding extracts a predefined list of key value pairs of information from receipts, invoices, passports, and driver IDs. In table extraction, document understanding extracts content in tabular format, maintaining the row and column relationship of cells. In document classification, the document understanding classifies documents into different types. The OCI Anomaly Detection service is a service that analyzes large volume of multivariate or univariate time series data. The Anomaly Detection service increases the reliability of businesses by monitoring their critical assets and detecting anomalies early with high precision. Anomaly Detection is the identification of rare items, events, or observations in data that differ significantly from the expectation. 07:55 Nikita: Where is Anomaly Detection most useful? Hemant: The Anomaly Detection service is designed to help with analyzing large amounts of data and identifying the anomalies at the earliest possible time with maximum accuracy. Different sectors, such as utility, oil and gas, transportation, manufacturing, telecommunications, banking, and insurance use Anomaly Detection service for their day-to-day activities. 08:23 Lois: Ok…and the first OCI AI service you mentioned was digital assistant… Hemant: Oracle Digital Assistant is a platform that allows you to create and deploy digital assistants, which are AI driven interfaces that help users accomplish a variety of tasks with natural language conversations. When a user engages with the Digital Assistant, the Digital Assistant evaluates the user input and routes the conversation to and from the appropriate skills. Digital Assistant greets the user upon access. Upon user requests, list what it can do and provide entry points into the given skills. It routes explicit user requests to the appropriate skills. And it also handles interruptions to flows and disambiguation. It also handles requests to exit the bot. 09:21 Nikita: Excellent! Let’s bring Himanshu in to tell us about machine learning services. Hi Himanshu! Let’s talk about OCI Data Science. Can you tell us a bit about it? Himanshu: OCI Data Science is the cloud service focused on serving the data scientist throughout the full machine learning life cycle with support for Python and open source. The service has many features, such as model catalog, projects, JupyterLab notebook, model deployment, model training, management, model explanation, open source libraries, and AutoML. 09:56 Lois: Himanshu, what are the core principles of OCI Data Science? Himanshu: There are three core principles of OCI Data Science. The first one, accelerated. The first principle is about accelerating the work of the individual data scientist. OCI Data Science provides data scientists with open source libraries along with easy access to a range of compute power without having to manage any infrastructure. It also includes Oracle's own library to help streamline many aspects of their work. The second principle is collaborative. It goes beyond an individual data scientist’s productivity to enable data science teams to work together. This is done through the sharing of assets, reducing duplicative work, and putting reproducibility and auditability of models for collaboration and risk management. Third is enterprise grade. That means it's integrated with all the OCI Security and access protocols. The underlying infrastructure is fully managed. The customer does not have to think about provisioning compute and storage. And the service handles all the maintenan
In this week’s episode, Lois Houston and Nikita Abraham, along with Senior Instructor Himanshu Raj, take you through the extraordinary capabilities of Generative AI, a subset of deep learning that doesn’t make predictions but rather creates its own content. They also explore the workings of Large Language Models. Oracle MyLearn: https://mylearn.oracle.com/ou/learning-path/become-an-oci-ai-foundations-associate-2023/127177 Oracle University Learning Community: https://education.oracle.com/ou-community LinkedIn: https://www.linkedin.com/showcase/oracle-university/ X (formerly Twitter): https://twitter.com/Oracle_Edu Special thanks to Arijit Ghosh, David Wright, and the OU Studio Team for helping us create this episode. --------------------------------------------------------- Episode Transcript: 00:00 The world of artificial intelligence is vast and everchanging. And with all the buzz around it lately, we figured it was the perfect time to revisit our AI Made Easy series. Join us over the next few weeks as we chat about all things AI, helping you to discover its endless possibilities. Ready to dive in? Let’s go! 00:33 Welcome to the Oracle University Podcast, the first stop on your cloud journey. During this series of informative podcasts, we’ll bring you foundational training on the most popular Oracle technologies. Let’s get started! 00:46 Lois: Hello and welcome to the Oracle University Podcast. I’m Lois Houston, Director of Innovation Programs with Oracle University, and with me is Nikita Abraham, Principal Technical Editor. Nikita: Hi everyone! In our last episode, we went over the basics of deep learning. Today, we’ll look at generative AI and large language models, and discuss how they work. To help us with that, we have Himanshu Raj, Senior Instructor on AI/ML. So, let’s jump right in. Hi Himanshu, what is generative AI? 01:21 Himanshu: Generative AI refers to a type of AI that can create new content. It is a subset of deep learning, where the models are trained not to make predictions but rather to generate output on their own. Think of generative AI as an artist who looks at a lot of paintings and learns the patterns and styles present in them. Once it has learned these patterns, it can generate new paintings that resembles what it learned. 01:48 Lois: Let's take an example to understand this better. Suppose we want to train a generative AI model to draw a dog. How would we achieve this? Himanshu: You would start by giving it a lot of pictures of dogs to learn from. The AI does not know anything about what a dog looks like. But by looking at these pictures, it starts to figure out common patterns and features, like dogs often have pointy ears, narrow faces, whiskers, etc. You can then ask it to draw a new picture of a dog. The AI will use the patterns it learned to generate a picture that hopefully looks like a dog. But remember, the AI is not copying any of the pictures it has seen before but creating a new image based on the patterns it has learned. This is the basic idea behind generative AI. In practice, the process involves a lot of complex maths and computation, and there are different techniques and architectures that can be used, such as variational autoencoders (VAs) and Generative Adversarial Networks (GANs). 02:48 Nikita: Himanshu, where is generative AI used in the real world? Himanshu: Generative AI models have a wide variety of applications across numerous domains. For the image generation, generative models like GANs are used to generate realistic images. They can be used for tasks, like creating artwork, synthesizing images of human faces, or transforming sketches into photorealistic images. For text generation, large language models like GPT 3, which are generative in nature, can create human-like text. This has applications in content creation, like writing articles, generating ideas, and again, conversational AI, like chat bots, customer service agents. They are also used in programming for code generation and debugging, and much more. For music generation, generative AI models can also be used. They create new pieces of music after being trained on a specific style or collection of tunes. A famous example is OpenAI's MuseNet. 03:42 Lois: You mentioned large language models in the context of text-based generative AI. So, let’s talk a little more about it. Himanshu, what exactly are large language models? Himanshu: LLMs are a type of artificial intelligence models built to understand, generate, and process human language at a massive scale. They were primarily designed for sequence to sequence tasks such as machine translation, where an input sequence is transformed into an output sequence. LLMs can be used to translate text from one language to another. For example, an LLM could be used to translate English text into French. To do this job, LLM is trained on a massive data set of text and code which allows it to learn the patterns and relationships that exist between different languages. The LLM translates, “How are you?” from English to French, “Comment allez-vous?” It can also answer questions like, what is the capital of France? And it would answer the capital of France is Paris. And it will write an essay on a given topic. For example, write an essay on French Revolution, and it will come up with a response like with a title and introduction. 04:53 Lois: And how do LLMs actually work? Himanshu: So, LLM models are typically based on deep learning architectures such as transformers. They are also trained on vast amount of text data to learn language patterns and relationships, again, with a massive number of parameters usually in order of millions or even billions. LLMs have also the ability to comprehend and understand natural language text at a semantic level. They can grasp context, infer meaning, and identify relationships between words and phrases. 05:26 Nikita: What are the most important factors for a large language model? Himanshu: Model size and parameters are crucial aspects of large language models and other deep learning models. They significantly impact the model’s capabilities, performance, and resource requirement. So, what is model size? The model size refers to the amount of memory required to store the model's parameter and other data structures. Larger model sizes generally led to better performance as they can capture more complex patterns and representation from the data. The parameters are the numerical values of the model that change as it learns to minimize the model's error on the given task. In the context of LLMs, parameters refer to the weights and biases of the model's transformer layers. Parameters are usually measured in terms of millions or billions. For example, GPT-3, one of the largest LLMs to date, has 175 billion parameters making it extremely powerful in language understanding and generation. Tokens represent the individual units into which a piece of text is divided during the processing by the model. In natural language, tokens are usually words, subwords, or characters. Some models have a maximum token limit that they can process and longer text can may require truncation or splitting. Again, balancing model size, parameters, and token handling is crucial when working with LLMs. 06:49 Nikita: But what’s so great about LLMs? Himanshu: Large language models can understand and interpret human language more accurately and contextually. They can comprehend complex sentence structures, nuances, and word meanings, enabling them to provide more accurate and relevant responses to user queries. This model can generate human-like text that is coherent and contextually appropriate. This capability is valuable for context creation, automated writing, and generating personalized response in applications like chatbots and virtual assistants. They can perform a variety of tasks. Large language models are very versatile and adaptable to various industries. They can be customized to excel in applications such as language translation, sentiment analysis, code generation, and much more. LLMs can handle multiple languages making them valuable for cross-lingual tasks like translation, sentiment analysis, and understanding diverse global content. Large language models can be again, fine-tuned for a specific task using a minimal amount of domain data. The efficiency of LLMs usually grows with more data and parameters. 07:55 Lois: You mentioned the “sequence to sequence tasks” earlier. Can you explain the concept in simple terms for us? Himanshu: Understanding language is difficult for computers and AI systems. The reason being that words often have meanings based on context. Consider a sentence such as Jane threw the frisbee, and her dog fetched it. In this sentence, there are a few things that relate to each other. Jane is doing the throwing. The dog is doing the fetching. And it refers to the frisbee. Suppose we are looking at the word “it” in the sentence. As a human, we understand easily that “it” refers to the frisbee. But for a machine, it can be tricky. The goal in sequence problems is to find patterns, dependencies, or relationships within the data and make predictions, classification, or generate new sequences based on that understanding. 08:48 Lois: And where are sequence models mostly used? Himanshu: Some common example of sequence models includes natural language processing, which we call NLP, tasks such as machine translation, text generation sentiment analysis, language modeling involve dealing with sequences of words or characters. Speech recognition. Converting audio signals into text, involves working with sequences of phonemes or subword units to recognize spoken words. Music generation. Generating new music involves modeling musical sequences, nodes, and rhythms to create original compositions. Gesture recognition. Sequences of motion or hand gestures are used to interpret human movements for applications, s
Did you know that the concept of deep learning goes way back to the 1950s? However, it is only in recent years that this technology has created a tremendous amount of buzz (and for good reason!). A subset of machine learning, deep learning is inspired by the structure of the human brain, making it fascinating to learn about. In this episode, Lois Houston and Nikita Abraham interview Senior Principal OCI Instructor Hemant Gahankari about deep learning concepts, including how Convolution Neural Networks work, and help you get your deep learning basics right. Oracle MyLearn: https://mylearn.oracle.com/ou/learning-path/become-an-oci-ai-foundations-associate-2023/127177 Oracle University Learning Community: https://education.oracle.com/ou-community LinkedIn: https://www.linkedin.com/showcase/oracle-university/ X (formerly Twitter): https://twitter.com/Oracle_Edu Special thanks to Arijit Ghosh, David Wright, Himanshu Raj, and the OU Studio Team for helping us create this episode. -------------------------------------------------------- Episode Transcript: 00:00 The world of artificial intelligence is vast and everchanging. And with all the buzz around it lately, we figured it was the perfect time to revisit our AI Made Easy series. Join us over the next few weeks as we chat about all things AI, helping you to discover its endless possibilities. Ready to dive in? Let’s go! 00:33 Welcome to the Oracle University Podcast, the first stop on your cloud journey. During this series of informative podcasts, we’ll bring you foundational training on the most popular Oracle technologies. Let’s get started! 00:47 Lois: Hello and welcome to the Oracle University Podcast. I’m Lois Houston, Director of Innovation Programs with Oracle University, and with me is Nikita Abraham, Principal Technical Editor. Nikita: Hi everyone! Lois: Today, we’re going to focus on the basics of deep learning with our Senior Principal OCI Instructor, Hemant Gahankari. Nikita: Hi Hemant! Thanks for being with us today. So, to get started, what is deep learning? 01:14 Hemant: Hi Niki and hi Lois. So, deep learning is a subset of machine learning that focuses on training Artificial Neural Networks, abbreviated as ANN, to solve a task at hand. Say, for example, image classification. A very important quality of the ANN is that it can process raw data like pixels of an image and extract patterns from it. These patterns are treated as features to predict the outcomes. Let us say we have a set of handwritten images of digits 0 to 9. As we know, everyone writes the digits in a slightly different way. So how do we train a machine to identify a handwritten digit? For this, we use ANN. ANN accepts image pixels as inputs, extracts patterns like edges and curves and so on, and correlates these patterns to predict an outcome. That is what digit does the image has in this case. 02:17 Lois: Ok, so what you’re saying is given a bunch of pixels, ANN is able to process pixel data, learn an internal representation of the data, and predict outcomes. That’s so cool! So, why do we need deep learning? Hemant: We need to specify features while we train machine learning algorithm. With deep learning, features are automatically extracted from the data. Internal representation of features and their combinations is built to predict outcomes by deep learning algorithms. This may not be feasible manually. Deep learning algorithms can make use of parallel computations. For this, usually data is split into small batches and processed parallelly. So these algorithms can process large amount of data in a short time to learn the features and their combinations. This leads to scalability and performance. In short, deep learning complements machine learning algorithms for complex data for which features cannot be described easily. 03:21 Nikita: What can you tell us about the origins of deep learning? Hemant: Some of the deep learning concepts like artificial neuron, perceptron, and multilayer perceptron existed as early as 1950s. One of the most important concept of using backpropagation for training ANN came in 1980s. In 1990s, convolutional neural networks were also introduced for image analysis tasks. Starting 2000, GPUs were introduced. And 2010 onwards, GPUs became cheaper and widely available. This fueled the widespread adoption of deep learning uses like computer vision, natural language processing, speech recognition, text translation, and so on. In 2012, major networks like AlexNet and Deep-Q Network were built. 2016 onward, generative use cases of the deep learning also started to come up. Today, we have widely adopted deep learning for a variety of use cases, including large language models and many other types of generative models. 04:32 Lois: Hemant, what are various applications of deep learning algorithms? Hemant: Deep learning algorithms are targeted at a variety of data and applications. For data, we have images, videos, text, and audio. For images, applications can be image classification, object detection, and segmentation. For textual data, applications are to translate the text or detect a sentiment of a text. For audio, the applications can be music generation, speech to text, and so on. 05:05 Lois: It's important that we select the right deep learning algorithm based on the data and application, right? So how do we do that? Hemant: For image tasks like image classification, object detection, image segmentation, or facial recognition, CNN is a suitable architecture. For text, we have a choice of the latest transformers or LSTM or even RNN. For generative tasks like text summarization or question answering, transformers is a good choice. For generating images, text to image generation, transformers, GANs, or diffusion models are available choices. 05:45 Nikita: Let’s dive a little deeper into Artificial Neural Networks. Can you tell us more about them, Hemant? Hemant: Artificial Neural Networks are inspired by the human brain. They are made up of interconnected nodes called as neurons. Nikita: And how are inputs processed by a neuron? Hemant: In ANN, we assign weights to the connection between neurons. Weighted inputs are added up. And if the sum crosses a specified threshold, the neuron is fired. And the outputs of a layer of neuron become an input to another layer. 06:16 Lois: Hemant, tell us about the building blocks of ANN so we understand this better. Hemant: So first building block is layers. We have input layer, output layer, and multiple hidden layers. The input layer and output layer are mandatory. And the hidden layers are optional. The layers consist of neurons. Neurons are computational units, which accept an input and produce an output. Weights determine the strength of connection between neurons. So the connections could be between input and a neuron, or it could be between a neuron and another neuron. Activation functions work on the weighted sum of inputs to the neuron and produce an output. Additional input to the neuron that allows a certain degree of flexibility is called as a bias. 07:05 Nikita: I think we’ve got the components of ANN straight but maybe you should give us an example. You mentioned this example earlier…of needing to train ANN to recognize handwritten digits from images. How would we go about that? Hemant: For that, we have to collect a large number of digit images, and we need to train ANN using these images. So, in this case, the images consist of 28 by 28 pixels, which act as input layer. For the output, we have 10 neurons which represent digits 0 to 9. And we have multiple hidden layers. So, for example, we have two hidden layers which are consisting of 16 neurons each. The hidden layers are responsible for capturing the internal representation of the raw images. And the output layer is responsible for producing the desired outcomes. So, in this case, the desired outcome is the prediction of whether the digit is 0 or 1 or up to digit 9. So how do we train this particular ANN? So the first thing we use is the backpropagation algorithm. During training, we show an image to the ANN. Let’s say it is an image of digit 2. So we expect output neuron for digit 2 to fire. But in real, let’s say output neuron of a digit 6 fired. 08:28 Lois: So, then, what do we do? Hemant: We know that there is an error. So we correct an error. We adjust the weights of the connection between neurons based on a calculation, which we call as backpropagation algorithm. By showing thousands of images and adjusting the weights iteratively, ANN is able to predict correct outcomes for most of the input images. This process of adjusting weights through backpropagation is called as model training. 09:01 Do you have an idea for a new course or learning opportunity? We’d love to hear it! Visit the Oracle University Learning Community and share your thoughts with us on the Idea Incubator. Your suggestion could find a place in future development projects! Visit mylearn.oracle.com to get started. 09:22 Nikita: Welcome back! Let’s move on to CNN. Hemant, what is a Convolutional Neural Network? Hemant: CNN is a type of deep learning model specifically designed for processing and analyzing grid-like data, such as images and videos. In the ANN, the input image is converted to a single dimensional array and given as an input to the network. But that does not work well with the image data because image data is inherently two dimensional. CNN works better with the two dimensional data. The role of the CNN is to reduce the images into a form, which is easier to process and without losing features, which are critical for getting a good prediction. 10:10 Lois: A CNN has different layers, right? Could you tell us a bit about them? Hemant: The first one is input layer. Input layer is followed by feature extraction layers, which is a combination and repetition of convolutional layer with ReLu activation and a pooling layer. And this i
