AI Model Collapse and the Dangers of AI-Generated Content
Digest
This episode of Tech Stuff delves into the issue of "model collapse" in AI, where AI models trained on data generated by other AI models can lead to a decline in accuracy and a loss of valuable information. The episode begins by drawing an analogy to a student writing a term paper using only other students' papers as sources, highlighting the potential for misinformation and lack of reliable citations. The episode then explores the concept of AI hallucinations, where AI models can produce incorrect or misleading information due to factors like pattern recognition and bias. The episode discusses how AI models can misinterpret patterns, leading to inaccurate results, and how human biases can be embedded in AI models through the training data. The episode then focuses on the issue of model collapse, where AI models trained on data generated by other AI models can lead to a degenerative process where models forget the true underlying data distribution. The episode highlights the potential for this issue to pollute the internet with inaccurate and nonsensical content, rendering it less useful. The episode concludes by emphasizing the importance of careful stewardship in AI model training, focusing on reputable sources and avoiding the use of garbage data to prevent model collapse and ensure the continued usefulness of AI.
Outlines
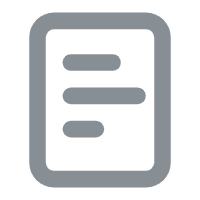
Introduction: The Feeling of Being Watched
This Chapter introduces the concept of "model collapse" in AI, drawing an analogy to a student writing a term paper using only other students' papers as sources, highlighting the potential for misinformation and lack of reliable citations.
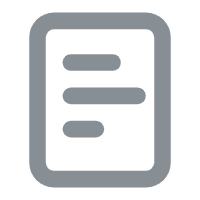
AI Hallucinations and Confabulations
This Chapter explores the concept of AI hallucinations, where AI models can produce incorrect or misleading information due to factors like pattern recognition and bias. The episode discusses how AI models can misinterpret patterns, leading to inaccurate results, and how human biases can be embedded in AI models through the training data.
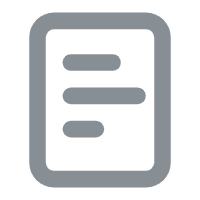
Model Collapse: The Curse of Recursion
This Chapter focuses on the issue of model collapse, where AI models trained on data generated by other AI models can lead to a degenerative process where models forget the true underlying data distribution. The episode highlights the potential for this issue to pollute the internet with inaccurate and nonsensical content, rendering it less useful.
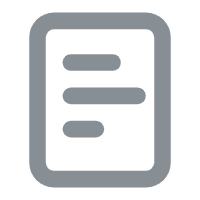
The Internet's Future: A Cluttered Mess?
This Chapter discusses the potential consequences of model collapse, where the internet could become filled with unreliable and nonsensical content generated by AI models, making it difficult to find accurate and valuable information.
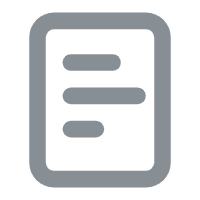
Stewardship and the Future of AI
This Chapter emphasizes the importance of careful stewardship in AI model training, focusing on reputable sources and avoiding the use of garbage data to prevent model collapse and ensure the continued usefulness of AI.
Keywords
Model Collapse
A phenomenon in AI where models trained on data generated by other AI models can lead to a decline in accuracy and a loss of valuable information. This occurs when AI models learn from data that is itself generated by AI, leading to a degenerative process where models forget the true underlying data distribution and produce increasingly inaccurate or nonsensical results.
AI Hallucinations
A phenomenon where AI models produce incorrect or misleading information, often due to factors like pattern recognition and bias. AI models can misinterpret patterns in data, leading to inaccurate conclusions, and human biases can be embedded in AI models through the training data, resulting in biased outputs.
Content Farms
Websites that produce a large volume of content, often using AI-generated content, in an effort to attract traffic from search engines. Content farms often prioritize quantity over quality, resulting in low-quality content that can be misleading or inaccurate.
Large Language Models (LLMs)
A type of AI model that is trained on massive amounts of text data and can generate human-like text, translate languages, write different kinds of creative content, and answer your questions in an informative way. LLMs are often used in applications like chatbots, search engines, and content generation.
Generative AI
A type of AI that can create new content, such as text, images, audio, and video. Generative AI models are trained on large datasets of existing content and can learn to generate new content that is similar to the training data. Generative AI is used in a wide range of applications, including art generation, music composition, and text writing.