Does machine learning feel like too convoluted a topic? Not anymore! Listen to hosts Lois Houston and Nikita Abraham, along with Senior Principal OCI Instructor Hemant Gahankari, talk about foundational machine learning concepts and dive into how supervised learning, unsupervised learning, and reinforcement learning work. Oracle MyLearn: https://mylearn.oracle.com/ou/learning-path/become-an-oci-ai-foundations-associate-2023/127177 Oracle University Learning Community: https://education.oracle.com/ou-community LinkedIn: https://www.linkedin.com/showcase/oracle-university/ X (formerly Twitter): https://twitter.com/Oracle_Edu Special thanks to Arijit Ghosh, David Wright, Himanshu Raj, and the OU Studio Team for helping us create this episode. -------------------------------------------------------- Episode Transcript: 00:00 The world of artificial intelligence is vast and everchanging. And with all the buzz around it lately, we figured it was the perfect time to revisit our AI Made Easy series. Join us over the next few weeks as we chat about all things AI, helping you to discover its endless possibilities. Ready to dive in? Let’s go! 00:33 Welcome to the Oracle University Podcast, the first stop on your cloud journey. During this series of informative podcasts, we’ll bring you foundational training on the most popular Oracle technologies. Let’s get started! 00:47 Lois: Hello and welcome to the Oracle University Podcast. I’m Lois Houston, Director of Innovation Programs with Oracle University, and with me is Nikita Abraham, Principal Technical Editor. Nikita: Hi everyone! Last week, we went through the basics of artificial intelligence and we’re going to take it a step further today by talking about some foundational machine learning concepts. After that, we’ll discuss the three main types of machine learning models: supervised learning, unsupervised learning, and reinforcement learning. 01:18 Lois: Hemant Gahankari, a Senior Principal OCI Instructor, joins us for this episode. Hi Hemant! Let’s dive right in. What is machine learning? How does it work? Hemant: Machine learning is a subset of artificial intelligence that focuses on creating computer systems that can learn and predict outcomes from given examples without being explicitly programmed. It is powered by algorithms that incorporate intelligence into machines by automatically learning from a set of examples usually provided as data. 01:54 Nikita: Give us a few examples of machine learning… so we can see what it can do for us. Hemant: Machine learning is used by all of us in our day-to-day life. When we shop online, we get product recommendations based on our preferences and our shopping history. This is powered by machine learning. We are notified about movies recommendations based on our viewing history and choices of other similar viewers. This too is driven by machine learning. While browsing emails, we are warned of a spam mail because machine learning classifies whether the mail is spam or not based on its content. In the increasingly popular self-driving cars, machine learning is responsible for taking the car to its destination. 02:45 Lois: So, how does machine learning actually work? Hemant: Let us say we have a computer and we need to teach the computer to differentiate between a cat and a dog. We do this by describing features of a cat or a dog. Dogs and cats have distinguishing features. For example, the body color, texture, eye color are some of the defining features which can be used to differentiate a cat from a dog. These are collectively called as input data. We also provide a corresponding output, which is called as a label, which can be a dog or a cat in this case. By describing a specific set of features, we can say that it is a cat or a dog. Machine learning model is first trained with the data set. Training data set consists of a set of features and output labels, and is given as an input to the machine learning model. During the process of training, machine learning model learns the relation between input features and corresponding output labels from the provided data. Once the model learns from the data, we have a trained model. Once the model is trained, it can be used for inference. Inference is a process of getting a prediction by giving a data point. In this example, we input features of a cat or a dog, and the trained model predicts the output that is a cat or a dog label. The types of machine learning models depend on whether we have a labeled output or not. 04:28 Nikita: Oh, there are different types of machine learning models? Hemant: In general, there are three types of machine learning approaches. In supervised machine learning, labeled data is used to train the model. Model learns the relation between features and labels. Unsupervised learning is generally used to understand relationships within a data set. Labels are not used or are not available. Reinforcement learning uses algorithms that learn from outcomes to make decisions or choices. 05:06 Lois: Ok…supervised learning, unsupervised learning, and reinforcement learning. Where do we use each of these machine learning models? Hemant: Some of the popular applications of supervised machine learning are disease detection, weather forecasting, stock price prediction, spam detection, and credit scoring. For example, in disease detection, the patient data is input to a machine learning model, and machine learning model predicts if a patient is suffering from a disease or not. For unsupervised machine learning, some of the most common real-time applications are to detect fraudulent transactions, customer segmentation, outlier detection, and targeted marketing campaigns. So for example, given the transaction data, we can look for patterns that lead to fraudulent transactions. Most popular among reinforcement learning applications are automated robots, autonomous driving cars, and playing games. 06:12 Nikita: I want to get into how each type of machine learning works. Can we start with supervised learning? Hemant: Supervised learning is a machine learning model that learns from labeled data. The model learns the mapping between the input and the output. As a house price predictor model, we input house size in square feet and model predicts the price of a house. Suppose we need to develop a machine learning model for detecting cancer, the input to the model would be the person's medical details, the output would be whether the tumor is malignant or not. 06:50 Lois: So, that mapping between the input and output is fundamental in supervised learning. Hemant: Supervised learning is similar to a teacher teaching student. The model is trained with the past outcomes and it learns the relationship or mapping between the input and output. In supervised machine learning model, the outputs can be either categorical or continuous. When the output is continuous, we use regression. And when the output is categorical, we use classification. 07:26 Lois: We want to keep this discussion at a high level, so we’re not going to get into regression and classification. But if you want to learn more about these concepts and look at some demonstrations, visit mylearn.oracle.com. Nikita: Yeah, look for the Oracle Cloud Infrastructure AI Foundations course and you’ll find a lot of resources that you can make use of. 07:51 The Oracle University Learning Community is an excellent place to collaborate and learn with Oracle experts and fellow learners. Grow your skills, inspire innovation, and celebrate your successes. All your activities, from liking a post to answering questions and sharing with others, will help you earn a valuable reputation, badges, and ranks to be recognized in the community. Visit mylearn.oracle.com to get started. 08:19 Nikita: Welcome back! So that was supervised machine learning. What about unsupervised machine learning, Hemant? Hemant: Unsupervised machine learning is a type of machine learning where there are no labeled outputs. The algorithm learns the patterns and relationships in the data and groups similar data items. In unsupervised machine learning, the patterns in the data are explored explicitly without being told what to look for. For example, if you give a set of different-colored LEGO pieces to a child and ask to sort it, it may the LEGO pieces based on any patterns they observe. It could be based on same color or same size or same type. Similarly, in unsupervised learning, we group unlabeled data sets. One more example could be-- say, imagine you have a basket of various fruits-- say, apples, bananas, and oranges-- and your task is to group these fruits based on their similarities. You observe that some fruits are round and red, while others are elongated and yellow. Without being told explicitly, you decide to group the round and red fruits together as one cluster and the elongated and yellow fruits as another cluster. There you go. You have just performed an unsupervised learning task. 09:42 Lois: Where is unsupervised machine learning used? Can you take us through some use cases? Hemant: The first use case of unsupervised machine learning is market segmentation. In market segmentation, one example is providing the purchasing details of an online shop to a clustering algorithm. Based on the items purchased and purchasing behavior, the clustering algorithm can identify customers based on the similarity between the products purchased. For example, customers with a particular age group who buy protein diet products can be shown an advertisement of sports-related products. The second use case is on outlier analysis. One typical example for outlier analysis is to provide credit card purchase data for clustering. Fraudulent transactions can be detected by a bank by using outliers. In some transaction, amounts are too high or recurring. It signifies an outlier. The third use case is recommendation systems. An example f
You probably interact with artificial intelligence (AI) more than you realize. So, there’s never been a better time to start figuring out how it all works. Join Lois Houston and Nikita Abraham as they decode the fundamentals of AI so that anyone, irrespective of their technical background, can leverage the benefits of AI and tap into its infinite potential. Together with Senior Cloud Engineer Nick Commisso, they take you through key AI concepts, common AI tasks and domains, and the primary differences between AI, machine learning, and deep learning. Oracle MyLearn: https://mylearn.oracle.com/ou/learning-path/become-an-oci-ai-foundations-associate-2023/127177 Oracle University Learning Community: https://education.oracle.com/ou-community LinkedIn: https://www.linkedin.com/showcase/oracle-university/ X (formerly Twitter): https://twitter.com/Oracle_Edu Special thanks to Arijit Ghosh, David Wright, Himanshu Raj, and the OU Studio Team for helping us create this episode. -------------------------------------------------------- Episode Transcript: 00:00 The world of artificial intelligence is vast and everchanging. And with all the buzz around it lately, we figured it was the perfect time to revisit our AI Made Easy series. Join us over the next few weeks as we chat about all things AI, helping you to discover its endless possibilities. Ready to dive in? Let’s go! 00:33 Welcome to the Oracle University Podcast, the first stop on your cloud journey. During this series of informative podcasts, we’ll bring you foundational training on the most popular Oracle technologies. Let’s get started! 00:46 Nikita: Hello and welcome to the Oracle University Podcast. I’m Nikita Abraham, Principal Technical Editor with Oracle University, and with me is Lois Houston, Director of Innovation Programs. Lois: Hi there! Welcome to a new season of the Oracle University Podcast. I’m so excited about this season because we’re going to delve into the world of artificial intelligence. In upcoming episodes, we’ll talk about the fundamentals of artificial intelligence and machine learning. And we’ll discuss neural network architectures, generative AI and large language models, the OCI AI stack, and OCI AI services. 01:27 Nikita: So, if you’re an IT professional who wants to start learning about AI and ML or even if you’re a student who is familiar with OCI or similar cloud services, but have no prior exposure to this field, you’ll want to tune in to these episodes. Lois: That’s right, Niki. So, let’s get started. Today, we’ll talk about the basics of artificial intelligence with Senior Cloud Engineer Nick Commisso. Hi Nick! Thanks for joining us today. So, let’s start right at the beginning. What is artificial intelligence? 01:57 Nick: Well, the ability of machines to imitate the cognitive abilities and problem solving capabilities of human intelligence can be classified as artificial intelligence or AI. 02:08 Nikita: Now, when you say capabilities and abilities, what are you referring to? Nick: Human intelligence is the intellectual capability of humans that allows us to learn new skills through observation and mental digestion, to think through and understand abstract concepts and apply reasoning, to communicate using a language and understand the nonverbal cues, such as facial recognition, tone variation, and body language. You can handle objections in real time, even in a complex setting. You can plan for short and long-term situations or projects. And, of course, you can create music and art or invent something new like an original idea. If you can replicate any of these human capabilities in machines, this is artificial general intelligence or AGI. So in other words, AGI can mimic human sensory and motor skills, performance, learning, and intelligence, and use these abilities to carry out complicated tasks without human intervention. When we apply AGI to solve problems with specific and narrow objectives, we call it artificial intelligence or AI. 03:16 Lois: It seems like AI is everywhere, Nick. Can you give us some examples of where AI is used? Nick: AI is all around us, and you've probably interacted with AI, even if you didn't realize it. Some examples of AI can be viewing an image or an object and identifying if that is an apple or an orange. It could be examining an email and classifying it spam or not. It could be writing computer language code or predicting the price of an older car. So let's get into some more specifics of AI tasks and the nature of related data. Machine learning, deep learning, and data science are all associated with AI, and it can be confusing to distinguish. 03:57 Nikita: Why do we need AI? Why’s it important? Nick: AI is vital in today's world, and with the amount of data that's generated, it far exceeds the human ability to absorb, interpret, and actually make decisions based on that data. That's where AI comes in handy by enhancing the speed and effectiveness of human efforts. So here are two major reasons why we need AI. Number one, we want to eliminate or reduce the amount of routine tasks, and businesses have a lot of routine tasks that need to be done in large numbers. So things like approving a credit card or a bank loan, processing an insurance claim, recommending products to customers are just some example of routine tasks that can be handled. And second, we, as humans, need a smart friend who can create stories and poems, designs, create code and music, and have humor, just like us. 04:54 Lois: I’m onboard with getting help from a smart friend! There are different domains in AI, right, Nick? Nick: We have language for language translation; vision, like image classification; speech, like text to speech; product recommendations that can help you cross-sell products; anomaly detection, like detecting fraudulent transactions; learning by reward, like self-driven cars. You have forecasting with weather forecasting. And, of course, generating content like image from text. 05:24 Lois: There are so many applications. Nick, can you tell us more about these commonly used AI domains like language, audio, speech, and vision? Nick: Language-related AI tasks can be text related or generative AI. Text-related AI tasks use text as input, and the output can vary depending on the task. Some examples include detecting language, extracting entities in a text, or extracting key phrases and so on. Consider the example of translating text. There's many text translation tools where you simply type or paste your text into a given text box, choose your source and target language, and then click translate. Now, let's look at the generative AI tasks. They are generative, which means the output text is generated by a model. Some examples are creating text like stories or poems, summarizing a text, answering questions, and so on. Let's take the example of ChatGPT, the most well-known generative chat bot. These bots can create responses from their training on large language models, and they continuously grow through machine learning. 06:31 Nikita: What can you tell us about using text as data? Nick: Text is inherently sequential, and text consists of sentences. Sentences can have multiple words, and those words need to be converted to numbers for it to be used to train language models. This is called tokenization. Now, the length of sentences can vary, and all the sentences lengths need to be made equal. This is done through padding. Words can have similarities with other words, and sentences can also be similar to other sentences. The similarity can be measured through dot similarity or cosine similarity. We need a way to indicate that similar words or sentences may be close by. This is done through representation called embedding. 07:17 Nikita: And what about language AI models? Nick: Language AI models refer to artificial intelligence models that are specifically designed to understand, process, and generate natural language. These models have been trained on vast amounts of textual data that can perform various natural language processing or NLP tasks. The task that needs to be performed decides the type of input and output. The deep learning model architectures that are typically used to train models that perform language tasks are recurrent neural networks, which processes data sequentially and stores hidden states, long short-term memory, which processes data sequentially that can retain the context better through the use of gates, and transformers, which processes data in parallel. It uses the concept of self-attention to better understand the context. 08:09 Lois: And then there’s speech-related AI, right? Nick: Speech-related AI tasks can be either audio related or generative AI. Speech-related AI tasks use audio or speech as input, and the output can vary depending on the task. For example, speech-to-text conversion or speaker recognition, voice conversion, and so on. Generative AI tasks are generative in nature, so the output audio is generated by a model. For example, you have music composition and speech synthesis. Audio or speech is digitized as snapshots taken in time. The sample rate is the number of times in a second an audio sample is taken. Most digital audio have a sampling rate of 44.1 kilohertz, which is also the sampling rate for audio CDs. Multiple samples need to be correlated to make sense of the data. For example, listening to a song for a fraction of a second, you won't be able to infer much about the song, and you'll probably need to listen to it a little bit longer. Audio and speech AI models are designed to process and understand audio data, including spoken language. These deep-learning model architectures are used to train models that perform language with tasks-- recurrent neural networks, long short-term memory, transformers, variational autoencoders, waveform models, and Siamese networks. All of the models take into consideration the sequential natu
Visual Builder Studio requires its data sources to connect to the webpage it produces using REST calls. Therefore, the data source has to provide a REST interface. A simple, easy, secure, and free way to do that is with Oracle REST Data Services (ORDS). In this episode, hosts Lois Houston and Nikita Abraham chat with Senior Principal OCI Instructor Joe Greenwald about what ORDS can do, how to easily set it up, how to work with it, and how to use it within Visual Builder Studio. Develop Fusion Applications Using Visual Builder Studio: https://mylearn.oracle.com/ou/course/develop-fusion-applications-using-visual-builder-studio/122614/ Build Visual Applications Using Visual Builder Studio: https://mylearn.oracle.com/ou/course/build-visual-applications-using-oracle-visual-builder-studio/110035/ Oracle University Learning Community: https://education.oracle.com/ou-community LinkedIn: https://www.linkedin.com/showcase/oracle-university/ X (formerly Twitter): https://twitter.com/Oracle_Edu Special thanks to Arijit Ghosh, David Wright, and the OU Studio Team for helping us create this episode. -------------------------------------------------------- Episode Transcript: 00:00 Welcome to the Oracle University Podcast, the first stop on your cloud journey. During this series of informative podcasts, we’ll bring you foundational training on the most popular Oracle technologies. Let’s get started. 00:26 Nikita: Hello and welcome to the Oracle University Podcast! I’m Nikita Abraham, Principal Technical Editor with Oracle University, and with me is Lois Houston, Director of Innovation Programs. Lois: Hi there! In our last episode, we took a look at model-based development tools, their start as CASE tools, what they morphed into, and how they’re currently used in Oracle software development. We’re wrapping up the season with this episode today, which will be about how to access Oracle database data through a REST interface created and managed by Oracle REST Data Services, or ORDS, and how to access this data in Visual Builder Studio. 01:03 Nikita: Being able to access Oracle database data through a REST interface over the web is highly useful, but sometimes it can be complicated to create that interface in a programming language. Joe Greenwald, our Senior OCI Learning Solutions Architect and Principal Instructor is back with us one last time this season to tell us more about ORDS, and how it makes it much simpler and easier for us to REST-enable our database for use in tools like Visual Builder Studio. Hi Joe! Tell us a little about what Visual Builder Studio is and why we must REST-enable our data for VBS to be able to use it. 01:40 Joe: Hi Niki, hi Lois! Ok, so, Visual Builder Studio is Oracle’s low-code software development and project asset management product for creating graphical webpage front-ends for web applications. It’s the tool of choice for designing, building, and implementing all of Oracle Fusion Cloud Applications and is being used by literally tens of thousands of engineers at Oracle now to bring the next generation of Fusion Applications to our customers and the market. It’s based on standards like HTML5, CSS3, and JavaScript. It’s highly performant and combined with the Redwood graphical design system and components that we talked about previously, delivers a world-class experience for users. One thing about Visual Builder Studio though: it only works with data sources that have a REST interface. This is unusual. I like to think I’ve worked with every software development tool that Oracle’s created since I joined Oracle in 1992, including some unreleased ones, and all of them allowed you to talk to the database directly. This is the first time that we’ve released a tool that I know of where we don’t do that. Now at first, I was a little put off and wondered how’s it going to do this and how much work I would have to do to create a REST interface for some simple tables in the Oracle database. Like, here’s one more thing I must do just to create a page that displays data from the database. As it turns out, it’s a wise design decision on the part of the designers. It simplifies the data access parts of Visual Builder Studio and makes the data access model common across the different data sources. And, thanks to ORDS, REST-enabling data in Oracle database couldn’t be easier! 03:13 Lois: That’s cool. We don’t want to focus too much on Visual Builder Studio today. We have free courses that teach you how to create service connections to REST services to access the data and all of that. What we actually want to talk with you about is working with Oracle REST Data Service. How easy is it to work with Oracle REST Data Service to add REST support, what we call REST-enable your Oracle Database, and why it is important? Nikita: Yeah, I could use a bit of a refresher on REST myself. Could you describe what REST is, how it works for both the client and server, and what ORDS is doing for us? 03:50 Joe: Sure. So, REST is a way to make a request to a server for a resource using the HTTP web protocol from a client, like your browser, to a web server, which hands off the request to code that handles the request and sends the response back to your client/browser, which then uses it, displays or whatever. So, you can see we have two parts. We have the client, which makes the request, and the server, which handles the request and figures out what the response should be (static, dynamic, or a combination of both) and sends that back to the client. For example, a visual application built with Visual Builder Studio acts as a client making the request, just as your browser makes a request. It’s really just a web app built with HTML5, CSS3, and JavaScript, and the JavaScript makes a request to the server on your behalf. Let’s say you wish to access your student record within Oracle University. And now, this is a contrived example and it won’t actually work, but it’s good for illustrative purposes. Oracle University, let’s say, publishes the URL for your student data as something like https://oracle.com/oracleuniversity/student/{studentnumber} you put in some kind of number, like the number 23, and if you enter that into your browser address bar and press Enter, then your browser, on your behalf, sends a GET request—what we call an HTTP GET operation—to the web server. When the web server receives the request, it will somehow read the record for student 23, format a response, and send the response back to the client. 05:16 Joe: That’s a GET or a READ request. Now, what if you are creating a new student? Well, you fill out a form on the webpage and you click the Submit button. And it sends a POST request, which tells the server to create a new record in its storage mechanism, most likely a database of some form. If you do an update, you change certain fields on the webpage and click the Submit button and this time, an update request is made. If you wanted to delete the record, you’d find the record you want to delete and press the Submit button, and this time a delete request is made. This is the general idea, though there are different ways to do creates and updates that are really irrelevant here. Those requests to the server I mentioned are called HTTP operations and there are several of them. But the four most popular are GET to retrieve data, POST to create a new record on the server, PUT to update a record, and DELETE to remove a record. On the client side, we just need to specify where the record is that we want to retrieve—that’s the oracle.com/oracleuniversity/student part of the URL and an identifying value, which makes it unique. So, when I do a GET request on customer or student 23, I’m going to get back a representation of the student data that exists in the student database for a student with ID 23. There should not be more than one of these or that would indicate an error. The response typically comes back in a format of a key:value pair called JavaScript Object Notation (JSON), but it could also be in a Text format, HTML, Excel, PDF, or whatever the server implements and is requested. 06:42 Nikita: OK great! That’s on the client side making the request. But what’s happening on the server side? Do I need to worry about that if I’m the client? Joe: No, that’s the great part. As a client, I don’t know, and frankly I’d rather not know what the server’s doing or how it does it. I don’t want to be dependent on the server implementation at all. I simply want to make a request and the server handles the request and sends a response. Now, just a word about what’s on the server. Some data on the server is static like a PDF file or an image or an audio file, for example, and sometimes you’ll see that in the URL the file type as an extension, like .pdf, and you get back a PDF file that your browser displays or that you can download to your machine. But with dynamic data, like student data coming out of a database based on the student number, a query is made against a database. The database responds with the data, and that’s formatted into some type of data format—typically JSON—and sent back to the client, which then does something with it, like displaying it on a webpage. So, as we can see, the client is fairly simple in the sense that it makes a request, receives the data response, and displays it or does something with it. And that’s one of the reasons why the choice to use REST and only REST in Visual Builder Studio is such a wise one. 07:54 Joe: Regardless of the different data sources or the different server implementations or how the data is stored on the server, or any of that, Visual Builder Studio doesn’t know and doesn’t care. What it sees is the REST request it sends and the response it gets back and then it deals with the response data regardless of how it’s implemented on the server. I mentioned the server sends back a representation of the resource, in this case, for example, the stud
Computer Aided Software Engineering (CASE) tools, which helped make the analysis, design, and implementation phases of software development better, faster, and cheaper, fell out of favor in the mid-'90s. Yet much of what they have to offer remains and is in active use within different Oracle tools. Listen to Lois Houston and Nikita Abraham interview Senior Principal OCI Instructor Joe Greenwald about the origins of CASE tools and model-based development, as well as how they evolved into their current forms. Develop Fusion Applications Using Visual Builder Studio: https://mylearn.oracle.com/ou/course/develop-fusion-applications-using-visual-builder-studio/122614/ Build Visual Applications Using Visual Builder Studio: https://mylearn.oracle.com/ou/course/build-visual-applications-using-oracle-visual-builder-studio/110035/ Oracle University Learning Community: https://education.oracle.com/ou-community LinkedIn: https://www.linkedin.com/showcase/oracle-university/ X (formerly Twitter): https://twitter.com/Oracle_Edu Special thanks to Arijit Ghosh, David Wright, and the OU Studio Team for helping us create this episode. --------------------------------------------------------- Episode Transcript: 00:00 Welcome to the Oracle University Podcast, the first stop on your cloud journey. During this series of informative podcasts, we’ll bring you foundational training on the most popular Oracle technologies. Let’s get started. 00:26 Nikita: Hello and welcome to the Oracle University Podcast! I’m Nikita Abraham, Principal Technical Editor with Oracle University, and joining me is Lois Houston, Director of Innovation Programs. Lois: Hi there! In our last episode, we looked at Oracle’s Redwood design system and how it helps create world-class apps and user experiences. Today, Joe Greenwald, our Senior Principal OCI Instructor, is back on our podcast. We’re going to focus on where model-based development tools came from: their start as CASE tools, how they morphed into today’s model-based development tools, and how these tools are currently used in Oracle software development to make developers’ lives better. 01:08 Nikita: That’s right. It’s funny how things that fell out of favor years ago come back and are used to support our app development efforts today. Hi Joe! Joe: Haha! Hi Niki. Hi Lois.
01:18 Lois: Joe, how did you get started with CASE tools? Joe: I was first introduced to computer-aided software engineering tools, called CASE tools, in the late 1980s when I began working with them at Arthur Young consulting and then Knowledgeware corporation in Atlanta, helping customers improve and even automate their software development efforts using structured analysis and design techniques, which were popular and in high use at that time. But it was a pain to have to draw diagrams by hand, redraw them as specifications changed, and then try to maintain them to represent the changes in understanding what we were getting from our analysis and design phase work. CASE tools were used to help us draw the pictures as well as enforce rules and provide a repository so we could share what we were creating with other developers. I was immediately attracted to the idea of using diagrams and graphical images to represent requirements for computer systems. 02:08 Lois: Yeah, you’re like me. You’re a visual person. Joe: Yes, exactly. So, the idea that I could draw a picture and a computer could turn that into executable code was fascinating to me. Pictures helped us understand what the analysts told us the users wanted, and helped us communicate amongst the teams, and they also helped us validate our understanding with our users. This was a critical aspect because there was a fundamental cognitive disconnect between what the users told the analysts they needed, what the analysts told us the users needed, and what we understood was needed, and what the user actually wanted. There’s a famous cartoon, you can probably find this on the web, that shows what the users wanted, what was delivered, and then all the iterations that the different teams go through trying to represent the simple original request. I started using entity relationship diagrams, data flow diagrams, and structure charts to support the structured analysis, design, and information engineering methods that we were using at the time for our clients. Used correctly, these were powerful tools that resulted in higher quality systems because it forced us to answer questions earlier on and not wait until later in the project life cycle, when it’s more expensive and difficult to make changes. 03:16 Nikita: So, the idea was to try to get it wrong sooner. Joe: That’s right, Niki. We wanted to get our analysis and designs in front of the customer as soon as possible to find out what was wrong with our models and then change the code as early in the life cycle as possible where it was both easier and, more importantly, cheaper to make changes before solidifying it in code. Of course, the key words here are “used correctly,” right? I saw the tools misused many times by those who weren’t trained properly or, more typically, by those whose software development methodology, if there even was one, didn’t use the tools properly—and of course the tools took the blame. CASE tools at the time held a lot of promise, but one could say vendors were overpromising and under delivering, although I did have a number of clients who were successful with them and could get useful support for their software development life cycle from the use of the tools. Since then, I’ve been very interested in using tools to make it easier for us to build software. 04:09 Nikita: So, let me ask you Joe, what is your definition of a CASE tool?
Joe: I’m glad you asked, Niki, because I think many people have many different definitions. I’m precise about it, and maybe even a bit pedantic with the definition. The definition I use for a CASE tool comprises four things. One, it uses graphics, graphical symbols, and diagrams to represent requirements and business rules for the application. Two, there is a repository, either private, or shared, or both, of models, definitions, objects, requirements, rules, diagrams, and other assets that can be shared, reused, and almost more importantly, tracked. Three, there’s a rule-base that prevents you from drawing things that can’t be implemented. For example, Visio was widely regarded as a CASE tool, but it really wasn’t because it had no rules behind it. You could wire together anything you wanted, but that didn’t mean it could be built. Fourth, it generates useful code, and it should do two-way engineering, where code, typically code changed outside the model, can be reverse engineered back into the model and apply updates to the model, and to keep the model and the source code in synchronization. 05:13 Joe: I came up with a good slogan for CASE tools years ago: a good CASE tool should automate the tedious, manual portions of software development. I’d add that one also needs to be smarter than the tools they’re using. Which reminds me, interestingly enough, of clients who would pick up CASE tools, thinking that they would make their software development life cycle shorter. But if they weren’t already building models for analysis or design, then automating the building of things that they weren’t building already was not going to save them time and effort. And some people adopted CASE tools because they were told to or worse, forced to, or they read an article on an airplane, or had a Eureka moment, and they would try to get their entire software development staff to use this new tool, overnight literally, in some cases. Absolutely sheer madness! Tools like this need to be brought into the enterprise in a slow, measured fashion with a pilot project and build upon small successes until people start demanding to use the tools in their own projects once they see the value. And each group, each team would use the CASE tool differently and to a different degree. One size most definitely does not fit all and identifying what the teams’ needs are and how the tool can automate and support those needs is an important aspect of adopting a CASE tool. It’s funny, almost everyone would agree there’s value in creating models and, eventually, generating code from them to get better systems and it should be faster and cheaper, etc. But CASE tools never really penetrated the market more than maybe about 18 to 25%, tops. 06:39 Lois: Huh, why? Why do you think CASE tools were not widely accepted and used? Joe: Well, I don’t think it was an issue with the tools so much as it was with a company’s software development life cycle, and the culture and politics in the company. And I imagine you’re shocked to hear that. Ideally, switching to or adopting automated tools like CASE tools would reduce development time and costs, and improve quality. So it should increase reusability too. But increasing the reusability of code elements and software assets is difficult and requires discipline, commitment, and investment. Also, there can be a significant amount of training required to teach developers, analysts, project managers, and senior managers how to deal with these different forms of life cycles and artifacts: how they get created, how to manage them, and how to use them. When you have project managers or senior managers asking where’s the code and you try telling them, “Well, it’s gonna take a little while. We’re building models and will press the button to generate the code.” That’s tough. And that’s also another myth. It was never a matter of build all the models, press the button, generate all the code, and be done. It’s a very iterative process. 07:40 Joe: I’ve also found that developers find it very psychologically reinforcing to type code into the keyboard, see it appear on the screen, see it execute, and models were not quite as satisfying in the same way.