Bias in AI
The tendency for AI models to reflect the biases present in the data they are trained on. This can lead to AI models making discriminatory or unfair decisions, as they may learn to associate certain groups of people with negative stereotypes. It is important to address bias in AI by ensuring that training data is diverse and representative of the real world.
Search Engine Optimization (SEO)
The process of optimizing a website to rank higher in search engine results pages (SERPs). SEO involves a variety of techniques, such as keyword research, content optimization, and link building, to improve a website's visibility and attract more organic traffic.
Fitzpatrick Skin Scale
A skin pigmentation metric used by dermatologists and researchers to classify skin color. The scale ranges from type 1 (very light skin) to type 6 (very dark skin). It is often used in research on skin cancer and other skin conditions.
Stable Diffusion
A generative AI platform that can create images from text descriptions. Stable Diffusion is known for its ability to generate high-quality images and its flexibility in creating different styles of images.
Parodolia
The tendency to perceive meaningful patterns in random or meaningless stimuli. This can lead to misinterpretations of images or sounds, such as seeing faces in clouds or hearing voices in static.
Q&A
What is "model collapse" in AI, and how does it occur?
Model collapse is a phenomenon where AI models trained on data generated by other AI models can lose accuracy and forget valuable information. This happens when AI models learn from data that is itself generated by AI, leading to a degenerative process where models forget the true underlying data distribution and produce increasingly inaccurate or nonsensical results.
What are AI hallucinations, and what factors contribute to them?
AI hallucinations occur when AI models produce incorrect or misleading information. This can be caused by factors like pattern recognition and bias. AI models can misinterpret patterns in data, leading to inaccurate conclusions, and human biases can be embedded in AI models through the training data, resulting in biased outputs.
How can content farms contribute to model collapse?
Content farms, which produce large volumes of content often using AI-generated content, can contribute to model collapse by polluting the internet with low-quality and inaccurate information. When AI models are trained on data from content farms, they may learn to produce similar low-quality content, further degrading the overall quality of information available online.
What are some potential consequences of model collapse?
Model collapse could lead to a future where the internet is filled with unreliable and nonsensical content generated by AI models, making it difficult to find accurate and valuable information. This could render the internet less useful and hinder our ability to access reliable knowledge.
How can we prevent model collapse and ensure the continued usefulness of AI?
To prevent model collapse, it is crucial to carefully curate the training data used for AI models. This involves focusing on reputable sources, avoiding the use of garbage data, and ensuring that training data is diverse and representative of the real world. By carefully guiding AI model training, we can minimize the risks of model collapse and ensure that AI continues to be a valuable tool for learning and innovation.
What are some examples of companies that have used AI to generate content, and what were the results?
CNET and HowStuffWorks are two examples of companies that have used AI to generate content. CNET faced criticism for not being transparent about its use of AI and for publishing articles with factual errors. HowStuffWorks laid off its human writers and editors after transitioning to AI-generated content, leading to protests from its editorial staff. These examples highlight the potential risks of relying solely on AI for content generation, as it can lead to a decline in quality and accuracy.
What is the role of human stewardship in the development of AI?
Human stewardship is crucial in the development of AI. It involves carefully guiding AI model training, ensuring that training data is accurate and representative, and addressing issues like bias and model collapse. By taking a responsible approach to AI development, we can ensure that AI remains a valuable tool for learning, innovation, and progress.
How can we ensure that AI models are not biased?
To minimize bias in AI models, it is essential to use diverse and representative training data. This involves ensuring that the data reflects the real world and includes individuals from different backgrounds, genders, ethnicities, and socioeconomic groups. Additionally, it is important to develop techniques for detecting and mitigating bias in AI models.
What are some of the ethical considerations surrounding the use of AI?
The use of AI raises a number of ethical considerations, including the potential for bias, the impact on employment, the spread of misinformation, and the potential for misuse. It is important to have open discussions about these issues and to develop ethical guidelines for the development and deployment of AI.
Show Notes
An AI image of a devious banker with way too many fingers can be entertaining, but could it also be a warning sign for the future of the Internet? We learn about some research that indicates future generative AI may be a real mess if it trains on other AI-generated content.
See omnystudio.com/listener for privacy information.