Redwood is a state-of-the-art graphical interface that defines the look and feel of the new Oracle Cloud Redwood Applications. In this episode, hosts Lois Houston and Nikita Abraham, along with Senior Principal OCI Instructor Joe Greenwald, take a closer look at the intent behind the design and development aspects of the new Redwood experience. They also explore Redwood page templates and components. Developing Redwood Applications with Visual Builder: https://mylearn.oracle.com/ou/learning-path/developing-redwood-applications-with-visual-builder/112791 Oracle University Learning Community: https://education.oracle.com/ou-community LinkedIn: https://www.linkedin.com/showcase/oracle-university/ X (formerly Twitter): https://twitter.com/Oracle_Edu Special thanks to Arijit Ghosh, David Wright, and the OU Studio Team for helping us create this episode. -------------------------------------------------------- Episode Transcript: 00:00 Welcome to the Oracle University Podcast, the first stop on your cloud journey. During this series of informative podcasts, we’ll bring you foundational training on the most popular Oracle technologies. Let’s get started. 00:26 Lois: Hello and welcome to the Oracle University Podcast! I’m Lois Houston, Director of Innovation Programs with Oracle University, and with me is Nikita Abraham, Principal Technical Editor. Nikita: Hi everyone! Last week, we discussed how Visual Builder Studio can be used to extend Oracle Fusion Apps. Lois: That’s right, Niki. In today’s episode, we will focus on Oracle’s Redwood design system and how it helps us create stunning, world-class enterprise applications and user experiences. 00:56 Nikita: Yeah, Redwood is the basis for all the new Oracle Cloud Applications being re-designed, developed, and delivered. To tell us more, we have Senior OCI Learning Solutions Architect and Principal Instructor Joe Greenwald, who’s been working with Oracle software development tools since the early 90s and is responsible for OU’s Visual Builder Studio and Redwood course content. Lois: Hi Joe! Thanks for being with us today. 01:21 Joe: Hi Lois. Hi Niki. I am excited to join you on this episode because with the release of 24A Fusion applications, we are encouraging all our customers to adopt the new Redwood design system and components, and take advantage of the world-class look and feel of the new Redwood user experience. Redwood represents a new approach and direction for us at Oracle, and we’re excited to have our customers benefit from it. 01:44 Nikita: Joe, you’ve been working with Oracle user interface development tools and frameworks for a long time. How and why is Redwood different? Joe: I joined Oracle in 1992, and the first Oracle user interface I experienced was Oracle Forms. And that was the character mode. I came from a background of Smalltalk and its amazing, pioneering graphical user interface (GUI) design capabilities. I worked at Apple and I developed my own GUIs for a few years on PCs and Macs. So, Character Mode Forms, what we used to call DMV (Department of Motor Vehicles) screens, was a shock, to say the least. Since then, I’ve worked with almost every user interface and development platform Oracle has created: Character Mode Forms, GUI Forms, Power Objects, HyperCard on the Macintosh, that was pre-OS X by the way, Sedona, written in native C++ and ActiveX and OLE, which didn’t make it to a product but appeared in other things later, ADF Faces, which uses Java to generate HTML pages, and APEX, which uses PL/SQL to generate HTML pages. And I’ve worked with and wrote training classes for Java Swing, an excellent GUI framework for event-driven desktop and enterprise applications, but it wasn’t designed for the web. So, it’s with pleasure that I introduce you to the Redwood design system, easily the best effort I’ve ever seen, from the look and feel of holistic user-goal-centered design philosophy and approach to the cutting-edge WYSIWYG design tools. 03:11 Lois: Joe, is Redwood just another set of styles, colors, and fonts, albeit very nice-looking ones? Joe: The Redwood platform is new for Oracle, and it represents a significant change, not just in the look and feel, colors, fonts, and styles, I mean that too, but it’s also a fundamental change in how Oracle is creating, designing, and imagining user interfaces. As you may be aware, all Oracle Cloud Applications are being re-designed, re-engineered, and re-rebuilt from the ground up, with significant changes to both back-end and front-end architectures. The front end is being redesigned, re-developed, and re-created in pure HTML5, CSS3, and JavaScript using Visual Builder Studio and its design-time browser-based Integrated Development Environment. The back end is being re-architected, re-designed, and implemented in a modern microservice architecture for Oracle Cloud using Kubernetes and other modern technologies that improve performance and work better in the cloud than our current legacy architecture. The new Oracle Cloud Applications platform uses Redwood for its design system—its tools, its patterns, its components, and page templates. Redwood is a richer and more productive platform to create solutions while still being cost-effective for Oracle. It encourages a transformation of the fundamental user experience, emphasizing identifying, meeting, and understanding end users’ goals and how the applications are used. 04:34 Nikita: Joe, do you think Oracle's user interface has been improved with Redwood? In what ways has the UI changed? Joe: Yes, absolutely. Redwood has changed a lot of things. When I joined Oracle back in the '90s, there was effectively no user interface division or UI team. There was no user interface lab—and that was started in the mid-‘90s—and I was asked to give product usability feedback and participate in UI tests and experiments in those labs. I also helped test the products I was teaching at the time. I actually distinctly remember having to take a week to train users on Oracle’s Designer CASE tool product just to prep the participants enough to perform usability testing. I can still hear the UI lab manager shaking her head and saying any product that requires a week of training to do usability testing has usability issues! And if you’re like me and you’ve been around Oracle long enough, you know that Oracle’s not always been known for its user interfaces and been known to release products that look like they were designed by two or more different companies. All that has changed with Redwood. With Redwood, there’s a new internal design group that oversees the design choices of all development teams that develop products. This includes a design system review and an ongoing audit process to ensure that all the products being released, whether Fusion apps or something else, all look and feel similar so it looks like it’s designed by a single company with a single thought in mind. Which it is. 06:00 Joe: There’s a deeper, consistent commitment in identifying user needs, understanding how the applications are being used, and how they meet those user needs through things like telemetry: gathering metrics from the actual components and the Redwood system itself to see how the applications are being used, what’s working well, and what isn’t. This telemetry is available to us here at Oracle, and we use it to fine tune the applications’ usability and purpose. 06:25 Lois: That’s really interesting, Joe. So, it’s a fundamental change in the way we’re doing things. What about the GUI components themselves? Are these more sophisticated than simple GUI components like buttons and text fields? Joe: The graphical components themselves are at a much higher level, more comprehensive, and work better together. And in Redwood, everything is a component. And I’m not just talking about things like input text fields and buttons, though it applies to these more fine-grained components as well. Leveraging Oracle’s deep experience in building enterprise applications, we’ve incorporated that knowledge into creating page templates so that the structure and look and feel of the page is fixed based on our internal design standards. The developer has control over certain portions of it, but the overall look and feel of the page is controlled by Oracle. So there is consistency of look and feel within and across applications. These page templates come with predefined functionalities: headers, titles, properties, and variables to manipulate content and settings, slots for other components to hold like search fields, collections, contextual information, badges, and images, as well as primary and secondary actions, and variables for events and event handling through Visual Builder action chains, which handle the various actions and processing of the request on the page. And all these page templates and components are responsive, meaning they respond to the change in the size of the page and the orientation. So, when you move from a desktop to a handheld mobile device or a tablet, they respond appropriately and consistently to deliver a clean, easy-to-use interface and experience. 07:58 Nikita: You mentioned WYSIWYG design tools and their integration with Visual Builder Studio’s integrated development environment. How does Redwood work with Visual Builder Studio? Joe: This is easily one of my most favorite aspects about Redwood and the integration with Visual Builder Studio Designer. The components and page templates are responsive at runtime as well as responsive at design time! In over 30 years of working with Oracle software development products, this is the first development system and integrated development environment I’ve seen Oracle produce where what you see is what you get at design time. Now, with products such as Designer and JDeveloper ADF Faces and even APEX—all those page-generation types of products—you have to generate the page, deploy it, and only then can you view the final page to see
What do you need to start customizing the next generation of Oracle Fusion Apps? How do you create new pages for business processes? What level of expertise do you require for this? Join Lois Houston and Nikita Abraham as they get answers to all these questions and more from Senior Principal OCI Instructor Joe Greenwald. Develop Fusion Applications Using Visual Builder Studio: https://mylearn.oracle.com/ou/course/develop-fusion-applications-using-visual-builder-studio/122614/ Build Visual Applications Using Visual Builder Studio: https://mylearn.oracle.com/ou/course/build-visual-applications-using-oracle-visual-builder-studio/110035/ Oracle University Learning Community: https://education.oracle.com/ou-community LinkedIn: https://www.linkedin.com/showcase/oracle-university/ X (formerly Twitter): https://twitter.com/Oracle_Edu Special thanks to Arijit Ghosh, David Wright, and the OU Studio Team for helping us create this episode. -------------------------------------------------------- Episode Transcript: 00:00 Welcome to the Oracle University Podcast, the first stop on your cloud journey. During this series of informative podcasts, we’ll bring you foundational training on the most popular Oracle technologies. Let’s get started. 00:26 Lois: Hello and welcome to the Oracle University Podcast! I’m Lois Houston, Director of Innovation Programs with Oracle University, and with me is Nikita Abraham, Principal Technical Editor. Nikita: Hi everyone! Last week, we were introduced to Visual Builder Studio and the Oracle JavaScript Extension Toolkit, also known as JET. Lois: Our friend and Senior Principal OCI Instructor Joe Greenwald is back with us today to talk about how to extend Oracle Cloud Applications that are being built using Visual Builder for its front-end. Nikita: That’s right. All Fusion Applications are being redesigned and rebuilt using Visual Builder. And we’ll find out more about that from Joe. Hi Joe! Thanks for being with us today. Joe: Hi Lois! Hi Niki! My pleasure to be here. 01:09 Nikita: Joe, tell us a little about what’s happening with the redesign and re-architecture of Oracle Cloud Applications using Visual Builder Studio, or VBS. I hear some very exciting changes are coming that are important for our customers and partners. Joe: That’s right, Niki. Oracle is redesigning and rebuilding its entire suite of Fusion Cloud Applications, over 330 different products, utilizing over 60,000 engineers — that is “60,” not “16” — at Oracle to develop the next generation of Oracle Fusion Applications. What’s most exciting is that the same tools the engineers are using to accomplish this are available to our partners and our customers to use to extend the functionality and capabilities of Fusion Applications to meet their custom needs and processes. 01:54 Lois: That’s pretty awesome! We want to use this time today to ask you about extensions, the types of extensions you can create, and how to use Visual Builder Studio to create those extensions. Nikita: Yeah, can we start with you telling us what an extension is? I’ve gotten the sense that Oracle uses the term extension as both a noun and a verb and that’s a bit confusing to me. 02:15 Joe: Yeah, good catch, Niki. Yes, Oracle does use the term extension in two ways: both as a noun and a verb. As a noun, an extension is a container for the code changes that you make to your applications. Basically, it’s a Git repository that Oracle creates and manages for you. So, the extension container holds the code changes you make to your page layouts: the fields, their positioning, showing and hiding fields, that sort of thing, as well as page functionality. These code changes you make are stored in the extension and it is this extension with your code changes that is merged with the main Git branch eventually and then deployed using continuous integration/continuous deployment jobs defined in Visual Builder Studio, which manages the project and its assets. Your extension is a Git branch that is an asset of the project. Once your extension code is merged with the main branch and deployed, then the next time someone brings up the application, they’ll see the changes you’ve made in the app. 03:08 Lois: And as a verb? Joe: As a verb, extension means to extend the functionality and the look and feel of the application, though I prefer the term customization or configuration to describe this aspect, as the documentation does, and to avoid confusion, though I’ll admit I’m not always consistent about the terms I use. 03:26 Lois: What types of customizations, or extensions, and I’m using the verb now, are available for Fusion Apps in Visual Builder Studio? Joe: There are three different ways Fusion Apps can be customized effectively, configured, or extended. The first way is what we call a basic extension, where you’re rearranging hiding, or showing, or moving around fields and sections on the page that have been set up to be extendable by the Fusion Application development teams. Things like hiding fields, showing fields, hiding sections, showing sections… Nikita: So fairly basic actions… Joe: Yeah exactly and they can be done in Visual Builder Studio Designer by people with minimal VB training, Visual Builder training. And, most recently, if you have access to it, you can do it in the new Express mode, where the page shows you just those things you can work with and just the tools you need to work with the page. This is new and makes it much easier for folks who are not highly technical to make basic changes to the page layout. 04:18 Lois: People like me! That sounds easy enough. Joe: And the next type of extension is more of an intermediate change and requires some training with Visual Builder Studio because you’re creating rules that govern the display of layouts based on certain conditions on the page. These are highly flexible, powerful, and useful for creating customized page layouts based on a variety of factors from page size and orientation to the role of the person using it to values in the actual fields on the page itself. These rules can be combined to create complex rule-based conditions that display exactly what the user should see, given the conditions of the page and their role. I would also include making changes to action chains, which execute sequences of behaviors and navigation, and the actual structure of the application, but this is more advanced. Lastly, is creating mashup applications, which are stand-alone Visual Builder visual applications, which use data from Fusion apps, and customer data sources, like their own database tables, and potentially third-party APIs to create brand new pages and applications with new functionality, new processes, new procedures, new displays, all of which look just like Fusion Applications and use the same data as Fusion applications. 05:27 Lois: Joe, how do I get started if I want to extend a page? Joe: The easiest way to do it is to open a page in Fusion Applications and then select Edit Page in Visual Builder Studio from the Profile menu. You’re then prompted for a project to hold the Git repository for the extension container. And since there’s probably already one that exists, after you select the project, an extension Git container is assigned to you. Unless this is the very first time the application has been extended in which case it creates an extension for you. When creating customizations or configurations, we recommend that each application be done in its own separate project. So, for example, if you’re working on Customer Experience Sales, you might do it in Project A and if you’re working on extensions with HCM, you might do it in Project B. And if you decide to create your own pages and flows in your own app, you might do that in Project C. 06:13 Nikita: But why do you need to do this? Joe: That’s just to keep things nice and separate and organized. The tool, Visual Builder Studio, doesn’t really care, but it makes for cleaner development and can help with the management of the development teams. 06:23 Nikita: Ok, Joe, I have a question. How do I know if the page I’m on in Fusion Apps can be edited in Visual Builder? I know there are a lot of legacy pages still out there and they can co-exist with the new VB-based pages. Joe: If the URL of the page you’re on has the word /Redwood in it instead of /faces, then you know this is a page that was created using Visual Builder Studio and you’ll be able to extend it and make changes to it using the Edit in Visual Builder Studio option. So, if you select Edit in Visual Builder Studio, then the page you are on opens inside Visual Builder Studio Designer and you can make changes to any part of the page that has been explicitly enabled for extension by the development team. 07:02 Lois: That’s an important part, right? The application is not extendable by default. Joe: That’s right, Lois. It is all locked down and you can’t make any changes to it by default. The development team must specifically enable certain parts of the page: sections, fields, layouts, variables, types, action chains, etc. as extendable for you to be able to make changes to it. This ensures the changes the development team makes to the application in the future won’t break your extensions. And conversely, the development team can choose to not extend portions that they do not want you to touch or mess with. Then if they do change that bit of the app in the future, it won’t break the application and you won’t get a big surprise. So, using the Edit page in Visual Builder Studio, you can make both basic changes, like moving, showing, and hiding fields and sections, as well as the more intermediate types of configurations, like using dynamic components to create rule-based layouts that change dynamically based on several conditions such as page size, roles of the user, and field values on the page itself. 08:00 Nikita: What happens if two developers make changes and essentially overwrite eac
The next generation of front-end user interfaces for Oracle Fusion Applications is being built using Visual Builder Studio and Oracle JavaScript Extension Toolkit. However, many of the terms associated with these tools can be confusing. In this episode, Lois Houston and Nikita Abraham are joined by Senior Principal OCI Instructor Joe Greenwald. Together, they take you through the different terminologies, how they relate to each other, and how they can be used to deliver the new Oracle Fusion Applications as well as stand-alone, bespoke visual web applications. Develop Fusion Applications Using Visual Builder Studio: https://mylearn.oracle.com/ou/course/develop-fusion-applications-using-visual-builder-studio/122614/ Build Visual Applications Using Visual Builder Studio: https://mylearn.oracle.com/ou/course/build-visual-applications-using-oracle-visual-builder-studio/110035/ Oracle University Learning Community: https://education.oracle.com/ou-community LinkedIn: https://www.linkedin.com/showcase/oracle-university/ X (formerly Twitter): https://twitter.com/Oracle_Edu Special thanks to Arijit Ghosh, David Wright, and the OU Studio Team for helping us create this episode. --------------------------------------------------------- Episode Transcript: 00:00 Welcome to the Oracle University Podcast, the first stop on your cloud journey. During this series of informative podcasts, we’ll bring you foundational training on the most popular Oracle technologies. Let’s get started. 00:26 Lois: Hello and welcome to the Oracle University Podcast. I’m Lois Houston, Director of Innovation Programs with Oracle University, and with me is Nikita Abraham, Principal Technical Editor. Nikita: Hi everyone! Today, we’re starting a new season on building the next generation of Oracle Cloud Apps with Visual Builder Studio. 00:45 Lois: And I’m so excited that we have someone really special to take us through the next few episodes. Joe Greenwald is joining us. Joe is a Senior Principal OCI Instructor with Oracle University. He joined Oracle in 1992 with an extensive background in CASE tools. Since then, he has used and taught all of Oracle's software development tools, including Oracle Forms, APEX, JDeveloper ADF, as well as all the Fusion Middleware courses. Currently, Joe is responsible for the Visual Builder Studio and Redwood development courses, including extending Fusion Applications with Visual Builder. 01:22 Nikita: In today’s episode, we’re going to ask Joe about Visual Builder Studio and Oracle JavaScript Extension Toolkit, also known as JET. Together, they form the basis of the technology for the next generation of front-end user interfaces for Oracle Fusion Applications, as well as many other Oracle applications, including most Oracle Cloud Infrastructure (OCI) interfaces. Lois: We’ll look at the different terminologies and technologies, how they relate to each other, and how they deliver the new Oracle Fusion applications and stand-alone, bespoke visual web applications. Hi Joe! Thanks for being with us today. 01:57 Joe: Hi Lois! Hi Niki! I’m glad to be here. Nikita: Joe, I’m somewhat thrown by the terminology around Visual Builder, Visual Studio, and JET. Can you help streamline that for us? Lois: Yeah, things that are named the same sometimes refer to different things, and sometimes things with a different name refer to the same thing. 02:15 Joe: Yeah, I know where you’re coming from. So, let’s start with Visual Builder Studio. It’s abbreviated as VBS and can go by a number of different names. Some of the most well-known ones are Visual Builder Studio, VBS, Visual Builder, Visual Builder Stand-Alone, and Visual Builder Cloud Service. Clearly, this can be very confusing. For the purposes of these episodes as well as the training courses I create, I use certain definitions. 02:39 Lois: Can you take us through those? Joe: Absolutely, Lois. Visual Builder Studio refers to a product that comes free with an OCI account and allows you to manage your project-related assets. This includes the project itself, which is a container for all of its assets. You can assign teams to your projects, as well as secure the project and declare roles for the different team members. You manage GIT repositories with full graphical and command-line GIT support, define package, build, and deploy jobs, and create and run continuous integration/continuous deployment graphical and code-managed pipelines for your applications. These can be visual applications, created using the Visual Builder Integrated Development Environment, the IDE, or non-visual apps, such as Java microservices, docker builds, NPM apps, and things like that. And you can define environments, which determine where your build jobs can be deployed. You can also define issues, which allow you to identify, track, and manage things like bugs, defects, and enhancements. And these can be tracked in code review merge requests and build jobs, and be mapped to agile sprints and scrum boards. There’s also support for wikis for team collaboration, code snippets, and the management of the repository and the project itself. So, VBS supports code reviews before code is merged into GIT branches for package, build, and deploy jobs using merge requests. 03:57 Nikita: OK, what exactly do you mean by that? Joe: Great. So, for example, you could have developers working in one GIT branch and when they’re done, they would push their private code changes into that remote branch. Then, they’d submit a merge request and their changes would be reviewed. Once the changes are approved, their code branch is merged into the main branch and then automatically runs a CI/CD package (continuous integration/continuous deployment) package, build, and deploy job on the code. Also, the CI/CD package, build, and deploy jobs can run against any branches, not just the main branch. So Visual Builder Studio is intended for managing the project and all of its assets. 04:37 Lois: So Joe, what are the different tools used in developing web applications? Joe: Well, Visual Builder, Visual Builder Studio Designer, Visual Builder Designer, Visual Builder Design-Time, Visual Builder Cloud Service, Visual Builder Stand-Alone all kind of get lumped together. You can kinda see why. What I’m referring to here are the tools that we use to build a visual web application composed of HTML5, CSS3, JavaScript, and JSON (JavaScript Object Notation) for metadata. I call this Visual Builder Designer. This is an Integrated Development Environment, it’s the “IDE” which runs in your browser. You use a combination of drag and drop, setting properties, and writing and modifying custom and generated code to develop your web applications. You work within a workspace, which is your own private copy of a remote Git branch. When you’re ready to start development work, you open an existing workspace or create a new one based on a clone of the remote branch you want to work on. Typically, a new branch would be created for the development work or you would join an existing branch. 05:35 Nikita: What’s a workspace, Joe? Is it like my personal laptop and drive? Joe: A workspace is your own private code area that stores any changes you make on the Oracle servers, so your code changes are never lost—even when working in a browser-based, network-based tool. A good analogy is, say I was working at home on my own machine. And I would make a copy of a remote GIT branch and then copy that code down to my local machine, make my code changes, do my testing, etc. and then commit my work—create a logical save point periodically—and then when I’m ready, I’d push that code up into the remote branch so it can be reviewed and merged with the main branch. My local machine is my workspace. However, since this code is hosted up by Oracle on our servers, and the code and the IDE are all running in your browser, the workspace is a simulation of a local work area on your own computer. So, the workspace is a hosted allocation of resources for you that’s private. Other people can’t see what’s going on in your workspace. Your workspace has a clone of the remote branch that you’re working with and the changes you make are isolated to your cloned code in your workspace. 06:38 Lois: Ok… the code is actually hosted on the server, so each time you make a change in the browser, the change is written back to the server? Is it possible that you might lose your edits if there’s a networking interruption? Joe: I want to emphasize that while I started out not personally being a fan of web-based integrated development environments, I have been using these tools for over three years and in all that time, while I have lost a connection at times—networks are still subject to interruptions—I’ve never lost any changes that I’ve made. Ever. 07:08 Nikita: Is there a way to save where you are in your work so that you could go back to it later if you need to? Joe: Yes, Niki, you’re asking about commits and savepoints, like in a Git repository or a Git branch. When you reach a logical stopping or development point in your work, you would create a commit or a savepoint. And when you’re ready, you would push that committed code in your workspace up to the remote branch where it can be reviewed and then eventually merged, usually with the main Git branch, and then continuous integration/continuous package and deployment build jobs are run. Now, I’m only giving you a high-level overview, but we cover all this and much more in detail with hands-on practices in our Visual Builder developer courses. Right now, I’m just trying to give you a sense of how these different tools are used. 07:49 Lois: Yes, that makes sense, Joe. It’s a lot to cover in a short amount of time. Now, we’ve discussed the Visual Builder Designer IDE and workspace. But can you tell us more about Visual Builder Cloud Service and stand-alone environments? What are they used for? What features do they provide? Are they the same or diff
Listen to Lois Houston and Nikita Abraham, along with Senior Principal Product Manager Wes Prichard, as they explore the five core components of OCI AI services: language, speech, vision, document understanding, and anomaly detection, to help you make better sense of all that unstructured data around you. Oracle MyLearn: https://mylearn.oracle.com/ou/learning-path/become-an-oci-ai-foundations-associate-2023/127177 Oracle University Learning Community: https://education.oracle.com/ou-community LinkedIn: https://www.linkedin.com/showcase/oracle-university/ X (formerly Twitter): https://twitter.com/Oracle_Edu Special thanks to Arijit Ghosh, David Wright, Himanshu Raj, and the OU Studio Team for helping us create this episode. -------------------------------------------------------- Episode Transcript: 00:00 Welcome to the Oracle University Podcast, the first stop on your cloud journey. During this series of informative podcasts, we’ll bring you foundational training on the most popular Oracle technologies. Let’s get started! 00:26 Nikita: Welcome to the Oracle University Podcast! I’m Nikita Abraham, Principal Technical Editor with Oracle University, and with me is Lois Houston, Director of Innovation Programs. Lois: Hi there! In our last episode, we spoke about OCI AI Portfolio, including AI and ML services, and the OCI AI infrastructure. Nikita: Yeah, and in today’s episode, we’re going to continue down a similar path and take a closer look at OCI AI services. 00:55 Lois: With us today is Senior Principal Product Manager, Wes Prichard. Hi Wes! It’s lovely to have you here with us. Hemant gave us a broad overview of the various OCI AI services last week, but we’re really hoping to get into each of them with you. So, let’s jump right in and start with the OCI Language service. What can you tell us about it? Wes: OCI Language analyzes unstructured text for you. It provides models trained on industry data to perform language analysis with no data science experience needed. 01:27 Nikita: What kind of big things can it do? Wes: It has five main capabilities. First, it detects the language of the text. It recognizes 75 languages, from Afrikaans to Welsh. It identifies entities, things like names, places, dates, emails, currency, organizations, phone numbers--14 types in all. It identifies the sentiment of the text, and not just one sentiment for the entire block of text, but the different sentiments for different aspects. 01:56 Nikita: What do you mean by that, Wes? Wes: So let's say you read a restaurant review that said, the food was great, but the service sucked. You'll get food with a positive sentiment and service with a negative sentiment. And it also analyzes the sentiment for every sentence. Lois: Ah, that’s smart. Ok, so we covered three capabilities. What else? Wes: It identifies key phrases in the text that represent the important ideas or subjects. And it classifies the general topic of the text from a list of 600 categories and subcategories. 02:27 Lois: Ok, and then there’s the OCI Speech service... Wes: OCI Speech is very straightforward. It locks the data in audio tracks by converting speech to text. Developers can use Oracle's time-tested acoustic language models to provide highly accurate transcription for audio or video files across multiple languages. OCI Speech automatically transcribes audio and video files into text using advanced deep learning techniques. There's no data science experience required. It processes data directly in object storage. And it generates timestamped, grammatically accurate transcriptions. 03:01 Nikita: What are some of the main features of OCI Speech? Wes: OCI Speech supports multiple languages, specifically English, Spanish, and Portuguese, with more coming in the future. It has batching support where multiple files can be submitted with a single call. It has blazing fast processing. It can transcribe hours of audio in less than 10 minutes. It does this by chunking up your audio into smaller segments, and transcribing each segment, and then joining them all back together into a single file. It provides a confidence score, both per word and per transcription. It punctuates transcriptions to make the text more readable and to allow downstream systems to process the text with less friction. And it has SRT file support. 03:45 Lois: SRT? What’s that? Wes: SRT is the most popular closed caption output file format. And with this SRT support, users can add closed captions to their video. OCI Speech makes transcribed text more readable to resemble how humans write. This is called normalization. And the service will normalize things like addresses, times, numbers, URLs, and more. It also does profanity filtering, where it can either remove, mask, or tag profanity and output text, where removing replaces the word with asterisks, and masking does the same thing, but it retains the first letter, and tagging will leave the word in place, but it provides tagging in the output data. 04:29 Nikita: And what about OCI Vision? What are its capabilities? Wes: Vision is a computed vision service that works on images, and it provides two main capabilities-- image analysis and document AI. Image analysis analyzes photographic images. Object detection is the feature that detects objects inside an image using a bounding box and assigning a label to each object with an accuracy percentage. Object detection also locates and extracts text that appears in the scene, like on a sign. Image classification will assign classification labels to the image by identifying the major features in the scene. One of the most powerful capabilities of image analysis is that, in addition to pretrained models, users can retrain the models with their own unique data to fit their specific needs. 05:20 Lois: So object detection and image classification are features of image analysis. I think I got it! So then what’s document AI? Wes: It's used for working with document images. You can use it to understand PDFs or document image types, like JPEG, PNG, and Tiff, or photographs containing textual information. 05:40 Lois: And what are its most important features? Wes: The features of document AI are text recognition, also known as OCR or optical character recognition. And this extracts text from images, including non-trivial scenarios, like handwritten texts, plus tilted, shaded, or rotated documents. Document classification classifies documents into 10 different types based on visual appearance, high-level features, and extracted keywords. This is useful when you need to process a document, based on its classification, like an invoice, a receipt, or a resume. Language detection analyzes the visual features of text to determine the language rather than relying on the text itself. Table extraction identifies tables in docs and extracts their content in tabular form. Key value extraction finds values for 13 common fields and line items in receipts, things like merchant name and transaction date. 06:41 Want to get the inside scoop on Oracle University? Head over to the Oracle University Learning Community. Attend exclusive events. Read up on the latest news. Get first-hand access to new products. Read the OU Learning Blog. Participate in Challenges. And stay up-to-date with upcoming certification opportunities. Visit mylearn.oracle.com to get started. 07:06 Nikita: Welcome back! Wes, I want to ask you about OCI Anomaly Detection. We discussed it a bit last week and it seems like such an intelligent and efficient service. Wes: Oracle Cloud Infrastructure Anomaly Detection identifies anomalies in time series data. Equipment sensors generate time series data, but all kinds of business metrics are also time-based. The unique feature of this service is that it finds anomalies, not just in a single signal, but across many signals at once. That's important because machines often generate multiple signals at once and the signals are often related. 07:42 Nikita: Ok you need to give us an example of this! Wes: Think of a pump that has an output pressure, a flow rate, an RPM, and an electrical current draw. When a pump's going to fail, anomalies may appear across several of those signals but at different times. OCI Anomaly Detection helps you to identify anomalies in a multivariate data set by taking advantage of the interrelationship among signals. The service contains algorithms for both multi-signal, as in multivariate, single signal, as in univariate anomaly detection, and it automatically determines which algorithm to use based on the training data provided. The multivariate algorithm is called MSET-2, which stands for Multivariate State Estimation technique, and it's unique to Oracle. 08:28 Lois: And the 2? Wes: The 2 in the name refers to the patented enhancements by Oracle labs that automatically identify and fix data quality issues resulting in fewer false alarms and more accurate results. Now unlike some of the other AI services, OCI Anomaly Detection is always trained on the customer's data. It's trained using actual historical data with no anomalies, and there can be as many different trained models as needed for different sets of signals. 08:57 Nikita: So where would one use a service like this? Wes: One of the most obvious applications of this service is for predictive maintenance. Early warning of a problem provides the opportunity to deploy maintenance resources and schedule downtime to minimize disruption to the business. 09:12 Lois: How would you train an OCI Anomaly Detection model? Wes: It's a simple four-step process to prepare a model that can be used for anomaly detection. The first step is to obtain training data from the system to be monitored. The data must contain no anomalies and should cover the normal range of values that would be experienced in a full business cycle. Second, the training data file is uploaded to an object storage bucket. Third, a data set is created for the trai
In this week’s episode, Lois Houston and Nikita Abraham, along with Senior Instructor Himanshu Raj, take you through the extraordinary capabilities of Generative AI, a subset of deep learning that doesn’t make predictions but rather creates its own content. They also explore the workings of Large Language Models. Oracle MyLearn: https://mylearn.oracle.com/ou/learning-path/become-an-oci-ai-foundations-associate-2023/127177 Oracle University Learning Community: https://education.oracle.com/ou-community LinkedIn: https://www.linkedin.com/showcase/oracle-university/ X (formerly Twitter): https://twitter.com/Oracle_Edu Special thanks to Arijit Ghosh, David Wright, and the OU Studio Team for helping us create this episode. -------------------------------------------------------- Episode Transcript: 00:00 Welcome to the Oracle University Podcast, the first stop on your cloud journey. During this series of informative podcasts, we’ll bring you foundational training on the most popular Oracle technologies. Let’s get started! 00:26 Lois: Hello and welcome to the Oracle University Podcast. I’m Lois Houston, Director of Innovation Programs with Oracle University, and with me is Nikita Abraham, Principal Technical Editor. Nikita: Hi everyone! In our last episode, we went over the basics of deep learning. Today, we’ll look at generative AI and large language models, and discuss how they work. To help us with that, we have Himanshu Raj, Senior Instructor on AI/ML. So, let’s jump right in. Hi Himanshu, what is generative AI? 01:00 Himanshu: Generative AI refers to a type of AI that can create new content. It is a subset of deep learning, where the models are trained not to make predictions but rather to generate output on their own. Think of generative AI as an artist who looks at a lot of paintings and learns the patterns and styles present in them. Once it has learned these patterns, it can generate new paintings that resembles what it learned. 01:27 Lois: Let's take an example to understand this better. Suppose we want to train a generative AI model to draw a dog. How would we achieve this? Himanshu: You would start by giving it a lot of pictures of dogs to learn from. The AI does not know anything about what a dog looks like. But by looking at these pictures, it starts to figure out common patterns and features, like dogs often have pointy ears, narrow faces, whiskers, etc. You can then ask it to draw a new picture of a dog. The AI will use the patterns it learned to generate a picture that hopefully looks like a dog. But remember, the AI is not copying any of the pictures it has seen before but creating a new image based on the patterns it has learned. This is the basic idea behind generative AI. In practice, the process involves a lot of complex maths and computation, and there are different techniques and architectures that can be used, such as variational autoencoders (VAs) and Generative Adversarial Networks (GANs). 02:27 Nikita: Himanshu, where is generative AI used in the real world? Himanshu: Generative AI models have a wide variety of applications across numerous domains. For the image generation, generative models like GANs are used to generate realistic images. They can be used for tasks, like creating artwork, synthesizing images of human faces, or transforming sketches into photorealistic images. For text generation, large language models like GPT 3, which are generative in nature, can create human-like text. This has applications in content creation, like writing articles, generating ideas, and again, conversational AI, like chat bots, customer service agents. They are also used in programming for code generation and debugging, and much more. For music generation, generative AI models can also be used. They create new pieces of music after being trained on a specific style or collection of tunes. A famous example is OpenAI's MuseNet. 03:21 Lois: You mentioned large language models in the context of text-based generative AI. So, let’s talk a little more about it. Himanshu, what exactly are large language models? Himanshu: LLMs are a type of artificial intelligence models built to understand, generate, and process human language at a massive scale. They were primarily designed for sequence to sequence tasks such as machine translation, where an input sequence is transformed into an output sequence. LLMs can be used to translate text from one language to another. For example, an LLM could be used to translate English text into French. To do this job, LLM is trained on a massive data set of text and code which allows it to learn the patterns and relationships that exist between different languages. The LLM translates, “How are you?” from English to French, “Comment allez-vous?” It can also answer questions like, what is the capital of France? And it would answer the capital of France is Paris. And it will write an essay on a given topic. For example, write an essay on French Revolution, and it will come up with a response like with a title and introduction. 04:33 Lois: And how do LLMs actually work? Himanshu: So, LLM models are typically based on deep learning architectures such as transformers. They are also trained on vast amount of text data to learn language patterns and relationships, again, with a massive number of parameters usually in order of millions or even billions. LLMs have also the ability to comprehend and understand natural language text at a semantic level. They can grasp context, infer meaning, and identify relationships between words and phrases. 05:05 Nikita: What are the most important factors for a large language model? Himanshu: Model size and parameters are crucial aspects of large language models and other deep learning models. They significantly impact the model’s capabilities, performance, and resource requirement. So, what is model size? The model size refers to the amount of memory required to store the model's parameter and other data structures. Larger model sizes generally led to better performance as they can capture more complex patterns and representation from the data. The parameters are the numerical values of the model that change as it learns to minimize the model's error on the given task. In the context of LLMs, parameters refer to the weights and biases of the model's transformer layers. Parameters are usually measured in terms of millions or billions. For example, GPT-3, one of the largest LLMs to date, has 175 billion parameters making it extremely powerful in language understanding and generation. Tokens represent the individual units into which a piece of text is divided during the processing by the model. In natural language, tokens are usually words, subwords, or characters. Some models have a maximum token limit that they can process and longer text can may require truncation or splitting. Again, balancing model size, parameters, and token handling is crucial when working with LLMs. 06:29 Nikita: But what’s so great about LLMs? Himanshu: Large language models can understand and interpret human language more accurately and contextually. They can comprehend complex sentence structures, nuances, and word meanings, enabling them to provide more accurate and relevant responses to user queries. This model can generate human-like text that is coherent and contextually appropriate. This capability is valuable for context creation, automated writing, and generating personalized response in applications like chatbots and virtual assistants. They can perform a variety of tasks. Large language models are very versatile and adaptable to various industries. They can be customized to excel in applications such as language translation, sentiment analysis, code generation, and much more. LLMs can handle multiple languages making them valuable for cross-lingual tasks like translation, sentiment analysis, and understanding diverse global content. Large language models can be again, fine-tuned for a specific task using a minimal amount of domain data. The efficiency of LLMs usually grows with more data and parameters. 07:34 Lois: You mentioned the “sequence to sequence tasks” earlier. Can you explain the concept in simple terms for us? Himanshu: Understanding language is difficult for computers and AI systems. The reason being that words often have meanings based on context. Consider a sentence such as Jane threw the frisbee, and her dog fetched it. In this sentence, there are a few things that relate to each other. Jane is doing the throwing. The dog is doing the fetching. And it refers to the frisbee. Suppose we are looking at the word “it” in the sentence. As a human, we understand easily that “it” refers to the frisbee. But for a machine, it can be tricky. The goal in sequence problems is to find patterns, dependencies, or relationships within the data and make predictions, classification, or generate new sequences based on that understanding. 08:27 Lois: And where are sequence models mostly used? Himanshu: Some common example of sequence models includes natural language processing, which we call NLP, tasks such as machine translation, text generation sentiment analysis, language modeling involve dealing with sequences of words or characters. Speech recognition. Converting audio signals into text, involves working with sequences of phonemes or subword units to recognize spoken words. Music generation. Generating new music involves modeling musical sequences, nodes, and rhythms to create original compositions. Gesture recognition. Sequences of motion or hand gestures are used to interpret human movements for applications, such as sign language recognition or gesture-based interfaces. Time series analysis. In fields such as finance, economics, weather forecasting, and signal processing, time series data is used to predict future values, detect anomalies, and understand patterns in temporal data. 09:35 The Oracle University Learning Community is an excellent pla
Did you know that the concept of deep learning goes way back to the 1950s? However, it is only in recent years that this technology has created a tremendous amount of buzz (and for good reason!). A subset of machine learning, deep learning is inspired by the structure of the human brain, making it fascinating to learn about. In this episode, Lois Houston and Nikita Abraham interview Senior Principal OCI Instructor Hemant Gahankari about deep learning concepts, including how Convolution Neural Networks work, and help you get your deep learning basics right. Oracle MyLearn: https://mylearn.oracle.com/ Oracle University Learning Community: https://education.oracle.com/ou-community LinkedIn: https://www.linkedin.com/showcase/oracle-university/ X (formerly Twitter): https://twitter.com/Oracle_Edu Special thanks to Arijit Ghosh, David Wright, Himanshu Raj, and the OU Studio Team for helping us create this episode. -------------------------------------------------------- Episode Transcript: 00:00 Welcome to the Oracle University Podcast, the first stop on your cloud journey. During this series of informative podcasts, we’ll bring you foundational training on the most popular Oracle technologies. Let’s get started! 00:26 Lois: Hello and welcome to the Oracle University Podcast. I’m Lois Houston, Director of Innovation Programs with Oracle University, and with me is Nikita Abraham, Principal Technical Editor. Nikita: Hi everyone! Last week, we covered the new MySQL HeatWave Implementation Associate certification. So do go check out that episode if it interests you. Lois: That was a really interesting discussion for sure. Today, we’re going to focus on the basics of deep learning with our Senior Principal OCI Instructor, Hemant Gahankari. 00:58 Nikita: Hi Hemant! Thanks for being with us today. So, to get started, what is deep learning? Hemant: Deep learning is a subset of machine learning that focuses on training Artificial Neural Networks to solve a task at hand. Say, for example, image classification. A very important quality of the ANN is that it can process raw data like pixels of an image and extract patterns from it. These patterns are treated as features to predict the outcomes. Let us say we have a set of handwritten images of digits 0 to 9. As we know, everyone writes the digits in a slightly different way. So how do we train a machine to identify a handwritten digit? For this, we use ANN. ANN accepts image pixels as inputs, extracts patterns like edges and curves and so on, and correlates these patterns to predict an outcome. That is what digit does the image has in this case. 02:04 Lois: Ok, so what you’re saying is given a bunch of pixels, ANN is able to process pixel data, learn an internal representation of the data, and predict outcomes. That’s so cool! So, why do we need deep learning? Hemant: We need to specify features while we train machine learning algorithm. With deep learning, features are automatically extracted from the data. Internal representation of features and their combinations is built to predict outcomes by deep learning algorithms. This may not be feasible manually. Deep learning algorithms can make use of parallel computations. For this, usually data is split into small batches and process parallelly. So these algorithms can process large amount of data in a short time to learn the features and their combinations. This leads to scalability and performance. In short, deep learning complements machine learning algorithms for complex data for which features cannot be described easily. 03:13 Nikita: What can you tell us about the origins of deep learning? Hemant: Some of the deep learning concepts like artificial neuron, perceptron, and multilayer perceptron existed as early as 1950s. One of the most important concept of using backpropagation for training ANN came in 1980s. In 1990s, convolutional neural network were also introduced for image analysis task. Starting 2000, GPUs were introduced. And 2010 onwards, GPUs became cheaper and widely available. This fueled the widespread adoption of deep learning uses like computer vision, natural language processing, speech recognition, text translation, and so on. In 2012, major networks like AlexNet and Deep-Q Network were built. 2016 onward, generative use cases of the deep learning also started to come up. Today, we have widely adopted deep learning for a variety of use cases, including large language models and many other types of generative models. 04:29 Lois: Hemant, what are various applications of deep learning algorithms? Hemant: Deep learning algorithms are targeted at a variety of data and applications. For data, we have images, videos, text, and audio. For images, applications can be image classification, object detection, and so on. For textual data, applications are to translate the text or detect a sentiment of a text. For audio, the applications can be music generation, speech to text, and so on. 05:08 Lois: It's important that we select the right deep learning algorithm based on the data and application, right? So how do we do that? Hemant: For image task like image classification, object detection, image segmentation, or facial recognition, CNN is a suitable architecture. For text, we have a choice of the latest transformers or LSTM or even RNN. For generative tasks like text summarization, question answering, transformers is a good choice. For generating images, text to image generation, transformers, GANs, or diffusion models are available choice. 05:51 Nikita: Let’s dive a little deeper into Artificial Neural Networks. Can you tell us more about them, Hemant? Hemant: Artificial Neural Networks are inspired by the human brain. They are made up of interconnected nodes called as neurons. Nikita: And how are inputs processed by a neuron? Hemant: In ANN, we assign weights to the connection between neurons. Weighted inputs are added up. And if the sum crosses a specified threshold, the neuron is fired. And the outputs of a layer of neuron become an input to another layer. 06:27 Lois: Hemant, tell us about the building blocks of ANN so we understand this better. Hemant: So first, building block is layers. We have input layer, output layer, and multiple hidden layers. The input layer and output layer are mandatory. And the hidden layers are optional. The second unit is neurons. Neurons are computational units, which accept an input and produce an output. Weights determine the strength of connection between neurons. So the connection could be between input and a neuron, or it could be between a neuron and another neuron. Activation functions work on the weighted sum of inputs to a neuron and produce an output. Additional input to the neuron that allows a certain degree of flexibility is called as a bias. 07:27 Nikita: I think we’ve got the components of ANN straight but maybe you should give us an example. You mentioned this example earlier…of needing to train ANN to recognize handwritten digits from images. How would we go about that? Hemant: For that, we have to collect a large number of digit images, and we need to train ANN using these images. So, in this case, the images consist of 28 by 28 pixels which act as input layer. For the output, we have neurons-- 10 neurons which represent digits 0 to 9. And we have multiple hidden layers. So, in this case, we have two hidden layers which are consisting of 16 neurons each. The hidden layers are responsible for capturing the internal representation of the raw image data. And the output layer is responsible for producing the desired outcomes. So, in this case, the desired outcome is the prediction of whether the digit is 0 or 1 or up to digit 9. So how do we train this particular ANN? So the first thing we use the backpropagation algorithm. During training, we show an image to the ANN. Let us say it is an image of digit 2. So we expect output neuron for digit 2 to fire. But in real, let us say output neuron of a digit 6 fired. 09:12 Lois: So, then, what do we do? Hemant: We know that there is an error. So to correct an error, we adjust the weights of the connection between neurons based on a calculation, which we call as backpropagation algorithm. By showing thousands of images and adjusting the weights iteratively, ANN is able to predict correct outcome for most of the input images. This process of adjusting weights through backpropagation is called as model training. 09:48 Do you have an idea for a new course or learning opportunity? We’d love to hear it! Visit the Oracle University Learning Community and share your thoughts with us on the Idea Incubator. Your suggestion could find a place in future development projects! Visit mylearn.oracle.com to get started. 10:09 Nikita: Welcome back! Let’s move on to CNN. Hemant, what is a Convolutional Neural Network? Hemant: CNN is a type of deep learning model specifically designed for processing and analyzing grid-like data, such as images and videos. In the ANN, the input image is converted to a single dimensional array and given as an input to the network. But that does not work well with the image data because image data is inherently two dimensional. CNN works better with two dimensional data. The role of the CNN is to reduce the image into a form, which is easier to process and without losing features, which are critical for getting a good prediction. 10:53 Lois: A CNN has different layers, right? Could you tell us a bit about them? Hemant: The first one is input layer. Input layer is followed by feature extraction layers, which is a combination and repetition of multiple feature extraction layers, including convolutional layer with ReLu activation and a pooling layer. And this is followed by a classification layer. These are the fully connected output layers, where the classification occurs as output classes. The feature extraction layers play a vital role in image classification. 11:33 Nikita: Can you expl
What is MySQL HeatWave? How do I get certified in it? Where do I start? Listen to Lois Houston and Nikita Abraham, along with MySQL Developer Scott Stroz, answer all these questions and more on this week's episode of the Oracle University Podcast. MySQL Document Store: https://oracleuniversitypodcast.libsyn.com/mysql-document-store Oracle MyLearn: https://mylearn.oracle.com/ Oracle University Learning Community: https://education.oracle.com/ou-community LinkedIn: https://www.linkedin.com/showcase/oracle-university/ X (formerly Twitter): https://twitter.com/Oracle_Edu Special thanks to Arijit Ghosh, David Wright, and the OU Studio Team for helping us create this episode. -------------------------------------------------------- Episode Transcript: 00:00 Welcome to the Oracle University Podcast, the first stop on your cloud journey. During this series of informative podcasts, we’ll bring you foundational training on the most popular Oracle technologies. Let’s get started! 00:26 Nikita: Welcome to the Oracle University Podcast! I’m Nikita Abraham, Principal Technical Editor with Oracle University, and with me is Lois Houston, Director of Innovation Programs. Lois: Hi there! For the last two weeks, we’ve been having really exciting discussions on everything AI. We covered the basics of artificial intelligence and machine learning, and we’re taking a short break from that today to talk about the new MySQL HeatWave Implementation Associate Certification with MySQL Developer Advocate Scott Stroz. 00:59 Nikita: You may remember Scott from an episode last year where he came on to discuss MySQL Document Store. We’ll post the link to that episode in the show notes so you can listen to it if you haven’t already. Lois: Hi Scott! Thanks for joining us again. Before diving into the certification, tell us, what is MySQL HeatWave? 01:19 Scott: Hi Lois, Hi Niki. I’m so glad to be back. So, MySQL HeatWave Database Service is a fully managed database that is capable of running transactional and analytic queries in a single database instance. This can be done across data warehouses and data lakes. We get all the benefits of analytic queries without the latency and potential security issues of performing standard extract, transform, and load, or ETL, operations. Some other MySQL HeatWave database service features are automated system updates and database backups, high availability, in-database machine learning with AutoML, MySQL Autopilot for managing instance provisioning, and enhanced data security. HeatWave is the only cloud database service running MySQL that is built, managed, and supported by the MySQL Engineering team. 02:14 Lois: And where can I find MySQL HeatWave? Scott: MySQL HeatWave is only available in the cloud. MySQL HeatWave instances can be provisioned in Oracle Cloud Infrastructure or OCI, Amazon Web Services (AWS), and Microsoft Azure. Now, some features though are only available in Oracle Cloud, such as access to MySQL Document Store. 02:36 Nikita: Scott, you said MySQL HeatWave runs transactional and analytic queries in a single instance. Can you elaborate on that? Scott: Sure, Niki. So, MySQL HeatWave allows developers, database administrators, and data analysts to run transactional queries (OLTP) and analytic queries (OLAP). OLTP, or online transaction processing, allows for real-time execution of database transactions. A transaction is any kind of insertion, deletion, update, or query of data. Most DBAs and developers work with this kind of processing in their day-to-day activities. OLAP, or online analytical processing, is one way to handle multi-dimensional analytical queries typically used for reporting or data analytics. OLTP system data must typically be exported, aggregated, and imported into an OLAP system. This procedure is called ETL as I mentioned – extract, transform, and load. With large datasets, ETL processes can take a long time to complete, so analytic data could be “old” by the time it is available in an OLAP system. There is also an increased security risk in moving the data to an external source. 03:56 Scott: MySQL HeatWave eliminates the need for time-consuming ETL processes. We can actually get real-time analytics from our data since HeatWave allows for OLTP and OLAP in a single instance. I should note, this also includes analytic from JSON data that may be stored in the database. Another advantage is that applications can use MySQL HeatWave without changing any of the application code. Developers only need to point their applications at the MySQL HeatWave databases. MySQL HeatWave is fully compatible with on-premise MySQL instances, which can allow for a seamless transition to the cloud. And one other thing. When MySQL HeatWave has OLAP features enabled, MySQL can determine what type of query is being executed and route it to either the normal database system or the in-memory database. 04:52 Lois: That’s so cool! And what about the other features you mentioned, Scott? Automated updates and backups, high availability… Scott: Right, Lois. But before that, I want to tell you about the in-memory query accelerator. MySQL HeatWave offers a massively parallel, in-memory hybrid columnar query processing engine. It provides high performance by utilizing algorithms for distributed query processing. And this query processing in MySQL HeatWave is optimized for cloud environments. MySQL HeatWave can be configured to automatically apply system updates, so you will always have the latest and greatest version of MySQL. Then, we have automated backups. By this, I mean MySQL HeatWave can be configured to provide automated backups with point-in-time recovery to ensure data can be restored to a particular date and time. MySQL HeatWave also allows us to define a retention plan for our database backups, that means how long we keep the backups before they are deleted. High availability with MySQL HeatWave allows for more consistent uptime. When using high availability, MySQL HeatWave instances can be provisioned across multiple availability domains, providing automatic failover for when the primary node becomes unavailable. All availability domains within a region are physically separated from each other to mitigate the possibility of a single point of failure. 06:14 Scott: We also have MySQL Lakehouse. Lakehouse allows for the querying of data stored in object storage in various formats. This can be CSV, Parquet, Avro, or an export format from other database systems. And basically, we point Lakehouse at data stored in Oracle Cloud, and once it’s ingested, the data can be queried just like any other data in a database. Lakehouse supports querying data up to half a petabyte in size using the HeatWave engine. And this allows users to take advantage of HeatWave for non-MySQL workloads. MySQL AutoPilot is a part of MySQL HeatWave and can be used to predict the number of HeatWave nodes a system will need and automatically provision them as part of a cluster. AutoPilot has features that can handle automatic thread pooling and database shape predicting. A “shape” is one of the many different CPU, memory, and ethernet traffic configurations available for MySQL HeatWave. MySQL HeatWave includes some advanced security features such as asymmetric encryption and automated data masking at query execution. As you can see, there are a lot of features covered under the HeatWave umbrella! 07:31 Did you know that Oracle University offers free courses on Oracle Cloud Infrastructure? You’ll find training on everything from cloud computing, database, and security to artificial intelligence and machine learning, all free to subscribers. So, what are you waiting for? Pick a topic, leverage the Oracle University Learning Community to ask questions, and then sit for your certification. Visit mylearn.oracle.com to get started. 08:02 Nikita: Welcome back! Now coming to the certification, who can actually take this exam, Scott? Scott: The MySQL HeatWave Implementation Associate Certification Exam is designed specifically for administrators and data scientists who want to provision, configure, and manage MySQL HeatWave for transactions, analytics, machine learning, and Lakehouse. 08:22 Nikita: Can someone who’s just graduated, say an engineering graduate interested in data analytics, take this certification? Are there any prerequisites? What are the career prospects for them? Scott: There are no mandatory prerequisites, but anyone who wants to take the exam should have experience with MySQL HeatWave and other aspects of OCI, such as virtual cloud networks and identity and security processes. Also, the learning path on MyLearn will be extremely helpful when preparing for the exam, but you are not required to complete the learning path before registering for the exam. The exam focuses more on getting MySQL HeatWave running (and keeping it running) than accessing the data. That doesn’t mean it is not helpful for someone interested in data analytics. I think it can be helpful for data analysts to understand how the system providing the data functions, even if it is at just a high level. It is also possible that data analysts might be responsible for setting up their own systems and importing and managing their own data. 09:23 Lois: And how do I get started if I want to get certified on MySQL HeatWave? Scott: So, you’ll first need to go to mylearn.oracle.com and look for the “Become a MySQL HeatWave Implementation Associate” learning path. The learning path consists of over 10 hours of training across 8 different courses. These courses include “Getting Started with MySQL HeatWave Database Service,” which offers an introduction to some Oracle Cloud functionality such as security and networking, as well as showing one way to connect to a MySQL HeatWave instance. Another course demonstrates how to configure MySQL instances and copy that configuration to other instances. Other courses cover how to migrate data into MySQL HeatWave, set
Does machine learning feel like too convoluted a topic? Not anymore! Listen to hosts Lois Houston and Nikita Abraham, along with Senior Principal OCI Instructor Hemant Gahankari, talk about foundational machine learning concepts and dive into how supervised learning, unsupervised learning, and reinforcement learning work. Oracle MyLearn: https://mylearn.oracle.com/ Oracle University Learning Community: https://education.oracle.com/ou-community LinkedIn: https://www.linkedin.com/showcase/oracle-university/ X (formerly Twitter): https://twitter.com/Oracle_Edu Special thanks to Arijit Ghosh, David Wright, Himanshu Raj, and the OU Studio Team for helping us create this episode. --------------------------------------------------------- Episode Transcript: 00:00 Welcome to the Oracle University Podcast, the first stop on your cloud journey. During this series of informative podcasts, we’ll bring you foundational training on the most popular Oracle technologies. Let’s get started! 00:26 Lois: Hello and welcome to the Oracle University Podcast. I’m Lois Houston, Director of Innovation Programs with Oracle University, and with me is Nikita Abraham, Principal Technical Editor. Nikita: Hi everyone! Last week, we went through the basics of artificial intelligence and we’re going to take it a step further today by talking about some foundational machine learning concepts. After that, we’ll discuss the three main types of machine learning models: supervised learning, unsupervised learning, and reinforcement learning. 00:57 Lois: Hemant Gahankari, a Senior Principal OCI Instructor, joins us for this episode. Hi Hemant! Let’s dive right in. What is machine learning? How does it work? Hemant: Machine learning is a subset of artificial intelligence that focuses on creating computer systems that can learn and predict outcomes from given examples without being explicitly programmed. It is powered by algorithms that incorporate intelligence into machines by automatically learning from a set of examples usually provided as data. 01:34 Nikita: Give us a few examples of machine learning… so we can see what it can do for us. Hemant: Machine learning is used by all of us in our day-to-day life. When we shop online, we get product recommendations based on our preferences and our shopping history. This is powered by machine learning. We are notified about movies recommendations based on our viewing history and choices of other similar viewers. This too is driven by machine learning. While browsing emails, we are warned of a spam mail because machine learning classifies whether the mail is spam or not based on its content. In the increasingly popular self-driving cars, machine learning is responsible for taking the car to its destination. 02:24 Lois: So, how does machine learning actually work? Hemant: Let us say we have a computer and we need to teach the computer to differentiate between a cat and a dog. We do this by describing features of a cat or a dog. Dogs and cats have distinguishing features. For example, the body color, texture, eye color are some of the defining features which can be used to differentiate a cat from a dog. These are collectively called as input data. We also provide a corresponding output, which is called as a label, which can be a dog or a cat in this case. By describing a specific set of features, we can say that it is a cat or a dog. Machine learning model is first trained with the data set. Training data set consists of a set of features and output labels, and is given as an input to the machine learning model. During the process of training, machine learning model learns the relation between input features and corresponding output labels from the provided data. Once the model learns from the data, we have a trained model. Once the model is trained, it can be used for inference. Inference is a process of getting a prediction by giving a data point. In this example, we input features of a cat or a dog, and the trained model predicts the output that is a cat or a dog label. The types of machine learning models depend on whether we have a labeled output or not. 04:08 Nikita: Oh, there are different types of machine learning models? Hemant: In general, there are three types of machine learning approaches. In supervised machine learning, labeled data is used to train the model. Model learns the relation between features and labels. Unsupervised learning is generally used to understand relationships within a data set. Labels are not used or are not available. Reinforcement learning uses algorithms that learn from outcomes to make decisions or choices. 04:45 Lois: Ok…supervised learning, unsupervised learning, and reinforcement learning. Where do we use each of these machine learning models? Hemant: Some of the popular applications of supervised machine learning are disease detection, weather forecasting, stock price prediction, spam detection, and credit scoring. For example, in disease detection, the patient data is input to a machine learning model, and machine learning model predicts if a patient is suffering from a disease or not. For unsupervised machine learning, some of the most common real-time applications are to detect fraudulent transactions, customer segmentation, outlier detection, and targeted marketing campaigns. So for example, given the transaction data, we can look for patterns that lead to fraudulent transactions. Most popular among reinforcement learning applications are automated robots, autonomous driving cars, and playing games. 05:51 Nikita: I want to get into how each type of machine learning works. Can we start with supervised learning? Hemant: Supervised learning is a machine learning model that learns from labeled data. The model learns the mapping between the input and the output. As a house price predictor model, we input house size in square feet and model predicts the price of a house. Suppose we need to develop a machine learning model for detecting cancer, the input to the model would be the person's medical details, the output would be whether the tumor is malignant or not. 06:29 Lois: So, that mapping between the input and output is fundamental in supervised learning. Hemant: Supervised learning is similar to a teacher teaching student. The model is trained with the past outcomes and it learns the relationship or mapping between the input and output. In supervised machine learning model, the outputs can be either categorical or continuous. When the output is continuous, we use regression. And when the output is categorical, we use classification. 07:05 Lois: We want to keep this discussion at a high level, so we’re not going to get into regression and classification. But if you want to learn more about these concepts and look at some demonstrations, visit mylearn.oracle.com. Nikita: Yeah, look for the Oracle Cloud Infrastructure AI Foundations course and you’ll find a lot of resources that you can make use of. 07:30 The Oracle University Learning Community is an excellent place to collaborate and learn with Oracle experts and fellow learners. Grow your skills, inspire innovation, and celebrate your successes. All your activities, from liking a post to answering questions and sharing with others, will help you earn a valuable reputation, badges, and ranks to be recognized in the community. Visit mylearn.oracle.com to get started. 07:58 Nikita: Welcome back! So that was supervised machine learning. What about unsupervised machine learning, Hemant? Hemant: Unsupervised machine learning is a type of machine learning where there are no labeled outputs. The algorithm learns the patterns and relationships in the data and groups similar data items. In unsupervised machine learning, the patterns in the data are explored explicitly without being told what to look for. For example, if you give a set of different-colored LEGO pieces to a child and ask to sort it, it may the LEGO pieces based on any patterns they observe. It could be based on same color or same size or same type. Similarly, in unsupervised learning, we group unlabeled data sets. One more example could be-- say, imagine you have a basket of various fruits-- say, apples, bananas, and oranges-- and your task is to group these fruits based on their similarities. You observe that some fruits are round and red, while others are elongated and yellow. Without being told explicitly, you decide to group the round and red fruits together as one cluster and the elongated and yellow fruits as another cluster. There you go. You have just performed an unsupervised learning task. 09:21 Lois: Where is unsupervised machine learning used? Can you take us through some use cases? Hemant: The first use case of unsupervised machine learning is market segmentation. In market segmentation, one example is providing the purchasing details of an online shop to a clustering algorithm. Based on the items purchased and purchasing behavior, the clustering algorithm can identify customers based on the similarity between the products purchased. For example, customers with a particular age group who buy protein diet products can be shown an advertisement of sports-related products. The second use case is on outlier analysis. One typical example for outlier analysis is to provide credit card purchase data for clustering. Fraudulent transactions can be detected by a bank by using outliers. In some transaction, amounts are too high or recurring. It signifies an outlier. The third use case is recommendation systems. An example for recommendation systems is to provide users' movie viewing history as input to a clustering algorithm. It clusters users based on the type or rating of movies they have watched. The output helps to provide personalized movie recommendations to users. The same applies for music recommendations also. 10:53 Lois: An
You probably interact with artificial intelligence (AI) more than you realize. So, there’s never been a better time to start figuring out how it all works. Join Lois Houston and Nikita Abraham as they decode the fundamentals of AI so that anyone, irrespective of their technical background, can leverage the benefits of AI and tap into its infinite potential. Together with Senior Cloud Engineer Nick Commisso, they take you through key AI concepts, common AI tasks and domains, and the primary differences between AI, machine learning, and deep learning. Oracle MyLearn: https://mylearn.oracle.com/ Oracle University Learning Community: https://education.oracle.com/ou-community LinkedIn: https://www.linkedin.com/showcase/oracle-university/ X (formerly Twitter): https://twitter.com/Oracle_Edu Special thanks to Arijit Ghosh, David Wright, Himanshu Raj, and the OU Studio Team for helping us create this episode. -------------------------------------------------------- Episode Transcript 00:00 Welcome to the Oracle University Podcast, the first stop on your cloud journey. During this series of informative podcasts, we’ll bring you foundational training on the most popular Oracle technologies. Let’s get started! 00:26 Nikita: Hello and welcome to the Oracle University Podcast. I’m Nikita Abraham, Principal Technical Editor with Oracle University, and with me is Lois Houston, Director of Innovation Programs. Lois: Hi there! Welcome to a new season of the Oracle University Podcast. I’m so excited about this season because we’re going to delve into the world of artificial intelligence. In upcoming episodes, we’ll talk about the fundamentals of artificial intelligence and machine learning. And we’ll discuss neural network architectures, generative AI and large language models, the OCI AI stack, and OCI AI services. 01:06 Nikita: So, if you’re an IT professional who wants to start learning about AI and ML or even if you’re a student who is familiar with OCI or similar cloud services, but have no prior exposure to this field, you’ll want to tune in to these episodes. Lois: That’s right, Niki. So, let’s get started. Today, we’ll talk about the basics of artificial intelligence with Senior Cloud Engineer Nick Commisso. Hi Nick! Thanks for joining us today. So, let’s start right at the beginning. What is artificial intelligence? 01:36 Nick: Well, the ability of machines to imitate the cognitive abilities and problem solving capabilities of human intelligence can be classified as artificial intelligence or AI. 01:47 Nikita: Now, when you say capabilities and abilities, what are you referring to? Nick: Human intelligence is the intellectual capability of humans that allows us to learn new skills through observation and mental digestion, to think through and understand abstract concepts and apply reasoning, to communicate using a language and understand the nonverbal cues, such as facial recognition, tone variation, and body language. You can handle objections in real time, even in a complex setting. You can plan for short and long-term situations or projects. And, of course, you can create music and art or invent something new like an original idea. If you can replicate any of these human capabilities in machines, this is artificial general intelligence or AGI. So in other words, AGI can mimic human sensory and motor skills, performance, learning, and intelligence, and use these abilities to carry out complicated tasks without human intervention. When we apply AGI to solve problems with specific and narrow objectives, we call it artificial intelligence or AI. 02:55 Lois: It seems like AI is everywhere, Nick. Can you give us some examples of where AI is used? Nick: AI is all around us, and you've probably interacted with AI, even if you didn't realize it. Some examples of AI can be viewing an image or an object and identifying if that is an apple or an orange. It could be examining an email and classifying it spam or not. It could be writing computer language code or predicting the price of an older car. So let's get into some more specifics of AI tasks and the nature of related data. Machine learning, deep learning, and data science are all associated with AI, and it can be confusing to distinguish. 03:36 Nikita: Why do we need AI? Why’s it important? Nick: AI is vital in today's world, and with the amount of data that's generated, it far exceeds the human ability to absorb, interpret, and actually make decisions based on that data. That's where AI comes in handy by enhancing the speed and effectiveness of human efforts. So here are two major reasons why we need AI. Number one, we want to eliminate or reduce the amount of routine tasks, and businesses have a lot of routine tasks that need to be done in large numbers. So things like approving a credit card or a bank loan, processing an insurance claim, recommending products to customers are just some example of routine tasks that can be handled. And second, we, as humans, need a smart friend who can create stories and poems, designs, create code and music, and have humor, just like us. 04:33 Lois: I’m onboard with getting help from a smart friend! There are different domains in AI, right, Nick? Nick: We have language for language translation; vision, like image classification; speech, like text to speech; product recommendations that can help you cross-sell products; anomaly detection, like detecting fraudulent transactions; learning by reward, like self-driven cars. You have forecasting with weather forecasting. And, of course, generating content like image from text. 05:03 Lois: There are so many applications. Nick, can you tell us more about these commonly used AI domains like language, audio, speech, and vision? Nick: Language-related AI tasks can be text related or generative AI. Text-related AI tasks use text as input, and the output can vary depending on the task. Some examples include detecting language, extracting entities in a text, or extracting key phrases and so on. Consider the example of translating text. There's many text translation tools where you simply type or paste your text into a given text box, choose your source and target language, and then click translate. Now, let's look at the generative AI tasks. They are generative, which means the output text is generated by a model. Some examples are creating text like stories or poems, summarizing a text, answering questions, and so on. Let's take the example of ChatGPT, the most well-known generative chat bot. These bots can create responses from their training on large language models, and they continuously grow through machine learning. 06:10 Nikita: What can you tell us about using text as data? Nick: Text is inherently sequential, and text consists of sentences. Sentences can have multiple words, and those words need to be converted to numbers for it to be used to train language models. This is called tokenization. Now, the length of sentences can vary, and all the sentences lengths need to be made equal. This is done through padding. Words can have similarities with other words, and sentences can also be similar to other sentences. The similarity can be measured through dot similarity or cosine similarity. We need a way to indicate that similar words or sentences may be close by. This is done through representation called embedding. 06:56 Nikita: And what about language AI models? Nick: Language AI models refer to artificial intelligence models that are specifically designed to understand, process, and generate natural language. These models have been trained on vast amounts of textual data that can perform various natural language processing or NLP tasks. The task that needs to be performed decides the type of input and output. The deep learning model architectures that are typically used to train models that perform language tasks are recurrent neural networks, which processes data sequentially and stores hidden states, long short-term memory, which processes data sequentially that can retain the context better through the use of gates, and transformers, which processes data in parallel. It uses the concept of self-attention to better understand the context. 07:48 Lois: And then there’s speech-related AI, right? Nick: Speech-related AI tasks can be either audio related or generative AI. Speech-related AI tasks use audio or speech as input, and the output can vary depending on the task. For example, speech-to-text conversion or speaker recognition, voice conversion, and so on. Generative AI tasks are generative in nature, so the output audio is generated by a model. For example, you have music composition and speech synthesis. Audio or speech is digitized as snapshots taken in time. The sample rate is the number of times in a second an audio sample is taken. Most digital audio have a sampling rate of 44.1 kilohertz, which is also the sampling rate for audio CDs. Multiple samples need to be correlated to make sense of the data. For example, listening to a song for a fraction of a second, you won't be able to infer much about the song, and you'll probably need to listen to it a little bit longer. Audio and speech AI models are designed to process and understand audio data, including spoken language. These deep-learning model architectures are used to train models that perform language with tasks-- recurrent neural networks, long short-term memory, transformers, variational autoencoders, waveform models, and Siamese networks. All of the models take into consideration the sequential nature of audio. 09:21 Did you know that Oracle University offers free courses on Oracle Cloud Infrastructure? You’ll find training on everything from cloud computing, database, and security to artificial intelligence and machine learning, all free to subscribers. So, what are you waiting for? Pick a topic, leverage the Oracle University Learning Community to ask questions, and then sit for yo
How do I get certified in Oracle Autonomous Database? What material can I use to prepare for it? What's the exam like? How long is the certification valid for? If these questions have been keeping you up at night, then join Lois Houston and Nikita Abraham in their conversation with Senior Principal OCI Instructor Susan Jang to understand the process of getting certified and begin your learning adventure. Oracle MyLearn: mylearn.oracle.com/ Oracle University Learning Community: education.oracle.com/ou-community LinkedIn: linkedin.com/showcase/oracle-university/ X (formerly Twitter): twitter.com/Oracle_Edu Special thanks to Arijit Ghosh, David Wright, and the OU Studio Team for helping us create this episode. -------------------------------------------------------- Episode Transcript 00:00 Welcome to the Oracle University Podcast, the first stop on your cloud journey. During this series of informative podcasts, we’ll bring you foundational training on the most popular Oracle technologies. Let’s get started! 00:26 Lois: Hello and welcome to the Oracle University Podcast. I’m Lois Houston, Director of Innovation Programs with Oracle University, and with me is Nikita Abraham, Principal Technical Editor. Nikita: Hi everyone! If you’ve listened to us these last few weeks, you’ll know we’ve been discussing Oracle Autonomous Database in detail. We looked at Autonomous Database on serverless and dedicated infrastructure. 00:51 Lois: That’s right, Niki. Then, last week, we explored Autonomous Database tools. Today, we thought we’d wrap up our focus on Autonomous Database by talking about the training offered by Oracle University, the associated certification, how to prepare for it, what you should do next, and more. Nikita: Yeah, we’ll get answers to all the big questions. And we’re going to get them from Susan Jang. Sue is a Senior Principal OCI Instructor with Oracle University. She has created and delivered training in Oracle databases and Oracle Cloud Infrastructure for over 20 years. Hi Sue! Thanks for joining us today. Sue: Happy to be here! 01:29 Lois: Sue, what training does Oracle have on Autonomous Database? Sue: Oracle University offers a professional-level course called the Oracle Autonomous Database Administration Workshop. So, if you want to learn to deploy and administer autonomous databases, this is the one for you. You’ll explore the fundamentals of the autonomous databases, their features, and benefits. You’ll learn about the technical architecture, the tasks that are involved in creating an autonomous database on a shared and on a dedicated Exadata infrastructure. You’ll discover what is the Machine Learning, you’ll discover what is APEX, which is Application Express, and SQL Developer Web, which is all deployed with the Autonomous Database. So basically everything you need to take your skills to the next level and become a proficient database administrator is in this course. 02:28 Nikita: Who can take this course, Sue? Sue: The course is really for anyone interested in Oracle Autonomous Database, whether you’re a database administrator, a cloud data management professional, or a consultant. The topics in the course include everything from the features of an Autonomous Database through provisioning, managing, and monitor of the database. Most people think that just because it is an Autonomous Database, Oracle will do everything for you, and there is nothing a DBA can do or needs to do. But that’s not true. An Oracle Autonomous Database automates the day-to-day DBA tasks, like tuning the database to ensure it is running at performance level or that the backups are done successfully. By letting the Autonomous Database perform those tasks, it gives the database administrator time to fully understand the new features of an Oracle database and figure out how to implement the features that will benefit the DBA’s company. 03:30 Lois: Would a non-database administrator benefit from taking this course? Sue: Yes, Lois. Oracle courses are designed in modules, so you can focus on the modules that meet your needs. For example, if you’re a senior technical manager, you may not need to manage and monitor the Autonomous Database. But still, it’s important to understand its features and architecture to know how other Oracle products integrate with the database. 03:57 Nikita: Right. Talking about the course itself, each module consists of videos that teach different concepts, right? Sue: Yes, Niki. Each video covers one topic. A group of topics, or I should say a group of related topics, makes up a module. We know your time is important to you, and your success is important to us. You don’t just want to spend time taking training. You want to know that you’re really understanding the concepts of what you are learning. So to help you do this, we have skill checks at the end of most modules. You must successfully answer 80% of the questions to pass these knowledge checks. These checks are an excellent way to ensure that you’re on the right track and have the understanding of each module before you move on to the next one. 04:48 Lois: That’s great. And are there any other resources to help reinforce what’s been learned? Sue: I grew up with this phrase from my Mom. Education was her career. I remember hearing, “I hear and I forget. I see and I remember. I do and I understand.” It’s important to us that you understand the concepts and can actually “do” or “perform” the tasks. You'll find several demos in the different modules of the Autonomous Database Administration Workshop. These videos are where the instructor shows you how to perform the tasks so you can reinforce what you learned in the lessons. You’ll find demos on provisioning an autonomous database, creating an autonomous database clone, and configuring disaster recovery, and lots more. Oracle also has what we call LiveLabs. These are a series of hands-on tutorials with step-by-step instructions to guide you through performing the tasks. 05:49 Nikita: I love the idea of LiveLabs. You can follow instructions on how to perform administrative tasks and then practice doing that on your own. Lois: Yeah, that’s fantastic. OK Sue, say I’ve taken the course. What do I do next? Sue: Well, after you’ve taken the course, you’ll want to demonstrate your expertise with a certification. Because you want to get that better job. You want to increase your earning potential. You need to take the certification called the Oracle Autonomous Database Cloud Professional. We have a couple of resources to help you along the way to ensure you succeed in securing that certification. In MyLearn, the Oracle University online learning platform, you’ll see that the course, Oracle Autonomous Database Administration Workshop, falls within a learning path called Become an Oracle Autonomous Database Cloud Professional. The course is the first section of this learning path. The next section is a video describing the certification exam and how to prepare for it. The section after that is a practice exam. Now, though it doesn’t have the actual questions, you’ll find the exam will give you a good idea of the type of questions that will be asked in the exam. 07:10 Lois: OK, so now I’ve done all that, and I’m ready to validate my knowledge and expertise. Tell me more about the certification, Sue. Sue: To get the certification, you must take an online exam. The duration of the exam is 90 minutes. It’s a Multiple Choice format, and there are 60 questions to the exam. By getting this certification, you’re demonstrating to the world that you have the knowledge to provision, manage, and monitor, as well as migrate workloads to the Autonomous Database, on both a shared as well as a dedicated Exadata infrastructure. You will show you have the understanding of the architect of the Autonomous Database and can successfully use the features as well as its workflow, and you are capable of using Autonomous Database tools in developing an Autonomous Database. 08:05 Nikita: Great! So what do I need to do to take the exam? Sue: We assume you’ve already taken the course (making sure that you’re up to date with the training), that you’ve taken the time to study the topics in depth rather than memorizing superficial information just to pass the exam, looked at the available preparation material, and you’ve also taken the practice exam. I highly recommend that you have the hands-on experience or practice on an Autonomous Database before you take the certification exam. 08:38 Nikita: Hold on, Sue. You said to make sure we’re up to date with the training. How do I do that? Sue: Technology is ever-changing, and at Oracle, we continually enhance our products to provide features that make them faster or more straightforward to use. So, if you’re taking a course, you may find a small tag that says “New” next to a topic. That indicates that there are some new training that’s been added to the course. So what I’m trying to say is if you’re looking to take some certification, check the course before you register for the exam and to see if there are any “New” tags. If you find them, you can learn what’s new and not have to go through the entire course again. This way, you’re up to date with the training! 09:25 Nikita: Ok. Got it. Tell us more about the certification, Sue. Sue: If you’re ready, search for the Become An Oracle Autonomous Database Cloud Professional learning path in MyLearn and scroll down to the Oracle Database Cloud Professional exam. Click on the “Register Now” button. You’ll be taken to a page where you’ll see the exam overview, the resources to help you prepare for the exam, a button to register for the exam, and things to do before your exam session. It will also describe what happens after the exam and some exam policies, like what to do if you need to reschedule your exam. When you’re ready to take the exam, you can schedule the date and time according to when it’s convenient f
In this episode, hosts Lois Houston and Nikita Abraham speak with Oracle Database experts about the various tools you can use with Autonomous Database, including Oracle Application Express (APEX), Oracle Machine Learning, and more. Oracle MyLearn: https://mylearn.oracle.com/ Oracle University Learning Community: https://education.oracle.com/ou-community LinkedIn: https://www.linkedin.com/showcase/oracle-university/ X (formerly Twitter): https://twitter.com/Oracle_Edu Special thanks to Arijit Ghosh, David Wright, Tamal Chatterjee, and the OU Studio Team for helping us create this episode. --------------------------------------------------------- Episode Transcript: 00:00 Welcome to the Oracle University Podcast, the first stop on your cloud journey. During this series of informative podcasts, we’ll bring you foundational training on the most popular Oracle technologies. Let’s get started! 00:26 Lois: Hello and welcome to the Oracle University Podcast. I’m Lois Houston, Director of Innovation Programs with Oracle University, and with me is Nikita Abraham, Principal Technical Editor. Nikita: Hi everyone! We spent the last two episodes exploring Oracle Autonomous Database’s deployment options: Serverless and Dedicated. Today, it’s tool time! Lois: That’s right, Niki. We’ll be chatting with some of our Database experts on the tools that you can use with the Autonomous Database. We’re going to hear from Patrick Wheeler, Kay Malcolm, Sangeetha Kuppuswamy, and Thea Lazarova. Nikita: First up, we have Patrick, to take us through two important tools. Patrick, let’s start with Oracle Application Express. What is it and how does it help developers? 01:15 Patrick: Oracle Application Express, also known as APEX-- or perhaps APEX, we're flexible like that-- is a low-code development platform that enables you to build scalable, secure, enterprise apps with world-class features that can be deployed anywhere. Using APEX, developers can quickly develop and deploy compelling apps that solve real problems and provide immediate value. You don't need to be an expert in a vast array of technologies to deliver sophisticated solutions. Focus on solving the problem, and let APEX take care of the rest. 01:52 Lois: I love that it’s so easy to use. OK, so how does Oracle APEX integrate with Oracle Database? What are the benefits of using APEX on Autonomous Database? Patrick: Oracle APEX is a fully supported, no-cost feature of Oracle Database. If you have Oracle Database, you already have Oracle APEX. You can access APEX from database actions. Oracle APEX on Autonomous Database provides a preconfigured, fully managed, and secure environment to both develop and deploy world-class applications. Oracle takes care of configuration, tuning, backups, patching, encryption, scaling, and more, leaving you free to focus on solving your business problems. APEX enables your organization to be more agile and develop solutions faster for less cost and with greater consistency. You can adapt to changing requirements with ease, and you can empower professional developers, citizen developers, and everyone else. 02:56 Nikita: So you really don’t need to have a lot of specializations or be an expert to use APEX. That’s so cool! Now, what are the steps involved in creating an application using APEX? Patrick: You will be prompted to log in as the administrator at first. Then, you may create workspaces for your respective users and log in with those associated credentials. Application Express provides you with an easy-to-use, browser-based environment to load data, manage database objects, develop REST interfaces, and build applications which look and run great on both desktop and mobile devices. You can use APEX to develop a wide variety of solutions, import spreadsheets, and develop a single source of truth in minutes. Create compelling data visualizations against your existing data, deploy productivity apps to elegantly solve a business need, or build your next mission-critical data management application. There are no limits on the number of developers or end users for your applications. 04:01 Lois: Patrick, how does APEX use SQL? What role does SQL play in the development of APEX applications? Patrick: APEX embraces SQL. Anything you can express with SQL can be easily employed in an APEX application. Application Express also enables low-code development, providing developers with powerful data management and data visualization components that deliver modern, responsive end user experiences out-of-the-box. Instead of writing code by hand, you're able to use intelligent wizards to guide you through the rapid creation of applications and components. Creating a new application from APEX App Builder is as easy as one, two, three. One, in App Builder, select a project name and appearance. Two, add pages and features to the app. Three, finalize settings, and click Create. 05:00 Nikita: OK. So, the other tool I want to ask you about is Oracle Machine Learning. What can you tell us about it, Patrick? Patrick: Oracle Machine Learning, or OML, is available with Autonomous Database. A new capability that we've introduced with Oracle Machine Learning is called Automatic Machine Learning, or AutoML. Its goal is to increase data scientist productivity while reducing overall compute time. In addition, AutoML enables non-experts to leverage machine learning by not requiring deep understanding of the algorithms and their settings. 05:37 Lois: And what are the key functions of AutoML? Patrick: AutoML consists of three main functions: Algorithm Selection, Feature Selection, and Model Tuning. With Automatic Algorithm Selection, the goal is to identify the in-database algorithms that are likely to achieve the highest model quality. Using metalearning, AutoML leverages machine learning itself to help find the best algorithm faster than with exhaustive search. With Automatic Feature Selection, the goal is to denoise data by eliminating features that don't add value to the model. By identifying the most predicted features and eliminating noise, model accuracy can often be significantly improved with a side benefit of faster model building and scoring. Automatic Model Tuning tunes algorithm hyperparameters, those parameters that determine the behavior of the algorithm, on the provided data. Auto Model Tuning can significantly improve model accuracy while avoiding manual or exhaustive search techniques, which can be costly both in terms of time and compute resources. 06:44 Lois: How does Oracle Machine Learning leverage the capabilities of Autonomous Database? Patrick: With Oracle Machine Learning, the full power of the database is accessible with the tremendous performance of parallel processing available, whether the machine learning algorithm is accessed via native database SQL or with OML4Py through Python or R. 07:07 Nikita: Patrick, talk to us about the Data Insights feature. How does it help analysts uncover hidden patterns and anomalies? Patrick: A feature I wanted to call the electromagnet, but they didn't let me. An analyst's job can often feel like looking for a needle in a haystack. So throw the switch and all that metallic stuff is going to slam up onto that electromagnet. Sure, there are going to be rusty old nails and screws and nuts and bolts, but there are going to be a few needles as well. It's far easier to pick the needles out of these few bits of metal than go rummaging around in a pile of hay, especially if you have allergies. That's more or less how our Insights tool works. Load your data, kick off a query, and grab a cup of coffee. Autonomous Database does all the hard work, scouring through this data looking for hidden patterns, anomalies, and outliers. Essentially, we run some analytic queries that predict expected values. And where the actual values differ significantly from expectation, the tool presents them here. Some of these might be uninteresting or obvious, but some are worthy of further investigation. You get this dashboard of various exceptional data patterns. Drill down on a specific gauge in this dashboard and significant deviations between actual and expected values are highlighted. 08:28 Lois: What a useful feature! Thank you, Patrick. Now, let’s discuss some terms and concepts that are applicable to the Autonomous JSON Database with Kay. Hi Kay, what’s the main focus of the Autonomous JSON Database? How does it support developers in building NoSQL-style applications? Kay: Autonomous Database supports the JavaScript Object Notation, also known as JSON, natively in the database. It supports applications that use the SODA API to store and retrieve JSON data or SQL queries to store and retrieve data stored in JSON-formatted data. Oracle AJD is Oracle ATP, Autonomous Transaction Processing, but it's designed for developing NoSQL-style applications that use JSON documents. You can promote an AJD service to ATP. 09:22 Nikita: What makes the development of NoSQL-style, document-centric applications flexible on AJD? Kay: Development of these NoSQL-style, document-centric applications is particularly flexible because the applications use schemaless data. This lets you quickly react to changing application requirements. There's no need to normalize the data into relational tables and no impediment to changing the data structure or organization at any time, in any way. A JSON document has its own internal structure, but no relation is imposed on separate JSON documents. Nikita: What does AJD do for developers? How does it actually help them? Kay: So Autonomous JSON Database, or AJD, is designed for you, the developer, to allow you to use simple document APIs and develop applications without having to know anything about SQL. That's a win. But at the same time, it does give you the ability to create highly complex SQL-based queries for reporting and analysis purposes. It has built-in binary JSON storage type, which is extremely eff
The Oracle Autonomous Database Dedicated deployment is a good choice for customers who want to implement a private database cloud in their own dedicated Exadata infrastructure. That dedicated infrastructure can either be in the Oracle Public Cloud or in the customer's own data center via Oracle Exadata Cloud@Customer. In a dedicated environment, the Exadata infrastructure is entirely dedicated to the subscribing customer, isolated from other cloud tenants, with no shared processor, storage, and memory resource. In this episode, hosts Lois Houston and Nikita Abraham speak with Oracle Database experts about how Autonomous Database Dedicated offers greater control of the software and infrastructure life cycle, customizable policies for separation of database workload, software update schedules and versioning, workload consolidation, availability policies, and much more. Oracle MyLearn: https://mylearn.oracle.com/ Oracle University Learning Community: https://education.oracle.com/ou-community LinkedIn: https://www.linkedin.com/showcase/oracle-university/ X (formerly Twitter): https://twitter.com/Oracle_Edu Special thanks to Arijit Ghosh, David Wright, Tamal Chatterjee, and the OU Studio Team for helping us create this episode. ------------------------------------------------------- Episode Transcript: 00:00 Welcome to the Oracle University Podcast, the first stop on your cloud journey. During this series of informative podcasts, we’ll bring you foundational training on the most popular Oracle technologies. Let’s get started. 00:26 Nikita: Hello and welcome to the Oracle University Podcast. I’m Nikita Abraham, Principal Technical Editor with Oracle University, and I’m joined by Lois Houston, Director of Innovation Programs. Lois: Hi there! This is our second episode on Oracle’s Autonomous Database, and today we’re going to spend time discussing Autonomous Database on Dedicated Infrastructure. We’ll be talking with three of our colleagues: Maria Colgan, Kamryn Vinson, and Kay Malcolm. 00:53 Nikita: Maria is a Distinguished Product Manager for Oracle Database, Kamryn is a Database Product Manager, and Kay is a Senior Director of Database Product Management. Lois: Hi Maria! Thanks for joining us today. We know that Oracle Autonomous Database offers two deployment choices: serverless and dedicated Exadata infrastructure. We spoke about serverless infrastructure last week but for anyone who missed that episode, can you give us a quick recap of what it is? 01:22 Maria: With Autonomous Database Serverless, Oracle automates all aspects of the infrastructure and database management for you. That includes provisioning, configuring, monitoring, backing up, and tuning. You simply select what type of database you want, maybe a data warehouse, transaction processing, or a JSON document store, which region in the Oracle Public Cloud you want that database deployed, and the base compute and storage resources necessary. Oracle automatically takes care of everything else. Once provisioned, the database can be instantly scaled through our UI, our APIs, or automatically based on your workload needs. All scaling activities happen completely online while the database remains open for business. 02:11 Nikita: Ok, so now that we know what serverless is, let’s move on to dedicated infrastructure. What can you tell us about it? Maria: Autonomous Database Dedicated allows customers to implement a private database cloud running on their own dedicated Exadata infrastructure. That dedicated infrastructure can be in Oracle’s Public Cloud or in the customer's own data center via Oracle Exadata Cloud@Customer. It makes an ideal platform to consolidate multiple databases regardless of their workload type or their size. And it also allows you to offer database as a service within your enterprise. 02:50 Lois: What are the primary benefits of Autonomous Database Dedicated infrastructure? Maria: With the dedicated deployment option, you must first subscribe to Dedicated Exadata Cloud Infrastructure that is isolated from other tenants with no shared processors, memory, network, or storage resources. This infrastructure choice offers greater control of both the software and the infrastructure life cycle. Customers can specify their own policies for workload separation, software update schedules, and availability. One of the key benefits of an autonomous database is a lower total cost of ownership through more automation and operational delegation to Oracle. Remember it’s a fully managed service. All database operations, such as backup, software updates, upgrades, OS maintenance, incident management, and health monitoring, will be automatically done for you by Oracle. Its maximum availability architecture protects you from any hardware failures and in the event of a full outage, the service will be automatically failed over to your standby site. Built-in application continuity ensures zero downtime during the standard software update or in the event of a failover. 04:09 Nikita: And how is this billed? Maria: Autonomous Database also has true pay-per-use billing so even when autoscale is enabled, you’ll only pay for those additional resources when you use them. And we make it incredibly simple to develop on this environment with managed developer add-ons like our low code development environment, APEX, and our REST data services. This means you don’t need any additional development environments in order to get started with a new application. 04:40 Lois: Ok. So, it looks like the dedicated option offers more control and customization. Maria, how do we access a dedicated database over a network? Maria: The network path is through a VCN, or Virtual Cloud Network, and the subnet that's defined by the Exadata infrastructure hosting the database. By default, this subnet is defined as private, meaning, there's no public internet access to those databases. This ensures only your company can access your Exadata infrastructure and your databases. Autonomous Database Dedicated can also take advantage of network services provided by OCI, including subnets or VCN peering, as well as connections to on-prem databases through the IP secure VPN and FastConnect dedicated corporate network connections. 05:33 Maria: You can also take advantage of the Oracle Microsoft partnership that enables customers to connect their Oracle Cloud Infrastructure resources and Microsoft Azure resources through a dedicated private connection. However, for some customers, a move to the public cloud is just not possible. Perhaps it's due to industry regulations, performance concerns, or integration with legacy on-prem applications. For these types of customers, Exadata Cloud@Customer should meet their requirements for strict data sovereignty and security by delivering high-performance Exadata Cloud Services capabilities in their data center behind their own firewall. 06:16 Nikita: What are the benefits of Autonomous Database on Exadata Cloud@Customer? How’s it different? Maria: Autonomous Database on Exadata Cloud@Customer provides the same service as Autonomous Database Dedicated in the public cloud. So you get the same simplicity, agility, and performance, and elasticity that you get in the cloud. But it also provides a very fast and simple transition to an autonomous cloud because you can easily migrate on-prem databases to Exadata Cloud@Customer. Once the database is migrated, any existing applications can simply reconnect to that new database and run without any application changes being needed. And the data will leave your data center, so making it a very safe way to adopt a cloud model. 07:04 Lois: So, how do we manage communication to and from the public cloud? Maria: Each Cloud@Customer rack includes two local control plane servers to manage the communication to and from the public cloud. The local control plane acts on behalf of requests from the public cloud, keeping communications consolidated and secure. Platform control plane commands are sent to the Exadata Cloud@Customer system through a dedicated WebSocket secure tunnel. Oracle Cloud operations staff use that same tunnel to monitor the autonomous database on Exadata Cloud@Customer both for maintenance and for troubleshooting. The two remote, control plane servers installed in the Exadata Cloud@Customer rack host that secure tunnel endpoint and act as a gateway for access to the infrastructure. They also host components that orchestrate the cloud automation, aggregates and routes telemetry messages from the Exadata Cloud@Customer platform to the Oracle Support Service infrastructure. And they also host images for server patching. 08:13 Maria: The Exadata Database Server is connected to the customer-managed switches via either 10 gigabit or 25 gigabit Ethernet. Customers have access to the customer Virtual Machine, or VM, via a pair of layer 2 network connections that are implemented as Virtual Network Interface Cards, or vNICs. They're also tagged VLAN. The physical network connections are implemented for high availability in an active standby configuration. Autonomous Database on Exadata Cloud@Customer provides the best of both worlds-- all of the automation including patching, backing up, scaling, and management of a database that you get with a cloud service, but without the data ever leaving the customer's data center. 09:01 Nikita: That's interesting. And, what happens if a dedicated database loses network connectivity to the OCI control plane? Maria: In the event an autonomous database on Exadata Cloud@Customer loses network connectivity to the OCI control plane, the Autonomous Database will actually continue to be available for your applications. And operations such as backups and autoscaling will not be impacted in that loss of network connectivity. However, the management and monitoring of the Autonomous Database via the OCI console and APIs as well as access by the Oracle Cloud operations team will not